Jump to a key chapter
Urban Spatial Analysis Definition
Urban Spatial Analysis is a crucial component of urban planning that involves examining and interpreting the spatial patterns and processes within urban areas. Through this analysis, planners and architects can understand how urban spaces are utilized and how these patterns impact the lives of urban residents. By utilizing various methods and tools, urban spatial analysis aids in designing more efficient and sustainable cities.
Key Concepts in Urban Spatial Analysis
When delving into urban spatial analysis, several key concepts are essential to understand:
- Spatial Distribution: How urban elements like residential, commercial, and industrial spaces are arranged across a city.
- Network Analysis: Studying the interconnectedness between different urban components, such as roads and public transport routes.
- Land Use Patterns: Identifying the functional usage of different urban areas, such as zoning zones for residential, commercial, and mixed-use.
- Demographic Analysis: Analyzing the population characteristics within specific urban areas.
Spatial Autocorrelation is a fundamental concept in urban spatial analysis. It refers to the phenomenon where similar values occur near one another in a spatial area. If areas with similar attributes are clustered together, this indicates a high level of spatial autocorrelation.
Consider a city where different neighborhoods have varying average income levels. In urban spatial analysis, a tool like Moran's I can be used to measure the spatial autocorrelation of income levels across the city. A higher value of Moran's I suggests that neighborhoods with similar income levels are clustered together, indicating an income spatial pattern.
Mathematical Tools in Urban Spatial Analysis
The use of mathematical and statistical tools is integral in urban spatial analysis. Here are a few crucial ones:
- Spatial Models: Mathematical frameworks used to represent the spatial relationships and processes in urban areas. An example is using a spatial econometric model to examine the diffusion of housing prices.
- Geostatistics: A branch of statistics focusing on spatial or spatiotemporal datasets. Geostatistical methods can be used to analyze and interpret various phenomena such as pollution levels across different urban zones.
- Geographical Information Systems (GIS): Software tools that enable urban planners to visualize, analyze, and interpret data to understand spatial relationships and trends.
Among the mathematical tools, the concept of Spatial Econometrics deserves a deeper exploration. This involves applying econometric techniques to spatial data considering spatial dependence and spatial heterogeneity. For example, in analyzing housing markets, spatial econometrics can account for how the price of a house is influenced not just by its own characteristics but also by the characteristics of neighboring houses and local amenities. The formula for a simple spatial autoregressive model can be expressed as:
\(Y = \rho WY + X\beta + \epsilon\)
Where:
- Y represents the dependent variable (e.g., housing prices).
- \rho represents the spatial auto-regressive coefficient.
- W is the spatial weights matrix capturing the relationship among different spatial units.
- X represents independent variables (e.g., distance to city center, number of bedrooms).
- \beta represents the parameters to be estimated.
- \epsilon represents the error terms.
This allows for a more comprehensive insight into the relationships across spatial entities, proving invaluable in urban planning and policy development.
Always consider the scale of analysis when performing urban spatial analysis. The same spatial pattern could indicate different phenomena when analyzed at a neighborhood versus city-wide scale.
Urban Spatial Analysis Methodology
Urban Spatial Analysis is integral in understanding the dynamics of city life. By studying the spatial arrangement and interactions within urban environments, you can gather insights into how cities function and identify areas for improvement. Various tools and data sources assist in conducting thorough analyses, enabling more informed urban planning.
Spatial Analysis Tools and Techniques
Urban spatial analysis relies on a suite of tools and techniques. Here are several prominent ones:
- Geographical Information Systems (GIS): Enables you to capture, store, manipulate, analyze, and present spatial or geographic data effectively.
- Remote Sensing: Employs satellite images to observe urban landscapes over time, helping in detecting changes and patterns.
- Spatial Autocorrelation Tools: This includes methods like Moran’s I and Geary’s C to determine the degree of correlation between similar data points distributed in space.
- Network Analysis: Useful in examining the flow of movement within urban infrastructures, such as transportation systems.
