Jump to a key chapter
What is a Data Catalog
A data catalog is an organized inventory of data assets across an organization, aimed at providing a comprehensive view of all available data. It is a crucial tool for managing, discovering, and governing data efficiently.Data catalogs use metadata to organize and index data sources, allowing users to quickly find and retrieve information they need.
Purpose and Importance of a Data Catalog
The purpose of a data catalog is to improve data accessibility and usability within an organization. By centralizing data information, it facilitates:
- Data Discovery: Users can easily find the data they need.
- Data Understanding: Provides context about data sources and their relationship to each other.
- Data Governance: Ensures appropriate data usage and compliance.
- Collaboration: Promotes data sharing and collaboration among teams.
Data Catalog: A centralized repository that acts as an inventory for all data assets in an organization, helping users discover, understand, and manage data.
Components of a Data Catalog
A fully functional data catalog consists of several key components:
- Metadata Repository: Stores metadata about each data asset, such as source, structure, and usage.
- Data Lineage: Tracks the origin and evolution of data across its lifecycle.
- Search and Discovery Tools: Enables users to locate data assets through various search methods.
- Collaboration Features: Facilitates user interaction and knowledge sharing about data assets.
- Security and Permissions: Controls access to data according to user roles and policies.
Consider a retail company using a data catalog. With all product sales data cataloged, analysts can easily search and analyze sales trends, inventory records, and customer feedback to make informed decisions.
The evolution of data catalogs can be traced back to traditional library card catalogs. As businesses began handling larger volumes of data, the need for a digital equivalent became essential. Modern data catalogs not only store information but are enriched with AI and machine learning capabilities to enhance search efficiency and automate data tagging. These advanced features allow data catalogs to evolve continuously, adapting to the growing complexity and volume of organizational data.
Data Catalog Concepts and Techniques
A data catalog is an essential tool for organizations looking to efficiently manage and utilize their data assets. By integrating various data management techniques, data catalogs enhance data discoverability and usability. Let’s explore some fundamental concepts and techniques associated with data catalogs.
Metadata in Data Catalogs
Metadata serves as the foundation of a data catalog. It provides descriptive information about data assets, helping users understand the source, structure, type, and relationships of data.
- Descriptive Metadata: Details the characteristics of data, such as title, creator, and descriptions.
- Structural Metadata: Describes the format and structure of data files or databases.
- Administrative Metadata: Deals with technical aspects, such as data creation date and usage rights.
Effective metadata management can significantly ease the process of data discovery and retrieval.
Data Lineage and Provenance
Understanding where data comes from and how it has been transformed over time is crucial. This is where data lineage and provenance play a role.
- Data Lineage: Maps out the data journey from its origin, through changes, to its current state.
- Data Provenance: Documents the data’s source and history to validate its authenticity and integrity.
Example: In the healthcare industry, tracing patient data through different electronic health records involves meticulous data lineage and provenance tracking to ensure patient information is both accurate and complete.
Search and Queryability in Data Catalogs
A data catalog without effective search capabilities is analogous to a library without a catalog system. Key features include:
- Text Search: Allows users to type queries and find relevant data assets quickly.
- Faceted Search: Lets users filter results based on various criteria, such as data type, date modified, or author.
- Advanced Querying: Supports complex searches using SQL or other query languages to locate specific data.
Leveraging AI-enabled search functions can greatly enhance the relevance of search results in large data catalogs.
Incorporating machine learning algorithms into data catalogs can revolutionize the way data is managed and accessed. For instance, by analyzing user interaction patterns, a data catalog can optimize metadata tagging, predict user needs, and suggest relevant data sets. Additionally, automated data classification helps in maintaining an updated inventory, thereby reducing manual errors. Integrating AI-driven insights into data catalogs not only streamlines data operations but also enhances the overall user experience, leading to smarter and quicker decision-making processes.
Importance of Data Cataloging in Business Studies
Data cataloging is pivotal in business studies as it supports efficient data management and drives data-driven decision-making. Its role in elevating the business landscape cannot be overstated. Let's delve into various aspects of its importance.
Enhancing Data Accessibility
A data catalog improves data accessibility by providing a structured directory of data resources. This is vital for any business because it:
- Enables quicker data discovery for analytics.
