Jump to a key chapter
Mid-term Forecasting Definition
Mid-term forecasting is a crucial practice in business planning that involves predicting the future state of various business metrics over a medium time frame, typically ranging from a few months to a couple of years. It serves as a bridge between short-term tactical decisions and long-term strategic planning.Effective mid-term forecasting enables businesses to anticipate market trends, manage resources efficiently, and make informed decisions that align with their overall objectives.
Mid-term forecasting refers to the process of estimating future events and performance metrics over a medium-duration time frame, usually spanning months to a few years. This practice aids in strategic planning and helps businesses allocate resources appropriately.
Key Components of Mid-term Forecasting
Understanding the key components involved in mid-term forecasting is essential to produce accurate and reliable forecasts. These components include:
- Data Collection: Gathering historical data on sales, market trends, customer behavior, and other relevant metrics.
- Trend Analysis: Identifying ongoing patterns and shifts in the business environment, such as technological advancements or economic changes.
- Model Selection: Choosing an appropriate mathematical model to make predictions. Common models include time series analysis and regression models.
- Assumption Setting: Establishing assumptions about future conditions to guide the forecasting process.
- Review and Adjustment: Continuously reviewing and adjusting forecasts as new data becomes available.
Suppose a retail company wants to forecast its mid-term sales for the next two years. The company may analyze previous years' sales data and market trends to predict future sales. It could use a time series model that accounts for seasonal sales patterns seen in past years. For instance, if the equation for the forecasted sales is \[S_t = a + bT + c\text{sin}(2\frac{\text{π}t}{12})\], \(S_t\) represents the sales at time \(t\), \(a\) is the base sales level, \(b\) is the linear growth rate, \(T\) denotes time, and \(c\text{sin}(2\frac{\text{π}t}{12})\) is the seasonal component.
Always remember that the accuracy of a mid-term forecast significantly depends on the accuracy and reliability of the input data. Make sure you source and clean data diligently.
The mathematical foundation of mid-term forecasting often involves advanced statistical techniques. A common approach is the ARIMA (AutoRegressive Integrated Moving Average) model, used extensively for time series data. This model blends differencing (to make data stationary), autoregression, and moving averages to predict future values based on a mixture of historical data and error terms.Understanding the ARIMA model requires you to delve into the specifics of its parameters:
- p: Number of lag observations in the model (lag order).
- d: Number of times that the raw observations are differenced to achieve stationarity.
- q: Size of the moving average window (order of the moving average).
Understanding Mid-term Forecasting
Mid-term forecasting is essential in business strategy, bridging the gap between the day-to-day operations and the broader, long-term goals of an organization. By projecting future trends and performance over a moderate time horizon, you can better align your immediate business actions with prospective developments. This ensures more informed decision-making and resource allocation.
Components of Mid-term Forecasting
To ensure successful mid-term forecasting, you need to consider several key components:
- Data Collection: Build a robust data set by gathering historical data, past performance metrics, and current market data to identify trends.
- Trend Analysis: Use analytical tools to identify underlying patterns in the data, aiding in the prediction of future trends.
- Model Selection: Choose suitable analytical models like time series analysis or ARIMA (AutoRegressive Integrated Moving Average), depending on the complexity of the data.
- Assumptions: Establish clear assumptions about future conditions based on current data trends.
- Review and Adjustment: Continuously monitor projections against actual performance and adjust the forecasting model as needed.
Consider a tech company that is expanding rapidly. To forecast the mid-term revenue growth, they collect sales data over the past five years and use a simple linear regression model. The model might indicate that the expected revenue is given by the equation: \[R_t = b_0 + b_1 T + \varepsilon_t\] Where \(R_t\) represents revenue, \(b_0\) is the intercept, \(b_1\) is the slope showing the rate of revenue growth per unit of time, and \(\varepsilon_t\) is the error term.
Incorporating qualitative data such as expert opinions and market news can provide additional insights into the mid-term forecasting process.
