Jump to a key chapter
Claim Cost Estimation Definition
Claim cost estimation refers to the process of predicting the financial expenditure required to settle claims in various sectors, particularly in insurance and project management. This estimation plays a pivotal role in financial planning and risk management, ensuring that sufficient resources are allocated to cover potential claims.
Importance of Claim Cost Estimation
Understanding the importance of claim cost estimation is essential for effective financial management. Accurate estimation helps in:
- Budgeting: Anticipates the funds needed for future claims.
- Pricing: Influences the pricing of insurance premiums and project bids.
- Risk Management: Aids in identifying and mitigating potential risks.
- Resource Allocation: Ensures resources are allocated effectively to manage claims.
Claim Cost Estimation Formula: The basic formula used in claim cost estimation can be represented as: \[ E = \text{Frequency of Claims} \times \text{Average Cost per Claim} \] where E is the estimated total cost.
The accuracy of claim cost estimation affects the financial performance and sustainability of organizations involved.
Methods of Claim Cost Estimation
There are several methods used to estimate claim costs effectively:
- Historical Data Analysis: Uses historical claim data to predict future costs.
- Actuarial Techniques: Employs statistical methods to forecast claim costs.
- Modeling: Utilizes mathematical models to simulate possible future scenarios.
- Expert Judgment: Involves the insight and experience of industry experts to make estimates.
Example of Claim Cost Estimation: Suppose an insurance company wants to estimate the total cost of future claims for car accidents. If they expect 100 claims next year and the average cost per claim is $5,000, then the estimated total claim cost would be:\[ E = 100 \times 5000 = 500,000 \] Therefore, the company should allocate $500,000 for car accident claims for that particular year.
A deeper understanding of claim cost estimation involves considering the variability inherent in claim costs, which can be influenced by factors such as inflation, legal changes, and policyholder behavior. Actuaries often use predictive analytics and machine learning models to enhance the accuracy of their estimates. An important mathematical tool in this context is the calculation of the standard deviation of claim costs, which indicates the degree of variability or dispersion from the average cost. This is calculated as follows: \[ \sigma = \sqrt{\frac{1}{N} \sum_{i=1}^{N} (x_i - \mu)^2} \] where \( \sigma \) is the standard deviation, \( x_i \) is each individual claim cost, \( \mu \) is the mean claim cost, and \( N \) is the number of claims. By understanding this variability, companies can better manage their financial reserves and pricing strategies.
Understanding Claim Cost Estimation
The estimation of claim costs is a critical component in financial planning for insurance companies and project managers. Predicting the funds required to address future claims ensures that organizations maintain financial stability.
Factors Influencing Claim Cost Estimation
Several factors play a role in accurately estimating claim costs:
- Historical data: Analyzing past claims to predict future trends.
- Inflation rates: Expected increases in costs due to inflation.
- Legal environment: Changes in laws that affect claim payouts.
- Demographics: Changes in population makeup that might affect claim frequency.
Claim Cost Estimation Formula: The essential formula for estimating claim costs is: \[ E = \text{Frequency of Claims} \times \text{Average Cost per Claim} \] where E is the estimated total expenditure.
Claim cost estimation is crucial in defining the premiums for insurance policies.
Approaches to Claim Cost Estimation
Various methodologies can be employed in estimating claim costs:
- Actuarial methods: Use statistical techniques to project long-term costs.
- Data modeling: Simulate different scenarios using mathematical models.
- Expert judgment: Leverages the insights from experienced professionals.
Example of Estimation: Consider a scenario where a company expects 150 claims at an average cost of $4,500 each. The estimated claim cost would be calculated as follows: \[ E = 150 \times 4500 = 675,000 \] This calculation forecasts a total of $675,000 in claim costs.
Diving deeper into claim cost estimation involves advanced statistical tools and predictive analytics to handle variability and uncertainty. Machine learning models can provide insights by discovering patterns within large data sets. A frequent tool utilized here is the computation of the standard deviation of claim costs which provides insights into variability: \[ \sigma = \sqrt{\frac{1}{N} \sum_{i=1}^{N} (x_i - \mu)^2} \] In this formula, \( \sigma \) represents the standard deviation, \( x_i \) denotes each claim cost, \( \mu \) is the mean claim cost, and \( N \) is the total number of claims. Properly understanding this helps companies manage reserves and strategize effectively.
