Jump to a key chapter
Understanding E-Waste and Its Importance
E-waste, or electronic waste, is a term that you will find regularly in any discourse about modern waste management and environmental conservation. It's no longer a niche topic, given the growing reliance on technology within all aspects of our lives. From your routine household electronics to sophisticated computing facilities, E-Waste is now a crucial subject of study in computer science.
Definition: What does E-Waste Mean?
E-Waste is the term used to describe discarded electronic or electrical devices. These can also include components and materials meant for their manufacturing or repair. If not properly managed, E-Waste can have significant environmental and health impacts.
E-Waste includes a wide range of products, everything from fridges and televisions, to mobile phones, computers and their components. Major types of E-Waste, however, are small household appliances, large household appliances, IT equipment, and telecommunication equipment. They are often rich sources of precious metals like gold and copper, but also contain hazardous substances like lead and mercury.
Imagine you buy a laptop, which, after a couple of years of use, becomes sluggish, perhaps damaged, or simply outdated. Instead of repairing or upgrading it, you dispose of it and buy a new one. The disposed laptop becomes part of the E-Waste ecosystem.
Impacts: Facts about E-Waste
Around the globe, millions of tonnes of E-Waste are generated each year. The issues it presents are multifaceted, including economic, environmental, and health concerns.
According to the United Nations, in 2019, the world generated a striking 53.6 million metric tonnes of E-Waste. Only 17.4 percent of this was officially recorded as properly collected and recycled.
Notably, E-Waste also has the potential to be a valuable resource. For instance:
- It contains precious metals: a tonne of mobile phones (approximately 6000 phones) contain about 130kg of copper, 3.5kg of silver and 340g of gold.
- Recovering these metals from E-Waste can be up to 50 times more profitable than mining from the earth.
Sizes and Types: Common E-Waste Examples
In this digital age, E-Waste comes in all shapes and sizes. From large appliances to tiny mobile components, E-Waste is a broad category. Let's take a more detailed look.
Category | Examples |
---|---|
Large equipment | Washing machines, refrigerators, photocopiers |
Small equipment | Mobiles, laptops, cameras, calculators |
IT and telecommunications equipment | Computers, printers, phones, routers |
Consumer equipment and solar panels | Televisions, electrical and electronic tools, solar panels |
This just highlights the surface of the diverse forms of E-Waste. With technology permeating every corner of our lives, the range of devices that end up as E-Waste continues to expand. Addressing the E-Waste challenge hence requires a robust understanding of not just computer science concepts, but also a deeper appreciation of environmental and social considerations.
E-Waste Management: The Science Behind It All
As the digital age progresses, so too does the urgency to devise effective solutions for E-Waste management. By blending a scientific understanding of electronics with principles of sustainable practices, one can navigate the intricate landscape of E-Waste management.
Indispensable Role of Computer Science in E-Waste Management
How does computer science play a role in E-Waste management, you might wonder? The ingredients to this mix include data analysis, machine learning, and algorithm development, among other elements. Breakthroughs in these areas have significant potential to revolutionize the way you approach E-Waste management.
Data is a pivotal force in understanding and targeting E-Waste. It covers a broad spectrum, from tracking the lifecycle of electronic products to predicting future trends in E-Waste generation and recycling. By harnessing this data, you can make informed decisions and devise solid strategies for E-Waste management. Specifically, it provides policymakers, manufacturers, and recyclers with insights into the types of waste being produced, where it's coming from, and how it's being disposed of.
Data Science involves extracting knowledge or insights from various forms of data. In the case of E-Waste management, it could involve analyzing patterns and trends from product lifecycle data, E-Waste recycling rates, and more.
From there, machine learning takes it up a notch, utilizing these vast pools of data to make accurate predictions. Machine learning models could be used to predict the patterns of E-Waste generation and identify potential opportunities for effective recycling and re-use.