The ARIMA model is a powerful tool for mid-term forecasting, especially for time series data. By understanding and utilizing the ARIMA components
: \(ARIMA(p,d,q)\), you apply a combination of autoregressive features \((p)\), differences to stationarize the data \((d)\), and moving averages of lagged forecast errors \((q)\). A typical ARIMA setup may look like this:\[ X_t = c + \phi_1 X_{t-1} + \phi_2 X_{t-2} + ... + \theta_1 \epsilon_{t-1} + \theta_2 \epsilon_{t-2} + ... + \epsilon_t \] Where:
- \(X_t\) is the differenced time series value at time \(t\)
- \(c\) is a constant
- \(\phi\) are the autoregressive coefficients
- \(\theta\) are the moving average coefficients
- \(\epsilon_t\) are white noise error terms
Mid-term Forecasting Techniques
Mid-term forecasting techniques are essential tools in business planning, helping predict future performance over a medium time frame. These techniques provide insights that inform strategic decisions, resource allocation, and market positioning. Various methods are employed to ensure that forecasts are as accurate and useful as possible.
Quantitative Techniques
Quantitative forecasting techniques rely on numerical data and statistical methods to predict future outcomes. They are particularly useful when there is ample historical data available. Some of the most commonly used quantitative techniques include:
- Time Series Analysis: This method involves analyzing data points collected or recorded at specific time intervals to identify trends.
- Exponential Smoothing: A technique that applies weighted averages to past observations, giving more weight to recent observations.
- Regression Analysis: Estimates the relationships among variables, often used to forecast economic trends or consumer behavior.
- ARIMA Models: AutoRegressive Integrated Moving Average is a time series model that captures various elements of past data like trends and seasonal patterns.
Imagine a tourist destination wanting to predict the number of visitors for the upcoming holiday season. By using a time series analysis, the tourist board can identify historical peaks and trends. This is done by applying a model like:\[ V_t = \beta_0 + \beta_1 t + \beta_2 \text{HolidayDummies} + \epsilon_t \] Where \(V_t\) represents the number of visitors at time \(t\), \(\beta_0\) is the intercept, \(\beta_1\) is the time coefficient, \(\beta_2\) adjusts for seasonal dummy variables correlating with holidays, and \(\epsilon_t\) is the error term.
Qualitative Techniques
Qualitative forecasting techniques are subjective in nature and typically involve expert judgment rather than numerical data analysis. They are useful when there is less historical data available, or when predictions about new trends or events are needed.Methods include:
- Delphi Method: A structured communication process that relies on a panel of experts to reach a consensus forecast.
- Market Research: Involves gathering opinions and preferences from consumers to anticipate demand.
- Scenario Building: Creating detailed and plausible scenarios about how the future might unfold based on current trends and possible disruptions.
When data is limited, qualitative methods like the Delphi Method can be particularly valuable, providing insights from a range of expert perspectives.
Scenario building is a complex but insightful technique for mid-term forecasting. It requires the combination of various qualitative elements to envision detailed future outcomes. This method involves identifying key factors that affect your industry, analyzing the potential impacts of each, and crafting scenarios that reflect possible futures. by introducing multiple scientific approaches, from logical deduction to creativity, these scenarios then inform mid-term strategic planning. Here is how you can get started:
- Identify Key Drivers: Determine the main factors that will influence outcomes, such as political, economic, social, and technological shifts.
- Develop Scenarios: Envision different future states by varying these key drivers to reflect uncertainty.
- Assess Implications: Analyze how each scenario could impact your business, affecting everything from consumer demand to regulatory changes.
- Plan Around Scenarios: Identify strategies and actions for each possible scenario to ensure you are prepared no matter what the future holds.
Mid-term Forecasting Applications
Mid-term forecasting plays a vital role in various business applications. By predicting future trends over a medium time frame, businesses can make more informed decisions, optimize operations, and enhance strategic planning. These forecasts can be applied to different areas such as finance, supply chain management, and market analysis to anticipate changes and adjust strategies accordingly.
