Jump to a key chapter
Define Pedestrian Movement
Pedestrian movement involves the study and understanding of how pedestrians navigate and use spaces. This field is significant in architecture and urban planning because it directly impacts the design and functionality of public spaces.
Understanding Pedestrian Flow
Pedestrian flow refers to the movement patterns of people walking through an area. It involves analyzing how pedestrians interact with their environment, including pathways, crosswalks, and public transport stations. Proper understanding helps improve efficiency, safety, and comfort in urban spaces.
- Pedestrian Density
- Walking Speed
- Route Choices
High pedestrian density can slow down movement and create congestion.
Factors Affecting Pedestrian Movement
Several factors influence pedestrian movement, shaping how individuals navigate spaces. Key factors include:
- Environmental Design: Features like sidewalks, street furniture, and lighting impact movement.
- Land Use: The distribution of commercial, residential, and recreational spaces affects pedestrian patterns.
- Social Interactions: People often adjust their paths based on social behaviors and interactions.
For instance, a well-lit, broad sidewalk in a busy commercial area will likely attract more pedestrians compared to a poorly lit and narrow walkway.
Measuring Pedestrian Movement
Measuring pedestrian movement involves various methods to collect data. Common techniques include:
- Manual Counting: Observers count pedestrians at specific locations.
- Automated Systems: Sensors and cameras that track pedestrian numbers and flows.
- Surveys: Collecting data on pedestrian preferences and behaviors through questionnaires.
Surveys can provide insights into why pedestrians prefer certain routes.
Recent advancements in technology have introduced the use of machine learning algorithms to analyze pedestrian movement patterns. These algorithms can process large datasets from sensors or CCTV footage, providing more accurate and comprehensive insights into pedestrian behaviors.This technology is increasingly being used in smart city planning to design more efficient and pedestrian-friendly urban environments.
Pedestrian Movement Theory
Pedestrian movement theory examines the patterns and dynamics of how people navigate through different environments. This is crucial for designing efficient urban areas that prioritize safety and accessibility.
Understanding Pedestrian Flow
Pedestrian flow deals with the movement of people walking through an area. It involves factors such as:
- Pedestrian Density: The number of people per unit area.
- Walking Speed: The average speed at which people walk.
- Route Choices: The paths that people choose to navigate through an area.
High pedestrian density can lead to slower movement and increased congestion.
Factors Affecting Pedestrian Movement
Many factors influence how pedestrians move through spaces:
- Environmental Design: This includes features like sidewalks, benches, and lighting.
- Land Use: The types and distributions of buildings and open spaces.
- Social Interactions: People's behavior and social interactions can influence their movement patterns.
A wide, well-lit sidewalk in a commercial area is likely to attract more pedestrians than a narrow, poorly lit walkway.
Measuring Pedestrian Movement
Various methods exist to measure pedestrian movement, helping planners design efficient spaces.
- Manual Counting: Observers physically count pedestrians at specific locations.
- Automated Systems: Sensors and cameras that track pedestrian numbers and flows.
- Surveys: Questionnaires designed to gather data on pedestrian preferences and behaviors.
Surveys can provide insights into why pedestrians prefer certain routes.
Advancements in technology are transforming how we analyze pedestrian movement through the use of machine learning algorithms. These systems process large datasets from sensors or camera footage to uncover intricate patterns in pedestrian behavior. This enables urban planners to create more pedestrian-friendly environments in smart cities.
Pedestrian Movement Analysis
Understanding how pedestrians move through different spaces is crucial for urban planning and architecture. This involves analyzing various methods and tools to ensure efficiency and safety.
Methods of Pedestrian Movement Analysis
There are several methods to understand pedestrian movement. Using the right method can help in obtaining accurate data and improving pedestrian infrastructure.
Manual Counting: The process where an observer physically counts the number of pedestrians passing through a specific point.
Example: In a busy shopping mall, analysts might count how many people pass through the main entrance every hour.
Automated Systems: Technology has enabled the use of sensors and cameras to automatically track pedestrian movement. These systems are often more accurate and can collect data over long periods.
Automatic Pedestrian Counters: Devices that use sensors or cameras to automatically count pedestrians.
Using machine learning, automated systems can process large datasets to identify patterns in pedestrian movement. This data can help urban planners design more efficient and pedestrian-friendly cities.