Each of these tools allows for a deeper understanding of urban phenomena by assessing how spatial variables interact. For instance, GIS technology assists in visualizing data layers, which can reveal important spatial relationships. Moreover, network analysis can highlight the connectivity and accessibility of urban areas, a vital consideration in traffic management and public transport planning.
A case study of using GIS in urban planning is the implementation of bus rapid transit (BRT) systems in many cities. Through GIS, planners analyzed population density maps alongside existing traffic data to design routes that maximized accessibility and efficiency. By overlaying these layers, planners could optimize stop placements and minimize traffic congestion.
GIS software like ArcGIS and QGIS can handle a wide range of spatial data formats, making them highly versatile tools for urban spatial analysis.
Data Sources for Urban Spatial Analysis
Reliable data sources are fundamental for performing urban spatial analysis. Here are several key data types frequently utilized:
- Demographic Data: Population statistics, age distribution, and other social metrics.
- Land Use Data: Information about how land is utilized, which can be sourced from planning authorities or public records.
- Transportation Data: Includes networks of roads, public transport routes, and traffic patterns.
- Environmental Data: Data on the natural environment, such as green spaces and pollution levels.
These data types form the basis of urban spatial analysis, providing essential insights into how different aspects of a city interrelate. Demographic data helps in understanding the composition of urban populations, while transportation data aids in mapping accessibility within the city. Environmental data is crucial for assessing the impact of urbanization on natural ecosystems.
An interesting aspect of data sources is the use of Crowdsourced Data, which is increasingly becoming invaluable in urban analysis. Platforms like OpenStreetMap gather volunteer contributions to create up-to-date maps and geographic information. Crowdsourced data is advantageous due to its flexibility and ability to rapidly respond to changes, such as new road constructions or urban developments.
Utilizing advanced techniques such as machine learning can also enhance the extraction of meaningful patterns from urban data. For instance, clustering algorithms can be applied to demographic data to identify distinct user profiles or settlement patterns. Such analysis can be represented mathematically as:
\[\text{arg min}_{C} \sum_{i=1}^{k} \sum_{x \, in \, C_i} \| x - \text{mean}(C_i) \|^2\]
Here, the aim is to minimize the sum of squares of the distances from each data point to its assigned cluster mean, making it possible to recognize urban districts with similar characteristics.
When working with data, remember to pay attention to the accuracy and timeliness of your sources to ensure reliable urban spatial analysis results.
Spatial Analysis in Urban Planning
Spatial Analysis in Urban Planning plays a vital role in assessing and developing effective urban environments. Utilizing various methodologies and technologies, it aids planners in making data-driven decisions to enhance urban spaces sustainably and efficiently.
Role of GIS in Urban Planning
Geographical Information Systems (GIS) are indispensable in urban planning due to their ability to process and analyze spatial data. GIS provides planners with tools to visualize spatial relationships and patterns within urban areas, facilitating informed decision-making.
With GIS, urban planners can:
- Map existing infrastructure: Visual representations of roads, utilities, and public facilities.
- Analyze spatial data: Evaluate land use patterns, population density, and traffic flows.
- Simulate urban growth: Project future scenarios based on current trends and data.
By integrating GIS with urban data, planners can model various urban scenarios, aiding in resource allocation and development planning. This leads to more efficient, sustainable urban environments.
An example of GIS application is in flood risk management. Using GIS, planners can overlay floodplain maps with population density and infrastructure layers to identify areas at risk. By assessing these risks, planners can develop strategies to mitigate potential flood impacts, such as constructing levees or updating zoning laws to limit development in high-risk areas.
GIS tools allow real-time data updating, making them ideal for dynamic urban scenarios where conditions change rapidly, like disaster management.
Trends in Urban Spatial Planning
Current trends in Urban Spatial Planning reflect the increasing integration of technology and data-driven approaches into planning processes. These trends help shape more adaptable and sustainable urban environments.
Some notable trends include:
- Smart Cities: Utilizing IoT (Internet of Things) and data analytics to enhance urban living conditions and resource management.
- Participatory Planning: Engaging citizens in the planning process through platforms that incorporate feedback into decision-making.
