Jump to a key chapter
Actuarial Forecast Definition
Actuarial Forecast is a crucial concept in financial and business sectors. It employs mathematical and statistical methods to predict future events that impact insurance, pensions, and investments. By doing so, it assists in decision-making processes for businesses and financial organizations.
Actuarial Forecast refers to the utilization of statistical techniques to estimate the likelihood of future events, particularly in finance and insurance domains. This forecast aids businesses in risk management and financial planning.
The Role of Actuarial Forecast in Business Decisions
In business environments, actuarial forecasts are vital for shaping strategic initiatives. They help in evaluating risks and determining potential outcomes. Here's how actuarial forecasts support business activities:
- Predicting financial liabilities in insurance and pension plans.
- Managing investment risks by analyzing future market trends.
- Assisting in pricing strategies for insurance products.
- Supporting enterprise risk management.
Suppose an insurance company wants to introduce a new life insurance policy. An actuarial forecast can employ historical data and mortality rates to predict possible claim amounts. For instance, if a trend shows that 5 out of 1000 claimed previously, this statistical probability helps in setting premiums, ensuring profitability.
Mathematical Models in Actuarial Forecasts
Actuarial forecasts heavily rely on mathematical models. These models utilize a range of variables to predict future events. Common models include:
- Life tables: Used to calculate mortality rates and project future life spans.
- Stochastic models: Incorporate randomness to simulate a variety of potential outcomes.
- Deterministic models: Based on set variables to estimate a consistent outcome.
Utilizing a blend of stochastic and deterministic models allows actuaries to forecast a range of possible future scenarios, enhancing accuracy.
Challenges in Developing Actuarial Forecasts
While actuarial forecasts are invaluable, they are not without challenges. Some of the key difficulties include:
- Data limitations: Incomplete or biased data can skew forecasts.
- Changing factors: Economic and regulatory changes can affect accuracy.
- Model risk: Assumptions in models may not always align with real-world events.
One of the most advanced techniques in actuarial forecasting today involves machine learning algorithms. These algorithms can process vast amounts of data quickly and efficiently, identifying patterns that traditional models may miss. By automating the actuarial forecasting processes, businesses can now achieve higher accuracy and adapt more swiftly to changes in their operational landscape. Machine learning enhances traditional actuarial methods by:
- Providing real-time data analysis to predict market shifts immediately.
- Reducing human bias in model predictions.
- Offering scalability for large datasets beyond conventional capabilities.
Actuarial Forecast Examples
Actuarial forecasts are essential tools in the financial sector, providing predictions based on statistical analysis. These forecasts inform a range of monetary decisions and risk assessments. Let's explore some examples to see how these predictions are used.In the insurance industry, actuarial forecasts help determine the premium rates. Insurers use historical claims data and statistical methods to estimate the likelihood and cost of future claims. By doing so, they ensure that the premium covers potential risks while remaining competitive.In pensions, actuarial forecasts help in calculating the future financial commitments of a pension plan. Actuaries consider factors like employee age demographics and expected retirement duration to ensure sufficient funds are available when liabilities arise.
An example of an actuarial forecast is in car insurance. A company might analyze accident statistics over the past decade to predict the cost of future claims. If data shows that younger drivers are more prone to accidents, the company might set higher premiums for this age group. Actuaries might use a formula like:\[P = L \times F\]where P represents the premium, L is the expected loss, and F is the loading factor for administrative costs and profit margins.
Another application is in risk management for investments. Actuarial forecasts assess economic factors like interest rate fluctuations by using economic models to predict future portfolio performance. This helps financial advisors tailor investment strategies to suit the risk appetite and expectations of clients.To delve deeper, consider a simplified model where the expected future value of an investment can be calculated using the formula:\[FV = PV \times (1 + r)^t\]Here, FV is the future value, PV is the present value, r is the annual interest rate, and t is the time period in years.
Always adjust actuarial forecasts as new data and economic conditions emerge, ensuring predictions remain relevant and accurate.
In recent years, the integration of big data and artificial intelligence in actuarial forecasts has revolutionized the field. By leveraging machine learning algorithms, actuaries can analyze vast datasets to identify intricate patterns and trends that were previously challenging to recognize. This approach allows for more granular analysis and can be particularly effective in:
- Evaluating consumer behavior in real-time to adjust insurance premiums dynamically.
- Monitoring and predicting long-term impacts of climate change on crop insurance.
- Optimizing health insurance plans by analyzing large datasets of medical records and claims.