Ever considered how Apple's recycling robot, 'Daisy', can disassemble an iPhone within minutes to recover valuable resources? This is through machine learning algorithms. The robot can be 'trained' to recognize parts and understand how to dismantle them, improving the efficiency and effectiveness of E-Waste recycling.
Algorithm development further enhances E-Waste management. Custom-built algorithms can automate and optimize numerous processes within the E-Waste management pipeline, such as the sorting and classification of E-Waste based on criteria like the type of waste, the materials it contains, and its potential for reuse and recycling. By improving the efficiency of these processes, you save time, reduce costs, and ultimately, decrease the environmental impact of E-Waste.
Techniques in Managing Different E-Waste Types
Each type of E-Waste comes with its unique mix of components, some valuable, some hazardous. Managing these effectively requires a range of techniques, classified into three main categories: reduction, reuse, and recycle.
Reduction
Reduction focuses on decreasing the amount of E-Waste produced. This can be achieved through changes at both the production and consumption ends of the electronic product lifecycle. On the production end, designing products that are durable and easily upgradable reduces the frequency of replacement, thus reducing the amount of E-Waste generated.
On the consumer end, educating users about responsible electronic consumption and maintaining their devices to extend their life is crucial. Simple practices such as regular software updates, proper cleaning and storage, and repairing instead of replacing can collectively make a significant impact on E-Waste reduction.
Reuse
Reuse involves finding a new purpose for old or discarded electronic items instead of them ending up in landfills. A common example would be the 'second-hand' market, where used items are resold. In addition, electronic components from discarded items can be used as parts in the repair and upgrade of other devices.
A study by the United Nations University found that up to 60% of the e-waste in Europe is not recycled or collected, but likely ended up in the 'second-hand' market or stored in households.
Recycle
Recycling involves reprocessing E-Waste to extract valuable materials for use in new products, thus reducing the need for virgin resources. There are various methods to recycle E-Waste depending on the type of waste and the materials it contains. Common processes include manual dismantling, shredding and separation, and hydrometallurgical and pyrometallurgical processing.
For instance, manual dismantling involves manually dismantling parts of WEEE (waste electrical and electronic equipment) to recover valuable materials. This is then followed by mechanical separation techniques such as magnetic separation and eddy current separation that segregate ferrous and non-ferrous metals, and finally, there's hydrometallurgical processing that uses chemical leaching to further separate and recover materials.
Broadly, the management strategies for E-Waste revolve around reducing the volume of waste, reusing wherever possible, and recycling responsibly. To achieve these, advances in computer science can be instrumental, offering innovative ways to track, predict, sort, and effectively manage the E-Waste we produce.
The Connection Between E-Waste and Computer Science
When you think of computer science, your mind likely navigates towards coding or perhaps cyber security. However, computer science plays an integral role in addressing the global challenge of E-Waste management. Understanding the link between these two distinct subjects is key to envisioning and developing effective, tech-led, sustainable solutions.
Computational Approaches for Reliable E-Waste Solutions
Computer science combines the power of computation and data analytics to improve resource efficiency and waste management. It bridges the gap between the production and end-of-life stages of electronics by providing tools for monitoring, controlling, and optimizing the methodology of E-Waste disposal.
Beginning with data analytics, it forms the foundation for any computational approach. The enormous quantity of data related to E-Waste production, disposal, and recycling can be structurally analysed for patterns and trends. This data-driven approach can reveal invaluable insights to streamline E-Waste management. From tracking individual products through their lifecycle to mapping regional trends in E-Waste disposal, data analytics assists in informed decision-making in real-time.
For instance, a multinational tech company could analyse data regarding their product lifecycles. This data analytics approach could expose valuable information on the most frequently displaced parts in a laptop. Having this data allows the company to improve the design or durability of those parts, thus extending the product's lifespan and reducing generated E-Waste.
Optimisation algorithms can also be applied to improve resource allocation in E-Waste management. For instance, an algorithm could be developed to automate the process of sorting E-Waste at a recycling facility, classifying waste based on properties such as weight, size, and composition. This 'smart' method of sorting can drastically increase recycling efficiency and ensure hazardous substances are handled appropriately.
