Jump to a key chapter
Definition of Quantitative Risk
Quantitative risk refers to the numerical assessment of potential adverse events or losses associated with a business decision or strategy. It involves using mathematical, statistical, and numerical techniques to estimate the likelihood and impact of risks within an organization. Understanding quantitative risk allows businesses to make informed decisions and develop effective risk management strategies.Quantitative risk provides a clear framework for evaluating threats and can be applied across various sectors, including finance, manufacturing, and healthcare. It involves both the identification and evaluation of risks to minimize potential negative outcomes.
Components of Quantitative Risk Analysis
Quantitative risk analysis comprises several key components that you should be familiar with:
- Probability: This involves determining the likelihood of a risk event occurring, which is typically expressed as a percentage.
- Impact: This refers to the potential consequences or losses resulting from the risk event, usually quantified in financial terms.
- Risk Assessment: This combines probability and impact to quantify risk levels using mathematical formulas and statistical models.
- Mitigation: Strategies are developed and implemented to reduce overall exposure to risk.
- Monitoring: Continuous observation is needed to manage risks effectively over time.
In quantitative risk analysis, the term risk quantification is crucial. It is the process of determining the value of the risk using mathematical models and calculations, typically represented as:\[Risk \, Value = Probability \, of \, Occurrence \times Impact\]
For instance, consider a company is investing in a new project with a 20% probability of failing, resulting in a potential loss of $100,000. The risk quantification formula would be:\[Risk \, Value = 0.20 \times 100,000 = 20,000\]This indicates a risk value of $20,000, which assesses the financial exposure linked to the project's failure.
Use software tools specifically designed for risk analysis, such as Monte Carlo simulations or proprietary risk management platforms, to support quantitative risk assessments.
While quantitative risk provides numerical precision, it has certain limitations that must be acknowledged for holistic risk management.Primary limitations include:
- Data Availability: Accurate risk quantification relies heavily on access to high-quality, relevant data. Insufficient data may lead to misleading results.
- Complex Models: The complexity of mathematical models can be a barrier for individuals lacking specialized training, hindering comprehension and practical application.
- Dynamic Environments: Risks can evolve over time, and quantitative models require regular updates to stay relevant, which can be resource-intensive.
- Integration with Qualitative Analysis: Combining quantitative analysis with qualitative insights offers a more comprehensive risk perspective.
Quantitative Risk Analysis in Business Studies
Understanding quantitative risk analysis is essential for grasping potential financial implications of uncertain events. This approach evaluates risks using numerical data, helping businesses identify, manage, and mitigate risks effectively. By focusing on probabilities and impacts, quantitative analysis enables informed decision-making.
Tools and Techniques for Quantitative Risk Analysis
Various tools and techniques are employed in quantitative risk analysis to provide accurate assessments. Here are some common methods used:
- Monte Carlo Simulation: Generates possible outcomes of a decision by running simulations, each time using different sets of random variables.
- Decision Trees: Provides a systematic, graphic representation of various decision paths and their potential outcomes.
- Value at Risk (VaR): Estimates the maximum potential loss in value of an asset or portfolio over a specific period for a given confidence interval.
- Sensitivity Analysis: Examines how different values of an independent variable will impact a particular dependent variable under a given set of assumptions.
In quantitative risk analysis, the term Expected Monetary Value (EMV) is crucial. It is calculated as:\[EMV = \sum (Probability \times Impact)\] and helps in determining the average outcome when considering future potential risks.
Consider an example where a company faces two potential risks:
Risk | Probability | Impact |
Market Decline | 10% | $50,000 loss |
Technical Failure | 5% | $10,000 loss |
Always ensure to cross-verify the underlying assumptions and data used in quantitative risk models to maintain accuracy and reliability in results.
Incorporating stress testing into quantitative risk analysis can further enhance understanding of potential vulnerabilities. Stress tests involve assessing how a business would perform under hypothetical adverse scenarios.Key components to consider during stress testing include:
- Identifying extreme yet plausible scenarios (e.g., economic recession, natural disasters).
- Evaluating the financial and operational impact of these scenarios.
- Developing contingency plans and strategies to ensure business continuity.
Quantitative Risk Techniques in Business Studies
In business studies, quantitative risk techniques play a vital role in analyzing and managing potential threats. These techniques rely on numerical data to evaluate the probability and impact of various risks, enabling businesses to make calculated decisions.
Common Techniques for Quantitative Risk Analysis
To effectively analyze risk, several techniques are commonly used:
- Monte Carlo Simulation: This technique applies computational algorithms that rely on repeated random sampling to predict the behavior of a system. It helps visualize potential outcomes of a risky decision.
