Jump to a key chapter
Investment vehicles, essential components of financial education, allow you to grow and manage your resources effectively over time.
Investment vehicles come in various forms, each serving a unique purpose to match different financial goals.
Investment vehicles refer to the different methods available to invest money and earn potential returns. Here are some common types:
- Stocks: Ownership shares in a corporation that offer potential for growth and dividends.
- Bonds: Debt securities that represent a loan made by an investor to a borrower; known for being less risky than stocks.
- Mutual Funds: Pooled funds from multiple investors, managed by professionals, allowing diversification.
- Exchange-Traded Funds (ETFs): Similar to mutual funds, but traded on stock exchanges, often tracking specific indices.
- Real Estate: Includes physical properties or real estate investment trusts (REITs) representing ownership in property assets.
Investment Vehicles are instruments through which individuals and institutions can place their funds and expect a future return on them.
Remember, more diversified investment vehicles generally present lower risk diversifications.
Suppose you buy 10 shares of a company at $50 each. If the company issues $2 per share as a dividend, your annual income would be \(10 \times 2 = 20\) dollars.
Though ETFs and mutual funds can look similar, their trading mechanisms differ. ETFs are bought and sold throughout the day like stocks, potentially affecting their valuation and liquidity. Understanding this can guide your decision on which vehicle to choose based on your trading confidence and strategies.
Each investment vehicle offers unique benefits that cater to diverse financial needs and risk appetites.
Investing in different types of investment vehicles can yield various benefits:
- Diversification: By spreading investments across different vehicles, you can reduce risk.
- Growth Potential: Stocks and mutual funds offer the potential for high returns over time.
- Steady Income: Bonds and real estate can provide regular income in the form of interest and rent.
- Liquidity: Some vehicles like ETFs and stocks have higher liquidity, allowing easier access to cash.
Consider investing $1,000 in a bond offering 5% annual interest compounded annually. Over five years, the investment grows to \( 1000\left(1 + \frac{0.05}{1}\right)^{5} = 1276.28 \) dollars.
Diversifying across different asset classes not only mitigates risk but also maximizes the potential for returns.
Real estate investment provides a dual advantage of asset appreciation and rental income. Although it requires a higher upfront cost and involves more expenses for upkeep, it often serves as a hedge against inflation. The property value appreciation can be calculated using the formula: \[ V = P\left(1 + r\right)^t \] where V represents the future value of the property, and P is the initial purchase price.
The intersection of investment strategies and computer science has given rise to sophisticated algorithms reshaping financial landscapes.
In the realm of investment algorithms, computer science plays a pivotal role. Algorithms, which are essentially step-by-step procedures for solving specific tasks, are utilized in optimizing and automating investment decisions.These algorithms are built on mathematical models and statistical techniques to predict market trends and make data-driven decisions. Let's explore the basics of how these algorithms function in the investment sector.
Investment algorithms in computer science are vital for automating and optimizing decision-making processes.
At the core of investment algorithms are key components like data processing, decision-making logic, and execution capabilities. These components work collectively to:
- Analyze Large Data Sets: Algorithms process vast amounts of financial data to identify trends.
- Predict Market Behavior: Advanced statistical models forecast market movements.
- Make Automated Trades: Algorithms can execute trades based on predefined criteria.
Consider a scenario where an algorithm analyzes stock prices over a period to calculate the average return. By employing the formula \[ \text{Average Return} = \frac{\sum_{i=1}^{n} r_i}{n} \] where \( r_i \) represents the returns for each period, the algorithm can determine the mean performance.
Investment algorithms often incorporate machine learning to improve accuracy over time by learning from historical data.
More complex algorithms utilize neural networks, which mimic the human brain's structure to identify non-linear relationships in financial data. This involves the use of activation functions such as the sigmoid function, calculated as: \[ \sigma(x) = \frac{1}{1 + e^{-x}} \] Neural networks can adaptively learn patterns that aren't immediately obvious, providing improved predictions and timing for investments.