Mid-term Forecasting Examples
Mid-term forecasting is widely used across different industries to aid in planning and decision-making.Here are a few examples:
- Financial Planning: Businesses use mid-term forecasts to predict revenue, expenses, and cash flows for budgeting purposes, ensuring they have adequate resources to achieve financial goals.
- Supply Chain Management: Forecasting demand helps in maintaining optimal inventory levels, reducing stockouts and excess inventory, thus improving profitability.
- Human Resources: Estimating future staffing needs based on projected business growth allows for effective workforce planning.
- Marketing Strategies: By anticipating changes in consumer behavior, companies can adjust their marketing campaigns to align with expected demands.
Consider a manufacturing company that aims to forecast product demand for the upcoming year. Using historical sales data, they can apply a linear regression model to predict future sales:\[ D_t = a + b \times Y_t + \varepsilon_t \] Where \(D_t\) is the predicted demand, \(a\) is the intercept, \(b\) represents the slope and indicates how much demand changes per unit change in year \(Y_t\), and \(\varepsilon_t\) is the error term.
In-depth understanding of mid-term forecasting can be extended by exploring the quantitative techniques involved. Techniques such as regression analysis and time series analysis are pivotal in creating reliable forecasts. In time series analysis, components like trend components \((T_t)\), seasonal components \((S_t)\), cyclic components \((C_t)\), and irregular components \((\varepsilon_t)\) are analyzed to generate a comprehensive forecast model. A full time series equation may look like this:\[ Y_t = T_t + S_t + C_t + \varepsilon_t \] The combination of these elements helps in creating a detailed and accurate prediction of future behaviors by identifying patterns and relationships from historical data.A Python script example for such an analysis might be structured as follows:
import pandas as pdimport matplotlib.pyplot as pltfrom statsmodels.tsa.arima_model import ARIMA# Load dataseries = pd.read_csv('historic_sales_data.csv', header=0, index_col=0)# Fit modelmodel = ARIMA(series, order=(5,1,0))model_fit = model.fit(disp=0)# Forecastforecast, stderr, conf_int = model_fit.forecast(steps=12)plt.plot(forecast)plt.show()This code snippet loads sales data, fits an ARIMA model, and forecasts sales for the next 12 months.
mid-term forecasting - Key takeaways
- Mid-term Forecasting Definition: Predicting future business metrics over a medium time frame of months to a few years, aiding strategic planning and resource allocation.
- Components of Mid-term Forecasting: Data collection, trend analysis, model selection, assumption setting, review, and adjustment are crucial for accurate forecasts.
- Mid-term Forecasting Techniques: Combines quantitative methods like time series analysis, ARIMA models, and qualitative methods like Delphi and scenario building.
- Mid-term Forecasting Applications: Essential for financial planning, supply chain management, human resources, and marketing strategies to anticipate changes.
- Mid-term Forecasting Examples: Retail forecasting using historical sales data and ARIMA models to predict future trends and demand.
- Understanding Mid-term Forecasting: Integrates advanced statistical techniques, such as ARIMA, to align business actions with anticipated market developments.
