Jump to a key chapter
Forecasting Risk Definition
In the realm of business studies, understanding forecasting risk is crucial for making informed decisions. It revolves around the uncertainty involved when making predictions about future business conditions. Reliable forecasts are essential for strategic planning, investing, budgeting, and resource allocation. However, due to ever-present uncertainties, forecasting risk can never be entirely eliminated.
Key Aspects of Forecasting Risk
Forecasting risk in business can be dissected into several key aspects that should be carefully considered:
- Volatility: The degree of fluctuation in market conditions can significantly affect forecasts.
- Complexity: Complicated variables and their interrelations can challenge accurate forecasting.
- Time Horizon: The longer the timeframe, the greater the forecasting risk due to increasing uncertainty over time.
Quantifying Forecasting Risk
Quantifying forecasting risk involves the use of various models and statistical tools. These tools enable businesses to assess the potential variability in their forecasts and make informed decisions. Statistical methods such as regression analysis, time series analysis, and Monte Carlo simulations can be used. For example, in regression analysis, a basic formula might look like this: \[ Y = a + bX + e \] Where:
- Y is the dependent variable (e.g., sales forecast)
- a is the intercept
- b is the slope coefficient
- X is the independent variable (e.g., advertising spend)
- e is the error term
Importance of forecasting risk: It refers to the uncertainty and potential for error in predicting future states or trends in a business context.
Consider a company estimating future sales based on current market trends. If consumer preferences shift unexpectedly, the actual sales could deviate significantly from the forecast, demonstrating forecasting risk.
Beyond basic methods, advanced models incorporate machine learning algorithms to improve accuracy and reduce forecasting risk. Techniques like neural networks analyze large datasets to identify hidden patterns and trends. Additionally, leveraging big data analytics and predictive analytics can provide deeper insights and more refined forecasts. These modern techniques help businesses adapt to rapidly changing environments, adjusting their strategies according to real-time data inputs. It's worth noting that the choice of model often depends on the specific context and available data, always keeping in mind the underlying uncertainties.
Remember that no forecasting method can offer guarantees. The objective is to minimize risk, not eliminate it.
Techniques of Forecasting Risk
Forecasting risk involves predicting future uncertainties in business, which is crucial for decision-making. Various techniques can be utilized to manage and mitigate this risk effectively.
Statistical Methods
Statistical methods are often employed to reduce forecasting risk, providing a quantitative basis for predictions. Examples include:
- Time Series Analysis: This method analyzes historical data to predict future trends.
- Regression Analysis: Establishes relationships between variables to forecast impacts. The formula for linear regression is: \[ Y = a + bX + e \]
- Monte Carlo Simulation: Uses random sampling and statistical modeling to compute risk probabilities.
Qualitative Techniques
Qualitative techniques apply expert judgment and market research to anticipate future trends, particularly useful when historical data is sparse. Key techniques include:
- Delphi Method: Uses a panel of experts to gain insights through multiple rounds of questioning.
- Scenario Analysis: Evaluates how future events might evolve by constructing different scenarios.
Imagine a tech company launching a new product. They might use scenario analysis to explore potential outcomes based on different market conditions, assessing the impact on projected sales.
Hybrid Approaches
Combining both qualitative and quantitative techniques often yields more reliable forecasts. Hybrid approaches capitalize on the strengths of statistical and expert methodologies. For example, integrating statistical forecasts with insights from industry experts can improve accuracy. This approach can blend computation rigor with qualitative insight, considering multiple facets of risk.
Machine learning is revolutionizing forecasting by offering advanced hybrid techniques. Algorithms like decision trees and neural networks can process vast data and adjust predictions as they learn from new information. Businesses can harness predictive analytics, which uses machine learning to enhance forecasting models continuously. Consider how a neural network might be trained on historical sales data; as new sales information becomes available, the model recalibrates its prediction patterns to improve future forecasts. This dynamic adaptation means businesses can respond more agility to changing conditions, reducing forecasting risk more than ever before.
Forecasting Risk Examples
Examples of forecasting risk in the business world highlight the multifaceted challenges of predicting future trends. These examples can provide you with valuable insights into how businesses navigate uncertainties and improve decision-making processes.
