Jump to a key chapter
Definition of Copulas
Copulas are a fundamental concept in statistics and probability theory used to study and model the dependence structure between random variables. They allow for the understanding of how variables are interconnected beyond simple correlation. By using copulas, you can model the joint distribution of random variables, illustrating how they vary together in a more nuanced manner.
Key Characteristics of Copulas
Copulas possess several vital characteristics that distinguish them from other statistical tools. Here are a few key features:
- Dependency Structure: Copulas primarily focus on capturing the dependence structure between random variables, irrespective of their marginal distributions.
- Flexibility: They can model a wide range of dependence structures, including both linear and nonlinear relationships.
- Uniform Margins: When constructing copulas, the marginal distributions of the random variables are transformed to uniform distributions over the interval [0, 1].
- Associative Property: Copulas allow for the combination of different marginal distributions into a joint distribution without being restricted to specific types.
A Copula is a multivariate probability distribution for which the marginal probability distribution of each variable is uniform on the interval [0, 1]. This allows for analyzing how random variables can be connected or dependent.
Let's consider two normal random variables, X and Y, where their dependency cannot be captured by linear correlation alone.An example copula can be written as the following expression:\[C(u, v) = \text{exp} \bigg( -((-2 \times \text{ln}(u))^{\theta} + (-2 \times \text{ln}(v))^{\theta} )^{1/\theta} \bigg)\]Here, \(u\) and \(v\) are the uniform transformations of X and Y, respectively, and \(\theta\) is a parameter that specifies the degree of dependency between X and Y.
Copulas are particularly useful in fields such as finance, where capturing the dependency between different entities like stocks is crucial for risk management.
The importance of copulas extends beyond theoretical applications. In practice, they are used widely in the financial industry, especially in portfolio risk management and credit risk modeling. Due to their ability to represent complex dependency structures, copulas help in better understanding and predicting potential outcomes.Consider the following types of copulas frequently used in finance:
- Gaussian Copula: Often utilized for simplicity and ease of calculation, though it assumes a normal distribution which may not always be representative of real data.
- Clayton Copula: Suitable for modeling asymmetric dependencies. It places more weight on the lower tail, making it useful for stress testing and extreme value analysis.
- Gumbel Copula: Ideal for capturing upper tail dependencies, where extreme values in both variables are of particular interest.
Copulas Explained for Students
In the world of probability and statistics, copulas are vital in understanding complex dependencies between variables. These tools help you explore relationships beyond what simple correlation can offer, allowing you to model the joint distribution of variables effectively.
Understanding Copulas in Detail
Copulas play a crucial role in capturing the dependence structure between random variables. They are defined as joint distribution functions with uniform marginals. Here's what you need to know:
- Dependency Structure: Copulas facilitate a deeper understanding of the dependency among variables.
- Transformation: They transform marginal distributions to uniform distributions.
- Flexibility: Enable modeling a broad spectrum of relationship patterns, both linear and nonlinear.
- Mathematical Representation: Copulas can be used in equations such as:\[U = F_X(X), V = F_Y(Y)\]where \(U\) and \(V\) are uniform variables and \(F_X\) and \(F_Y\) are respective distribution functions.
Consider two variables, X and Y, which have a complex dependency structure. A copula helps illustrate this relationship in a more detailed manner. For instance, the Gaussian Copula might be used as: \[C(u, v, \rho) = \Phi_2(\Phi^{-1}(u), \Phi^{-1}(v), \rho)\]Here, \(\Phi_2\) is a bivariate normal cumulative distribution function, and \(\rho\) represents the correlation coefficient.
Remember, copulas are utilized in various fields, including finance and meteorology, due to their versatility in depicting dependencies.
Copulas originate from mathematical and statistical theories but find real-world applications in numerous fields. You may encounter distinct types of copulas, each serving specific needs.For example:
- Clayton Copula: This type shines in scenarios focusing on tail dependencies, common in financial risk assessment.
- Gumbel Copula: Particularly effective in scenarios with upper tail dependencies, useful in predicting extreme weather events.
Examples of Copulas in Business
In the business domain, the application of copulas is instrumental in understanding the dependency between various factors. Through copulas, complex relationships, such as those between financial instruments, can be modeled to aid in risk management and predictive analysis.
Use of Copulas in Financial Markets
In financial markets, copulas are employed to better understand dependencies between different assets. This understanding is crucial for risk management, particularly in the construction and analysis of investment portfolios. Using copulas, risk managers can assess how assets within a portfolio might react to market shifts in correlation with each other.Several types of copulas are popularly used in finance:
- Gaussian Copula: Often used for its simplicity, it assumes normal distribution dependencies, widely applied in portfolio diversification.