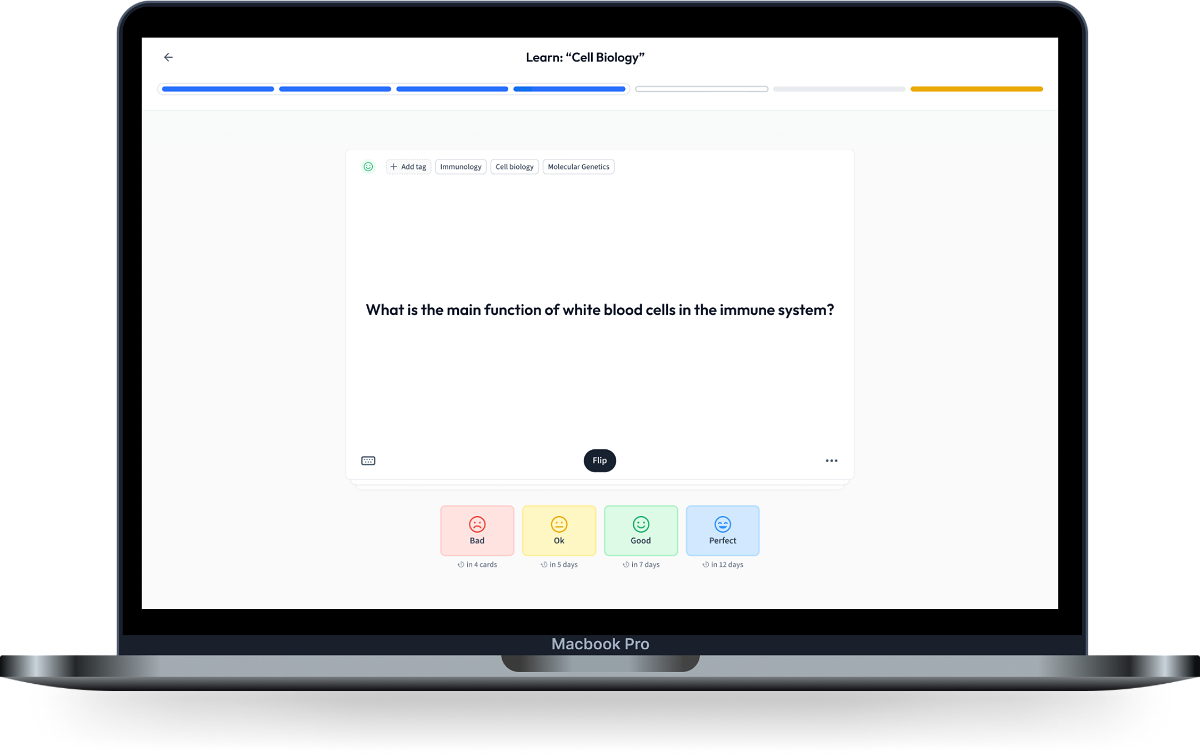
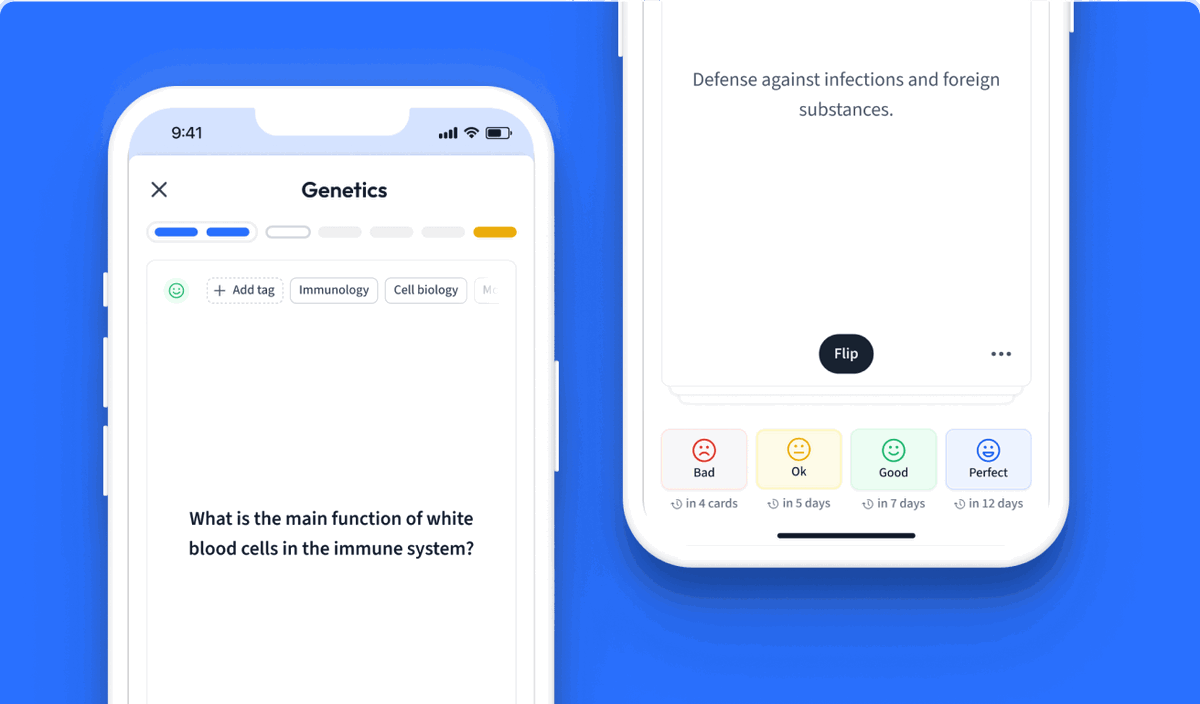
Learn with 24 mid-term forecasting flashcards in the free StudySmarter app
We have 14,000 flashcards about Dynamic Landscapes.
Already have an account? Log in
Frequently Asked Questions about mid-term forecasting
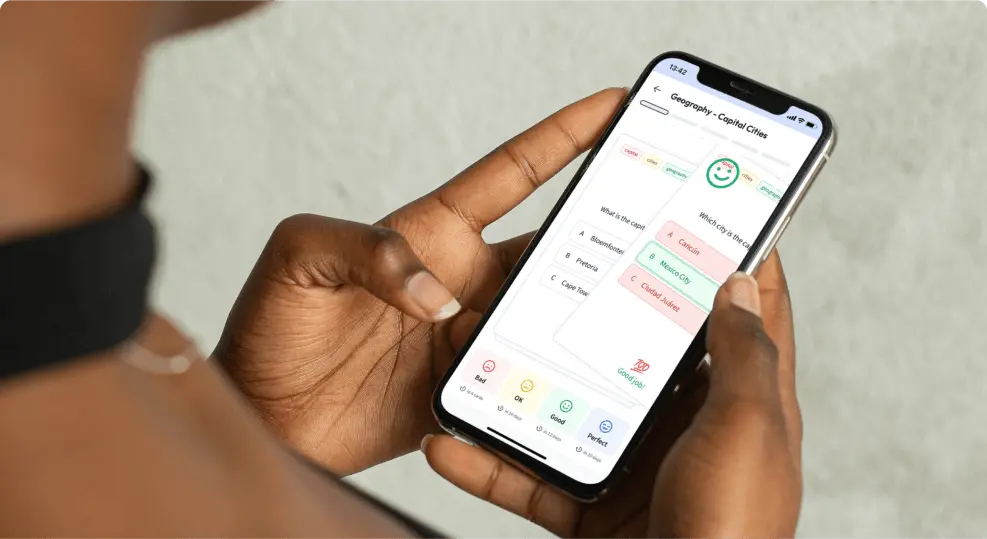
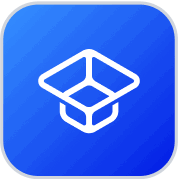
About StudySmarter
StudySmarter is a globally recognized educational technology company, offering a holistic learning platform designed for students of all ages and educational levels. Our platform provides learning support for a wide range of subjects, including STEM, Social Sciences, and Languages and also helps students to successfully master various tests and exams worldwide, such as GCSE, A Level, SAT, ACT, Abitur, and more. We offer an extensive library of learning materials, including interactive flashcards, comprehensive textbook solutions, and detailed explanations. The cutting-edge technology and tools we provide help students create their own learning materials. StudySmarter’s content is not only expert-verified but also regularly updated to ensure accuracy and relevance.
Learn more