Moreover, computational modelling can be used in forecasting future E-Waste trends. Advanced models using Artificial Intelligence and Machine Learning can accurately predict future E-Waste generation based on past and present data. This predictive power can help stakeholders better prepare for and mitigate the risks associated with increasing E-Waste.
The Ellen MacArthur Foundation estimates that applying circular economy principles into global E-Waste management could generate benefits of $62 billion annually for the EU alone. These principles include designing for durability, recyclability, and upgradability, and are heavily reliant on the insights and efficiencies generated by computational approaches.
How Computer Science Can Help Reduce E-Waste
Computer science not only capacitates informed decision-making in E-Waste management but also gives rise to innovative practices for E-Waste reduction. In other words, it equips us with advanced methods to curb the generation of E-Waste at the very start.
Design is where it begins. Upgradable and modular designs extend a product's life without compromising on its functionalities. Computer-aided designs and simulations can help manufacturers create products in a way that makes them more durable, repairable and upgradable, invariably leading to a reduction in E-Waste. Such designs allow products to be updated with the latest technologies without a complete overhaul, reducing the need for new acquisitions and hence, staunching the flow of E-Waste.
Software innovations for energy and resource efficiency are another way computer science can help reduce E-Waste. Computer scientists can develop programs and applications that require less hardware capacity, leading to longer-lasting devices. They can also create software that improves a device's energy efficiency, thereby reducing the overall environmental footprint of electronic goods.
Consider a scenario where a device’s operating system becomes more energy-conscious with an update. It could intelligently regulate the battery consumption of major applications in idle state. This translates into lesser strain on hardware, thereby enhancing the device's lifespan and staving off the moment it turns into E-Waste.
In the longer term, computer science can even lead to the development of virtualisation technologies and services, which can dramatically decrease the need for physical hardware. This could mean shifting from personal computers to cloud-based systems, that utilise resources more efficiently and generate significantly less E-Waste.
Overall, by developing practices for smart design, promoting the importance of digital data, encouraging re-utilisation, and educating about responsible e-consumption, computer science can provide meaningful, sustainable strategies to reduce and manage the global E-Waste problem.
Devising Effective E-Waste Solutions
To address the mounting issues centred around E-Waste, a broad spectrum of solutions needs to be examined. Implementing E-Waste solutions not only demands understanding of the technical facets but also requires a strong grasp of social, economic, and regulatory challenges. The good news, however, resides in the scope that computer science provides to foster ground-breaking solutions.
Using Computer Science for Innovative E-Waste Solutions
Using computer science and its constantly evolving facets, we can devise and build revolutionary E-Waste management platforms, offer detailed insights for robust policy responses, develop apps and interactive media to raise awareness, and employ machine learning and AI to optimise recycling processes.
A machine-learning model refers to the mathematical model of sample data or observations, known as "training data", used for forward-looking prediction. They are used to predict the patterns of E-Waste production and disposal, leading to effective strategies for E-Waste management.
Creating E-Waste management platforms and databases can digitise operations and ensure transparency and traceability in the E-Waste management process. With smart applications that can map out E-Waste logistics, or cloud-based repositories that keep track of the E-Waste stream, stakeholders can ensure voluntary compliance with E-Waste regulations.
Data-driven and technology-led analysis also plays a crucial role in shaping policy responses. Leveraging computer science tools can provide detailed insights into various aspects of the E-Waste conundrum, such as information on quantity and type of E-Waste, existing technological, logistic and policy gaps etc. This information can inform robust policy making, be it for manufacturers, consumers, recyclers, or regulatory bodies.
By using GPS and IoT sensors, data can be generated that shows the real-time movement of specific E-Waste items. This constant and accurate monitoring supports transparency, helps identify loopholes, and aids in policy enhancements. It also helps establish accountability, making it tough for any illicit E-Waste trade to thrive.