- Decision Trees: These graphic representations outline different decision paths along with their associated risks and rewards, aiding in systematic decision-making.
- Value at Risk (VaR): This method estimates the greatest potential loss of an asset or portfolio for a given confidence level over a set period.
- Sensitivity Analysis: Evaluates how changes in input variables impact the outcome, thereby identifying which variables influence risk the most.
The Expected Monetary Value (EMV) is a critical calculation for quantitative risk. It is represented as:\[EMV = \sum (Probability \times Impact)\] and it aids in determining the average projected value of various risk scenarios.
Consider a business facing two potential risks:
Risk | Probability | Impact |
Market Downturn | 15% | $40,000 loss |
Data Breach | 10% | $20,000 loss |
Regular updates of quantitative risk models are crucial as they provide relevant assessments responsive to market changes.
Integrating stress testing with quantitative risk techniques provides additional insights into extreme risk scenarios and business vulnerabilities. Stress tests evaluate an organization's resilience under adverse conditions beyond normal risk analysis.Stress Testing Considerations:
- Identify plausible, impactful scenarios such as economic recessions or breach of security
- Analyze potential financial and operational impacts
- Adapt risk mitigation strategies based on test outcomes
Quantitative Risk Management Strategies
To effectively manage risks in business, you must employ quantitative risk management strategies. These strategies use numerical data to identify and address potential threats, ensuring informed decision-making and risk mitigation.
Quantitative Risk Assessment Steps
Conducting a thorough risk assessment involves several key steps:
- Risk Identification: Recognize all potential threats that could impact business objectives.
- Risk Quantification: Use statistical and mathematical models to measure the likelihood of each risk and its impact. This often involves using formulas such as:\[Risk \, Value = Probability \times Impact\]
- Prioritization: Rank risks based on their quantified impact and probability, focusing on those with the highest risk values.
- Mitigation Planning: Develop strategies to reduce or eliminate the biggest risks. This can involve diversifying investments, buying insurance, or implementing robust security systems.
- Implementation and Monitoring: Execute the mitigation plans and continuously monitor the business environment for changes or new risks.
Let's say a company wants to assess the risks related to launching a new product. During the risk identification phase, the company may find the possibility of a competitor launching a similar product (Probability = 25%) and a risk of production delay (Probability = 10%). Assessing the impact of the competitor launching a similar product results in a potential loss of revenue of $200,000, while the production delay could lead to a $50,000 loss. Using risk quantification:
Description | Probability | Impact | Risk Value |
Competitor Product | 25% or 0.25 | $200,000 | \(0.25 \times 200,000 = 50,000\) |
Production Delay | 10% or 0.10 | $50,000 | \(0.10 \times 50,000 = 5,000\) |
Utilize quantitative software tools that offer simulations and statistical analysis to streamline the assessment process.
Examples of Quantitative Risk in Business
In business, quantitative risk examples often involve assessing financial exposures. Here are some practical examples:
- Stock Market Investment: Calculating the potential losses due to market volatility by determining the likelihood of adverse price movements and their financial impact using Value at Risk (VaR).
- Project Management: Analyzing cost overruns by assessing the probability of delays and additional expenses, then quantifying the resultant financial pressure on project budgets.
- Currency Fluctuation: Estimating the risk of foreign exchange rate changes on international transactions impacting revenue and profit margins.
While applying quantitative risk in examples, businesses can include a sophisticated modeling technique known as real options analysis. This involves evaluating the flexibility in investment decisions when facing uncertain market conditions. It allows businesses to model multiple paths and strategic alternatives, evaluating scenarios such as:
- Expanding operations when market conditions are favorable.
- Deferring projects during high-risk periods to minimize potential losses.
- Abandoning unfavorable ventures early to save resources and reallocate.
Benefits of Quantitative Risk Analysis
Employing quantitative risk analysis offers numerous advantages in business decision-making, including:
- Precision: Provides numerical insights into potential risks, enabling precise risk measurement and prioritization.
- Informed Decision-Making: Facilitates data-driven decisions by supplying a solid analytical foundation.
- Resource Allocation: Helps allocate resources efficiently by identifying priority risk areas needing mitigation strategies.
- Strategic Planning: Supports long-term planning by evaluating the potential impacts and probabilities of future events.
- Improved Risk Awareness: Enhances understanding and awareness of potential threats across different business sectors.
quantitative risk - Key takeaways
- Definition of Quantitative Risk: Numerical assessment of potential adverse events or losses using mathematical, statistical, and numerical techniques.