Claim Cost Estimation Techniques
When managing the financial aspects of a business, understanding claim cost estimation techniques is crucial. These techniques help predict the financial resources required to cover potential claims, particularly in industries such as insurance and construction. A range of methods can be employed to ensure that estimations are as accurate as possible.
Examples of Claim Cost Estimation Techniques
Different strategies are used to estimate claim costs effectively. Some commonly employed techniques include the following:
- Historical Data Analysis: Reviews past claims data to recognize trends and patterns that can predict future costs.
- Actuarial Techniques: Utilizes mathematical and statistical methods to model and forecast claim behavior.
- Simulation Modeling: Uses computational models to predict how different factors might affect future claims.
- Expert Judgment: Relies on the insights of industry experts to provide qualitative assessments of potential claim costs.
Example: Consider an insurance firm wanting to estimate future liabilities growing from home damage claims. Assuming 200 expected claims with an average claim cost of $6,000, the total claim cost estimation would be calculated as follows: \[ E = 200 \times 6000 = 1,200,000 \] This suggests the company should set aside $1,200,000 to meet these anticipated claims.
Taking a more comprehensive look at claim cost estimation can involve more complex variables and analytical methods. For instance, the application of regression analysis aids in understanding the relationship between claim frequency and relevant external factors such as weather patterns or economic cycles. Besides, sampling and survival analysis are advanced actuarial methods deployed to forecast long-term claim development over the lifecycle of assets or policies. An important statistical measure here is the standard deviation, which quantifies the amount of variation in claim amounts: \[ \sigma = \sqrt{\frac{1}{N} \sum_{i=1}^{N} (x_i - \mu)^2} \] where \( \sigma \) is the standard deviation, \( x_i \) is each value in the dataset, \( \mu \) is the mean of the dataset, and \( N \) is the number of occurrences. Understanding this helps businesses manage risks more strategically.
Business Studies Claim Cost Meaning
Claim cost estimation plays a vital role in financial management and planning within various sectors. This process involves predicting the amount of money required to settle or process claims. Effective estimation provides companies with the ability to allocate resources appropriately and mitigate risks associated with insufficient funds.
Estimate of What a Claim Will Cost
The process of estimating what a claim will cost is essential for managing financial risk. Accurate estimations help:
- Strengthen budgeting decisions by anticipating necessary funds.
- Affect the pricing of services or products, such as insurance policies.
- Enhance overall risk management strategies.
Claim Cost Estimation Formula: The fundamental formula used is: \[ E = \text{Frequency of Claims} \times \text{Average Cost per Claim} \] where E denotes the estimated total cost.
Insurance companies utilize complex algorithms and data analytics to refine the accuracy of claim cost estimations.
Example: Imagine a scenario in which a company forecasts 120 claims next year with an average payout of $7,500 per claim. The estimated claim cost calculation would be: \[ E = 120 \times 7500 = 900,000 \] Therefore, the firm should allocate $900,000 for expected claims.
A deeper exploration into claim cost estimation touches on the integration of predictive analytics and big data. These advanced techniques are increasingly being used to predict future claim costs with greater precision. For instance, by analyzing large volumes of historical data, machine learning models can identify patterns and forecast potential future trends. In modeling unpredictability in claims, understanding standard deviation is crucial. It measures the extent of variance in claim amounts from the average. The calculation is as follows: \[ \sigma = \sqrt{\frac{1}{N} \sum_{i=1}^{N} (x_i - \mu)^2} \] Here, \( \sigma \) represents standard deviation, \( x_i \) each claim cost, \( \mu \) the mean claim cost, and \( N \) the number of claims. This insight helps organizations in maintaining financial readiness for a variety of claim scenarios.
claim cost estimation - Key takeaways
- Claim cost estimation definition: The process of predicting financial expenditure needed to settle claims, crucial for financial planning and risk management in sectors like insurance and project management.
- Basic formula: Claim cost estimation is calculated as \( E = \text{Frequency of Claims} \times \text{Average Cost per Claim} \, where \( E \) is the estimated total cost.
- Techniques: Methods include historical data analysis, actuarial techniques, modeling, and expert judgment, each providing different insights and accuracy levels.
- Importance in business: Understanding claim cost estimation helps in budgeting, pricing, risk management, and resource allocation, essential for financial health and stability.
- Examples: Estimation scenarios generally consider expected claim counts and average costs, with companies adjusting financial resources accordingly.