Furthermore, computer science can be used to cultivate a community of informed consumers. Through augmented reality (AR), virtual reality (VR), or simple blogs and video tutorials, consumers can be aided with knowledge regarding correct disposal methods, nearby recycling centres, the importance of minimaling E-Waste creation, etc. The role of mass communication in moulding consumer behaviour and attitude toward E-Waste cannot be overstated.
Finally, applying machine learning and AI for sorting and recycling E-Waste can transform E-Waste recycling into a profit-making venture, beyond the apparent environmental benefits. Machine learning models can predict and sort E-Waste more accurately, while AI can optimise processes, finding the most efficient method to dismantle and extract valuable materials from E-Waste with minimum human intervention.
The economic opportunities offered by E-Waste recycling are significant. For instance, estimates suggest that the value of raw materials present in E-Waste globally reached approximately 57 billion USD in 2019, more than the Gross Domestic Product (GDP) of most countries in the world. AI and machine learning can help unlock this potential.
Case Studies: Successful E-Waste Solutions and Their Impact
Across the globe, several successful initiatives and projects leveraging the power of computer science have contributed to effective E-Waste management. Here, two examples are shared: e-Waste Management System (e-WMS) in India and BlueOak in the US.
e-Waste Management System (e-WMS) in India is an excellent case of a digitised platform for end-to-end traceability of E-Waste. With its label tracking system, the app allows real-time tracking and reporting of E-Waste from generation to disposal, the level of operations, compliance status, and more. The implementation of this system has significantly improved transparency in E-Waste handling, thus enhancing regulatory oversight while curtailing unauthorised trading and handling of E-Waste.
The e-WMS system has been very well received, recording 30,000+ metric tonnes of E-Waste processed through its registered recyclers in a single fiscal year. Its success underscores the power of digitised platforms for E-Waste management.
Another case is BlueOak in the US, which exemplifies how technological advancements can make E-Waste recycling a profitable venture. BlueOak leverages technologies such as Machine Learning and Artificial Intelligence to recover precious metals from E-Waste with a minimal environmental footprint. For instance, BlueOak's mini-refinery uses a smelting process that maximises metal recovery while reducing harmful emissions.
The impacts of these innovative E-Waste solutions are twofold. They present viable methods of handling the logistics and optimising resource recovery from E-Waste. And they also underscore the case for economic opportunities in E-Waste management. As such, these cases serve as motivational blueprints for future endeavours, emphasising that with computer science, efficient and effective E-Waste management systems can indeed be created and sustained.
According to a report by McKinsey & Company, modernising the extraction of metals from the E-Waste stream could be $10 billion industry for the United States. Companies like BlueOak stand as testament to this.
The Future of E-Waste
E-Waste presents significant environmental, economic and social challenges. Approaching these challenges requires not just remediation of the current state of affairs, but also understanding future trends. Predictive models, augmented by machine learning and artificial intelligence, will be major players in this scenario. Simultaneously, the growing recognition of E-Waste's significance is trickling into the educational landscape, mandating a fresh perspective on computer science education.
Predicting Trends: What's Next for E-Waste and Its Management
The tale of E-Waste is one of constant evolution, moulded by the developments in electronic and digital landscapes. Its growing scale, the complexity of the products involved, and shifting consumer habits are some of the factors that shape the future of E-Waste management. Accurate prediction of these trends is crucial, making the role of data science, machine learning, and AI pivotal.
Using predictive models, one can anticipate the future volume of E-Waste based on the current rate of consumption and disposal of electronic goods. For instance, based on historical data about the lifespan of devices and the rate at which they're replaced, one can predict with some certainty when these devices are likely to end up as E-Waste. Such models can be optimised using machine learning algorithms that continually improve their predictions based on newer data.
It's like forecasting the weather. Just as meteorologists use historical data on temperature, humidity, wind speed and many other variables to predict future weather conditions, these models use similar data points related to E-Waste generation and management to predict future trends.