- Clayton Copula: Useful for modeling dependencies where a change in one variable can have significant implications on another, particularly in joint tail risks.
- Gumbel Copula: Best for capturing upper tail dependencies, making it suitable for determining potential simultaneous failures in banking sectors.
Imagine analyzing the joint risk of multiple banking institutions. With a Gumbel Copula, the focus is on the simultaneous extreme losses, captured by the formula:\[C(u, v) = \text{exp}\big\big[(-\text{ln}(u)^\theta - \text{ln}(v)^\theta)^{1/\theta}\big\big]\]This equation assists in understanding how likely it is for extreme losses to occur concurrently, allowing for better risk preparations.
Copulas enable more accurate risk modeling and decision-making in business by allowing you to explore dependencies beyond basic correlations.
In insurance, copulas are pivotal in aggregating and estimating potential losses from multiple risk exposures.Consider an insurer covering various types of risks, such as property and casualty, wherein losses are not independent but rather correlated with each other. Using copulas, one could construct a joint probability distribution of these correlated losses effectively. This is particularly important when calculating capital reserves using regulatory frameworks such as Solvency II, which require detailed modeling of dependencies.By using copulas, insurers can perform scenario analysis, stress testing, and risk management more effectively. An accurate understanding of complex risk dependencies informs better pricing, underwriting, and reserve allocation decisions, thereby positioning businesses for resilience in challenging financial environments.
Copula Techniques in Mathematical Models in Business
Understanding copula techniques is essential in business, especially for analyzing dependencies between economic variables. Copulas provide a way to model complex interactions, helping you predict outcomes more accurately.
Basic Copula Techniques
Basic copula techniques involve the application of simple copulas to model variable dependencies. These techniques are accessible and useful for straightforward analysis in business scenarios.Common basic copulas include:
- Gaussian Copula: Useful for modeling normal relationships with symmetric dependencies.
- Clayton Copula: Suited for highlighting the lower tail dependencies.
- Gumbel Copula: Effective in capturing dependencies at the upper tail.
Consider a scenario where you are assessing the dependency between sales of two products. Using a Gaussian Copula, you determine how changes in one product's sales might influence the other's, helping in making informed stock decisions.
Basic copula techniques are ideal for preliminary analysis, providing insights into dependencies without complex model requirements.
Advanced Copula Techniques
For more intricate dependency structures, advanced copula techniques come into play. These involve the use of complex copula models to analyze variable relationships. Such techniques are vital in environments with non-linear interactions.Consider Archimedean Copulas, which offer flexibility and can model a range of dependencies efficiently. The mathematical representation for a popular Archimedean Copula, the Frank Copula, is given by:\[C(u, v, \theta) = -\frac{1}{\theta}\ln\left(1 + \frac{(e^{-\theta u} - 1)(e^{-\theta v} - 1)}{e^{-\theta} - 1}\right)\]Here, \(\theta\) is a parameter that controls the dependency level.
Advanced copula strategies often involve constructing hierarchical structures known as vine copulas. These allow for modeling dependencies among large sets of variables by organizing them into nested trees, providing a multi-dimensional view of correlation.Vine copulas are particularly effective in high-dimensional problems, as they can be adapted to capture different dependencies at various levels, offering a comprehensive analysis compared to traditional methods.
Role of Copulas in Risk Management
Copulas are crucial in risk management, enabling you to assess the likelihood of simultaneous financial risks occurring. They are especially valuable in portfolio management and financial derivatives.Using copulas, risk managers can:
- Estimate Joint Risks: By modeling dependencies, copulas help quantify the probability of multiple asset drops.
- Optimize Asset Diversification: Understanding dependencies can aid in constructing diversified portfolios with minimized risk.
- Develop Stress Testing Scenarios: Copulas support the simulation of extreme market conditions, essential for stress testing.
A well-designed copula model can significantly enhance the accuracy of risk calculations and provide a robust framework for strategic decision-making.
In credit risk modeling, copulas are employed to estimate the default probability of a group of loans or credit assets. By representing the dependencies between these assets, copulas facilitate a better understanding of how systemic factors may lead to simultaneous defaults.Consider a CDO (Collateralized Debt Obligation) where copulas are used to model the correlation among the underlying assets. The insights garnered from such models enable effective pricing, management of CDOs, and compliance with regulatory requirements. In such cases, copulas contribute not only to risk assessment but also to deciding appropriate capital reserves, thus maintaining financial stability.
copulas - Key takeaways
- Definition of Copulas: Multivariate probability distributions that model the dependence structure between random variables, with each variable having a uniform marginal distribution on the interval [0, 1].