- Quantitative Risk Components: Probability (likelihood of risk), Impact (potential consequences), Risk Assessment, Mitigation, Monitoring.
- Risk Quantification Formula: Calculated as Risk Value = Probability of Occurrence × Impact.
- Quantitative Risk Techniques in Business Studies: Includes Monte Carlo Simulation, Decision Trees, Value at Risk (VaR), Sensitivity Analysis.
- Quantitative Risk Management: Involves risk identification, risk quantification, prioritization, mitigation planning, implementation, and monitoring.
- Examples of Quantitative Risk in Business: Stock Market Investment, Project Management, Currency Fluctuation.
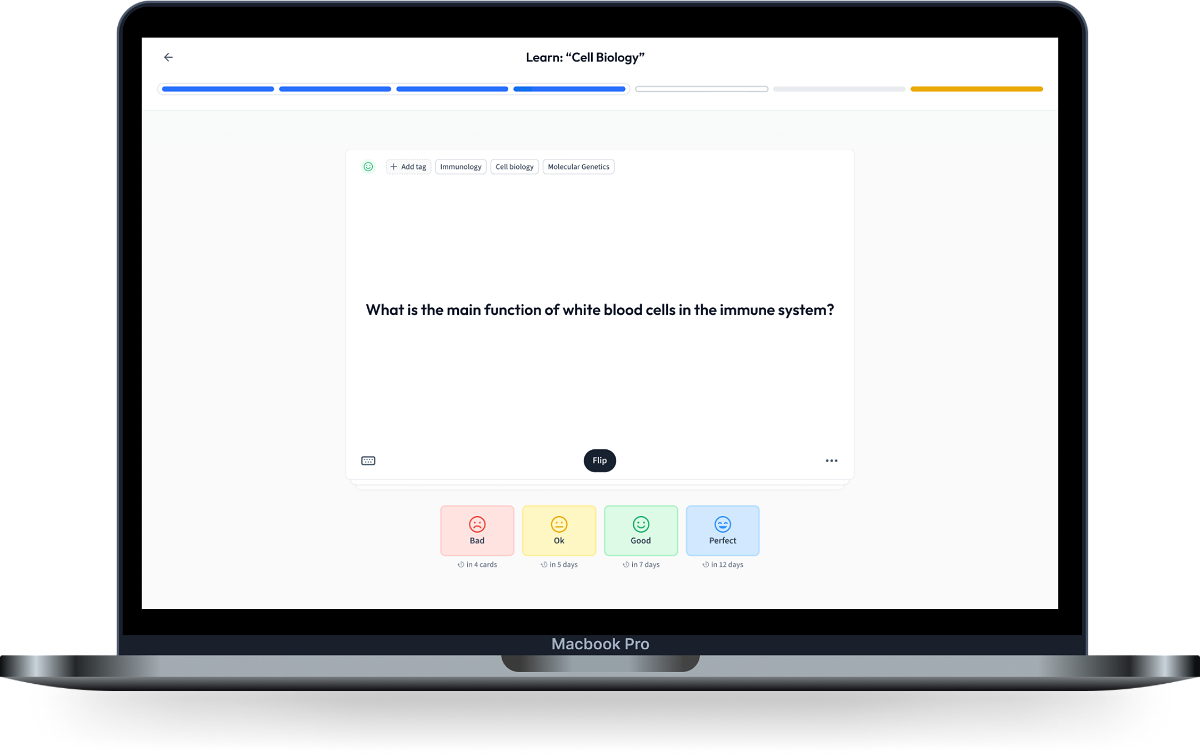
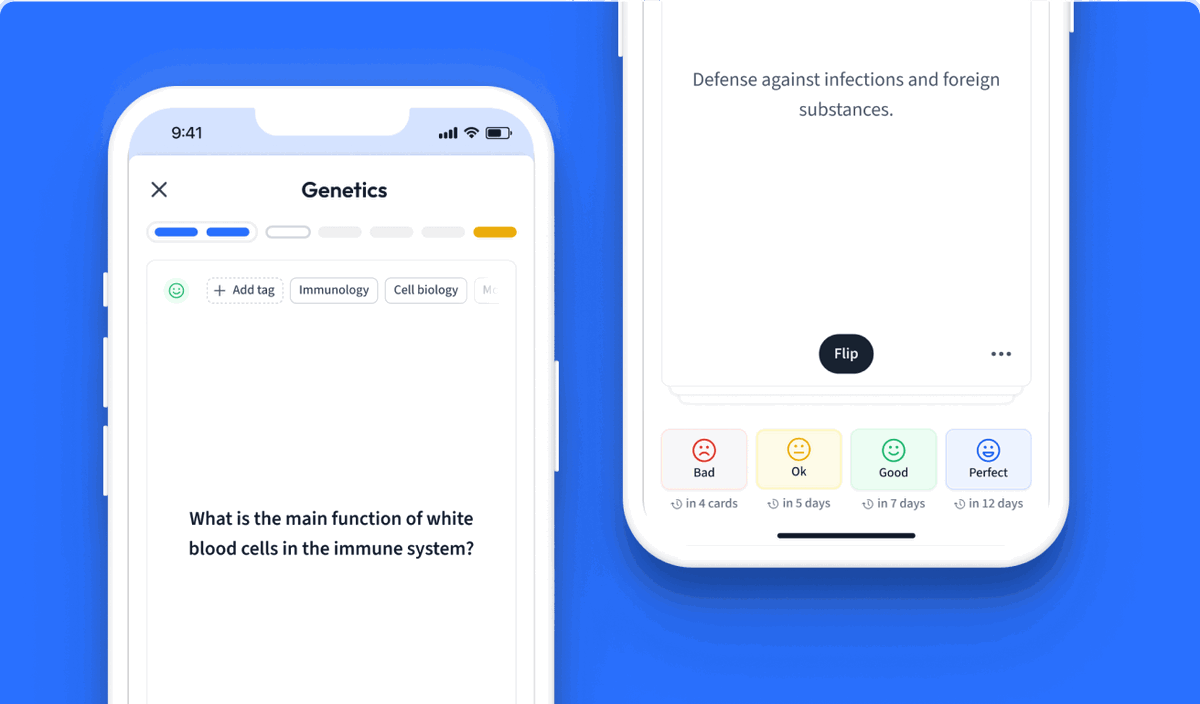
Learn with 24 quantitative risk flashcards in the free StudySmarter app
We have 14,000 flashcards about Dynamic Landscapes.
Already have an account? Log in
Frequently Asked Questions about quantitative risk