Algorithms are indispensable tools in enhancing the efficiency and success of investment vehicles.
The role of algorithms in investment vehicles is multi-faceted. They serve the primary functions of risk assessment, market analysis, and trade execution:
- Risk Assessment: Algorithms help analyze portfolio risk through models like Value at Risk (VaR).
- Market Analysis: Utilizing sentiment analysis, algorithms assess market sentiment from various data sources.
- Trade Execution: High-frequency trading (HFT) algorithms execute numerous trades in fractions of a second.
Software platforms like Interactive Brokers employ trading algorithms that let users automate their strategies for consistent and swift trade execution.
Using algorithms, investors can react to market changes in real-time, seizing opportunities as they arise.
Algorithmic trading's success is partially attributed to its ability to eliminate human emotions such as fear and greed, which often skew investment decisions. Algorithms follow rigorous logical paths, influenced solely by hard data. This objectivity can offer a competitive edge, especially in volatile markets. Additionally, they employ strategies like arbitrage to profit from price discrepancies across markets — an intricate method that involves trading the same asset in different markets at varied prices.
Computer Science Investment Methods
In the modern world, investment methods in computer science offer innovative ways to manage and enhance investment portfolios. These methods leverage technology to analyze financial markets and automate trade decisions, unlocking new potential for savvy investors.Understanding these techniques can provide a competitive advantage and facilitate informed investment decisions.
Techniques for Analyzing Investment Vehicles
Analyzing investment vehicles requires a combination of quantitative and qualitative techniques to assess their potential. These involve data-driven strategies that include:
- Fundamental Analysis: This involves evaluating the underlying value of an asset by examining financial statements, market conditions, and economic factors.
- Technical Analysis: Utilizing charts and statistical figures to predict future price movements based on historical data.
- Quantitative Models: Mathematical models to evaluate assets, often involving statistical tools like regression analysis.
MACD = EMA(12) - EMA(26)Signal Line = EMA(MACD, 9)Histogram = MACD - Signal LineThis calculation assists in determining trade signals based on market momentum.
Fundamental Analysis evaluates the intrinsic value of an asset by considering economic factors and financial health.
Suppose you are analyzing a company's financial health by reviewing its revenue growth, profit margins, and debt levels. A steady revenue growth trend might signal that the company is thriving in its market.
Technical analysis can be supported by tools like the Relative Strength Index (RSI) to indicate overbought or oversold conditions.
Quantitative models are often employed by financial firms to automate analysis, reducing human bias. An example is the Black-Scholes model, used to calculate options pricing, represented by the formula:
C = S0N(d1) - Xe^{-rt}N(d2)where C is the call option price, S0 is the current stock price, X is the exercise price, and t is the time to expiration.
Innovative Investment Vehicle Techniques in Computing
In the landscape of computing, investment vehicle techniques have evolved to include complex algorithms and artificial intelligence. These innovations facilitate sophisticated analysis and heightened trading capabilities:
- Algorithmic Trading: Uses pre-programmed instructions for trading, optimizing speed and accuracy in financial markets.
- Machine Learning Models: These algorithms learn from data patterns to enhance decision-making and predictions.
- Blockchain Technology: Brings transparency and security to transactions, impacting online investment practices.
import pandas as pdfrom trading_platform import calculate_signaldata = pd.read_csv('market_data.csv')signal = calculate_signal(data)if signal == 'buy': execute_trade('buy', amount)This code snippet illustrates a basic setup for automated trade execution based on calculated market signals.
Algorithmic Trading is the process of using algorithms to execute trades at high speed and frequency based on specific market analyses.
A hedge fund uses a machine learning model to analyze historical price data and identify the best times for entering or exiting market positions.
Blockchain technology, while commonly known for cryptocurrencies, is increasingly applied in secure financial transactions.
Blockchain's decentralized database model ensures the integrity of transaction records. It operates by maintaining a ledger where every participant holds a copy, making it nearly impossible to alter without consensus. This characteristic not only elevates security for digital currencies but is also beneficial for recording contracts and transaction verification across various industries.
