Jump to a key chapter
Definition of Forecast Reliability
Forecast reliability is a crucial concept in business studies that refers to the degree of accuracy with which future demand, sales, or other business metrics are predicted compared to the actual outcomes that occur later. It highlights the trustworthiness of the forecasted data's ability to represent future realities.
Understanding forecast reliability goes beyond just comparing forecasted and actual data. It delves into the methodologies used for forecasts, the data sources, and how unforeseen factors impact the predictions. Businesses often rely on various models, such as time series analysis, regression models, or machine learning algorithms, to enhance the reliability of their forecasts.
Forecast Accuracy: This is a closely related term that measures the degree of closeness of the forecasts to the actual outcomes. High forecast accuracy typically means high forecast reliability.
- Importance of Forecasts: Businesses need reliable forecasts to plan for production, manage inventories, allocate resources effectively, and streamline operations.
- Key Factors Affecting Reliability: These include quality of data, forecasting models used, assumptions made, and external market factors.
- Measuring Reliability: Often involves statistical metrics like Mean Absolute Percentage Error (MAPE) or Root Mean Square Error (RMSE).
Factors Affecting Forecast Reliability
Understanding the factors that impact forecast reliability is essential, as these can directly influence the accuracy of business predictions.
Data Quality
The quality of data used in forecasting is paramount. High-quality data is accurate, up-to-date, and relevant, leading to more reliable forecasts. Errors in data, such as missing values or inaccuracies, can significantly skew predictions.
Regular data cleaning and updates are crucial to maintain high data quality.
Forecasting Models
Different models provide different levels of reliability. Choosing the appropriate model for your specific business context is vital. Common models include time series analysis, regression, and machine learning algorithms. Each has its own strengths and weaknesses, and the choice often depends on data complexity and availability.
Suppose a company uses a linear regression model to predict sales. If the relationship between variables is not linear, this model may not be reliable. Exploring alternative models like a polynomial regression might yield better results. The formula for a simple linear regression is given by \( y = \alpha + \beta x \), where \( y \) is the dependent variable, \( x \) is the independent variable, \( \alpha \) is the intercept, and \( \beta \) is the slope.
Assumptions in Forecasting
Forecasting models often rely on specific assumptions, such as market conditions remaining constant or certain trends continuing. If these assumptions are incorrect, reliability decreases significantly. Ensuring that assumptions reflect realistic scenarios is key.
Examine how assumptions impact reliability with hypothetical changes in market scenarios. Consider a forecasting model assuming a steady economic growth rate. An unexpected recession will violate this assumption, leading to poor forecast reliability. To model such scenarios, sensitivity analysis can be applied. This involves adjusting the input variables to see how changes affect the forecasted outcome. A simple sensitivity analysis might recalculate forecasts using different economic growth rates, using the formula \( F' = F \times (1 + r) \), where \( F' \) is the adjusted forecast, \( F \) is the original forecast, and \( r \) is the change in growth rate.
External Market Factors
External factors such as economic conditions, market dynamics, and regulatory changes can impact forecast reliability. These factors are often difficult to predict but must be incorporated into forecasts as best as possible. Mitigating their effects requires a holistic approach to forecasting that includes scenario planning and risk analysis.
Scenario Planning: A strategic planning method used to create flexible long-term plans by considering different future scenarios and their possible impacts on business decisions.
Forecast Reliability in Business Decision-Making
In the realm of business decision-making, understanding forecast reliability is pivotal. Forecasts guide key business strategies and decisions, from inventory management to resource allocation.
Accuracy of Forecasting Methods
Various methods are employed to improve the accuracy of business forecasts. Each method has its strengths based on the context and data available. Some commonly used methods include:
- Time Series Analysis: Utilizes historical data to predict future values by identifying patterns over time.
- Regression Models: Establish relationships between variables to forecast outcomes, guided by the equation \( y = \alpha + \beta x \).
- Machine Learning Algorithms: Use large datasets to build models that predict future events by learning from past trends.
Consider a company predicting future sales using a time series model. If the historical data shows clear seasonal patterns, models like the Holt-Winters seasonal method might be appropriate. The Holt-Winters model can be expressed by \( L_t = \alpha (y_t - S_{t-m}) + (1-\alpha)(L_{t-1} + T_{t-1}) \), where \( L_t \) is the level, \( T_t \) is the trend, and \( S_t \) is the seasonal component.
Impact of Data Quality
Data quality is a cornerstone in achieving reliable forecasts. High-quality data should be:
- Accurate: Free from errors and reflects true values.
- Timely: Up-to-date to ensure relevance.
- Consistent: Uniform across data sources and over time.
Incorporating automation in data collection and cleansing processes can significantly enhance data quality.
