Jump to a key chapter
Credibility Theory Definition
Credibility theory is a statistical approach used primarily in actuarial science to make predictions about future claims or events based on past observations. It helps you estimate parameters by balancing past data (experience) with external sources such as global data or expert opinions.
Understanding Credibility in Business Studies
In business studies, understanding credibility often revolves around trust and reputation, both of which are critical assets for any entity. However, when viewed through the lens of credibility theory, the focus shifts to quantifiable metrics that can predict future outcomes based on historical data.
You can think of credibility as a way to reconcile two pieces of information: your own data (which may have limitations due to its size or relevance) and external benchmarks. The primary goal is to find the most robust estimate to base decisions.
The credibility factor (Z) is at the heart of credibility theory. It ranges from 0 to 1, indicating the weight assigned to one's data in contrast to the weight given to an external data source. This factor is crucial in determining how much reliance you should place on your data for prediction purposes.
Suppose a new retailer is trying to forecast the demand for a product using sales data from just six months. They have access to industry data that spans several years. The credibility factor might lean towards the industry data (Z < 0.5) to ensure the forecast integrates broader trends over unproven, shorter datasets.
Remember, the credibility factor can be adjusted as more internal data becomes available, increasing accuracy in predictions over time.
To better illustrate, consider a case where you have observed historical data regarding product sales. The calculation of the credibility factor typically depends on the variance and volume of past data. A common formula used is: \[Z = \frac{n}{n+k}\]where \(n\) is the number of observations (i.e., the volume of your historical data) and \(k\) is a value that reflects the variance. As \(n\) increases, \(Z\) approaches 1, indicating higher reliability of your estimate.
The Role of Credibility Theory in Actuarial Science
Actuarial science heavily relies on credibility theory, particularly in the field of insurance and risk management. The primary aim is to predict the likelihood of future events, making it essential to balance company-specific data with extensive industry-wide studies.
Insurance companies use credibility theory to calculate premiums. They must decide how much variance to attribute to individual claims data versus the collective industry risk. This helps in setting fair premiums while also maintaining company solvency.
The Bayesian approach plays a significant role in actuarial credibility. It involves updating the probability estimates for a hypothesis as new evidence or information becomes available, making predictions more adaptive and informed.
Consider a small health insurance provider estimating the premium for a new plan. Initially, they may heavily weigh national health statistics to set the base premium. Over time, with more claims data from this new plan, they can adjust the credibility factor to better reflect actual experience, leading to more accurate premium calculations.
In actuarial applications, credibility theory often intersects with other statistical principles. For instance, blending Bayesian approaches with credibility theory leads to a more dynamic system of analysis. An actuarial professional might start with an initial belief or prior distribution and revise it using Bayes' theorem as more data becomes available. Mathematically, this is represented as:\[P(H|E) = \frac{P(E|H) \, P(H)}{P(E)}\]where \(P(H|E)\) is the posterior probability, \(P(E|H)\) is the likelihood, \(P(H)\) is the prior probability, and \(P(E)\) is the marginal likelihood. This formula forms the basis of updating beliefs within credibility calculations and is pivotal for accurate predictions.
Credibility Theory Examples
The use of credibility theory aids in making informed predictions by integrating internal data with wider insights. This empowers you to achieve more accurate results in risk assessment, premium determination, and beyond. Let's delve into some specific applications in the following sections.
Real-World Applications of Credibility Theory
In practice, credibility theory is an invaluable tool across various sectors. It helps organizations in predicting future trends and outcomes by synthesizing both historical data and industry benchmarks.For instance, in the insurance industry, firms frequently use credibility methods to accurately set premiums based on a combination of their own loss experience and broader market data.Financial institutions leverage credibility estimates to assess credit risk. By blending customer-specific data with large-scale economic indicators, they refine their credit scoring systems.Healthcare analytics apply these principles to predict patient care costs. By combining data from internal records and larger health datasets, they can accurately budget and set pricing structures.Certain steps are followed when implementing credibility in these applications:
- Identify the volume and variance of internal data, which determines its weight in predictions.
- Calculate the credibility factor (Z) to gauge the influence of internal vs external data sources.
- Combine both internal data and external data efficiently to attain the most credible estimate.
Consider a car insurance company that uses credibility theory to determine premiums. The company analyzes claims for individual policyholders and compares them to industry standards. If a driver has fewer accidents than average, their claims data is weighted more heavily, leading to a potential discount on premiums.
The effectiveness of credibility theory is magnified with increased internal data, offering stronger forecasts over time.
