Jump to a key chapter
Dependent Risk Models Definition Business Studies
In business studies, understanding the intricacies of dependent risk models is crucial. These models are essential for analyzing interrelated risks within business processes, allowing for more informed decision-making. By using dependent risk models, you can evaluate complex risk interdependencies and assess their potential impact on your business.
Understanding Dependent Risk Models in Business
Dependent risk models represent the interconnected nature of risks. These models help in analyzing how multiple risks in a business environment can influence each other. By understanding these interdependencies, you can forecast potential risks more accurately and devise strategies to mitigate them effectively.Key components of dependent risk models include:
- Risk Factors: Elements within your business that may lead to risk exposure.
- Correlation: The degree to which risks are interrelated.
- Probability Distribution: The likelihood of various risk outcomes occurring.
Dependent Risk Models: These models assess interrelated risks that can affect business operations simultaneously. Such models help in predicting risk behavior and making informed decisions.
Consider a manufacturing company facing two risks: supply chain disruption and raw material price increase. If a natural disaster affects suppliers, not only would there be delays, but there could also be a price hike due to scarcity. Here, dependent risk models aide in understanding this interrelation and guide management in contingency planning, such as holding more inventory or seeking alternative suppliers.
Understanding risk interdependencies helps in building robust strategies that are more resilient to external shocks.
Role of Dependent Risk Models in Business Analysis
Dependent risk models play a critical role in business analysis by providing insights into how risks are connected and can trigger systemic failures. These models are pivotal in various business aspects, such as strategic planning, operational management, and financial forecasting.Key applications in business analysis:
- Risk Assessment: Evaluate overall risk exposure and prioritize risk management efforts.
- Decision Making: Make strategic decisions based on comprehensive risk analyses.
- Financial Planning: Anticipate potential losses and budget for risk-related expenses.
Consider the financial crisis of 2008, which highlighted the impact of interconnected financial risks. Dependent risk models allow businesses to view risks not in isolated silos but as part of a network, where a problem in one area can cascade through multiple business functions or sectors. For insurance companies, modeling both natural and man-made risks in tandem can reveal vulnerabilities that might not be apparent when considering each risk individually. By leveraging complex calculations and simulations, such as Monte Carlo simulations, you can predict a wide array of outcomes and their probabilities, better enhancing your strategic risk management.
Actuarial Theory for Dependent Risks Measures Orders and Models
Actuarial theory is a cornerstone for understanding how dependent risks interact within complex systems. By leveraging mathematical and statistical methods, actuarial theory enables you to develop models that predict risk outcomes based on past occurrences and underlying patterns. This theoretical foundation is key to creating robust strategies for managing risk in various sectors.
Basic Concepts of Actuarial Theory
To effectively utilize actuarial theory, it is important to grasp its foundational concepts. These are critical to understanding how risks are quantified and managed.The basic principles include:
- Probability and Statistics: These are essential for calculating risk exposure and outcomes.
- Financial Mathematics: Used to assess the present value of future cash flows, particularly in insurance and pension calculations.
- Risk Models: Mathematical representations that simulate potential risk scenarios.
Actuarial Theory: A discipline that applies mathematical and statistical methods to assess risk in insurance, finance, and related fields.
Financial mathematics in actuarial theory often involves the time value of money, a crucial concept for valuing future financial obligations.
Key Measures and Orders in Dependent Risk Models
Dependent risk models require specific measures and orders to effectively quantify and manage risk correlations. Here, measures and orders include both statistical methods and theoretical constructs that highlight risk interdependencies.Key measures include:
- Copulas: Functions that describe the dependence structure between random variables, separating the marginal distributions from their joint distribution.
- Correlation Coefficients: Values such as Pearson's or Spearman's that indicate the degree of relationship between two variables.
- Tail Dependence: A measure of the degree of dependence in the tails of the distribution, useful for understanding extreme outcomes.