- Characteristics: Copulas capture dependency structure beyond correlation, use uniform marginals, and allow combination of different distributions into a joint distribution.
- Examples of Copulas: Gaussian Copula models normal relationships; Clayton Copula highlights lower tail dependencies; Gumbel Copula captures upper tail dependencies in variables.
- Copulas in Finance: Used for risk management, assessing asset dependencies, and portfolio analysis. Helps in scenarios like stress testing and extreme value analysis.
- Copula Techniques: Basic and advanced methods used to model dependencies in economic variables. Includes Gaussian Copula for simple analysis and Archimedean Copulas for complex dependencies.
- Application in Business: Copulas model dependencies in financial instruments, aiding risk management, predictive analysis, and strategic planning in fields such as finance and insurance.
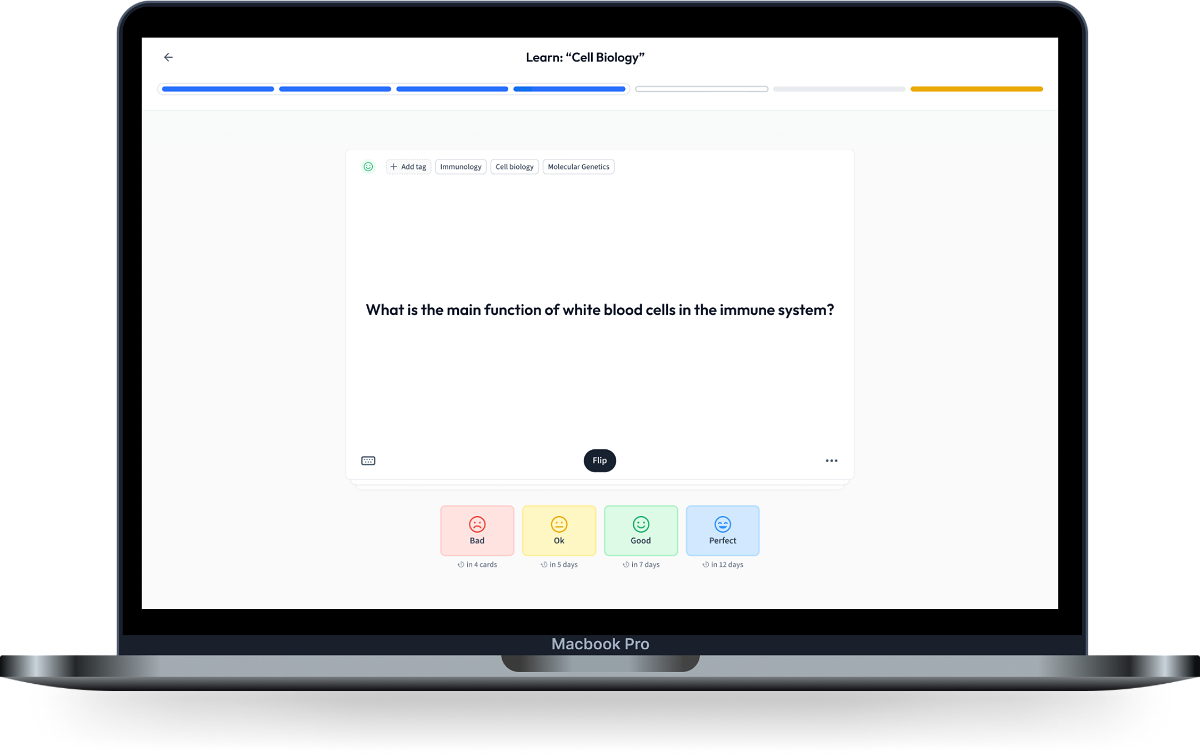
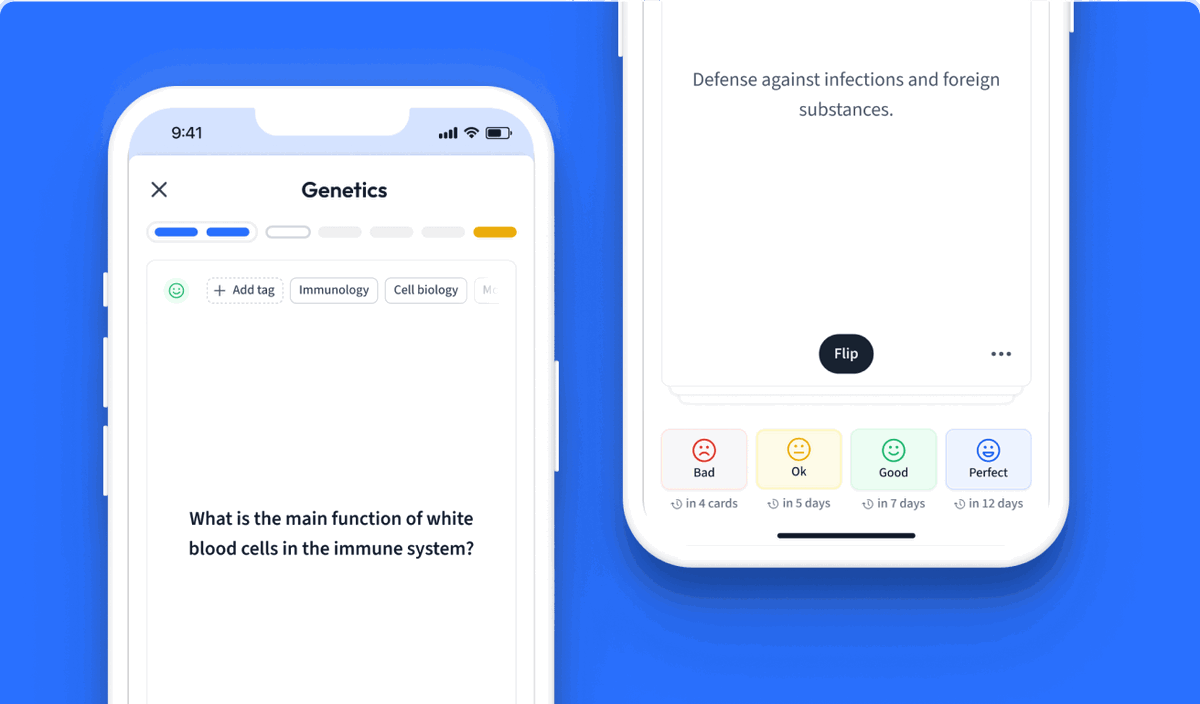
Learn with 24 copulas flashcards in the free StudySmarter app