Let’s take a deeper look at the mathematical formulation in a practical scenario for health insurance. Assume you have data for patient claims over the last few years, and large data from the national health department regarding similar claims nationwide. You want to predict the claims for the upcoming year.The credibility factor \(Z\) determines how much to rely on each data source. This is calculated by:\[Z = \frac{n}{n+k}\]Here, \(n\) is the number of patient's historical observations you own, while \(k\) often represents the measure of variance in claims. As \(n\) increases, \(Z\) grows, reflecting greater faith in your own data. If \(k\) is large, implying high variability, \(Z\)'s growth is moderated.Now combine this result:\[E(\text{Future Claims}) = Z \times \text{Internal Data} + (1-Z) \times \text{External Data}\]This equation indicates how intrinsic and extrinsic data proportionally contribute to the credibility-weighted forecast.
Credibility Techniques in Different Industries
Different industries adopt varied credibility techniques depending on their specific needs and data characteristics. Understanding these techniques deepens your grasp on how various sectors maximize predictive accuracy.In actuarial science, credibility techniques are foundational in calculating reserves for future payouts. Companies analyze historical claims data alongside mortality rates to better set aside funds, ensuring sustainability.Marketing and consumer analytics harness credibility techniques for customer segmentation and personalization strategies. Data from customer interactions are paired with market research to achieve refined audience targeting.In operations management, credibility estimates are employed for inventory forecasting. By combining sales history with market dynamics, firms optimize stock levels and minimize waste.A general approach to employing credibility in these contexts involves:
- Gathering extensive historical data to establish a baseline.
- Using statistical models to calculate the credibility factor (Z).
- Integrating internal insights with larger market datasets for balanced outcomes.
A manufacturing company predicts future product demands using credibility theory. They merge sales data with industry trends to determine the best stock levels. By adjusting and recalculating the credibility factor, they optimize their production runs efficiently.
Taking a closer look at marketing applications, firms blend customer purchase behavior with larger demographic data through credibility methods. Suppose you're a retailer analyzing customer purchase frequency. By calculating a tailored credibility factor, predictions about future interest can focus more on personalized shopping histories or broader market averages where appropriate. The key lies in the proportionate weighting: \[S = Z \times C + (1-Z) \times M\]where \(S\) is the future sales estimate, \(C\) represents customer-specific buying patterns, and \(M\) is the market general data. This blend aids in highly customized marketing strategies, potentially enhancing CRM systems and promoting retention.
Credibility Theory Techniques
Credibility theory is pivotal in statistical methods, particularly in actuarial contexts, where it helps integrate various data sources to forecast future occurrences. Knowing these techniques is essential for making accurate predictions.
Common Methods in Credibility Theory
In credibility theory, several methods are utilized to balance the weight given to different datasets. These approaches help you assign appropriate confidence levels to data and incorporate external information.The common methods include:
- Buhlmann Model: This model provides a structured way to determine the credibility factor by balancing past experiences with broader datasets pertaining to specific metrics.
- Empirical Bayes Method: By using preliminary internal data, this method gradually refines predictions as additional data becomes available, adapting to new patterns.
- Bayesian Approach: A more advanced method that updates predictions by combining prior distributions with new evidence. It is adaptive and robust, suitable for diverse and dynamic datasets.
Method | Feature |
Buhlmann Model | Balances individual vs collective experience |
Empirical Bayes | Refines with new data gradually |
Bayesian Approach | Adaptively updates predictions |
Consider a car insurer using the Buhlmann Model to assess premiums. The company analyzes historical claims data and adjusts it by adding weights from broader industry trends to find a reliability factor that suits individual policyholders.
For the Buhlmann Model, the credibility factor \(Z\) is calculated using the formula:\[Z = \frac{n}{n + k}\]where \(n\) is the volume of individual data, and \(k\) is a constant representing between-group variability. Including both parts ensures you calculate an optimal value of reliability, allowing the prediction to remain stable amidst fluctuations.
Accuracy improves by adjusting the credibility factor as more data is accumulated, enhancing precision in results over time.
Advanced Credibility Techniques for Actuarial Purposes
In actuarial science, advanced credibility techniques allow for intricate risk assessments and financial predictions by blending complex data sources.Highly developed methods include:
- Conjugate Priors: These are used within Bayesian approaches to align prior distributions, offering mathematically convenient updates as new data arises. This simplifies calculations significantly.
- Mixed Linear Models: These are utilized to work with hierarchical datasets, allowing you to model complex nonlinear relationships present in actuarial data.
