Jump to a key chapter
Introduction to Turbulence Modelling for CFD
Turbulence modelling for Computational Fluid Dynamics (CFD) plays a pivotal role in simulating and predicting fluid behaviour in various engineering and scientific applications. This overview aims to introduce you to the fundamental concepts and components essential for understanding and implementing turbulence models within CFD studies.
Understanding the Basics of Computational Fluid Dynamics and Turbulence Modelling
Computational Fluid Dynamics (CFD) is a branch of fluid mechanics that uses numerical analysis and data structures to solve and analyse problems involving fluid flows. Computers are used to simulate the interaction of liquids and gases with surfaces defined by boundary conditions. Turbulence modelling is a critical aspect of CFD that deals with the simulation of the random, chaotic motions of fluid particles which are not well understood or easily predictable.
Turbulence refers to the irregular, chaotic movement of fluid particles, in contrast to a laminar flow where the fluid moves in smooth layers. Turbulence is common in most real-world fluid flows, including the flow of air over an aircraft wing or water flowing in a pipe.
Example: In predicting the weather, turbulence models are used in the computational simulation to understand how the different layers of air interact with each other, leading to different weather patterns.
Highly accurate turbulence models are essential for the accurate prediction of flows in engineering applications, from aerodynamics to hydrodynamics.
Essential Components of Turbulence Modelling in Fluid Mechanics
The complexity of turbulence in fluid mechanics requires a robust approach to modelling. Several key components are fundamental in creating realistic simulations of turbulent flows. Understanding these components helps in selecting the appropriate model for a given application.
- Reynolds Averaged Navier-Stokes (RANS) Equations: A set of equations that are used to describe the motion of fluid. They average the turbulence effects over time, providing a mean flow prediction.
- Large Eddy Simulation (LES): A modelling approach that simulates large-scale eddies in turbulent flow while modelling the effects of smaller scales.
- Direct Numerical Simulation (DNS): A comprehensive approach that calculates all scales of turbulence directly without any modelling, requiring significant computational resources.
Example: An automotive engineer uses RANS equations to predict the aerodynamic drag on a new car design. This helps in optimising the shape for better fuel efficiency.
While the DNS method offers the most detailed and accurate representation of turbulent flows, its high computational cost makes it impractical for most engineering applications. LES strikes a balance, offering detailed insights into large-scale turbulence structures with a manageable computational demand. RANS, being the most computationally efficient, is widely used for industrial applications despite its less detailed nature. The decision on which turbulence model to use heavily depends on the specific requirements of the simulation, including the level of detail required and available computational resources.
Turbulence Model K-Epsilon Explained
The K-Epsilon model represents one of the most commonly used approaches in turbulence modelling within the realm of Computational Fluid Dynamics (CFD). It is renowned for its versatility and reliability in simulating turbulent flows in various engineering disciplines, notably aerospace engineering. This model, grounded on the Reynolds-Averaged Navier-Stokes (RANS) equations, significantly simplifies the complexities of turbulent flow, making it an indispensable tool in the analysis and design of engineering systems.
The Core Principles of the K-Epsilon Turbulence Model
The K-Epsilon model operates on two primary equations that govern turbulent kinetic energy (k) and its rate of dissipation (ε). These equations provide a mathematical framework for predicting the behaviour of turbulent flows, thus facilitating a deeper understanding of fluid dynamics in various applications. The model's efficacy lies in its ability to adapt to a wide range of flow conditions, making it a staple in turbulence modelling for engineers and researchers.
Kinetic Energy (k): Represents the energy stored in turbulence, which manifests as velocity fluctuations within the fluid.Rate of Dissipation (ε): Measures how quickly turbulent kinetic energy is transformed into thermal energy due to viscosity.
Example: When simulating the airflow over an aircraft wing, the K-Epsilon model uses the values of k and ε to predict how the turbulent air will interact with the wing surface, crucial for aerodynamic design and analysis.
The K-Epsilon model is particularly effective in 'fully developed' turbulent flow scenarios, such as flow in pipes or around bluff bodies, where the turbulence is well-established and follows a predictable pattern.
Applying K-Epsilon in Aerospace Engineering
In aerospace engineering, the application of the K-Epsilon turbulence model extends across a myriad of scenarios, from the design of aircraft wings to the assessment of jet propulsion systems. Its ability to accurately predict turbulent flows around complex shapes and under a variety of operating conditions makes it an essential tool for aerospace engineers aiming to optimise performance and efficiency while ensuring safety and compliance with regulatory standards.