In finance, you may use the Spearman’s rank correlation to assess the relationship between two stock returns. Unlike Pearson's, Spearman’s correlation works with ranks of values, providing a measure of how well the relationship between variables can be described by a monotonic function. It is computed as:\[\text{r}_s = 1 - \frac{6 \sum d_i^2}{n(n^2 - 1)}\]where \(d_i\) represents the difference between ranks for each observation, and \(n\) is the number of observations.
The concept of tail dependence is particularly significant in financial risk management, especially when assessing the risk of extreme events in portfolios, such as market crashes or catastrophic losses. Tail dependence provides a more comprehensive view by illustrating how likely extreme losses in one risk factor are related to extreme losses in another. This understanding aids in stress testing and scenario analysis, pivotal for entities like insurance companies and banks. For example, in bivariate distributions, the tail dependence coefficient is calculated as:\[\lambda_L = \lim_{u \to 0^+} P(X < F_X^{-1}(u) | Y < F_Y^{-1}(u))\]This analysis is crucial for preparing strategies to minimize impacts during financial downturns.
Techniques in Dependent Risk Assessment
Understanding and assessing dependent risks is vital for making informed business decisions. By employing a variety of techniques, you can better manage and mitigate risks that are interrelated. Below, the focus is on both qualitative and quantitative methods, along with essential tools used to ensure accurate risk assessment.
Qualitative and Quantitative Techniques
In the realm of dependent risk assessment, techniques are broadly divided into qualitative and quantitative methods. Each has its significance and application:Qualitative Techniques:
- Scenario Analysis: Used to explore and evaluate different possible future outcomes based on hypothetical situations.
- Expert Judgment: Involves leveraging the knowledge of experienced individuals to assess risks.
- SWOT Analysis: Assesses risks by identifying strengths, weaknesses, opportunities, and threats.
- Statistical Models: Use data and statistical methods to predict risk dynamics.
- Monte Carlo Simulation: Employs random sampling to project risk probabilities across large datasets, enhancing the robustness of risk estimates.
- Regression Analysis: Evaluates the relationship between dependent and independent risk factors.
Monte Carlo Simulation: A computational algorithm that uses repeated random sampling to calculate the probabilistic outcomes of uncertain processes.
Consider a project manager assessing the risk of project delays due to dependent factors such as resource availability and weather conditions. By applying Monte Carlo Simulation, the manager can simulate various scenarios and establish a probability distribution of project completion times.
Incorporating both qualitative and quantitative assessments can lead to more robust risk management strategies.
Tools for Accurate Dependent Risk Assessment
To ensure accuracy in dependent risk assessment, various tools and software are employed. These tools enhance the ability to model, simulate, and visualize risk interactions effectively.Key Tools Include:
- Risk Management Software: Applications like Palisade's @RISK and Oracle Crystal Ball which perform simulations and scenario analyses.
- Data Analytics Platforms: Tools such as Tableau and Power BI are used to visualize and analyze complex datasets.
- Programming Languages: Python and R are widely used for developing custom risk models given their extensive libraries for statistical analysis.
A deeper understanding of dependent risk assessments comes from exploring advanced statistical tools like Bayesian Networks. These networks use probabilities to model the dependencies among variables, enabling you to infer risk interconnections effectively. Bayesian Networks can be particularly useful in scenarios with scant data, as they incorporate prior knowledge into the prediction model. For instance, the Bayes' theorem in a Bayesian risk assessment model calculates updated probabilities as evidence increases:\[P(H|E) = \frac{P(E|H) \cdot P(H)}{P(E)}\]Here, \(P(H|E)\) is the probability of hypothesis \(H\) given evidence \(E\), \(P(E|H)\) is the likelihood of \(E\) assuming \(H\) is true, \(P(H)\) is the prior probability of \(H\), and \(P(E)\) is the probability of the evidence. Utilizing Bayesian networks allows businesses to refine risk predictions, even progressively, as more data becomes available.
Modelling Dependence with Copulas and Applications to Risk Management
In risk management, understanding the dependencies between various risk factors is vital. A powerful tool for modeling such dependencies is the Copula, which provides a way to describe the relationship between variables and allows you to view these dependencies without assuming the same marginal distribution.