Actuarial Forecasting Techniques
Actuarial forecasting involves various techniques that provide businesses with a view into potential future financial events by analyzing data and applying statistical models. These techniques are crucial for risk management, especially in the insurance and finance industries. Let's explore these methodologies and see how they are practically applied in business settings.
Actuarial Forecast Techniques in Practice
Several key techniques are utilized in creating actuarial forecasts. Understanding these methods is essential for any aspiring actuary.
- Survival Models: These models are used to predict life expectancy and the probability of survival at different ages.
- Credibility Theory: A method of improving premium predictions by adjusting past observed experience with relevant supplementary information.
- Monte Carlo Simulations: Used for risk assessment and predictive modeling by running numerous simulations under varied assumptions.
- Regression Analysis: Establishes relationships between variables to forecast future trends.
Consider a health insurance company aiming to set premiums for a new policy. Actuaries might use survival models to estimate the life expectancy of policyholders. They might calculate the probability of survival using a formula such as:\[S(t) = e^{-\text{H}(t)}\]where S(t) represents the survival function, and H(t) is the cumulative hazard at time t. These calculations assist in estimating the financial risk associated with the policy.
In practice, actuaries combine multiple techniques to produce more reliable and comprehensive forecasts.
Actuarial Science Forecasting Methods
Actuarial science offers a range of forecasting methods that cater to various financial challenges. Understanding these methods is vital for predicting and mitigating future risks.
- Time Series Analysis: Analyzes past data trends to forecast future values, often used in financial market predictions.
- Lifecycle Modeling: Used in pension schemes to predict long-term fund requirements based on employee demographics.
- Loss Distribution Approach: Estimates potential losses by modeling the underlying statistical distribution of losses experienced.
Advances in technology have bolstered actuarial science with additional forecasting capabilities. For instance, machine learning algorithms enable actuaries to analyze enormous datasets quickly and accurately, going beyond the constraints of traditional models. Such technology-driven approaches are integral to grasping complex patterns and anomalies in financial datasets.Machine learning methods such as neural networks, random forests, and support vector machines empower actuaries to build adaptive models that continuously learn and refine forecasts based on new data inputs. This can improve predictions in challenging areas like fraud detection and claims management.A Table of Techniques:
Technique | Application |
Neural Networks | Pattern recognition in large datasets |
Random Forests | Decision making in complex multi-variable datasets |
Support Vector Machines | Classifying and analyzing data points |
Actuarial Forecast Application
In the realm of business and finance, actuarial forecasts play a pivotal role in predicting future events and managing risks. These forecasts utilize statistical and mathematical models to analyze historical data, providing insights that guide decision-making. Here, you will explore the various ways actuarial forecasts are applied across different sectors.
Insurance Sector Applications
Within the insurance sector, actuarial forecasting is fundamental to setting premiums and managing reserves. Actuaries assess a wide array of risks, from natural disasters to changes in life expectancy, using models such as:
- Mortality Tables: Predict the probability of death at each age, which is essential for life insurance.
- Loss Models: Estimate the frequency and severity of claims.
- Catastrophe Models: Assess the impact of extreme events like hurricanes and earthquakes.
An example of actuarial forecast application in insurance is determining auto insurance premiums. Insurers use historical data and regression analysis to predict the likelihood of an accident for different driver profiles. Consider a formula:\[P = \frac{L}{(1 + i)^n} \]where P is the premium, L is the expected loss, i is the interest rate, and n is the number of periods.
Insurance companies often use multi-variate analysis to see how different factors like age and vehicle type influence accident probabilities.
Pension Fund Management
In pension fund management, actuarial forecasts are critical for ensuring that there are adequate funds available to meet future liabilities. Actuaries use lifecycle models to project the long-term financial status of a pension plan, considering factors such as contribution rates, workforce demographics, and expected return on investments. Calculations might involve present value formulas like:\[PV = \frac{FV}{(1 + r)^t} \]where PV signifies the present value, FV is the future value, r is the discount rate, and t is the time period. These calculations help in determining necessary adjustments to contribution rates or investment strategies.
In addition to standard actuarial models, pension fund management increasingly uses stochastic simulations to account for uncertainty in demographic and financial assumptions. These simulations model thousands of paths of potential demographic and financial variables, providing a distribution of possible future outcomes. Such an approach helps identify scenarios where the plan might become underfunded, thereby enabling proactive strategies. The integration of machine learning further enhances precision by identifying complex patterns in historical data, which might affect future forecasts. This includes adapting contributions based on real-time data analysis and using algorithms to optimize investment strategies in light of forecasted economic conditions. Such technology-driven methods are transforming traditional pension fund management, offering a more resilient means of ensuring financial security for future retirees.
actuarial forecast - Key takeaways
- Actuarial Forecast Definition: Utilization of statistical techniques to predict future events in finance and insurance for risk management and planning.