Example: An aerospace engineer may use the K-Epsilon model to simulate the turbulent wake behind an aircraft. This information is crucial for determining the best wing shape for reducing drag and improving fuel efficiency.
A remarkable application of the K-Epsilon model in aerospace engineering is its use in predicting the behaviour of boundary layers over aircraft surfaces and the onset of turbulence. This prediction is vital for estimating skin friction drag, which directly affects the aircraft's performance. By using the K-Epsilon model, engineers can make informed decisions about surface treatments or modifications to minimise drag, thereby enhancing the aircraft's aerodynamic efficiency without compromising its structural integrity.
Due to its computational efficiency compared to more complex models, the K-Epsilon model is particularly well-suited for the iterative design processes prevalent in aerospace engineering, where multiple simulations are conducted to refine designs.
K Omega Turbulence Model in Detail
Overview of K Omega Model for Turbulence Studies
The K Omega model is renowned for its accuracy in simulating turbulence, particularly near walls, which is critical in engineering applications. It incorporates two transport equations: one for the turbulent kinetic energy (K) and another for the specific rate of dissipation (Ω). This dual-equation approach enables the model to more accurately predict the effects of turbulence across a broad range of flows compared to simpler models.The success of the K Omega model lies in its ability to handle both the inner and outer regions of turbulent boundary layers effectively, making it an indispensable tool for engineers and researchers seeking to optimize designs and processes.
- Turbulent Kinetic Energy (K): This component represents the energy contained in the turbulence, necessary for its sustenance.
- Specific Rate of Dissipation (Ω): It measures the rate at which turbulent kinetic energy is converted into thermal energy, dissipating the turbulence.
Example: In the design of an aircraft wing, the K Omega model would be utilised to simulate airflow around the wing. It would help identify areas of high turbulence that might affect the aircraft's lift and drag, enabling designers to modify the wing shape for optimal performance.
One of the key strengths of the K Omega model is its robust performance in predicting flows with adverse pressure gradients, which are common in many engineering situations, such as airflow over wings.
The Role of K Omega Model in Applied Computational Fluid Dynamics
In Applied CFD, the K Omega model serves a crucial function, accurately predicting turbulent flows around complex geometries and in conditions where the flow transitions from laminar to turbulent. Its adaptability and precision make it a preferred choice for simulations in aerospace, automotive, marine, and environmental engineering.By providing detailed insights into the nature and distribution of turbulence, the model helps engineers to enhance the aerodynamic performance of vehicles, improve the efficiency of energy systems, and mitigate environmental impacts. The K Omega model also plays a pivotal role in research, aiding the development of more efficient and innovative solutions to challenging fluid dynamics problems.
An important application of the K Omega model in CFD is its use in the design of more efficient combustion systems for aerospace engines. By accurately simulating the turbulent mixing of fuel and air, engineers can optimise combustion processes to increase thrust while reducing emissions. Similarly, in wind turbine design, the model's predictions can lead to designs that extract more energy from prevailing wind conditions, significantly enhancing energy capture efficiency.These applications underscore the model's importance not only in advancing technological capabilities but also in contributing to sustainable development goals by enabling the design of more efficient systems with lower environmental footprints.
Despite its complexity, the K Omega model's adaptability to various boundary conditions and flow types makes it a valuable tool in the CFD analyst's repertoire, facilitating the accurate simulation of turbulent flows in a wide range of practical applications.
A One Equation Turbulence Model for Aerodynamic Flows
The one equation turbulence model simplifies the complex phenomena of turbulent aerodynamic flows into a manageable formula, facilitating its study and application in Computational Fluid Dynamics (CFD). This model, focusing on a singular transport equation for a specific turbulence variable, marks a foundational approach for beginners and streamlined applications where computational efficiency is paramount.Its implementation in CFD simulations offers a balance between accuracy and computational demand, making it an attractive option for initial design assessments and educational purposes.
Introduction to the One Equation Turbulence Model
The one equation turbulence model typically revolves around the transport equation for turbulent kinetic energy (k). It calculates turbulent viscosity by combining this kinetic energy with a scale of length derived from the flow domain's geometry. This simplified approach abstracts the complexities of turbulent flows into a singular variable, reducing the computational load while providing insight into the turbulent characteristics of the flow. Formula for Turbulent Kinetic Energy (k): \[k = \frac{1}{2}(u'^2 + v'^2 + w'^2)\], where \(u'\), \(v'\), and \(w'\) represent the velocity fluctuations in three orthogonal directions.