Basics of Modelling Dependence with Copulas
Copulas are statistical tools that you use to capture and model the dependence structure between random variables. They enable the separation of marginal distributions from the joint distribution, which is crucial for understanding how different risks might coalesce.Essential characteristics of copulas include:
- Sklar's Theorem: States any multivariate joint distribution can be expressed using copulas.
- Parameterization: Copulas can be parameterized to fit different types of dependency structures.
- Types of Copulas: Gaussian, Clayton, and Gumbel copulas are popular versions, each modeling different types of tail dependence.
Copula: A function that joins one-dimensional marginal distribution functions to form a multivariate distribution, capturing the dependency between random variables.
Copulas are advantageous because they allow for the modeling of variable dependencies without needing the variables to have identical marginals.
The use of copulas extends beyond theoretical constructs into practical applications in finance and insurance, where understanding tail dependencies is critical. Tail dependence is particularly significant when rare events occur simultaneously. For instance, in a financial crisis, multiple asset classes may experience joint tail events, which only copulas can appropriately capture. The Clayton and Gumbel copulas are specifically useful for modeling such asymmetric dependencies, focusing on lower and upper tail dependencies respectively. This capability allows for more precise risk forecasting and capital allocation strategies.
Applying Copulas in Risk Management
Incorporating copulas into risk management frameworks enhances your ability to assess and mitigate the impact of risk dependencies across portfolios or business processes. Here are key application areas:
- Portfolio Risk Management: Copulas help in constructing better-diversified portfolios by modeling asset return dependencies, reducing the risk of large losses.
- Insurance: Used for aggregation of insurance risks, copulas assist in pricing and managing correlated risks.
- Credit Risk: By modeling defaults as correlated events, copulas aid in assessing the joint probability of default in a credit portfolio.
Consider a bank with a portfolio of loans. By applying a copula-based model, the bank determines the likelihood that a certain percentage of loans will default simultaneously due to economic downturns. This enables the institution to set aside the necessary capital reserves to cushion against possible financial shocks, ensuring stability.
Dependency Analysis Models Risk
Understanding how risks are related in a business context is key to managing them effectively. Dependency analysis models play a critical role in this process by identifying and quantifying the relationships between risks. Utilizing such models enables you to assess the potential impact of risk interactions, thereby enhancing risk management strategies.
Various Models for Dependency Analysis
In risk management, dependency analysis models help in examining the relationships between different risk factors. These models can be broadly categorized based on their methodological approach and application.Common models include:
- Linear Regression Models: Used to explore the linear relationship between dependent and independent variables.
- Copula Models: These models are particularly useful for capturing nonlinear dependencies between variables.
- Bayesian Networks: A graphical model representing probabilistic relationships among variables.
Imagine an insurer using copula models to evaluate the potential dependency between flood and earthquake insurance claims. This enables the insurer to design policies considering the likelihood of simultaneous claims.
Bayesian Networks are particularly insightful for complex systems with interdependencies, helping you to visualize and compute the effects of changes across the network. They facilitate scenario analysis and allow for updating risk probabilities as new information emerges. For instance, Bayes' theorem is fundamental in these networks:\[P(A|B) = \frac{P(B|A) \times P(A)}{P(B)}\] The formula shows how to update the probability of an event \(A\) given new information \(B\). This is critical in dynamically changing environments.
Choosing the right model relies on understanding the nature of your data and the specific dependencies you wish to explore.
Impact of Dependency Analysis on Business Risk
Applying dependency analysis impacts business risk management significantly. By recognizing how risks correlate, businesses can undertake informed decision-making.The impact of dependency analysis includes:
- Improved Risk Assessment: More accurate identification and quantification of risks.
- Enhanced Decision Making: Better informed decisions regarding risk mitigation and resource allocation.
- Efficient Risk Mitigation: Strategies that consider risk interdependencies can be more effective.
Dependency Analysis: A method employed to understand and model the relationships and interactions between different risk factors within a business context.
