Jump to a key chapter
Definition of Actuarial Risk Theory
Actuarial Risk Theory is a fundamental framework used in the insurance industry and financial sectors to assess, quantify, and manage uncertainty and potential risks. It employs various mathematical and statistical models to predict the financial implications of uncertain future events.
Understanding Actuarial Risk Theory
At its core, actuarial risk theory aims to determine how likely certain events are to occur and what risks these events pose. This involves calculating probabilities and potential financial impacts of events such as natural disasters, accidents, or life events, like death or retirement. Actuaries use this theory to help organizations create policies that minimize incoming risks while ensuring sustainability. To understand how this works, an actuary would employ statistical methods to model how likely certain events are to occur, applying these models to make decisions about reserves and pricing. The principle formula often used in this context is the expected value formula, represented as:
- Expected Value: \(E(X) = \sum (x_i \cdot P(x_i))\)
Expected Value is a central concept in probability and statistics, representing the average outcome when an experiment is repeated many times.
For example, consider an insurance company trying to estimate the potential losses from insuring cars. Suppose there are three scenarios: minor accidents, major accidents, and no accidents, with respective probabilities of 0.1, 0.3, and 0.6, and corresponding financial consequences of $1,000, $10,000, and $0. The expected value of the financial impact is therefore calculated as:
- \(E(X) = (0.1 \times 1000) + (0.3 \times 10000) + (0.6 \times 0) = $3,100\)
Actuaries often use Monte Carlo simulations to assess risk in more complex scenarios where analytical solutions are not feasible.
Monte Carlo simulations are powerful tools in actuarial science. They use random sampling to calculate results about an objective, often employed when there are numerous variables or highly stochastic environments. For instance, the simulation might model thousands of scenarios, each simulating the potential outcome of insuring a vehicle over a year.These simulations take into account numerous risk factors like driver age, vehicle type, weather conditions, and potential accidents. Monte Carlo simulation output provides a probability distribution of possible outcomes, allowing actuaries to foresee potential risks under different conditions. This insight is essential for making informed decisions about risk management strategies, pricing policies, and financial planning. While these methods are sophisticated, it’s crucial to input unbiased and accurate data into the model to derive trustworthy outcomes.
Meaning of Actuarial Risk Theory in Business
Actuarial Risk Theory plays a crucial role in the business sector, particularly within the insurance and finance industries. It is a scientific approach that involves utilizing mathematics and statistics to assess and manage future risks.
Role in Insurance and Finance
Actuarial risk theory is essential in helping businesses predict and understand potential liabilities. This involves:
- Calculating the likelihood of events such as natural disasters or accidents.
- Estimating potential financial impacts.
- Developing strategies to mitigate risks.
Risk Assessment is the identification and analysis of potential risks that could negatively impact key business initiatives or projects.
Imagine a life insurance company that needs to calculate the premium for a new policy. An actuary would:
- Analyze statistical data on life expectancy and factors such as age and health conditions.
- Use this data in actuarial models to estimate the probability of paying out the policy within certain periods.
- Adjust the premium pricing based on the estimated risk and expected payout.
Actuarial risk theory relies heavily on mathematical tools, such as:
Statistical Distribution | A mathematical function that describes all the possible values and likelihoods that a random variable can take within a given range. |
Probability Theory | Used to predict future events and manage risks associated with uncertain outcomes. |
Stochastic Processes | A statistical process involving a sequence of random variables to model systems that evolve over time. |
In advanced applications, actuaries might employ complex models, including finite mixture models to represent the probability distribution of risks more accurately. These models help in capturing the uncertainty and variability of risk data more effectively. Furthermore, the use of Copulas allows actuaries to model and analyze the dependencies between different risk factors. For instance, considering how various economic indicators like inflation and interest rates together impact an insurance portfolio, rather than viewing them in isolation. This multivariate approach provides a comprehensive risk management framework that can be critical in an interconnected financial environment.
Modern Actuarial Risk Theory Developments
Modern advancements in actuarial risk theory have led to more sophisticated tools and models, enabling actuaries to better predict and manage risks in dynamic environments. These developments are crucial in addressing contemporary challenges in risk assessment and management.
Advancements in Risk Modeling Techniques
Recent improvements in actuarial risk theory involve the integration of advanced technology and statistical methods. Some impactful developments include:
- Machine Learning Algorithms: Used for identifying patterns and predicting potential risks based on large datasets.