Role of Assumptions in Forecasting
Forecasting models often rely on assumptions to simplify complex phenomena. These might include assumptions about market stability or consumer behavior. Unrealistic assumptions can lead to unreliable forecasts. Always tailor assumptions to reflect possible scenarios.
Consider the importance of sensitivity analysis when working with assumptions. This involves altering assumptions to observe potential impacts on forecasts. For instance, if adjusting a demand growth rate assumption by 10% significantly alters forecasted sales, the model's reliability hinges heavily on that assumption. The basic approach to sensitivity analysis can be illustrated by analyzing costs through \( C = FC + (VC \times Q) \), where \( C \) is total cost, \( FC \) is fixed cost, \( VC \) is variable cost per unit, and \( Q \) is quantity produced. Altering \( VC \) or \( Q \) demonstrates potential impacts on \( C \).
External Market Influences
External factors play a significant role in forecast reliability. These include economic shifts, technological advancements, and regulatory changes. Incorporating these influences within your forecasts is crucial, although challenging. Techniques such as scenario planning allow businesses to prepare for various potential market conditions.
Scenario Planning: It involves creating and analyzing different future scenarios to prepare strategic responses and build robust forecasts.
Impact of Unreliable Forecasts on Business
Unreliable forecasts can have profound effects on business operations, often leading to inefficiencies or missed opportunities. Businesses rely heavily on forecasts for planning purposes, and when these aren't trustworthy, the implications can be numerous.
Some key impacts of unreliable forecasts include:
- Inventory Management Issues: Inaccurate forecasts can lead to overstocking or understocking. This misalignment can incur unnecessary costs or result in lost sales.
- Resource Allocation: Misguided forecasts may prompt inappropriate allocation of resources, affecting operational efficiency.
- Financial Planning: Companies may experience budget discrepancies and financial strain due to erroneous forecasting data.
Consider a retail business that relies on forecasted demand to order inventory. If winter jacket demand is overestimated, the business might overstock jackets. The cost of unsold inventory could be substantial, impacting the business's financial health.
Exploring how macroeconomic factors influence forecast dependability can offer deeper insights. For example, economic indicators like GDP growth and unemployment rates affect consumer spending trends, impacting sales forecasts. Misestimating the GDP growth rate could skew sales forecasts significantly. This adjustment can be modeled by examining the elasticity of demand with respect to income, given by \( E_d = \frac{\% \Delta Q}{\% \Delta I} \), where \( E_d \) is elasticity, \( \Delta Q \) is quantity variation, and \( \Delta I \) is income variation.
Techniques to Improve Forecast Reliability
To ensure forecast reliability, businesses can utilize several techniques that incorporate best practices and advanced technology.
Use historical data as a reference point, but also incorporate real-time data for enhanced accuracy.
Here are some approaches to consider:
- Utilizing Advanced Analytics: Implementing machine learning algorithms can help identify patterns and anomalies in data, improving forecast accuracy.
- Scenario Analysis: Testing different scenarios to understand potential variations in outcomes is key to building robust forecasts.
- Continuous Monitoring and Adjustment: Regularly updating forecasts with new data and insights ensures they remain useful and relevant.
A company might employ a neural network model to process large sets of data. As it processes more data over time, the model's forecasts should increase in reliability, adapting to changes in the data whenever they occur.
Challenges in Ensuring Forecast Accuracy
Achieving high levels of forecast accuracy presents numerous challenges. Businesses must navigate through several hurdles to refine their forecasting processes.
Forecast Accuracy measures the extent to which forecasted values align with actual outcomes, often quantified using metrics such as Mean Absolute Percentage Error (MAPE).
Key challenges include:
- Data Limitations: Incomplete or outdated data can reduce forecast precision.
- Market Volatility: Sudden shifts in market conditions demand highly adaptive forecasting models.
- Complexity of Models: As forecasting models grow more intricate, maintaining their accuracy becomes more challenging.
Consider the role of cognitive biases in forecasting. For example, decision-makers might rely too heavily on past experiences or exhibit overconfidence in certain assumptions. These biases can skew forecasts. Using tools such as adjusted error analysis, which involves compensating for known biases and errors based on previous forecasting performance, can be expressed by \( A' = O + (O - F) \), where \( A' \) is the adjusted forecast, \( O \) is the observed value, and \( F \) is the forecasted value.
forecast reliability - Key takeaways
- Forecast Reliability Definition: Measures the accuracy of predicted business metrics against actual outcomes, emphasizing trustworthiness.
- Factors Affecting Forecast Reliability: Includes data quality, choice of forecasting models, assumptions, and external market factors.
- Impact of Unreliable Forecasts on Business: Can cause issues like inventory mismanagement, resource misallocation, and financial planning errors.