- Sustainable Design: Emphasizing eco-friendly infrastructure and promoting green urban spaces to reduce environmental impacts.
Smart cities, for instance, leverage technology to improve urban services, from traffic management to energy utilization. Planners can deploy sensors and applications to collect real-time data, allowing for more responsive and efficient urban management.
The trend towards Big Data in urban planning is reshaping how cities are analyzed and developed. Big data involves collecting and analyzing massive datasets from various urban sensors and platforms to gain deeper insights into urban dynamics. This data is often processed using advanced algorithms and machine learning techniques to identify patterns and predict outcomes.
For example, transportation planners use big data to optimize traffic light patterns, reduce congestion, and improve public transport efficiency. Mathematically, this can be represented using optimization algorithms like:
\[\min \, f(x) = \sum_{i=1}^{n} \left( c_i \cdot x_i \right) \]
Subject to:
\[\sum_{j=1}^{m} a_{ij} \cdot x_i \leq b_j, \quad \forall j\]
Here, f(x) is the objective function minimizing traffic congestion costs, with n representing routes and m constraints like available public transport units. The information derived from big data allows planners to develop smarter, more responsive urban solutions.
Integrating multiple urban datasets can provide comprehensive insights, crucial for addressing complex urban challenges.
Analysis Techniques for Urban Form and Design
Urban form and design play a pivotal role in creating functional and aesthetically pleasing cities. The methods used to analyze these aspects can be broadly categorized into quantitative and qualitative approaches. Both offer unique insights that together inform comprehensive urban planning and development strategies.
Quantitative Methods for Urban Form Analysis
Quantitative methods in analyzing urban form involve the use of numerical data and statistical tools to examine physical and spatial characteristics of urban areas. These methods provide a structured approach to understanding urban form, allowing planners to measure, compare, and predict urban dynamics quantitatively.
Prominent quantitative methods include:
- Spatial Statistics: Techniques to identify spatial patterns, correlations, and clusters.
- Regression Analysis: Used to determine relationships between different urban variables.
- Network Analysis: Examines the connectivity and accessibility of urban infrastructure systems.
- GIS-based Analysis: Utilizes geospatial data for mapping and analyzing urban phenomena. It assists in visualizing spatial distribution and land-use patterns.
Quantitative analysis often involves mathematical modeling. For instance, when examining land-use patterns, you may employ a regression model:
\[Y = \beta_0 + \beta_1X_1 + \beta_2X_2 + ... + \beta_nX_n + \epsilon\]
where Y is the dependent variable (like land value), X are independent variables (such as distance to city center or availability of amenities), and \(\epsilon\) is the error term. This model helps in predicting how different factors impact urban land values.
An application of regression analysis can be observed in studying the impact of green spaces on property prices. By analyzing data across a city, planners found that properties closer to parks and amenities generally have higher values. This analysis helps determine the optimal allocation of green spaces to maximize both property values and quality of urban life.
Applying spatial autocorrelation tools like Moran's I can reveal whether the spatial layout of a variable (e.g., crime rates) is random or demonstrates a patterned trend.
Qualitative Approaches in Urban Design
Qualitative approaches are essential for understanding the social and cultural dimensions of urban design. Unlike quantitative methods, qualitative techniques focus on subjective assessments, contextual interpretations, and human experiences within urban spaces.
Key qualitative methods include:
- Observational Studies: Involves direct observation of how people interact with their environment.
- Interviews and Surveys: Gather insights from residents about their perceptions and experiences.
- Case Studies: Detailed examination of specific urban projects to understand best practices and failures.
- Participatory Design: Engages local communities in the design process to ensure their needs and desires are met.
These approaches help planners capture the complexities of urban life that pure data-driven methods might overlook. For example, by observing public spaces, you can understand how urban design elements influence social interactions and accessibility.
Participatory design offers a deep insight into urban design by incorporating a broader range of voices in the planning process. This method acknowledges that residents hold valuable local knowledge about their neighborhoods and through structured workshops and collaborative sessions, planners can derive design solutions that are more aligned with community needs. One mathematical aspect of participatory planning is using graph theory to model community feedback and preferences through network diagrams, visualizing the connections and priorities of local residents.