Such predictive models can also factor in the changes in consumer behaviour and technological advancements. The increasing trend of consumers choosing to repair over replace, the growth in the second-hand electronics market, or the impact of a new product launch on the disposal rate of older devices - these are all variables that can significantly influence E-Waste generation. Therefore, having an adaptive model that can account for these changes is important.
On the flip side, predicting recycling trends can inform strategies to tap into E-Waste as a source of valuable resources. Knowing what kind of E-Waste is expected to come into recycling centres can enable them to optimise their processes and make better use of these resources.
A study by the International Telecommunication Union predicts that E-Waste volumes could grow by 21% by 2030. That’s 74.7 million tons or the weight equivalent of almost 30,000 Statues of Liberty. Insights like this highlight the importance of predictive modelling in devising future-focused E-Waste management strategies.
The Growing Importance of E-Waste in Computer Science Education
As the implications of E-Waste continue to grow, so does its importance within the realm of computer science education. Until recently, the focus has largely been on teaching students to develop technologies with little consideration of their eventual disposal. As we face the dire complexities of E-Waste, there is a growing call to incorporate sustainability into computer science curriculums, which blends the art of programming and design with principles of sustainable development and responsible consumption.
Firstly, there's a need to increase awareness about the E-Waste crisis amongst computer science students. Basic training modules on E-Waste, its consequences, and responsible design could become a fundamental part of the computer science curriculum. This understanding acts as a catalyst for students to think about the full lifecycle of products they design and develop, prompting sustainable practices.
Sustainable design in the context of computer science involves building hardware and software solutions that are resource-efficient and environmentally-friendly, taking into account all stages of the product lifecycle, from raw materials extraction through to end-of-life management.
Next, it's important to promote applied learning about E-Waste management within the computer science curriculum. This could be done through laboratory experiments or field visits, which offer hands-on experience with E-Waste recycling processes. By engaging directly with E-Waste and its impact, students can gain a deep, visceral understanding of the issue, which is likely to foster a sense of responsibility and initiative.
The incorporation of E-Waste management projects within coursework and research can also propel academic exploration in this domain. Guided by the principles of data science and machine learning, these projects can lead to the development of innovative tools for tracking, categorising, recycling, and re-using E-Waste. The accumulated knowledge can further contribute to closing the E-Waste loop and spreading awareness about its devastating effects.
An excellent example of this is how the Machine Learning for E-Waste Recyclability Prediction, a project by Massachusetts Institute of Technology students, uses computer vision techniques to identify and segregate E-Waste. Furthermore, the European project e-Waste School, which involves both teachers and students, has multiple promising activities integrating E-Waste education within the school curriculum.
Overall, bringing in E-Waste education within the computer science realm does not only better prepare future tech developers to think sustainably, but also rests on the hope that such a paradigm shift could lead to more sustainable tech behaviour at large, potentially changing the E-Waste narrative for the better.
E-Waste - Key takeaways
E-Waste refers to discarded electronic or electrical devices or materials meant for their manufacturing or repair.
Major types of E-Waste include small household appliances, large household appliances, IT equipment, and telecommunication equipment.
The world generated 53.6 million metric tonnes of E-Waste in 2019, but only 17.4 percent was officially recorded as properly collected and recycled.
Computer Science plays an important role in E-Waste management, including data analysis, machine learning, and algorithm development.
E-Waste management techniques involve reduction, reuse, and recycle. Each technique requires understanding the unique mix of components in different types of E-Waste.
Learn faster with the 15 flashcards about E Waste
Sign up for free to gain access to all our flashcards.