Automated systems can also provide data on pedestrian speed and direction.
Example: A camera placed at a busy intersection records pedestrian movement, and software analyzes this footage to extract data on foot traffic patterns.
Surveys: Surveys gather data directly from pedestrians about their preferences and behaviors. This qualitative data is essential for understanding the reasons behind certain pedestrian choices.
- Preferences on walking routes
- Feelings of safety
- Improvements needed
Surveys can be conducted in-person or online, and they're particularly helpful for understanding user satisfaction.
Tools for Pedestrian Movement Analysis
Various tools are used in pedestrian movement analysis, each offering unique benefits and applicable in different scenarios. These tools help gather and analyze data, contributing to a comprehensive understanding of pedestrian patterns.
GPS Devices: GPS technology can track the location and movement of pedestrians. This tool helps in understanding detailed movement patterns and distance covered.
- Accurate location tracking
- Data over extended periods
- Analysis of route choices
Global Positioning System (GPS): A satellite-based system used to determine precise location and time information.
Example: Equipping participants in a study with GPS trackers to monitor their routes and gain insights into their walking habits.
Advanced GPS systems, along with analytical software, can process large datasets to create detailed heat maps of pedestrian movement. Such maps can visualize areas with high pedestrian traffic, aiding in infrastructure planning.
Heat maps generated from GPS data can visualize high traffic pedestrian zones.
Now, let's consider mathematical methods used in pedestrian analysis. Mathematical models help simulate and predict pedestrian movement patterns. For instance, using fluid dynamics to model pedestrian flow similar to the movement of fluids can be effective. Here's an example of a simple mathematical equation used in pedestrian flow modeling:
The equation \(F = k \times D\) explains the relationship between pedestrian flow (F), density (D), and a proportional constant (k). If pedestrian density increases, flow can be impacted. Let's break down each variable:
Variable | Description |
F | Pedestrian Flow |
D | Pedestrian Density |
k | Proportional Constant |
Analyzing these variables helps planners understand congestion points and manage pedestrian flow efficiently.
Urban Pedestrian Movement Patterns
Understanding urban pedestrian movement patterns is essential for creating functional and efficient urban spaces. This section will delve into the methodologies and factors influencing these patterns.
Studying Urban Pedestrian Movement Patterns
Studying urban pedestrian movement involves various techniques to gather and analyze data about how people navigate city environments. Accurate data collection is crucial for improving infrastructure and ensuring safety.
- Manual Counting: Observers physically count pedestrians at specific locations.
- Automated Systems: Sensors and cameras automatically track pedestrian numbers and flows.
- Surveys: Collect data on pedestrian preferences and behaviors through questionnaires.
Automated systems can provide continuous data collection, which is more practical for long-term studies.
For instance, using automated cameras at busy traffic intersections can help urban planners identify peak pedestrian traffic times.
Advanced technologies such as machine learning are increasingly used to enhance pedestrian movement analysis. Machine learning algorithms can process large datasets from various sensors or CCTV footage to identify complex patterns that might not be evident from simple observations.Using these advanced tools, urban planners can make data-driven decisions to design more efficient and pedestrian-friendly environments in modern smart cities.
Factors Influencing Urban Pedestrian Movement Patterns
Various factors influence how pedestrians move through urban spaces. Recognizing these factors helps in designing more pedestrian-friendly cities.
- Environmental Design: Infrastructure elements like sidewalks, street furniture, and lighting influence movement.
- Land Use: Distribution of commercial, residential, and recreational spaces impacts pedestrian patterns.
- Social Interactions: People adjust their paths based on social behaviors and interactions.
Light, temperature, and weather conditions can significantly influence pedestrian behavior.
A well-lit, broad sidewalk with clear signage will attract more pedestrians compared to a poorly lit, narrow path.
Pedestrian Density and Traffic Flow: Mathematical models are often used to simulate pedestrian movement and density.
Variable | Description |
F | Pedestrian Flow |
D | Pedestrian Density |
k | Proportional Constant |
Understanding these variables helps planners manage pedestrian congestion more effectively.