In one case in Copenhagen, planners used a mix of interviews, workshops, and public polling, combined with network analysis, to decide on the most-needed community facilities. This approach ensures that urban designs are not only functional but also culturally and socially inclusive.
While quantitative data might tell you what is happening in an urban area, qualitative methods can help explain why it is happening.
Urban Spatial Pattern Analysis
Urban Spatial Pattern Analysis involves studying the arrangement and distribution of various elements within urban areas. Understanding these patterns is crucial for effective urban planning and development. By employing different analytical methods, planners can evaluate how these patterns affect city life and the built environment.
Identifying Urban Patterns
Identifying urban patterns requires a comprehensive approach that combines several analytical tools. This process helps analysts detect and interpret various spatial relationships and distributions that exist in urban areas. Here are key methods used to identify these patterns:
- Zoning Analysis: Examines the allocation of land for different purposes, such as residential, commercial, and industrial.
- Clustering Analysis: Groups areas with similar characteristics to reveal patterns like commercial districts or residential zones.
- Density Mapping: Visualizes population density and distribution, highlighting areas of high and low intensity.
These methods are integral in uncovering how homes, businesses, and public spaces are spread across an urban landscape, directly impacting resource allocation and service provision. For example, clustering analysis might reveal concentrated commercial areas that demand better public transport links.
An instance of urban pattern identification is the analysis of car ownership in relation to public transportation access. Using spatial analysis, planners can map areas with low car ownership yet limited public transport access, indicating a need for improved transit services. The identification of such a pattern can guide policy formulation to ensure equitable transport accessibility.
Consider using machine learning algorithms for pattern recognition, as they can handle vast datasets and reveal insights not immediately visible through traditional methods.
Impacts of Spatial Patterns on Urban Development
The spatial patterns within urban areas significantly affect their development and sustainability. By examining these patterns, urban planners can make informed decisions that enhance city functionality and livability.
Here are several impacts of spatial patterns on urban development:
- Traffic Congestion: High-density employment areas often lead to increased traffic, necessitating better transit options.
- Green Space Distribution: Uneven allocation of parks can affect residents' quality of life, influencing urban policy for equal access.
- Infrastructure Costs: Dispersed spatial patterns can increase expenses related to infrastructure development, such as roads and utilities.
One intriguing aspect of spatial patterns is their influence on urban heat islands (UHIs). UHIs occur when urbanization creates regions significantly warmer than their rural surroundings. The arrangement of buildings and materials that absorb and retain heat contributes to this effect. To measure UHIs' impacts, planners use spatial equations and models, predicting temperature variations by:
\[UHI = T_{urban} - T_{rural}\]
where \(T_{urban}\) is the temperature in the urban area, and \(T_{rural}\) is the surrounding rural temperature. Analyzing these patterns helps in designing strategies to mitigate UHIs, such as increasing urban vegetation and using reflective building materials.
Urban planners can use GIS tools to simulate the impacts of spatial designs, assessing various developmental scenarios and their long-term effects on urban environments.
urban spatial analysis - Key takeaways
- Urban Spatial Analysis Definition: Involves examining spatial patterns and processes within urban areas to aid urban planning.
- Urban Spatial Analysis Methodology: Utilizes tools like GIS, network analysis, and data sources such as demographic and land use data.
- Spatial Analysis in Urban Planning: Incorporates GIS, smart city trends, and sustainable design to enhance urban environments.
- Analysis Techniques for Urban Form and Design: Combines quantitative methods like spatial statistics with qualitative approaches for comprehensive insights.
- Urban Spatial Pattern Analysis: Focuses on identifying and interpreting spatial arrangements that impact urban development.
- Spatial Autocorrelation: A key concept indicating similar values occurring near each other, measured by tools like Moran's I.
Learn faster with the 10 flashcards about urban spatial analysis
Sign up for free to gain access to all our flashcards.