- Generalized Linear Models (GLMs): These models bring flexibility in modeling different types of data distributions, enabling robust predictions even in varied contexts.
In the Generalized Linear Model (GLM), a link function relates the expected value of the data distribution to the linear predictor, enhancing predictive capacities. This involves multiple independent variables influencing dependent outcomes, broadening application scopes.
A health insurer employs GLMs to estimate policyholder risk. By using extensive historical health data, they predict future claims more precisely, tuning policy conditions accordingly.
In actuarial contexts, employing GLMs allows companies to handle varied insurance products systematically. The model's structure leverages both categorical and continuous data:\[g(\mu) = X\beta\]Here, \(g\) is the link function, \(\mu\) is the expected value of the response, \(X\) the matrix of predictors, and \(\beta\) represents the coefficients. Properly coordinating these components, insurers streamline product pricing and strategizing under dynamic market conditions. Moreover, extending this framework with conjugate priors within Bayesian methods reduces computational load, granting efficiency in handling extensive datasets.
Importance of Credibility in Decision Making
In decision making, credibility plays a crucial role by providing a framework for evaluating how much you should trust specific data sources over others. This ensures informed and reliable business decisions, minimizing risks and uncertainties. Credibility theory offers the statistical methodologies to balance current and past data, leading to more accurate and consistent decision-making outcomes. Understanding this enhances your company's adaptability to ever-changing environments.
Impact of Credibility Theory on Business Decisions
The influence of credibility theory on business decisions is profound and varied. By using this theory, organizations are better equipped to assess the reliability of their data, integrate external sources, and make decisions that stand the test of time.Here's how credibility theory impacts business choices:
- Risk Management: Helps in evaluating risks by combining company-specific data with industry benchmarks. This balanced approach leads to informed risk-taking and better financial health.
- Forecasting: In business forecasting, credibility theory aids in more accurate predictions by giving due weight to different datasets based on their reliability.
- Strategic Planning: By integrating data from various sources, it becomes easier to plan long-term strategies which are robust against data variability and market shifts.
An example is seen in retail, where a company employing credibility theory can better forecast demand for products by merging internal sales data with external market trends. This allows them to allocate their stock more precisely, avoiding overproduction and stockouts.
Accurate decisions emerge when internal data's weight increases with credibility, reflecting improved data quality over time.
Consider a company evaluating whether to launch a new product line. They can utilize credibility theory to assess demand, considering both internal sales figures and external market analytics. By calculating the credibility factor using:\[Z = \frac{n}{n+k}\]where \(n\) represents the total internal data entries and \(k\) is a constant depicting variance, they calibrate how heavily reliance should be placed on internal assessments versus broader market data. The weighted formula\[C = Z \times D_i + (1-Z) \times D_e\]where \(C\) is the combined prediction, \(D_i\) is internal sales data, and \(D_e\) is external data, crafts a robust vintage of credibility in decision-making pathways.
Building Effective Strategies with Credibility Theory
Incorporating credibility theory into strategic planning can significantly bolster your company's overall performance. By blending diverse data sources, businesses craft strategies that are not only innovative but also grounded in reliable evidence. Here are the ways credibility enhances strategy development:
- Data Fusion: Merges traditional company data with new datasets, enriching the context and perspective needed for effective strategy.
- Predictive Analysis: Leverages combined data to predict future trends more accurately, leading to preemptive strategic adjustments.
- Scenario Planning: Strengthens planning for various market conditions by adjusting the credibility factors to reflect changes in data availabilities and relevance.
For example, a tech start-up can use credibility theory to strategically plan product rollouts by assessing current market gaps (external data) in conjunction with their own R&D insights (internal data). This results in a competitive edge.
In strategy planning, models integrating credibility theory offer a robust mathematical approach. The general model used to incorporate credibility in strategic decisions is:\[S = Z \times I + (1-Z) \times E\]Here, \(S\) is the strategic output, \(I\) represents internal insights, and \(E\) involves external evaluations. By calibrating the credibility factor, \(Z\), strategies are aligned closer with actionable data. This mathematical formulation validates transitioning innovations to overcome uncertainty barriers. Thus, credibility theory methodically balances sources, instilling confidence in decision outcomes and speeding up market adaptation.
credibility theory - Key takeaways
- Credibility Theory Definition: A statistical approach used primarily in actuarial science for predicting future claims or events by balancing past data with external sources.
- Credibility Factor (Z): Central to credibility theory, it indicates the weight given to one's data versus external data, ranging from 0 to 1.