- Facilitates efficient data retrieval, saving time.
- Improves collaboration among departments by offering a unified data view.
Picture an e-commerce firm using a data catalog to monitor customer purchase patterns. By utilizing these insights, the marketing team can tailor promotional strategies effectively.
Supporting Data Governance
Data governance is a critical component of business integrity and compliance, and data cataloging plays a crucial role in this. It:
- Ensures data accuracy and consistency through comprehensive cataloging.
- Enforces data usage policies and access controls.
- Provides audit trails with data lineage tracking.
Implementing a robust data governance mechanism within a data catalog can prevent unauthorized access and misuse of data resources.
Facilitating Data-Driven Decision Making
The use of a data catalog empowers businesses to make informed decisions based on quality data. This is achieved through:
- Ease of access to real-time data.
- Comprehensive data insights enabling strategic planning.
- Continuous monitoring of data usage trends to refine business strategies.
The integration of artificial intelligence (AI) into data catalogs is transforming the way businesses utilize data. AI-driven data catalogs can not only automate data tagging and cleansing processes but also provide predictive analytics and pattern recognition. This enhances the analytical capabilities of businesses significantly. Furthermore, AI can identify data anomalies and suggest corrective actions, aiding businesses to maintain high data integrity and reliability. Such advancements set the stage for more proactive and strategic decision-making in business operations.
Data Catalog Examples for Students
Understanding data catalogs can be made easier by exploring practical examples relevant to business studies. Through these examples, you can grasp how data cataloging can be applied in real-world scenarios.
Academic Research Database Catalog
In academic institutions, research databases are crucial for organizing vast amounts of scholarly data. Using a data catalog in this context can:
- Provide a centralized repository for dissertation and thesis metadata, including authors, topics, and publication dates.
- Facilitate student access to peer-reviewed articles and journals quickly.
- Allow faculty members to track ongoing research trends and collaborate effectively.
Example: A university library employs a data catalog to organize and index its digital and print resources. This enables students to search for materials by subject or author, making research more efficient.
Retail Business Sales Catalog
Retail businesses can leverage data catalogs to manage sales data effectively. A retail sales catalog can:
- Compile sales forecasts, employee schedules, and inventory levels.
- Enable data-driven decisions for pricing strategies and promotions.
- Track consumer patterns and preferences for targeted marketing campaigns.
Retail chains often use data catalogs to integrate customer feedback into their product offerings. By cataloging data from customer surveys and reviews, retailers can identify popular products and discontinue those underperforming. Additionally, advanced analytics through data catalogs can predict shopping trends, enabling retailers to adjust their inventory accordingly. Such proactive measures help retail businesses maintain competitiveness and adapt to changing consumer demands.
Healthcare Patient Records Management
In healthcare, data catalogs are utilized to organize and manage patient records. This includes:
- Storing electronic health records (EHR) with complete patient history.
- Providing physicians with quick access to patient data for more accurate diagnoses.
- Maintaining compliance with healthcare regulations through secure data management.
Example: A hospital employs a data catalog to manage patient health records, lab results, and treatment plans. This allows healthcare professionals to coordinate care more effectively and improves patient care quality.
data cataloging - Key takeaways
- What is a Data Catalog: A data catalog is a centralized repository and inventory of data assets in an organization, enhancing data discovery, understanding, governance, and collaboration.
- Importance in Business Studies: Data cataloging supports efficient data management and data-driven decision-making, essential in improving productivity and strategic business planning.
- Components of a Data Catalog: Includes metadata repository, data lineage, search and discovery tools, collaboration features, and security permissions.
- Data Catalog Techniques for Students: Employ data catalog in academic and research databases to organize scholarly data and facilitate efficient academic collaboration.
- Data Catalog Examples: Examples include its application in retail business sales management, healthcare patient records, and academic research databases for enhanced data management.
- AI and Machine Learning Enhancements: Integration of AI and machine learning in data catalogs enhances search efficiency, metadata tagging, and overall data management, leading to improved decision-making processes.