Recent studies have explored the use of fluid dynamics to model pedestrian flow. By treating pedestrian movement similar to the flow of fluids, planners can better predict congestion points and optimize pathways for ease of movement. For example, using the Navier-Stokes equations, which are fundamental in fluid mechanics, allows for more accurate simulations: \[ \frac{\text{D}\rho}{\text{D}t} = -\rho (abla \bullet \textbf{u}) \ \] where \(\rho\) represents pedestrian density and \(\textbf{u}\) velocity. These advanced methods offer deeper insights into pedestrian dynamics and contribute to more robust urban planning strategies.
Improving Pedestrian Movement
Enhancing pedestrian movement is essential for creating safer, more efficient, and enjoyable urban environments. Various strategies and technologies can be employed to achieve this objective.
Strategies to Facilitate Pedestrian Movement
Implementing effective strategies can greatly improve the flow and safety of pedestrian traffic. Here are some key strategies:
- Wide Sidewalks: Providing ample space for pedestrians to walk can reduce congestion and improve flow.
- Crosswalks: Well-marked and timed crosswalks can enhance pedestrian safety.
- Signage: Clear and informative signs help guide pedestrians efficiently.
- Pedestrian Zones: Areas restricted to pedestrian use improve the comfort and safety of foot traffic.
Pedestrian Zone: A designated area where vehicle access is limited or prohibited to prioritize pedestrian movement.
A prime example of a pedestrian-friendly area is Times Square in New York City, which has designated pedestrian zones.
Wider sidewalks not only accommodate more pedestrians but also encourage local business patronage.
Mathematical models can help in strategizing pedestrian movement. For instance, if you want to determine the optimal width of a sidewalk, you could use the following equation to account for pedestrian density and flow rate:\[ W = \frac{F}{D \cdot v} \ \]where:
Variable | Description |
W | Width of the sidewalk (meters) |
F | Pedestrian Flow (people per minute) |
D | Pedestrian Density (people per square meter) |
v | Pedestrian Speed (meters per minute) |
Another advanced strategy involves the use of simulation software to model pedestrian movement in crowded environments, such as stadiums or transit hubs. These simulations use complex algorithms to predict how people will move based on various factors, such as entry and exit points, obstacles, and walking speeds.By adjusting different variables, planners can find the most efficient layouts to facilitate smooth pedestrian flow, thus avoiding potential bottlenecks.
Technology in Improving Pedestrian Movement
Modern technology plays a critical role in enhancing pedestrian movement. These innovative tools allow urban planners to collect data, analyze patterns, and implement solutions more effectively.
Pedestrian Tracking Systems: Devices or software that monitor and analyze pedestrian movement in real-time.
For example, smart cameras equipped with computer vision technology can count the number of pedestrians and analyze their movement patterns.
Smart lighting can adjust brightness levels based on pedestrian activity, enhancing both safety and energy efficiency.
Technology also enables the application of mathematical models to predict and improve pedestrian flow. For instance, by using data from tracking systems, planners can employ the following equation to model pedestrian movements:\[ P_m = \sum_{i=1}^{n} (f_i \cdot t_i) \ \]where:
Variable | Description |
P_m | Predicted Movement (people per hour) |
f_i | Flow rate at point i (people per minute) |
t_i | Time spent at point i (minutes) |
n | Number of measured points |
Another exciting application of technology in pedestrian movement is the use of augmented reality (AR) for navigation. AR can provide real-time information and guidance to pedestrians through their smartphones or smart glasses. This can be particularly useful in complex environments like airports or large campuses, ensuring that pedestrians can easily find their way without getting lost.By integrating AR with data from pedestrian tracking systems, urban planners can offer highly personalized and efficient navigation experiences.
Pedestrian Movement - Key takeaways
- Define Pedestrian Movement: Study of how pedestrians navigate and use spaces, influencing architecture and urban planning.
- Pedestrian Movement Analysis: Methods to understand pedestrian movement, such as manual counting, automated systems, and surveys.
- Factors Influencing Pedestrian Movement: Environmental design, land use, and social interactions affect how individuals navigate spaces.
- Urban Pedestrian Movement Patterns: Techniques and factors like environmental design influencing pedestrian navigation in city environments.
- Improving Pedestrian Movement: Strategies like wide sidewalks and technology (e.g., pedestrian tracking systems) to enhance pedestrian flow and safety.