- Importance in Actuarial Science: Used to predict future events and set insurance premiums by balancing individual claims data with industry-wide information.
- Bayesian Approach in Actuarial Credibility: Updates probability estimates with new data to adapt predictions, enhancing accuracy in actuarial practices.
- Credibility Techniques: Include Buhlmann Model, Empirical Bayes Method, and Bayesian Approach to balance data weights for predictive accuracy.
- Importance of Credibility in Decision Making: Offers a framework to evaluate data trustworthiness, integrating past data and external information for informed business decisions.
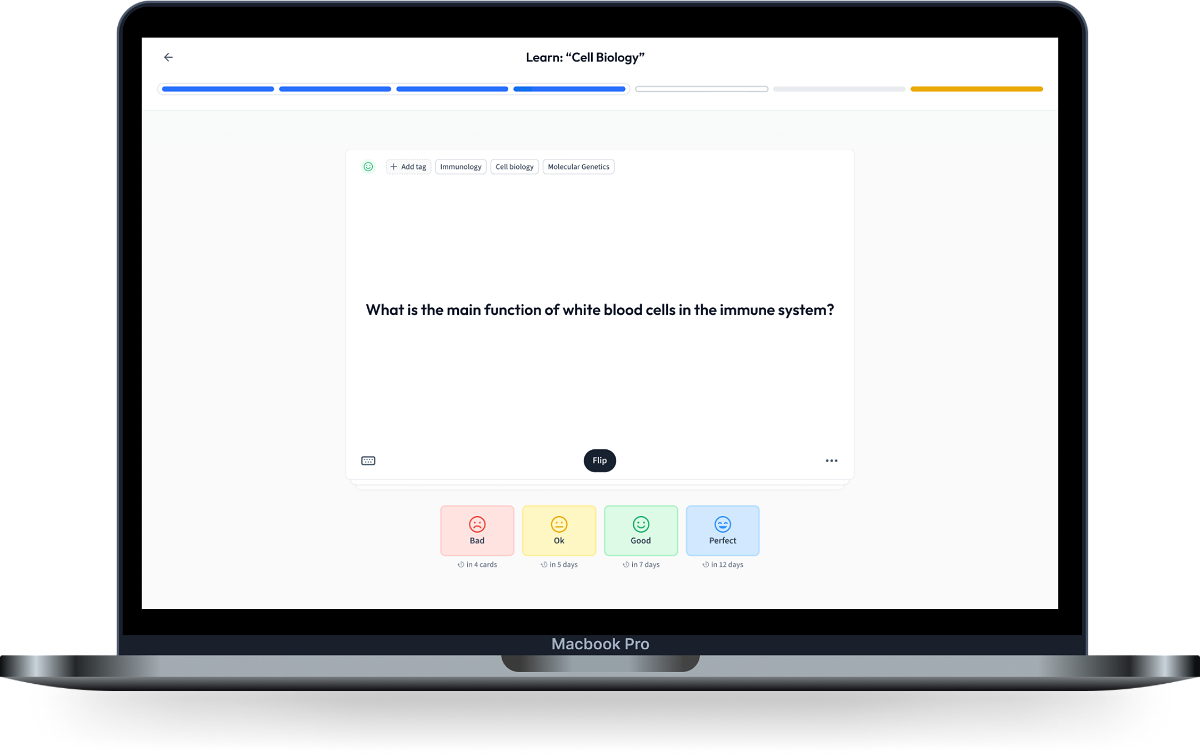
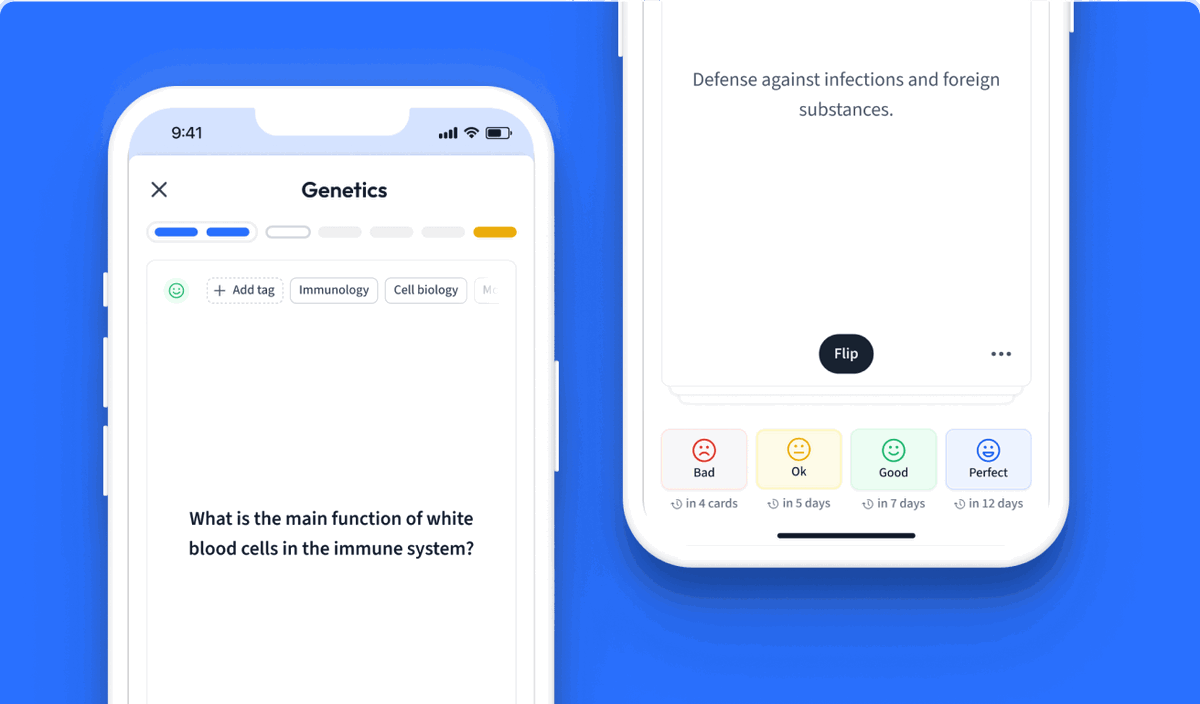
Learn with 24 credibility theory flashcards in the free StudySmarter app
We have 14,000 flashcards about Dynamic Landscapes.
Already have an account? Log in
Frequently Asked Questions about credibility theory
