Jump to a key chapter
What is Descriptive Analytics
Descriptive Analytics is a branch of business analytics that focuses on interpreting historical data to discern patterns and trends. This approach helps organizations understand the underlying reasons behind past performance and supports informed decision-making. It is crucial in transforming large volumes of data into actionable insights.
Definition of Descriptive Analytics in Business
Descriptive Analytics in the business context involves analyzing historical data to gain insights into past performance. The primary goal is to extract valuable patterns and trends by examining past behavior, allowing businesses to make more informed decisions.
You often encounter situations where businesses need to evaluate past performances. Descriptive analytics serves this need by offering insights derived from historical data. It helps in identifying patterns or trends that significantly affect business decisions. A typical process of descriptive analytics involves:
- Data gathering: Collecting historical data from various sources.
- Data processing: Cleaning and organizing data in a meaningful format.
- Data analysis: Using statistical tools to analyze data patterns.
- Visualization: Presenting data insights using charts or graphs.
Consider a retail company analyzing its sales data from the past year. By applying descriptive analytics, the company may determine that sales increased significantly during holiday seasons. This insight can inform business strategies like stock inventory or planning marketing campaigns in the future.
Descriptive analytics is often used alongside predictive and prescriptive analytics to provide a comprehensive view.
Descriptive analytics shines when it comes to understanding consumer behavior. Imagine you're a part of a marketing team wanting to analyze customer preferences based on previous purchase data. By leveraging descriptive analytics, you can categorize customers based on past transactions, preferences, and buying patterns. This not only helps in crafting personalized marketing strategies but also improves customer satisfaction. Descriptively analytical tools like SQL databases and reporting tools such as Tableau play an integral role. Utilizing these technologies allows analysts to efficiently process vast amounts of data and convert them into insightful visual reports. For example, a sales trend analysis algorithm might use SQL to filter and group sales data by time frame and region. The results can then be visualized in a histogram or line chart, facilitating easier interpretation and decision-making. Additionally, mathematical interpretation is sometimes necessary to quantify impacts. Consider analyzing the average sales revenue growth over different quarters. A simple mathematical formula might be used, such as the growth rate formula: \[\text{Growth Rate} = \frac{\text{Current Period Revenue} - \text{Previous Period Revenue}}{\text{Previous Period Revenue}} \times 100\%\] Such computations enable businesses to better gauge performance over time, providing another layer of understanding on how to strategize their growth.
Descriptive Analytics Examples in Business Studies
Descriptive analytics plays a vital role in helping businesses understand patterns from historical data. Through various applications, companies can make informed decisions that drive success. This section explores real-world instances where descriptive analytics is applied in business.
Real-World Applications of Descriptive Analytics
In today’s data-driven environment, descriptive analytics is implemented widely across business sectors. Some notable applications include customer behavior analysis, financial performance tracking, and supply chain management. By integrating descriptive analytics into these fields, businesses can gain a clearer understanding of their operations and adapt their strategies accordingly.
In terms of customer behavior analysis, descriptive analytics aids in understanding customer preferences and purchasing patterns. This insight is often visualized through customer segmentation or trend analysis.
A well-known fashion retailer used descriptive analytics to analyze seasonal sales data. By studying past purchasing trends, they discovered customers preferred specific styles during festive seasons. This led to optimized stock levels and targeted marketing initiatives, boosting sales significantly.
Consider using tools like SPSS and Excel, which are widely used for conducting descriptive statistical analysis.
In financial performance tracking, descriptive analytics provides deep insights into past revenue, expenses, and other crucial financial metrics. This tracking can help identify the difference between expected and actual financial performance using variance analysis. For example, variance can be calculated using: \[\text{Variance} = \text{Actual Value} - \text{Expected Value}\] Such analysis will show whether a company is over or under budget. In the context of supply chain management, descriptive analytics assists in monitoring inventory levels, supplier performance, and delivery schedules. By applying analytical models, businesses can forecast demand more accurately and reduce unnecessary inventory costs. Imagine a technology company that wants to optimize its supply chain. By leveraging descriptive analytics, the company identifies that certain suppliers consistently lead to delays. They utilized the following formula for calculating supplier lead time: \[\text{Lead Time} = \text{Delivery Date} - \text{Order Date}\] Calculated lead times across various suppliers helped the company to choose more reliable partners, thus ensuring timely product delivery to consumers.