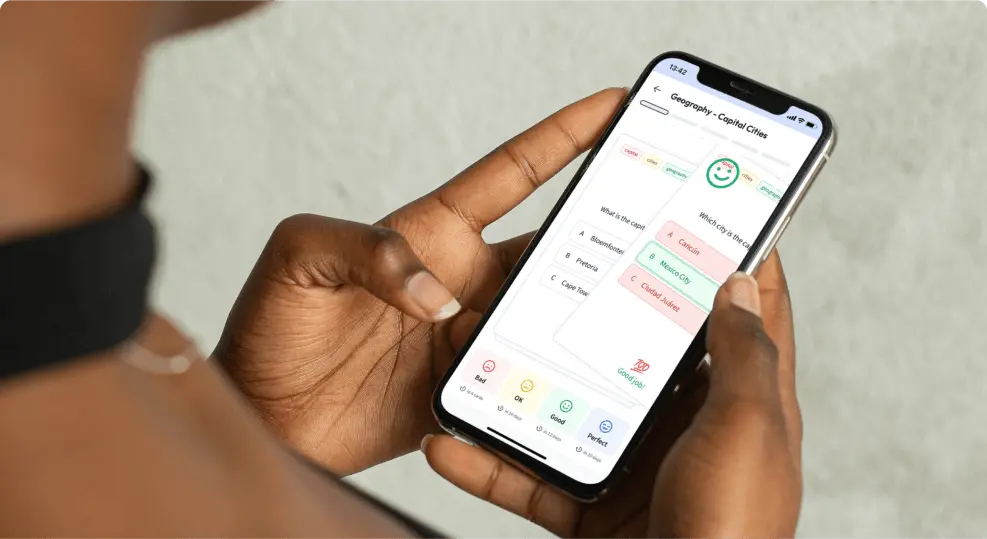
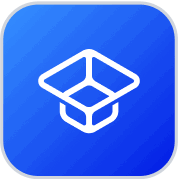
About StudySmarter
StudySmarter is a globally recognized educational technology company, offering a holistic learning platform designed for students of all ages and educational levels. Our platform provides learning support for a wide range of subjects, including STEM, Social Sciences, and Languages and also helps students to successfully master various tests and exams worldwide, such as GCSE, A Level, SAT, ACT, Abitur, and more. We offer an extensive library of learning materials, including interactive flashcards, comprehensive textbook solutions, and detailed explanations. The cutting-edge technology and tools we provide help students create their own learning materials. StudySmarter’s content is not only expert-verified but also regularly updated to ensure accuracy and relevance.
Learn more