Examples of Dependent Risk Models in Finance
Dependent risk models play a vital role in the world of finance by revealing how interconnected risks can affect investment portfolios, insurance, and credit systems. By understanding these models, you gain insights into how financial institutions manage the complexities of correlated financial risks. These models aid in creating strategies that anticipate systemic risks, helping protect assets in uncertain economic conditions.
Case Studies of Dependent Risk Models
Examining real-world applications of dependent risk models can offer valuable insights into their effectiveness and adaptability in finance. Below are notable case studies:
- Financial Crisis of 2008: During this period, many financial institutions employed copula models to assess credit risk. The Gaussian copula, used extensively for pricing collateralized debt obligations (CDOs), highlighted dependency challenges when assumptions about the normal distribution of risks proved inadequate under market stress.
- Portfolio Diversification: Banks use multivariate GARCH models to manage portfolios, assessing time-varying correlations and volatilities between assets.
- Insurance Risk: Insurers apply copula models to evaluate the dependency between different types of claims, such as property and casualty, to manage joint extreme events better.
Gaussian Copula: A statistical model used to describe the dependency structure between multiple financial risks, often applied in credit risk management to simulate correlations in financial portfolios.
The limitations of the Gaussian copula unveiled during the 2008 financial crisis led to the development of more sophisticated models that account for tail dependencies and non-linear correlations. The t-copula, an advancement, includes heavy-tailed distributions to represent extreme financial events better. It is defined by its degrees of freedom \(v\) and is expressed as:\[ C_T(u_1, u_2; v, \rho) = \text{T}_2(\text{T}^{-1}(u_1), \text{T}^{-1}(u_2); v, \rho) \]where \(\text{T}\) and \(\text{T}^{-1}\) are the cumulative and inverse cumulative distribution functions of the t-distribution. By integrating heavy-tailed characteristics, the t-copula provides a more robust framework for assessing extreme dependencies between risk factors in financial markets.
Lessons from Dependent Risk Models in Financial Contexts
In financial contexts, dependent risk models provide critical lessons in risk assessment and management, highlighting the importance of understanding risk interconnections. Employing such models equips financial institutions with foresight to tackle systemic challenges.Several key lessons are derived from these models:
- Accurate Risk Valuation: Models improve the valuation of financial assets by incorporating dependencies, leading to better pricing strategies.
- Stress Testing: They enable institutions to conduct stress testing under various scenarios, identifying potential vulnerabilities in financial systems.
- Capital Allocation: Dependent risk models inform decisions on capital reserves, ensuring adequate buffers for correlated risks.
Consider a bank analyzing its credit portfolio's exposure to economic downturns. By using a multivariate GARCH model, the bank evaluates how macroeconomic variables such as unemployment rates and GDP influence loan defaults concurrently. The model helps optimize capital allocation by highlighting risk correlation, ensuring the bank maintains sufficient reserves to withstand economic shocks.
dependent risk models - Key takeaways
- Dependent Risk Models Definition: Models assessing interrelated risks in business, predicting risk behavior, and aiding decision-making.
- Actuarial Theory for Dependent Risks: Utilizes mathematical and statistical methods to predict risk outcomes, key for managing risk in sectors like insurance and finance.
- Techniques in Dependent Risk Assessment: Divided into qualitative (e.g., scenario analysis, expert judgment) and quantitative methods (e.g., statistical models, Monte Carlo simulation).
- Modelling Dependence with Copulas: Captures dependency between variables, allowing separation of marginal distributions to describe relationships without assuming identical distributions.
- Dependency Analysis Models Risk: Models that quantify relationships between risks, categorized by methodologies like linear regression, copulas, and Bayesian networks.
- Examples in Finance: Use of Gaussian copula models in credit risk assessments, multivariate GARCH models for portfolio diversification, and insurance risk management through copula models.
Learn faster with the 24 flashcards about dependent risk models
Sign up for free to gain access to all our flashcards.