Educational Investment Theory in CS
Educational investment theory in computer science provides an analytical framework to enhance decision-making related to investments in educational technologies. By applying principles from both education and finance, this theory aims to maximize academic outcomes efficiently.Combining these disciplines allows for more effective allocation of resources and strategic planning tailored to modern educational needs.
Application of Educational Investment Theory in CS
The application of educational investment theory in computer science involves utilizing computational methods to optimize educational budgets and resources. Here’s how it can be implemented:
- Resource Allocation Models: Use algorithms to distribute resources optimally across different educational initiatives.
- Cost-Benefit Analysis: Evaluate educational technologies based on their costs and potential educational benefits.
- Predictive Analytics: Leverage data science to forecast educational outcomes and inform investment decisions.
An educational institution decides to allocate its budget to new computer labs. Using linear programming, the institution calculates the optimal number of computers and software licenses to purchase while staying within budget constraints.
Incorporating predictive analytics can provide foresight into future educational needs, aiding proactive investment.
Predictive analytics in educational investment employs machine learning to analyze trends and predict future educational performance. It involves techniques such as logistic regression and decision trees. Logistic regression is often used to model binary outcomes, representing the probability of an event as \( P(Y=1|X) = \frac{e^{\beta_0 + \beta_1X}}{1 + e^{\beta_0 + \beta_1X}} \). This method helps in identifying key factors influencing educational results, thus enabling more strategic investments.
Understanding Financial Data Structures for Students
Understanding financial data structures is crucial for students aiming to navigate the financial aspects of computer science. These structures are fundamental for storing, manipulating, and analyzing financial information efficiently.They include various data types and structures such as arrays, linked lists, stacks, trees, and graphs—each serving a unique purpose in financial analysis. For instance, trees, specifically binary trees, are used to represent structured financial data in a hierarchical manner.
Data Structures are organized formats to store and manage data efficiently, encompassing arrays, linked lists, and more.
A binary search tree may be used to manage a database of transactions, allowing for efficient search, insertion, and deletion operations. Each node represents a transaction, and the tree's structure enables quick traversal to locate specific records.
Familiarity with different data structures enhances your ability to solve complex problems in financial applications.
Graphs are gaining popularity for modeling complex relationships in financial systems, often used in network analysis for risk assessment. Graph algorithms, like Dijkstra's for finding the shortest path, are employed to determine optimal financial routes or transactions. This algorithm iteratively selects the closest vertex, calculating distances to adjacent vertices using:\[ d(v) = \min(d(v), d(u) + w(u, v)) \]where d(v) is the distance to vertex \(v\), u is a vertex already in the subset of considered nodes, and w(u,v) is the edge weight.
investment vehicles - Key takeaways
- Investment Vehicles: Instruments like stocks, bonds, mutual funds, ETFs, and real estate that allow individuals to invest money for potential returns.
- Diversification and Risk Management: Diversified investment vehicles reduce risk compared to more concentrated investments.
- Investment Algorithms in Computer Science: Utilized for automating and optimizing investment decisions through data processing and statistical techniques.
- Computer Science Investment Methods: Techniques like fundamental and technical analysis, combined with quantitative models, for evaluating investment vehicles.
- Educational Investment Theory in CS: Applying principles from education and finance to optimize educational resources and strategies.
- Financial Data Structures for Students: Understanding of data structures like arrays, trees, and graphs to efficiently handle and analyze financial data.