- Techniques to Improve Forecast Reliability: Employing advanced analytics, scenario analysis, and continuous adjustment of forecasts.
- Forecast Reliability in Business Decision-Making: Guides key business strategies by ensuring accurate forecasts for effective decisions.
- Challenges in Ensuring Forecast Accuracy: Includes data limitations, market volatility, and model complexity, affecting forecast reliability.
Learn faster with the 24 flashcards about forecast reliability
Sign up for free to gain access to all our flashcards.
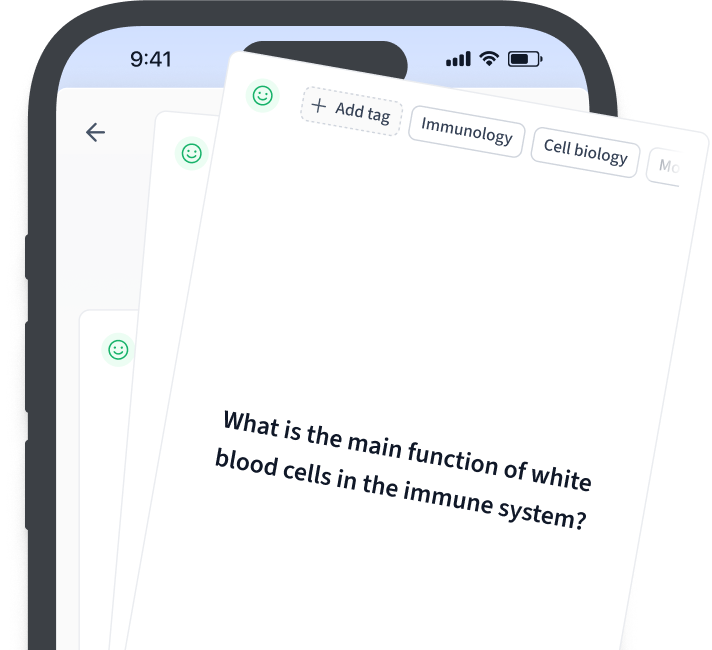
Frequently Asked Questions about forecast reliability
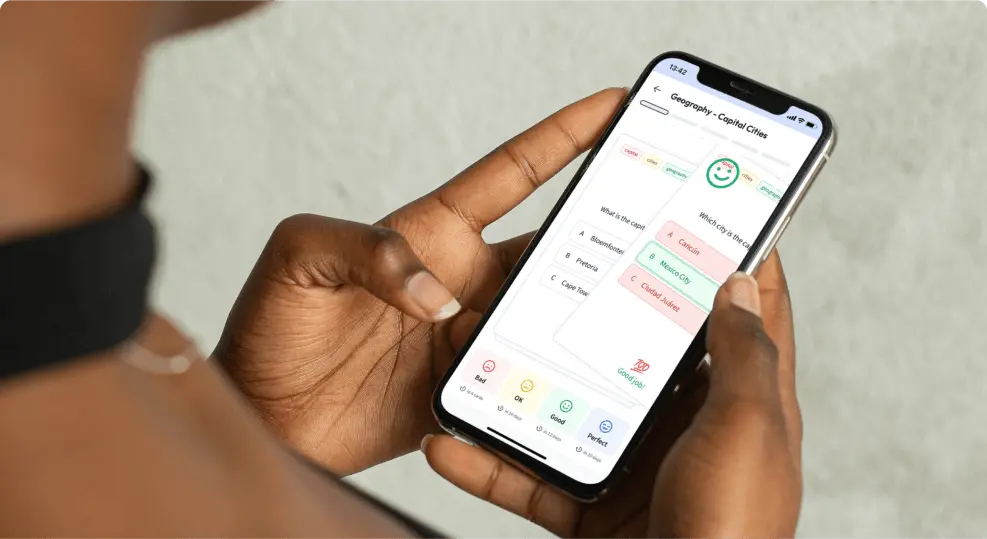
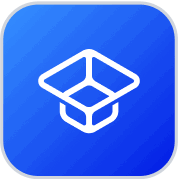
About StudySmarter
StudySmarter is a globally recognized educational technology company, offering a holistic learning platform designed for students of all ages and educational levels. Our platform provides learning support for a wide range of subjects, including STEM, Social Sciences, and Languages and also helps students to successfully master various tests and exams worldwide, such as GCSE, A Level, SAT, ACT, Abitur, and more. We offer an extensive library of learning materials, including interactive flashcards, comprehensive textbook solutions, and detailed explanations. The cutting-edge technology and tools we provide help students create their own learning materials. StudySmarter’s content is not only expert-verified but also regularly updated to ensure accuracy and relevance.
Learn more