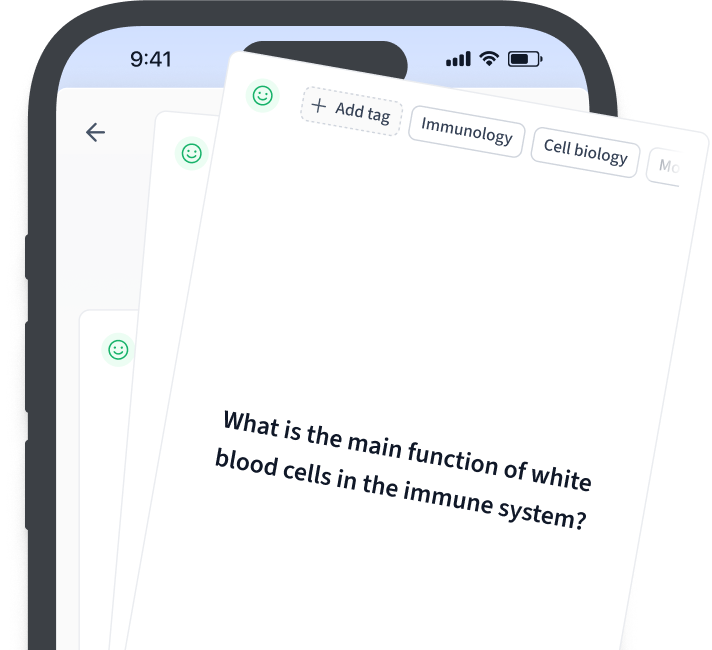
Frequently Asked Questions about dependent risk models
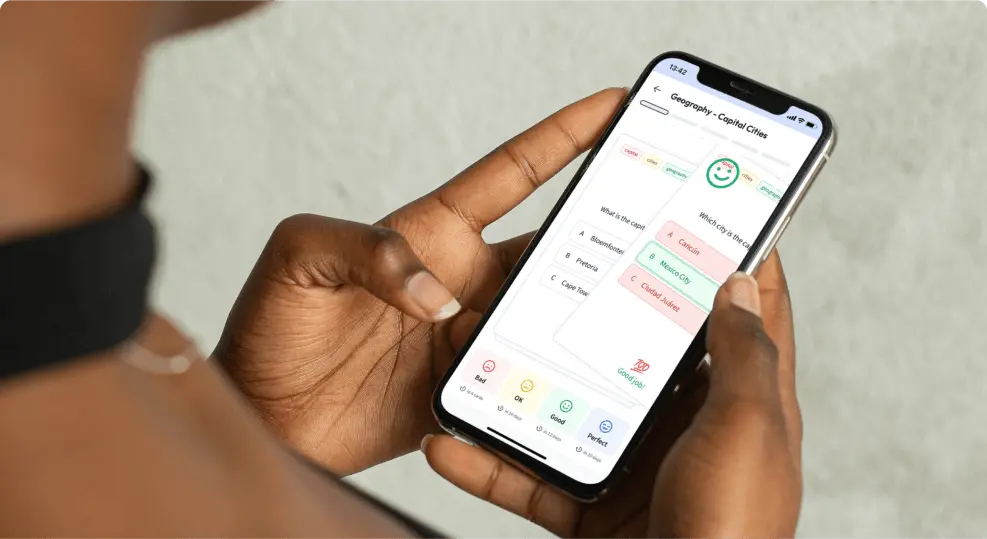
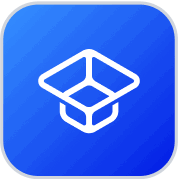
About StudySmarter
StudySmarter is a globally recognized educational technology company, offering a holistic learning platform designed for students of all ages and educational levels. Our platform provides learning support for a wide range of subjects, including STEM, Social Sciences, and Languages and also helps students to successfully master various tests and exams worldwide, such as GCSE, A Level, SAT, ACT, Abitur, and more. We offer an extensive library of learning materials, including interactive flashcards, comprehensive textbook solutions, and detailed explanations. The cutting-edge technology and tools we provide help students create their own learning materials. StudySmarter’s content is not only expert-verified but also regularly updated to ensure accuracy and relevance.
Learn more