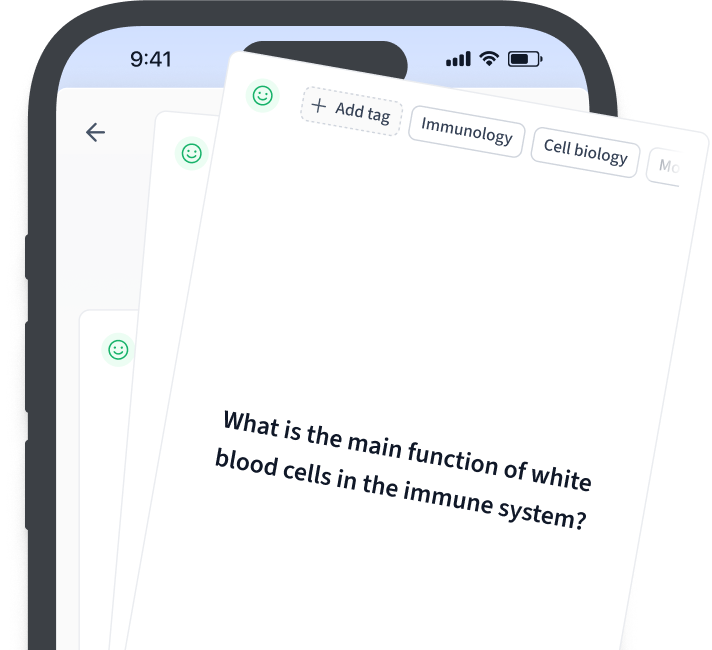
Frequently Asked Questions about urban spatial analysis
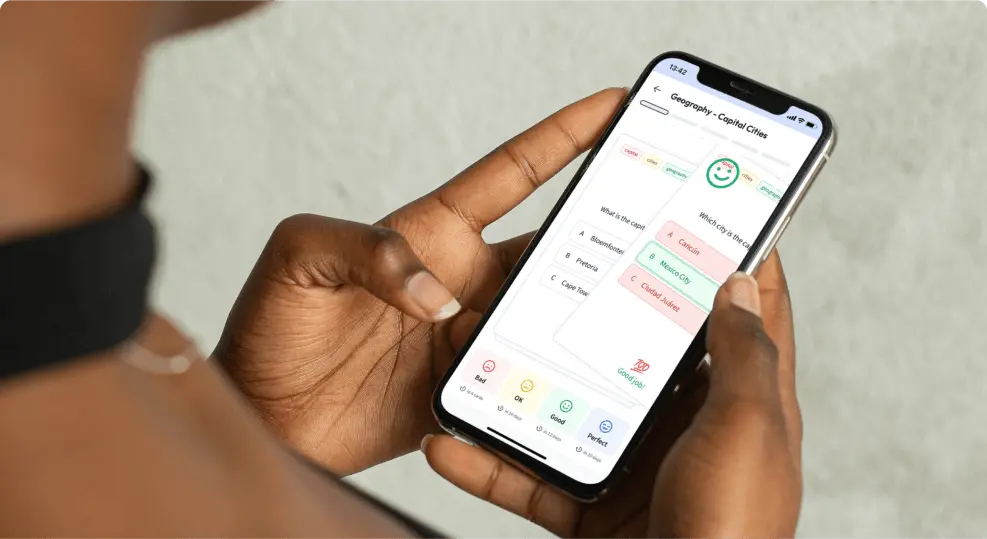
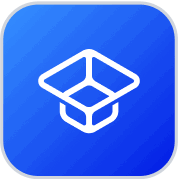
About StudySmarter
StudySmarter is a globally recognized educational technology company, offering a holistic learning platform designed for students of all ages and educational levels. Our platform provides learning support for a wide range of subjects, including STEM, Social Sciences, and Languages and also helps students to successfully master various tests and exams worldwide, such as GCSE, A Level, SAT, ACT, Abitur, and more. We offer an extensive library of learning materials, including interactive flashcards, comprehensive textbook solutions, and detailed explanations. The cutting-edge technology and tools we provide help students create their own learning materials. StudySmarter’s content is not only expert-verified but also regularly updated to ensure accuracy and relevance.
Learn more