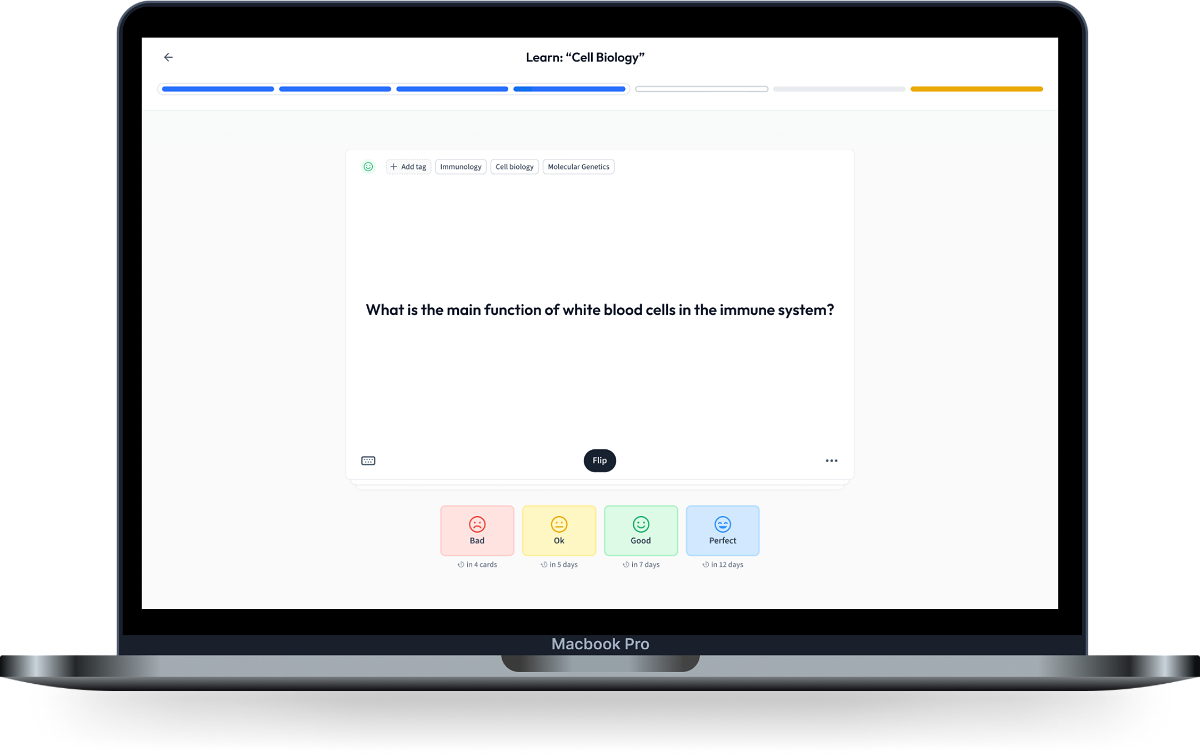
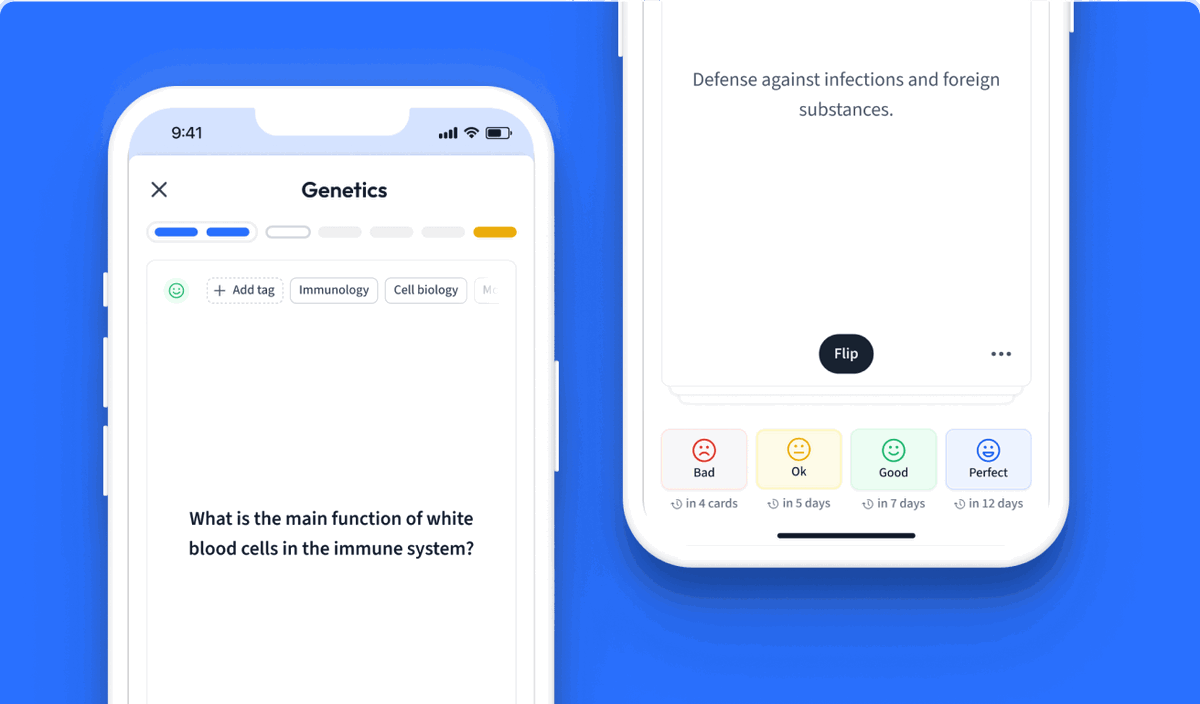
Learn with 12 investment vehicles flashcards in the free StudySmarter app
Already have an account? Log in
Frequently Asked Questions about investment vehicles
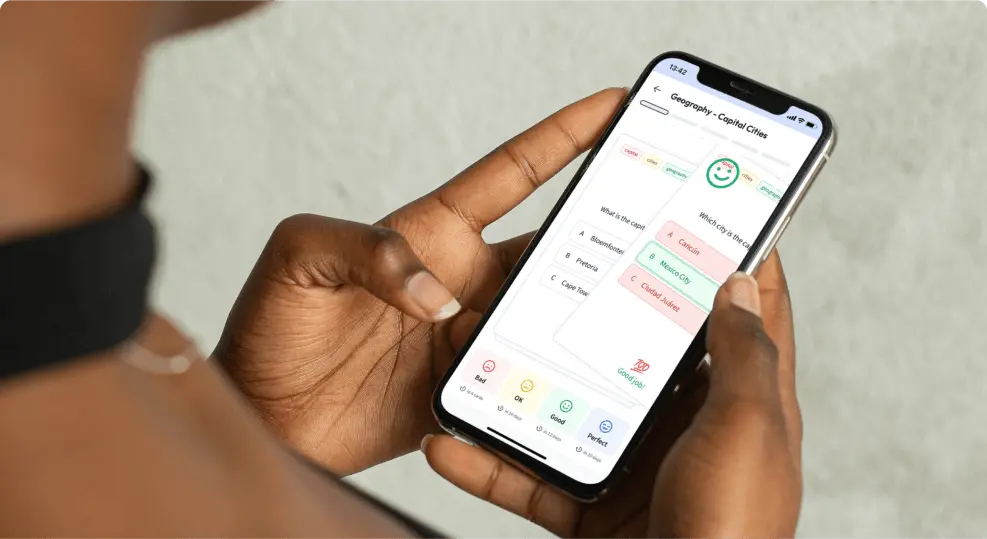
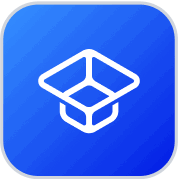
About StudySmarter
StudySmarter is a globally recognized educational technology company, offering a holistic learning platform designed for students of all ages and educational levels. Our platform provides learning support for a wide range of subjects, including STEM, Social Sciences, and Languages and also helps students to successfully master various tests and exams worldwide, such as GCSE, A Level, SAT, ACT, Abitur, and more. We offer an extensive library of learning materials, including interactive flashcards, comprehensive textbook solutions, and detailed explanations. The cutting-edge technology and tools we provide help students create their own learning materials. StudySmarter’s content is not only expert-verified but also regularly updated to ensure accuracy and relevance.
Learn more