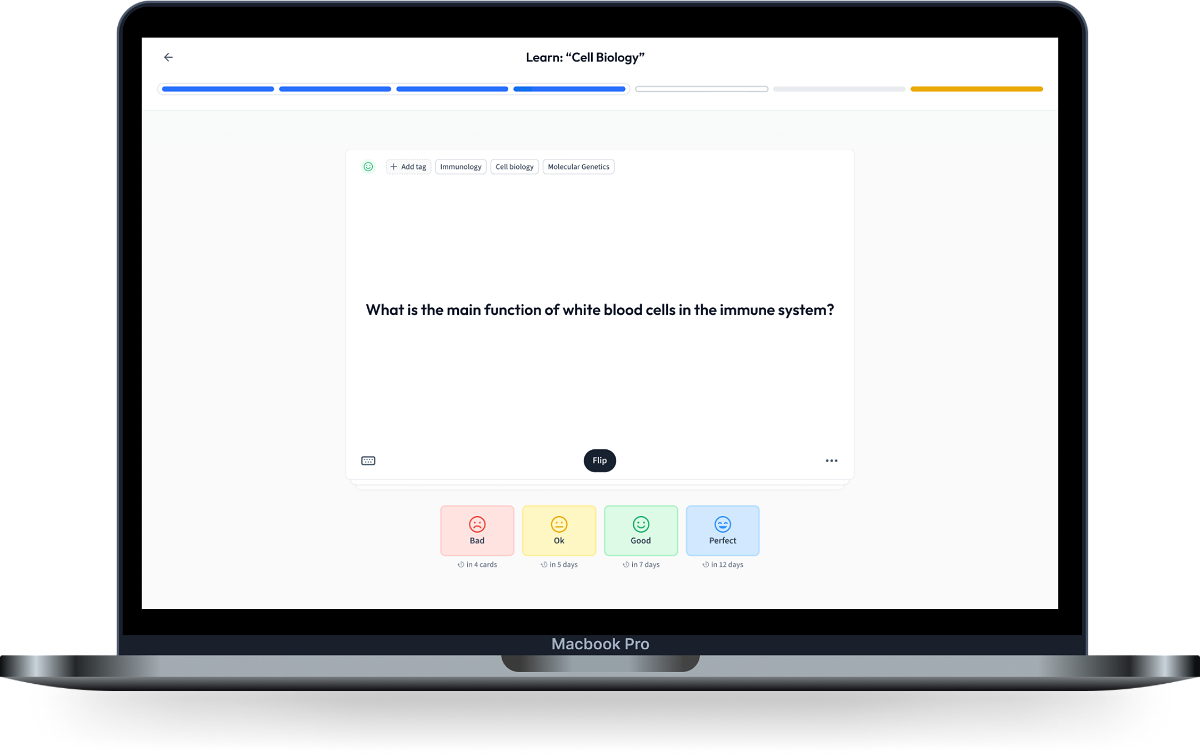
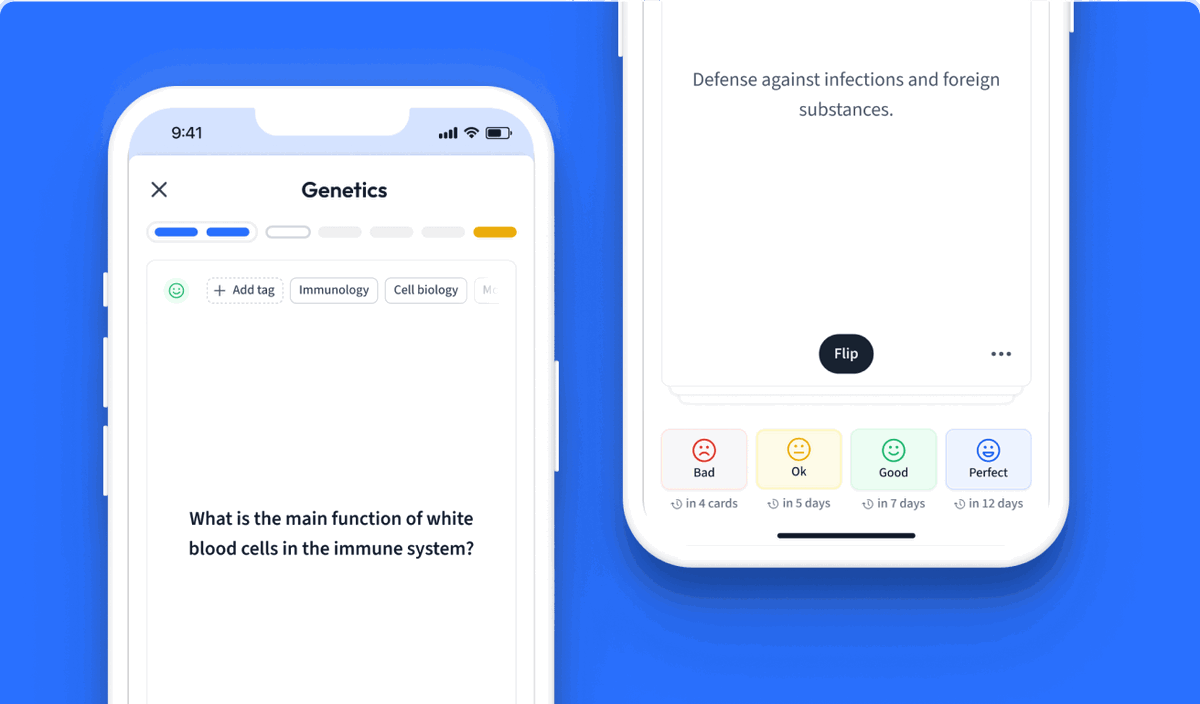
Learn with 15 Pedestrian Movement flashcards in the free StudySmarter app
We have 14,000 flashcards about Dynamic Landscapes.
Already have an account? Log in
Frequently Asked Questions about Pedestrian Movement
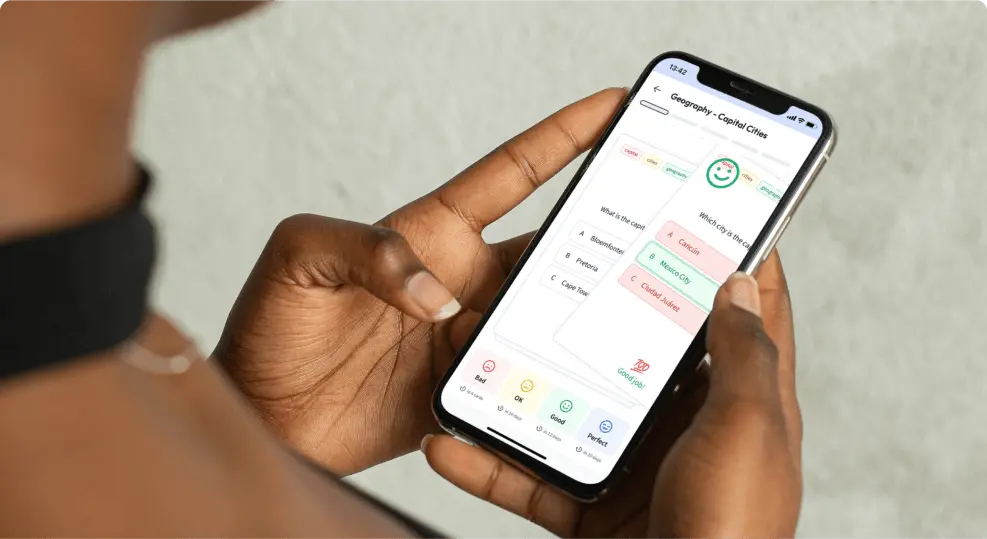
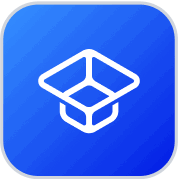
About StudySmarter
StudySmarter is a globally recognized educational technology company, offering a holistic learning platform designed for students of all ages and educational levels. Our platform provides learning support for a wide range of subjects, including STEM, Social Sciences, and Languages and also helps students to successfully master various tests and exams worldwide, such as GCSE, A Level, SAT, ACT, Abitur, and more. We offer an extensive library of learning materials, including interactive flashcards, comprehensive textbook solutions, and detailed explanations. The cutting-edge technology and tools we provide help students create their own learning materials. StudySmarter’s content is not only expert-verified but also regularly updated to ensure accuracy and relevance.
Learn more