- Big Data Analytics: Allows actuaries to analyze vast amounts of data for more accurate risk forecasts.
- Dynamic Financial Analysis (DFA): Simulates a company's financial condition under various scenarios to better gauge risk exposure.
Machine Learning refers to the use of algorithms and statistical models that enable computers to perform tasks without explicit instructions, relying on patterns and inference.
Imagine an insurance company wanting to forecast claims costs over the next year. By applying machine learning algorithms to historical claims data, the company can:
- Identify trends and correlations not evident through traditional methods.
- Forecast potential future claims with a higher degree of accuracy.
- Implement pricing models that better reflect future risk levels.
Quantum computing is an emerging area showing potential in solving complex risk management problems more efficiently than classical computing methods.
Quantum computing, although still in its nascent stage, promises to revolutionize the field of risk management. By handling vast datasets simultaneously, quantum computers could solve complex problems in seconds that would take traditional computers hours or days. Quantum Risk Modeling: This approach involves leveraging quantum algorithms to run extensive simulations, thus providing actuaries with unmatched insights into potential risk events. For instance, the application of quantum annealing processes can optimize portfolio management, reducing risks and enhancing returns on investment. While practical applications are only beginning to emerge, the integration of quantum computing with actuarial risk theory holds promise for future advancements, offering new, efficient methods to tackle existing and unforeseen challenges in the field.
Actuarial Risk Technique Example in Practice
Understanding the practical application of actuarial risk theory involves analyzing real-world scenarios where actuarial methods are used to manage and predict risks effectively. These applications are crucial in various sectors, notably in insurance and finance.
Application of Risk Theory in Actuarial Science
In actuarial science, risk theory is integral for assessing potential risks and their financial impacts. Actuaries apply it through:
- Calculating life expectancy and mortality rates for life insurance policies.
- Forecasting potential claims in property and casualty insurance.
- Analyzing retirement benefits and pension fund management.
Suppose an actuary needs to calculate the premium for a health insurance policy. By analyzing historical data and applying risk models, the actuary can:
- Determine the expected frequency and cost of claims.
- Adjust the premium pricing based on the estimated likelihood of claims.
- Ensure that the policy remains profitable while offering competitive terms.
Importance of Actuarial Risk Theory in Decision Making
Actuarial risk theory informs crucial decisions in the business world by providing quantitative assessments of risks. Decisions influenced by this theory include:
- Determining reserves for insurance companies to cover potential claims.
- Setting prices for insurance products to stay competitive while balancing risk.
- Developing strategies for investment risks and managing retirement funds.
Decision-makers in finance often use scenario analysis alongside actuarial risk theory to explore the potential outcomes of various strategic options.
Tools and Techniques in Modern Actuarial Risk Theory
Modern actuarial risk theory incorporates sophisticated tools and techniques to address complex risks. Key developments include:
- Monte Carlo Simulations: A method for modeling the probability of different outcomes to understand potential risks.
- Data Analytics: Leveraging big data to enhance the accuracy of risk predictions.
- Stress Testing: Assessing the resilience of financial portfolios under adverse conditions.
A deep dive into stress testing reveals its importance in assessing financial stability. Organizations use this technique to simulate potential crises and evaluate their capacity to absorb shocks. In stress testing, actuaries may:
- Analyze how extreme market conditions could impact insurance claims.
- Determine necessary financial buffers to withstand economic downturns.
- Build scenarios involving drastic changes in interest rates or unemployment rates.
How Actuarial Risk Theory Influences Business Strategies
Actuarial risk theory shapes business strategies by offering insights into risk management and financial planning. Companies use actuarial principles to:
- Develop risk-adjusted pricing for products and services.
- Optimize capital allocation for higher returns.
- Design effective risk transfer strategies, such as reinsurance programs.
Reinsurance involves a company purchasing insurance to mitigate the risk of large claims, spreading the potential risk to another entity.
actuarial risk theory - Key takeaways
- Definition of Actuarial Risk Theory: A framework for assessing, quantifying, and managing risks using mathematical and statistical models.
- Purpose: To predict financial implications of uncertain future events in insurance and finance.
- Expected Value Formula: Used by actuaries to model event probabilities and outcomes.
- Monte Carlo Simulations: A tool to evaluate complex risks by modeling numerous scenarios.