- Factors and variability: Influenced by historical data, inflation, legal changes, and demographics, requiring advanced statistical tools and predictive analytics, such as standard deviation, for accuracy.
Learn faster with the 24 flashcards about claim cost estimation
Sign up for free to gain access to all our flashcards.
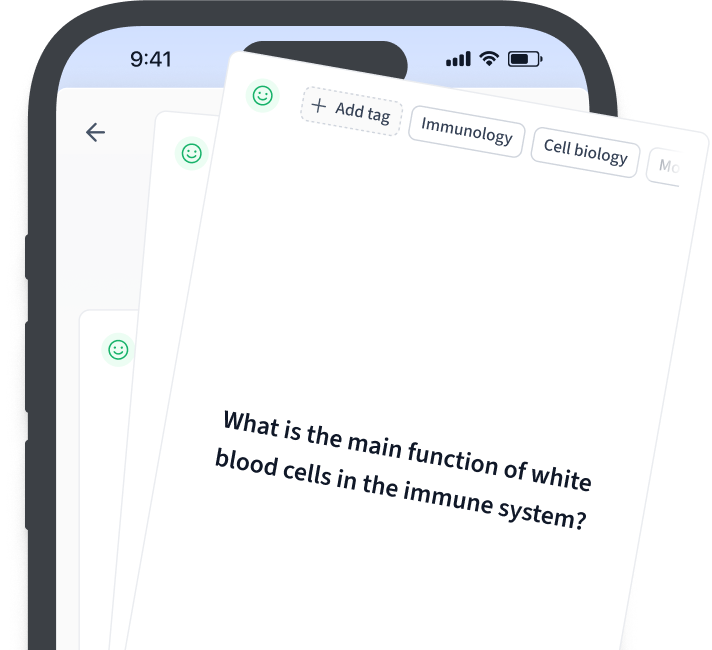
Frequently Asked Questions about claim cost estimation
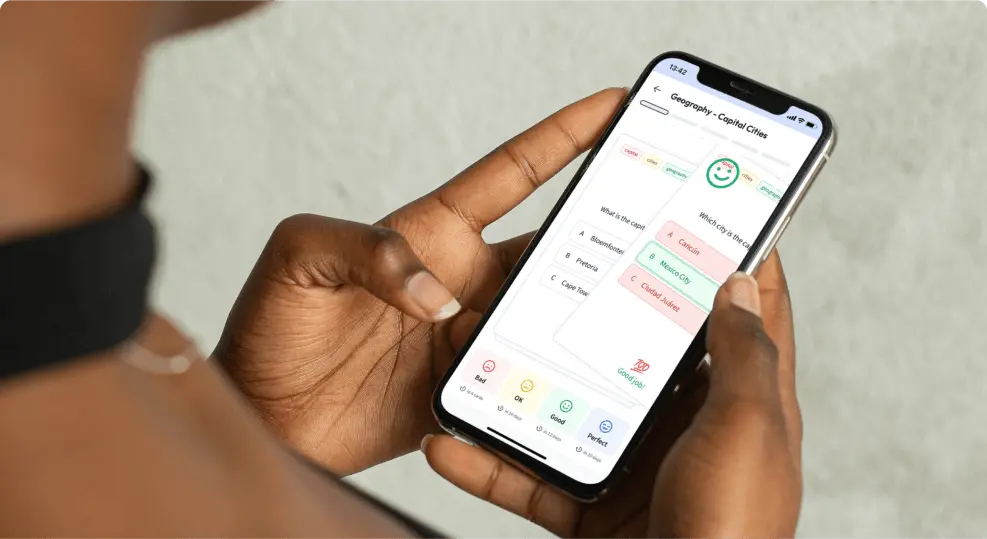
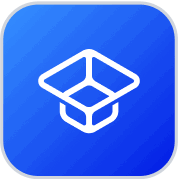
About StudySmarter
StudySmarter is a globally recognized educational technology company, offering a holistic learning platform designed for students of all ages and educational levels. Our platform provides learning support for a wide range of subjects, including STEM, Social Sciences, and Languages and also helps students to successfully master various tests and exams worldwide, such as GCSE, A Level, SAT, ACT, Abitur, and more. We offer an extensive library of learning materials, including interactive flashcards, comprehensive textbook solutions, and detailed explanations. The cutting-edge technology and tools we provide help students create their own learning materials. StudySmarter’s content is not only expert-verified but also regularly updated to ensure accuracy and relevance.
Learn more