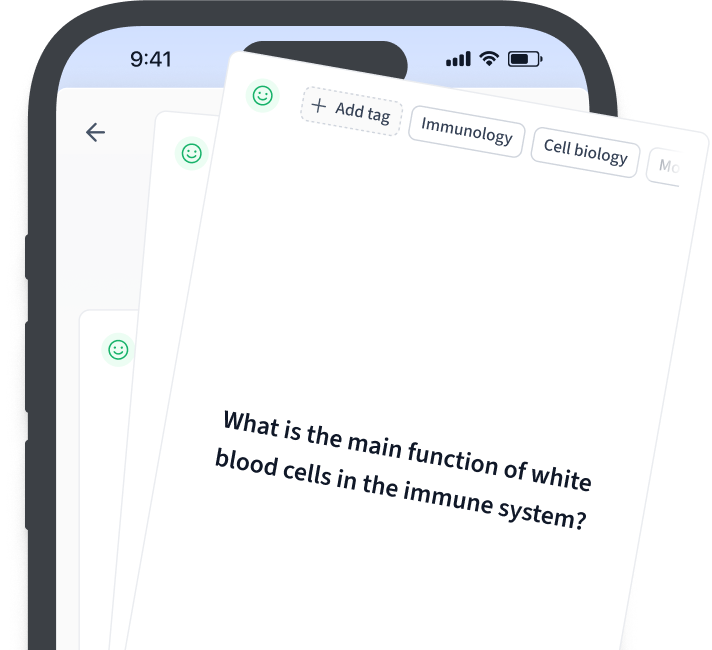
Frequently Asked Questions about E Waste
What is e waste?
How to reduce e waste?
Why is e waste a problem?
What is an example of e waste?
How much e waste is produced each year?
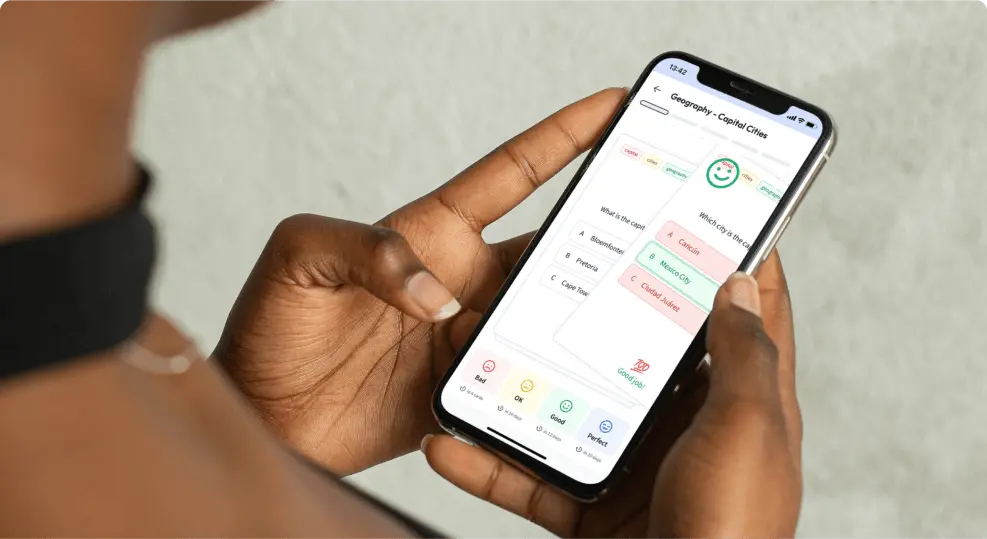
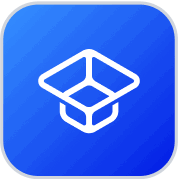
About StudySmarter
StudySmarter is a globally recognized educational technology company, offering a holistic learning platform designed for students of all ages and educational levels. Our platform provides learning support for a wide range of subjects, including STEM, Social Sciences, and Languages and also helps students to successfully master various tests and exams worldwide, such as GCSE, A Level, SAT, ACT, Abitur, and more. We offer an extensive library of learning materials, including interactive flashcards, comprehensive textbook solutions, and detailed explanations. The cutting-edge technology and tools we provide help students create their own learning materials. StudySmarter’s content is not only expert-verified but also regularly updated to ensure accuracy and relevance.
Learn more