Sales Forecasting in the Retail Sector
In the retail industry, businesses often face challenges due to forecasting risk when predicting sales for upcoming seasons. Companies might depend on historical sales data and seasonal trends to estimate future demand, yet unexpected factors like economic shifts or changes in consumer behavior can lead to inaccuracies. To illustrate this, consider the formula often used to predict sales based on advertising spend: \[ \text{Sales} = a + b(\text{Advertising}) + e \] Let's assume historical data suggests that for every $1,000 spent on advertising, sales potentially increase by $5,000. This linear relationship may not always hold during unexpected events like a market downturn.
A retail chain derived predictions from past data, suggesting a 20% increase in winter coat sales. However, an unusually warm winter resulted in decreased demand, highlighting the forecasting risk involved.
Technology and Innovation Predictions
Tech companies often experience forecasting risk when predicting the impact of new innovations. The rapid pace of technological change can render current products obsolete quickly. One analytical method to assess risk is the use of Monte Carlo simulation, where the following process is applied:
- Define the potential outcomes for an innovation launch.
- Use random sampling to simulate various scenarios.
- Evaluate the range of possible impacts on market share.
In tech forecasting, machine learning is an emerging tool used to anticipate trends. Machine learning models can process large datasets, learning from patterns to make predictions about future market behavior. For example, a machine learning algorithm may be trained on historical data, customer feedback, and macroeconomic indicators to forecast tech adoption rates. The adaptability of machine learning aids in minimizing forecasting risk by updating predictions in response to new data inputs, providing a dynamic edge over traditional forecasting methods.
Remember, effective use of forecasting models often involves combining quantitative data with qualitative market insights to enhance accuracy.
Financial Risk Forecasting
Financial risk forecasting involves predicting potential risks that can affect the financial health of a business. This process is essential for making strategic decisions that safeguard against losses and ensure sustainable growth. By identifying and evaluating financial risks, businesses can prepare better and mitigate adverse impacts.
Risk Forecasting Methodology
There are various methodologies employed in risk forecasting, each offering unique insights and benefits. These methodologies often combine quantitative and qualitative analyses to enhance predictive accuracy.
Quantitative Analysis: This involves numerical techniques that rely on data and statistical models to forecast risk. Examples include regression analysis, time series analysis, and Monte Carlo simulations.
Consider a company using regression analysis to predict its future cash flow based on historical data. The basic equation might look like this: \[ Y = a + bX + e \]Where:
- Y represents future cash flow.
- a is the intercept.
- b is the gradient or the rate of change.
- X is an independent variable, such as sales.
- e is the error term.
It is crucial to ensure that data used in quantitative analyses are accurate and reflective of current market trends to improve forecasting reliability.
Qualitative Analysis: This approach relies on expert judgment and insights to forecast risks. It is particularly valuable when numerical data are limited or when evaluating emerging risks.
Advanced econometrics and machine learning techniques are increasingly used in financial risk forecasting. When using machine learning, a model such as a neural network can analyze massive datasets to discern patterns and predict risks. For instance, consider a neural network trained to predict credit risk by learning from a history of borrower information and economic trends. As new data arrives, the model adjusts its weights, enhancing its forecasting accuracy. This approach is particularly beneficial in volatile financial markets where traditional models might fall short.
Forecasting Risk in Decision Making
Forecasting risk plays a critical role in decision-making processes within a business. Effective risk forecasting allows companies to anticipate challenges and make informed decisions that can mitigate potential negative impacts. Here’s how forecasting risk is integrated into decision making:
- Strategic Planning: Risk forecasts inform long-term strategies by highlighting potential vulnerabilities and opportunities.
- Investment Decisions: Understanding potential risks helps in evaluating the feasibility and potential returns of investment options.
- Resource Allocation: Forecasts guide the optimal allocation of resources, reducing wastage and improving efficiency.
Scenario analysis is a valuable technique for incorporating risk forecasting into decision-making. It involves creating multiple scenarios based on different assumptions about future conditions, allowing decision makers to visualize potential outcomes and devise strategic contingencies. For instance, a tech company might create different scenarios for a new product launch considering factors like market adoption rate, competitor activity, and economic conditions. This method provides a comprehensive view of risk implications, facilitating well-rounded decision-making.
forecasting risk - Key takeaways
- Forecasting risk definition: The uncertainty and potential for error in predicting future business conditions, crucial for informed decision-making.
- Techniques of forecasting risk: Employs both statistical methods (e.g., regression analysis, time series) and qualitative approaches (e.g., Delphi Method, scenario analysis).
- Forecasting risk examples: Highlight unpredictable changes in sales forecasts due to external factors like consumer behavior or technological change.