- Key Business Applications: Used for predicting financial liabilities, managing investment risks, pricing insurance products, and supporting risk management.
- Examples of Actuarial Forecasts: Determining insurance premium rates and calculating financial commitments in pension plans.
- Common Forecasting Techniques: Includes life tables, stochastic models, deterministic models, survival models, and more.
- Challenges in Actuarial Forecasting: Data limitations, changing economic factors, and model risks affecting forecast accuracy.
- Advanced Techniques: Integration of machine learning for pattern recognition and enhanced predictive accuracy in actuarial science.
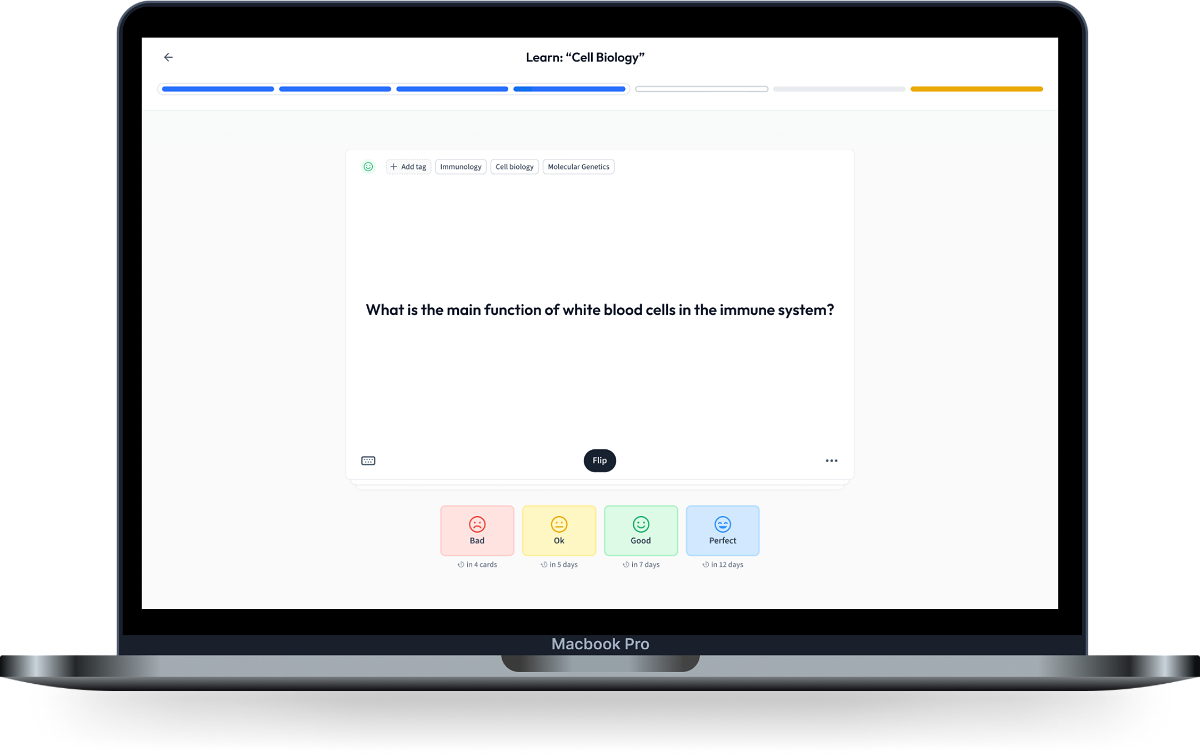
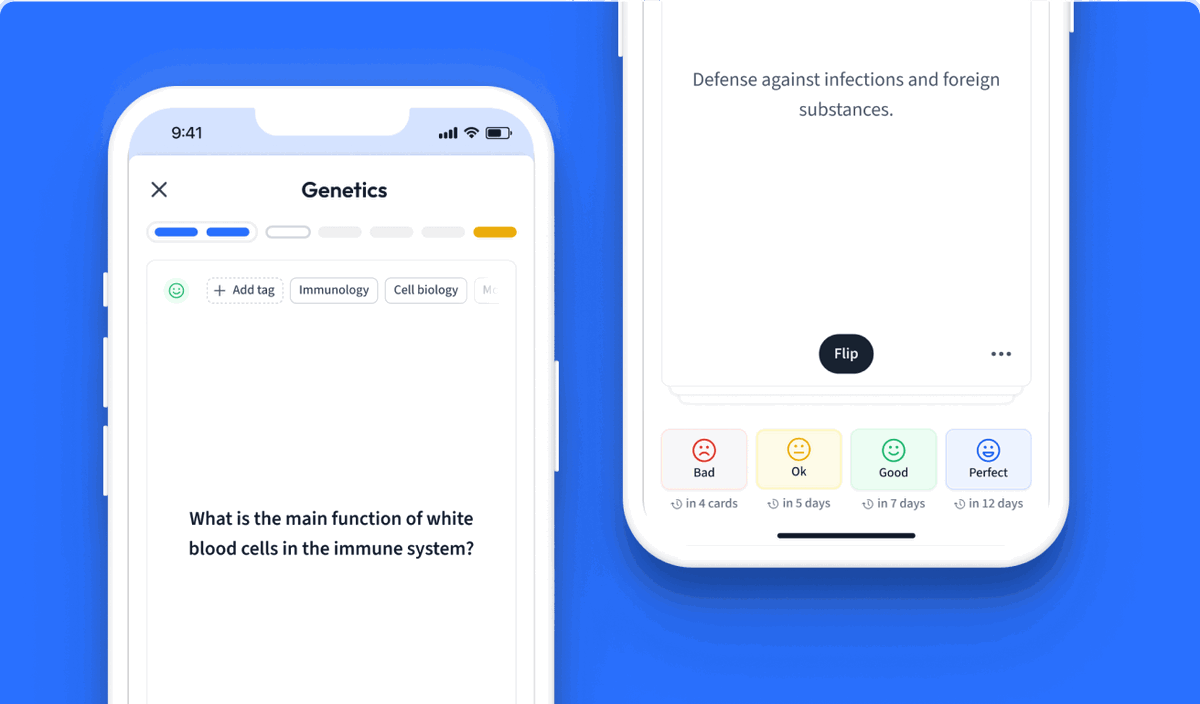
Learn with 24 actuarial forecast flashcards in the free StudySmarter app
We have 14,000 flashcards about Dynamic Landscapes.
Already have an account? Log in