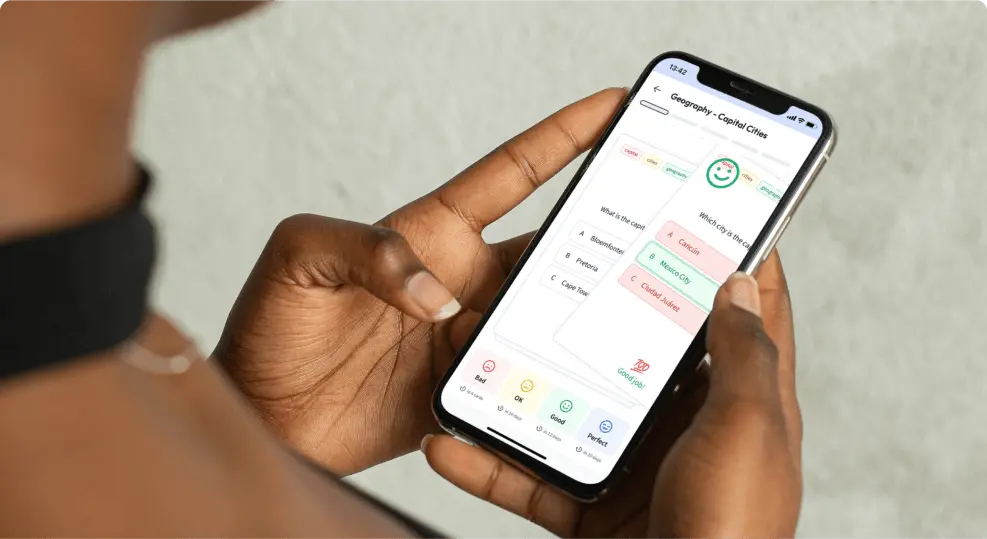
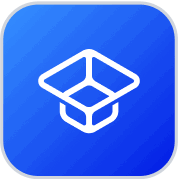
About StudySmarter
StudySmarter is a globally recognized educational technology company, offering a holistic learning platform designed for students of all ages and educational levels. Our platform provides learning support for a wide range of subjects, including STEM, Social Sciences, and Languages and also helps students to successfully master various tests and exams worldwide, such as GCSE, A Level, SAT, ACT, Abitur, and more. We offer an extensive library of learning materials, including interactive flashcards, comprehensive textbook solutions, and detailed explanations. The cutting-edge technology and tools we provide help students create their own learning materials. StudySmarter’s content is not only expert-verified but also regularly updated to ensure accuracy and relevance.
Learn more