- Financial risk forecasting: Predicts potential risks affecting financial health, using quantitative methods like regression analysis and qualitative insights.
- Risk forecasting methodology: Combines data-driven models with expert judgment to assess and predict business risks effectively.
- Forecasting risk in decision making: Helps businesses anticipate challenges, improve strategic planning, investment decisions, and resource allocation.
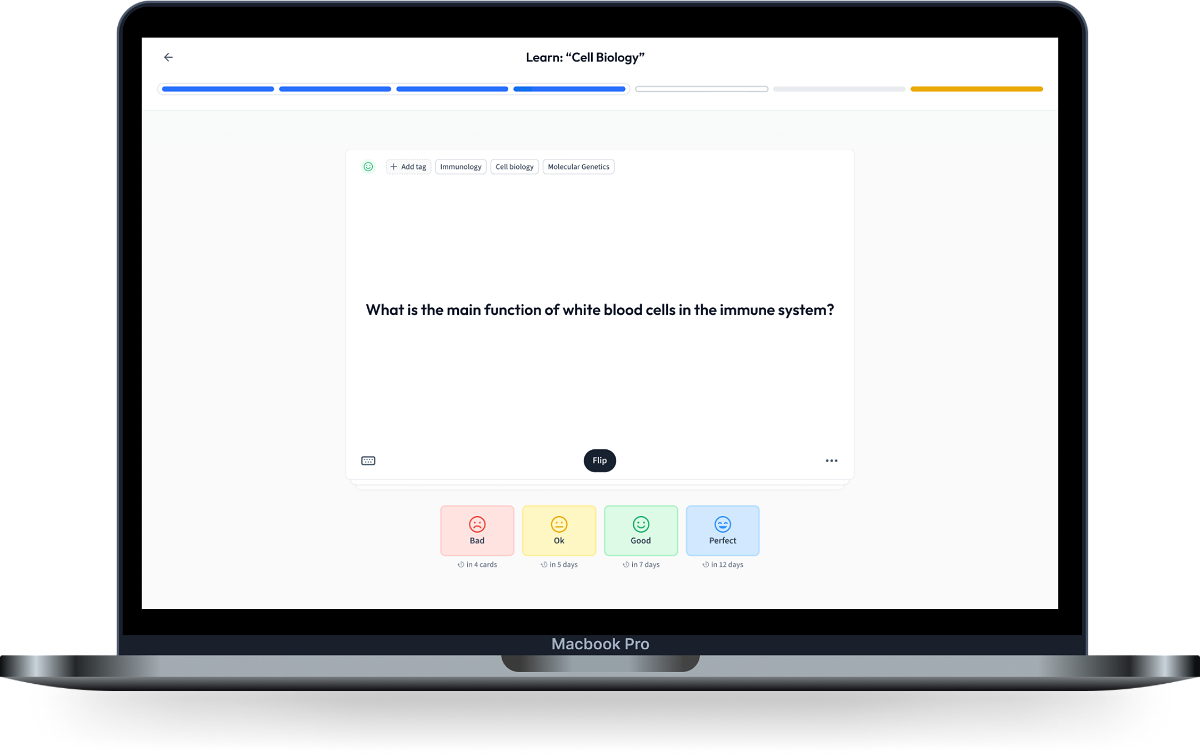
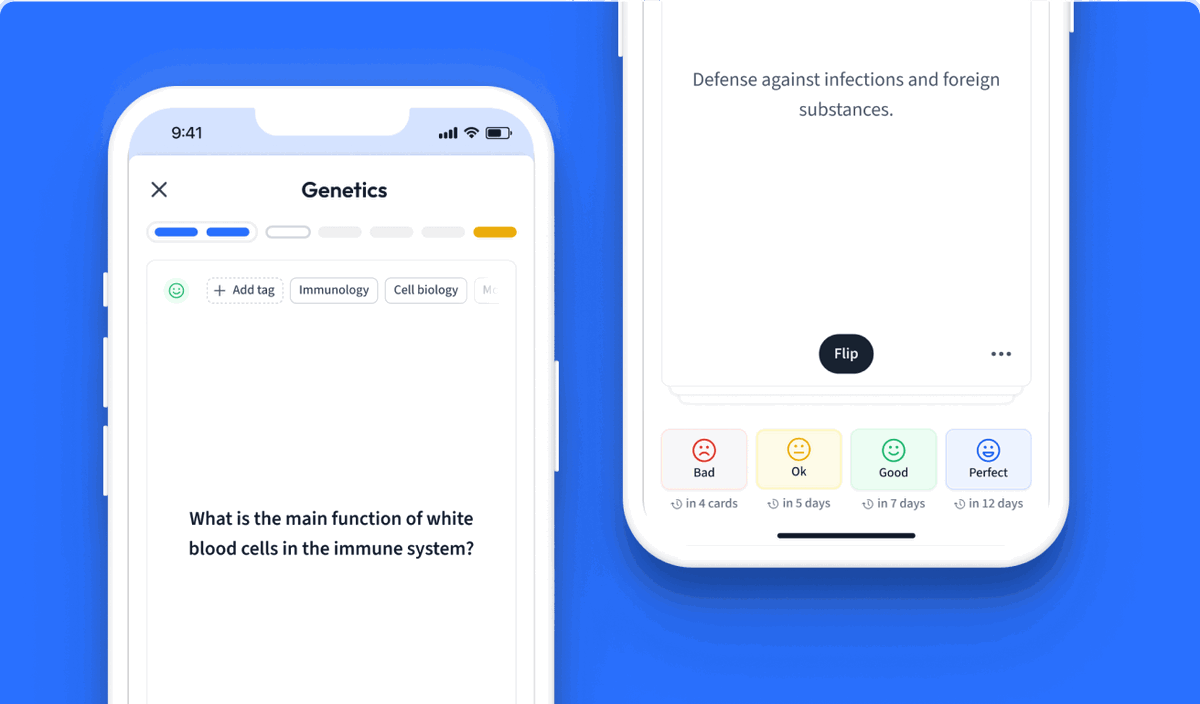
Learn with 24 forecasting risk flashcards in the free StudySmarter app
Already have an account? Log in
Frequently Asked Questions about forecasting risk
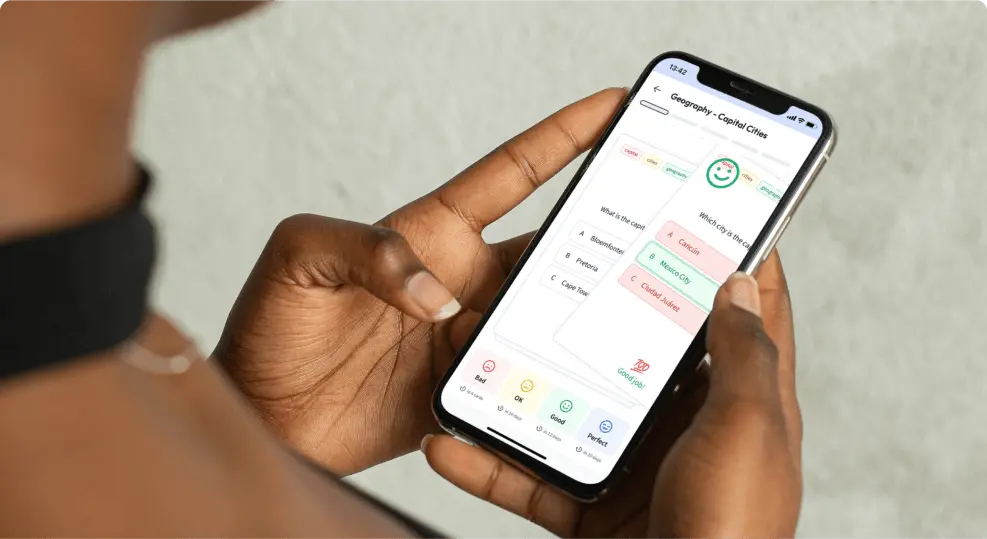
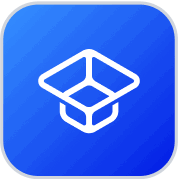
About StudySmarter
StudySmarter is a globally recognized educational technology company, offering a holistic learning platform designed for students of all ages and educational levels. Our platform provides learning support for a wide range of subjects, including STEM, Social Sciences, and Languages and also helps students to successfully master various tests and exams worldwide, such as GCSE, A Level, SAT, ACT, Abitur, and more. We offer an extensive library of learning materials, including interactive flashcards, comprehensive textbook solutions, and detailed explanations. The cutting-edge technology and tools we provide help students create their own learning materials. StudySmarter’s content is not only expert-verified but also regularly updated to ensure accuracy and relevance.
Learn more