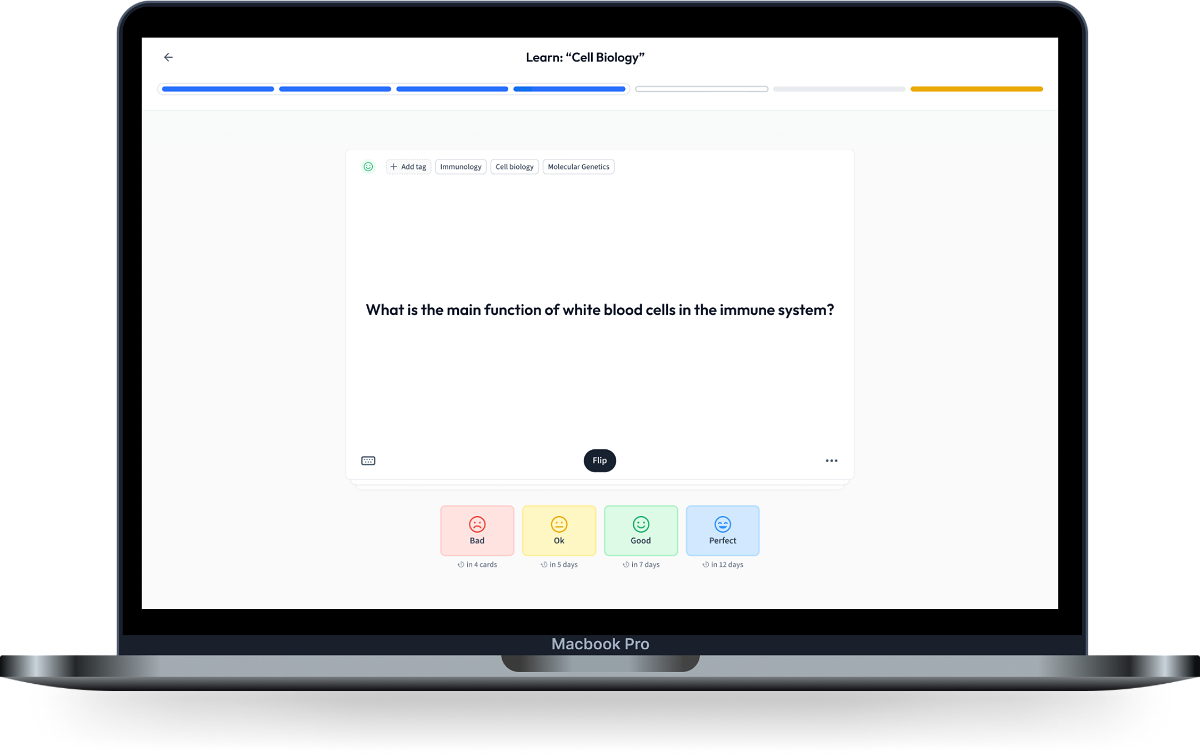
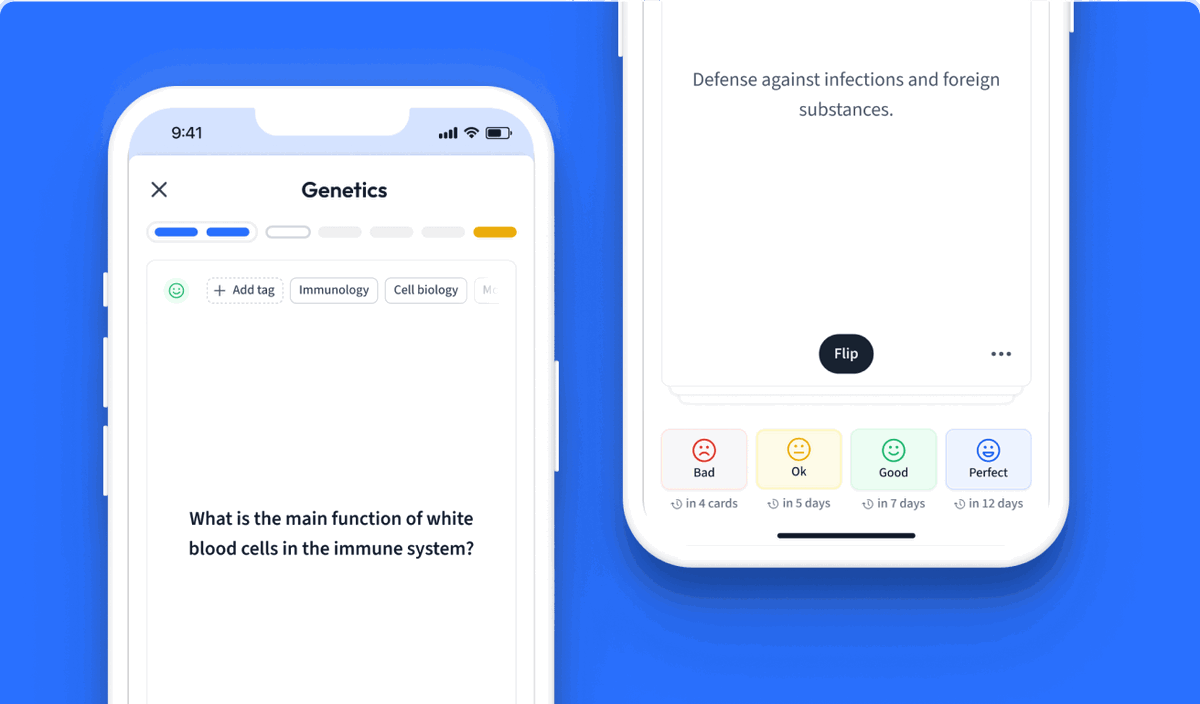
Learn with 12 data cataloging flashcards in the free StudySmarter app
We have 14,000 flashcards about Dynamic Landscapes.
Already have an account? Log in
Frequently Asked Questions about data cataloging
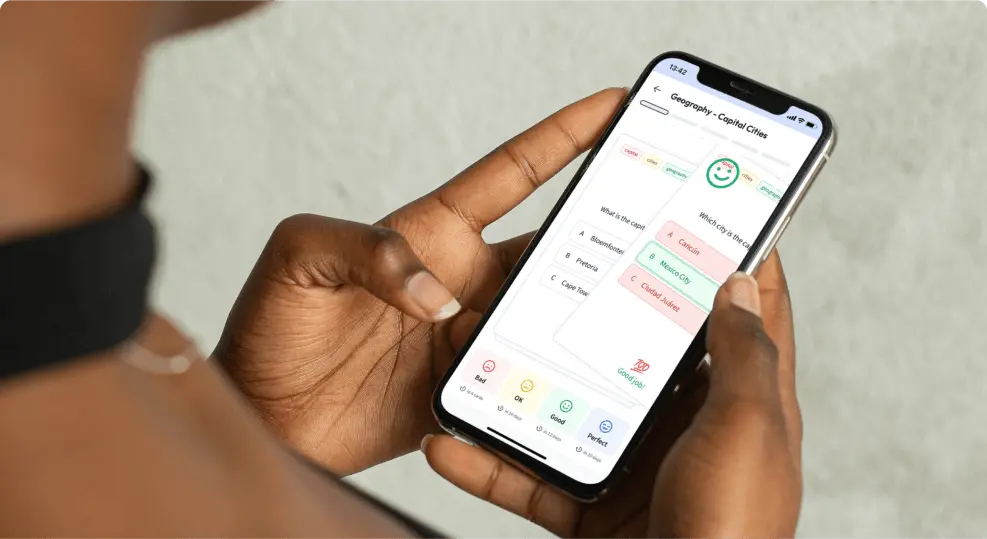
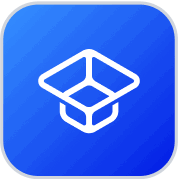
About StudySmarter
StudySmarter is a globally recognized educational technology company, offering a holistic learning platform designed for students of all ages and educational levels. Our platform provides learning support for a wide range of subjects, including STEM, Social Sciences, and Languages and also helps students to successfully master various tests and exams worldwide, such as GCSE, A Level, SAT, ACT, Abitur, and more. We offer an extensive library of learning materials, including interactive flashcards, comprehensive textbook solutions, and detailed explanations. The cutting-edge technology and tools we provide help students create their own learning materials. StudySmarter’s content is not only expert-verified but also regularly updated to ensure accuracy and relevance.
Learn more