Techniques of Descriptive Analytics
Descriptive analytics utilizes a range of techniques to transform raw data into comprehensible insights. By employing these methods, businesses can better understand their historical data and identify patterns and trends that influence their operations.
Common Methods in Descriptive Analytics
Descriptive analytics comprises several methods that are essential in analyzing historical data. These techniques include data aggregation, data mining, and data visualization.
Data Aggregation involves collecting and summarizing data from different sources to provide a unified overview. This process is crucial for decision-making as it highlights key performance indicators.
Consider a company that analyzes monthly sales data across its branches. By aggregating this data, it can discern trends, such as increased sales during summer, to adjust future inventory and marketing plans.
Another significant technique is data mining, where algorithms scrutinize large datasets to discover correlations and patterns. This method unveils hidden relationships within the data that can be of strategic value.
In data mining, patterns recognized through clustering can be used to segment customers into groups with shared characteristics. This segmentation is vital for targeted marketing and can be executed using algorithms like K-means. The mathematical foundation often begins with calculating the distance between data points using formulas such as the Euclidean distance: \[\text{Distance} = \sqrt{(x_2 - x_1)^2 + (y_2 - y_1)^2}\] By applying this formula, businesses categorize customers based on proximity, potentially leading to more effective marketing strategies.
Effective data mining can significantly enhance customer satisfaction by delivering customized experiences.
Data visualization is a third approach, wherein complex data is displayed as graphs or charts, allowing for quick interpretation. It supports decision-makers by transforming abstract numbers into compelling visual stories. Popular tools include Tableau or Power BI.
A financial institution uses line charts to present market trends over time. This visualization helps analysts notice patterns in stock performance, informing investment strategies.
Benefits of Descriptive Analytics in Business
Descriptive analytics plays a pivotal role in empowering businesses to make informed decisions. By analyzing past data, it provides invaluable insights that can enhance operational efficiency and strategic planning. In this section, you'll explore the numerous advantages of incorporating descriptive analytics into business operations.
Understanding Customer Behavior
Gaining insights into customer behavior is crucial for tailoring services and products to meet customer needs. Descriptive analytics helps businesses analyze historical customer data to identify purchasing patterns and preferences. By employing descriptive statistical tools, you can segment customers based on their buying habits. This segmentation aids in developing targeted marketing strategies and delivering personalized customer experiences. Consider using customer data to create profiles with attributes such as:
- Purchase frequency
- Product preferences
- Average spend per transaction
Imagine an online bookstore that uses descriptive analytics to track the genres most purchased by customers. By understanding these preferences, the bookstore can recommend titles that align with customer interests, enhancing both sales and customer satisfaction.
Customer satisfaction can significantly improve when businesses use descriptive analytics to personalize communication and offers.
Optimizing Operational Efficiency
Descriptive analytics aids in streamlining business processes by pinpointing inefficiencies and enhancing productivity. By analyzing past performance data, businesses can identify bottlenecks and areas of improvement across various operations. For instance, manufacturing firms can leverage data on production cycles, inventory levels, and equipment utilization. Through this analysis, these firms can determine patterns and implement changes that boost efficiency. A common approach might include monitoring production line data to assess:
- Machine downtime
- Cycle times
- Material wastage
A beverage company found through descriptive analytics that certain machines in its production line had frequent downtime due to minor technical issues. By addressing these issues, the company reduced downtime by 30%, resulting in enhanced productivity.
Deep-diving into inventory management through descriptive analytics can reveal useful insights. Let's say you manage a retail company seeking to optimize stock levels. By examining past sales data, lead times, and carrying costs, descriptive analytics aids in optimizing your reorder quantities, minimizing overstock or stockouts.The Economic Order Quantity (EOQ) model is a powerful tool here, providing insights into the ideal order size to minimize total costs. The EOQ formula is given by: \[\text{EOQ} = \sqrt{\frac{2DS}{H}}\] where:
By applying the EOQ model, businesses can determine the most cost-efficient order quantity, thereby achieving significant cost savings.descriptive analytics - Key takeaways
- Descriptive Analytics: Interprets historical data to find patterns and trends, crucial for making informed business decisions.
- Definition in Business: Analyzing historical data to extract insights into past performance, aiding informed decision-making.
- Descriptive Analytics Process: Involves data gathering, processing, analysis, and visualization to transform raw data into actionable insights.
- Examples in Business: Used in customer behavior analysis, financial performance tracking, and supply chain management.
- Techniques: Includes data aggregation, data mining, and data visualization to analyze and present historical data.