Frequently Asked Questions about actuarial forecast
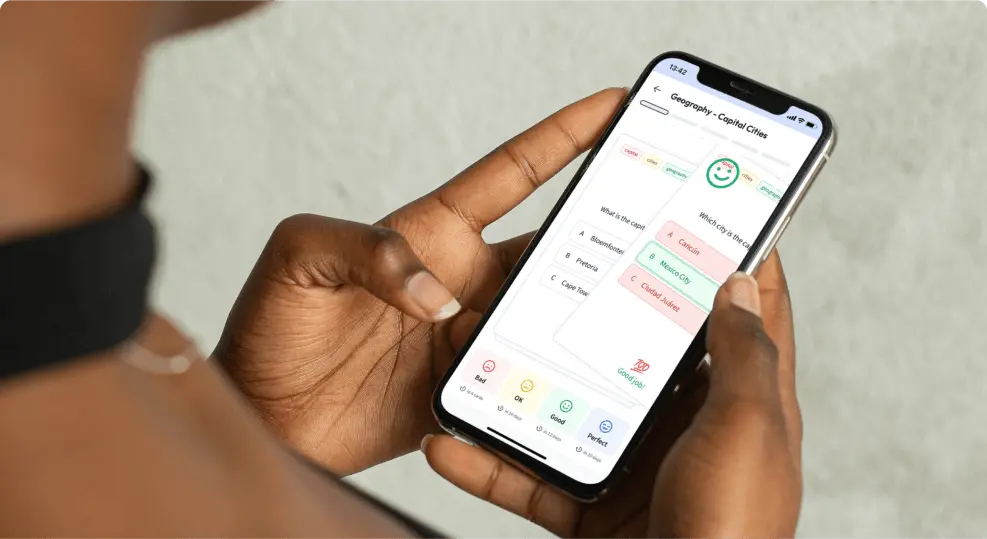
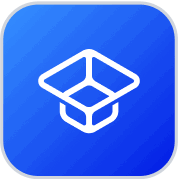
About StudySmarter
StudySmarter is a globally recognized educational technology company, offering a holistic learning platform designed for students of all ages and educational levels. Our platform provides learning support for a wide range of subjects, including STEM, Social Sciences, and Languages and also helps students to successfully master various tests and exams worldwide, such as GCSE, A Level, SAT, ACT, Abitur, and more. We offer an extensive library of learning materials, including interactive flashcards, comprehensive textbook solutions, and detailed explanations. The cutting-edge technology and tools we provide help students create their own learning materials. StudySmarter’s content is not only expert-verified but also regularly updated to ensure accuracy and relevance.
Learn more