We have 14,000 flashcards about Dynamic Landscapes.
Already have an account? Log in
Frequently Asked Questions about copulas
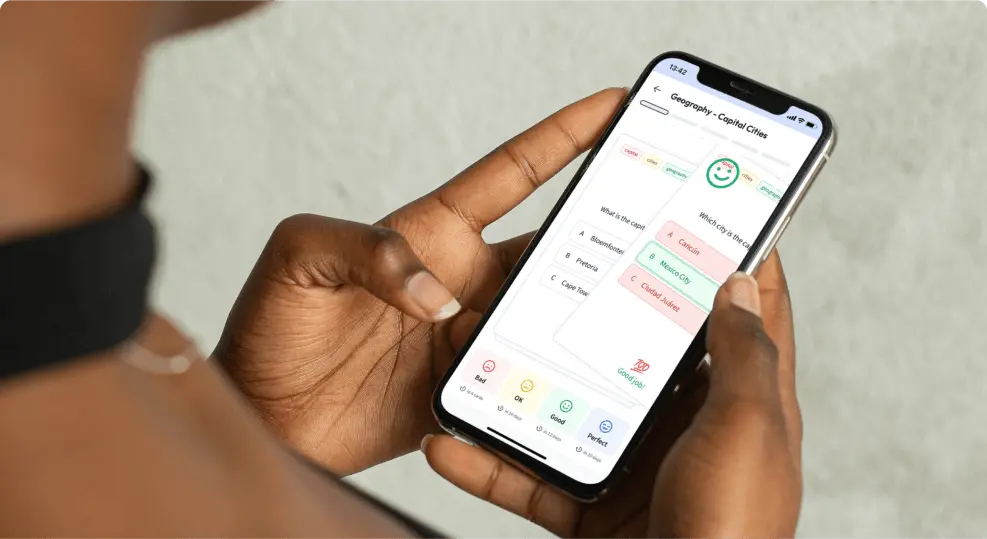
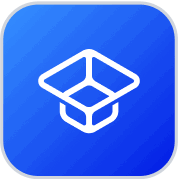
About StudySmarter
StudySmarter is a globally recognized educational technology company, offering a holistic learning platform designed for students of all ages and educational levels. Our platform provides learning support for a wide range of subjects, including STEM, Social Sciences, and Languages and also helps students to successfully master various tests and exams worldwide, such as GCSE, A Level, SAT, ACT, Abitur, and more. We offer an extensive library of learning materials, including interactive flashcards, comprehensive textbook solutions, and detailed explanations. The cutting-edge technology and tools we provide help students create their own learning materials. StudySmarter’s content is not only expert-verified but also regularly updated to ensure accuracy and relevance.
Learn more