- Modern Developments: Integration of machine learning, big data analytics, and dynamic financial analysis into actuarial risk theory.
- Applications: Used in pricing, risk assessment, and developing sustainable business strategies in insurance and finance.
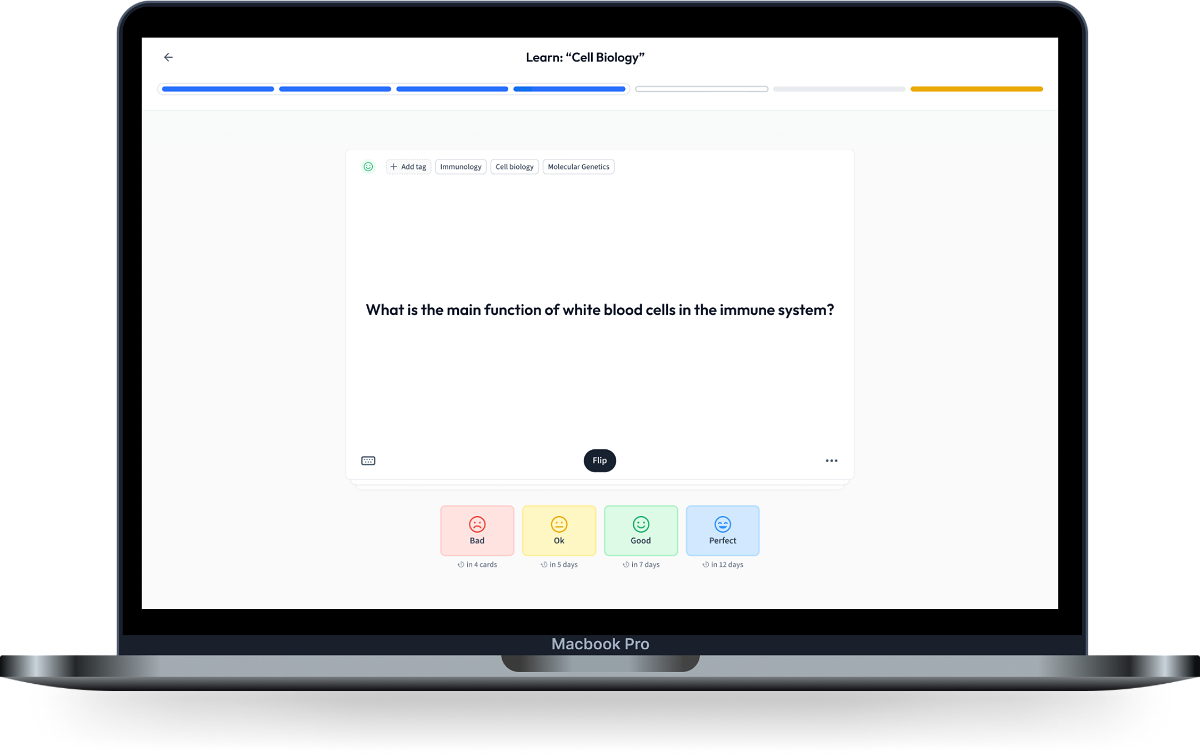
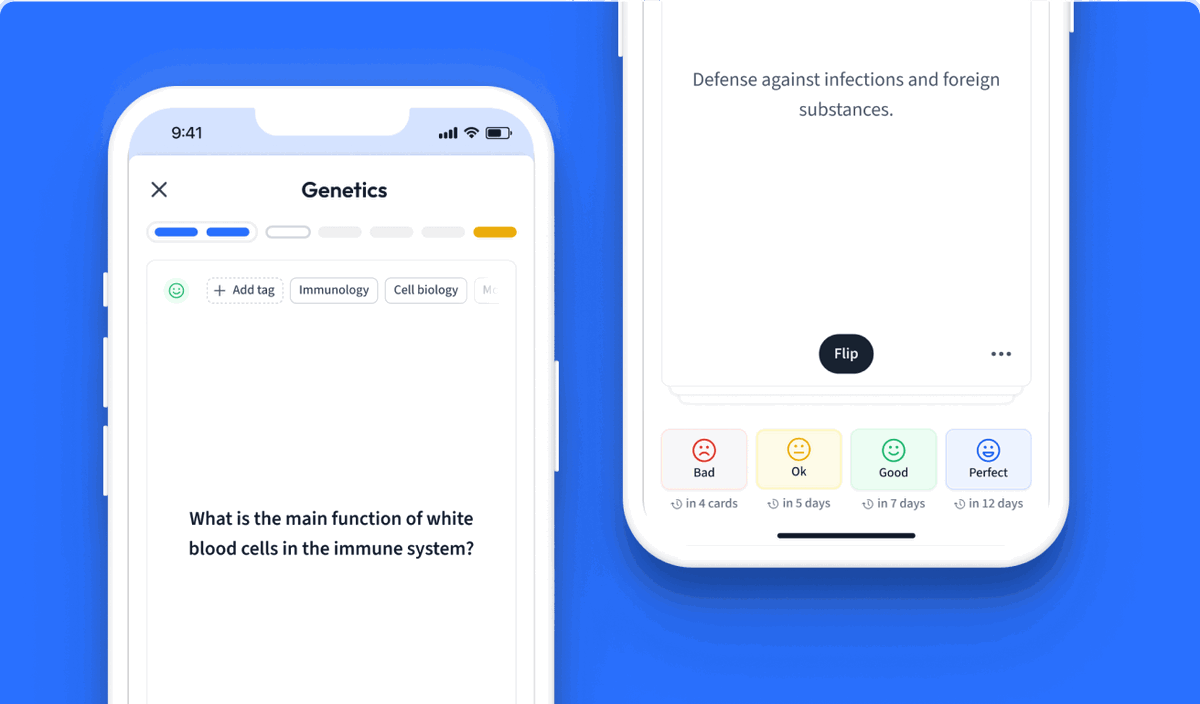
Learn with 24 actuarial risk theory flashcards in the free StudySmarter app
We have 14,000 flashcards about Dynamic Landscapes.
Already have an account? Log in
Frequently Asked Questions about actuarial risk theory
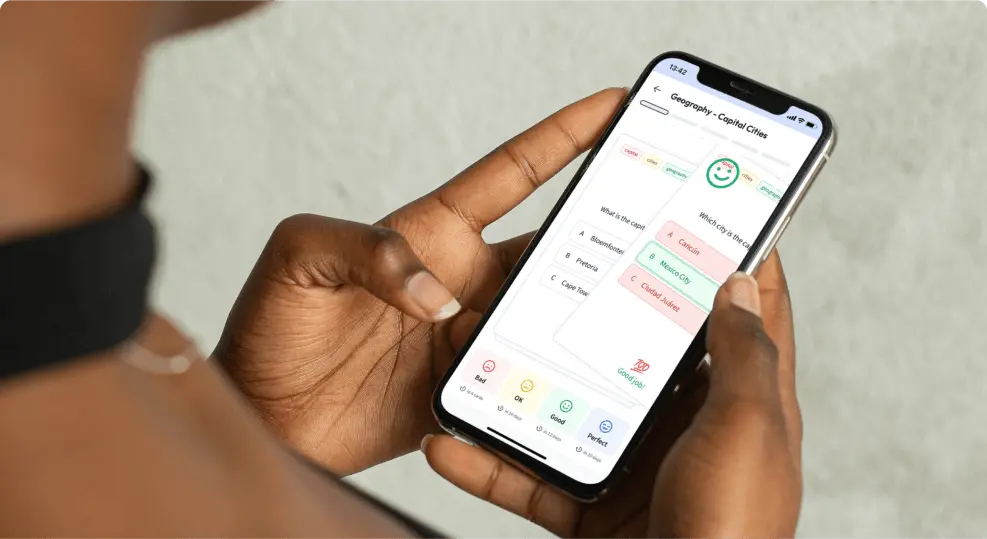
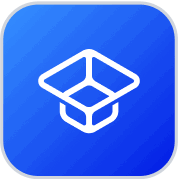
About StudySmarter
StudySmarter is a globally recognized educational technology company, offering a holistic learning platform designed for students of all ages and educational levels. Our platform provides learning support for a wide range of subjects, including STEM, Social Sciences, and Languages and also helps students to successfully master various tests and exams worldwide, such as GCSE, A Level, SAT, ACT, Abitur, and more. We offer an extensive library of learning materials, including interactive flashcards, comprehensive textbook solutions, and detailed explanations. The cutting-edge technology and tools we provide help students create their own learning materials. StudySmarter’s content is not only expert-verified but also regularly updated to ensure accuracy and relevance.
Learn more