- Benefits: Enhances understanding of customer behavior and optimizes operational efficiency through data-driven insights.
Learn faster with the 140 flashcards about descriptive analytics
Sign up for free to gain access to all our flashcards.
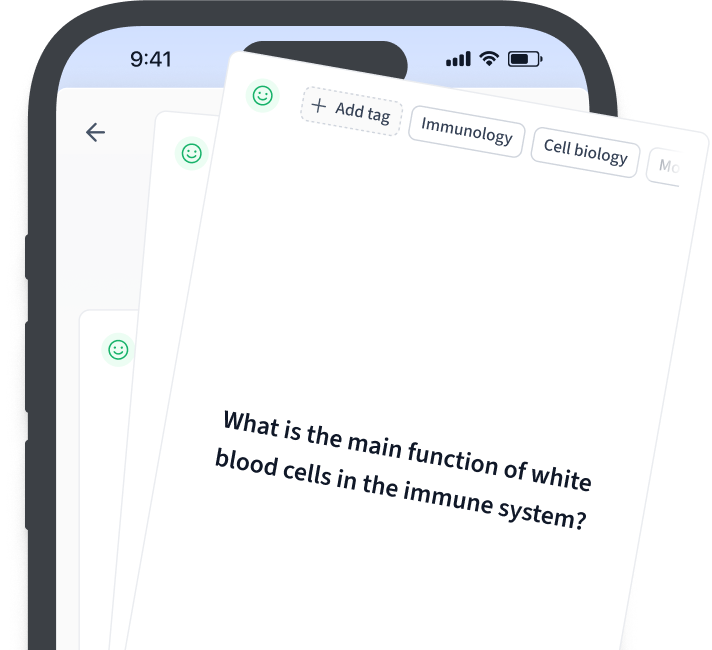
Frequently Asked Questions about descriptive analytics
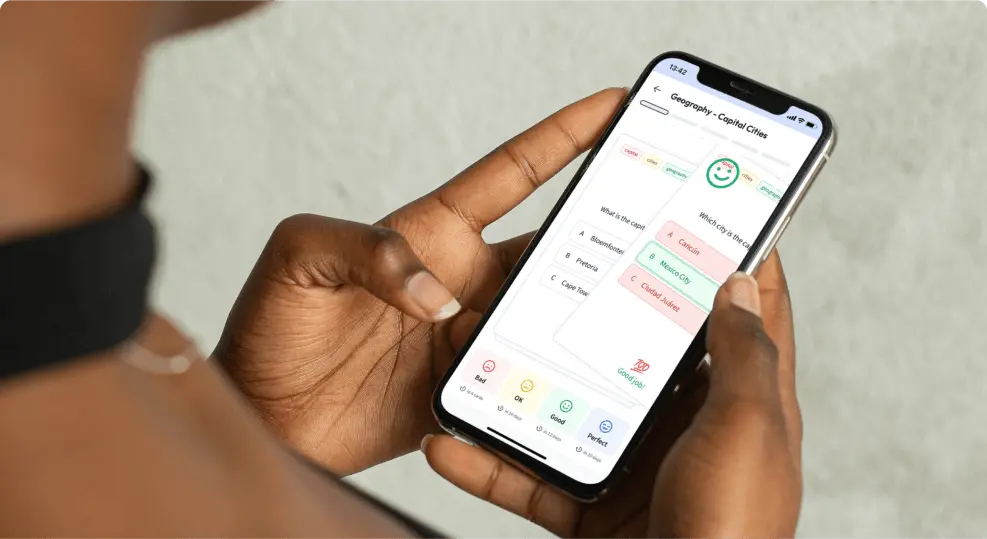
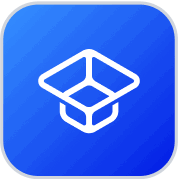
About StudySmarter
StudySmarter is a globally recognized educational technology company, offering a holistic learning platform designed for students of all ages and educational levels. Our platform provides learning support for a wide range of subjects, including STEM, Social Sciences, and Languages and also helps students to successfully master various tests and exams worldwide, such as GCSE, A Level, SAT, ACT, Abitur, and more. We offer an extensive library of learning materials, including interactive flashcards, comprehensive textbook solutions, and detailed explanations. The cutting-edge technology and tools we provide help students create their own learning materials. StudySmarter’s content is not only expert-verified but also regularly updated to ensure accuracy and relevance.
Learn more