The one equation model is especially useful in situations where computational resources are limited or a quick estimation of flow characteristics is needed.
How the One Equation Model Enhances Turbulence Modelling for CFD
Despite its simplifications, the one equation turbulence model enhances CFD simulations by providing a foundational understanding of turbulent flows. It allows for the efficient prediction of turbulent boundary layers, which is critical in many aerodynamic applications such as the design and analysis of aircraft wings and wind turbines.By focusing on turbulent kinetic energy, the model leverages a key aspect of turbulence to inform about energy distribution and dissipation within the flow. This insight is crucial for estimating drag and lift forces, which directly impact the performance and efficiency of aerodynamic designs.
Example: An aerospace engineer employs the one equation model to quickly evaluate the effects of modifications to an aircraft's wing design on its aerodynamic performance. By simulating the flow around the wing, the model helps identify areas where adjustments can reduce turbulent drag and improve fuel efficiency.
One fascinating application of the one equation turbulence model is in the design of high-performance sports equipment, such as cycling helmets. Engineers use the model to predict how modifications to the helmet’s shape can minimise air resistance, thereby enhancing an athlete's performance. The model's ability to quickly provide insights into turbulent flow behaviour without extensive computational demands makes it invaluable for iterative design processes, where multiple variations are assessed to find the optimal configuration.Additionally, the one equation model serves as an educational tool, introducing students to turbulence modelling concepts. By simplifying the mathematical complexity, it allows learners to grasp the fundamental principles of turbulence and its impact on fluid flows, laying the groundwork for more advanced studies in fluid dynamics.
Turbulence Modeling - Key takeaways
- Turbulence Modelling: A crucial part of Computational Fluid Dynamics (CFD) used to simulate the random, chaotic motions of fluid particles.
- Reynolds Averaged Navier-Stokes (RANS) Equations: Average the turbulence effects over time to provide mean flow predictions, commonly used in turbulence modelling for CFD.
- K-Epsilon Turbulence Model: A widely used turbulence model based on RANS equations, involving two equations for turbulent kinetic energy (k) and its dissipation rate (ε), particularly effective in predicting 'fully developed' turbulent flows.
- K Omega Turbulence Model: Incorporates two transport equations for turbulent kinetic energy (K) and specific rate of dissipation (Ω), known for its performance in predicting near-wall turbulence.
- A One Equation Turbulence Model: Simplifies turbulence modelling by focusing on a single transport equation, usually for turbulent kinetic energy (k), balancing accuracy and computational efficiency.
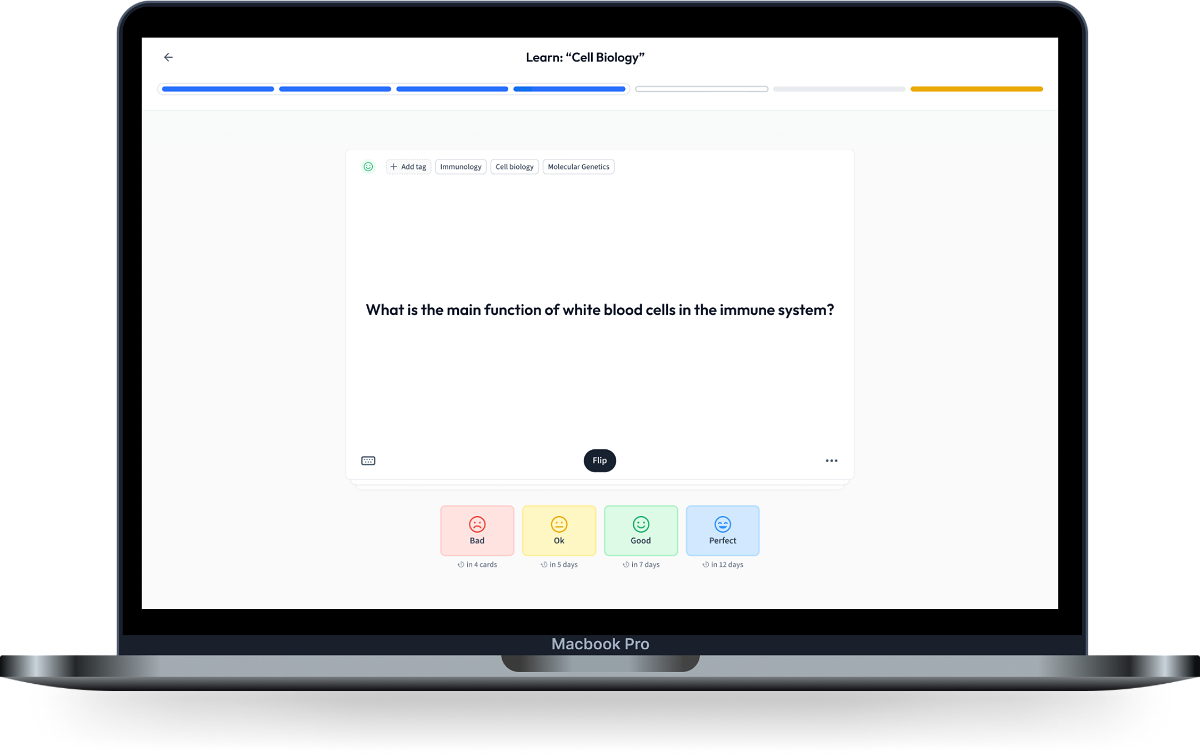
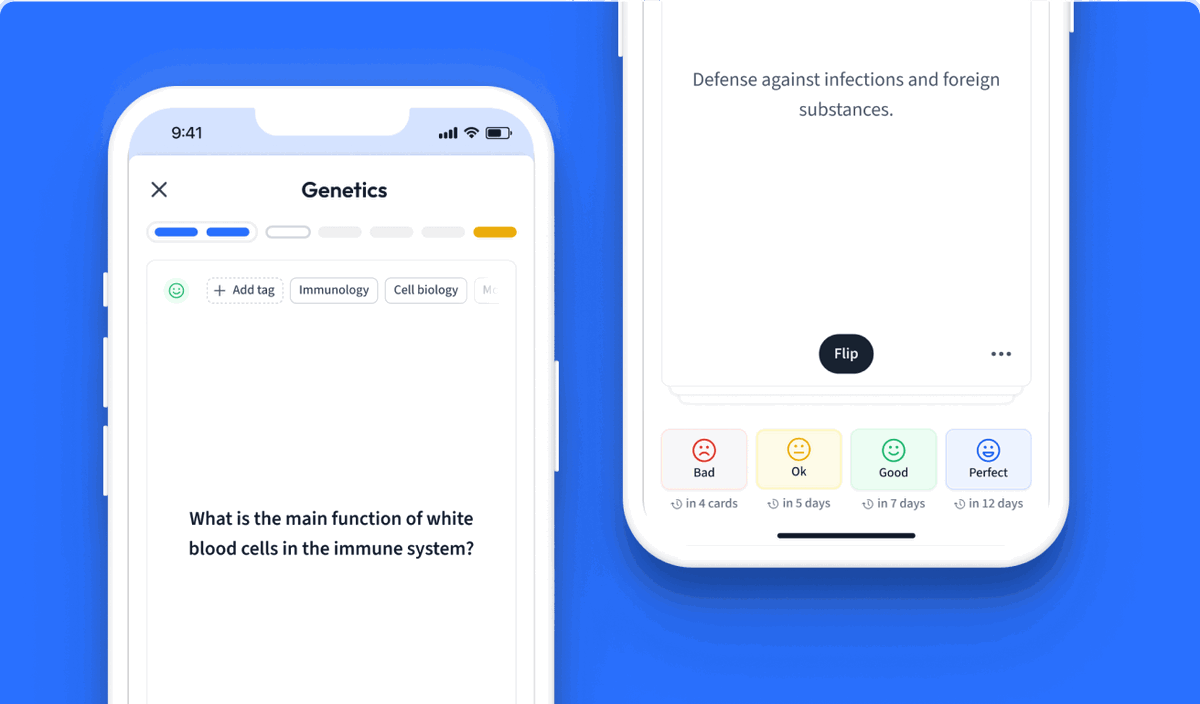
Learn with 12 Turbulence Modeling flashcards in the free StudySmarter app
We have 14,000 flashcards about Dynamic Landscapes.
Already have an account? Log in
Frequently Asked Questions about Turbulence Modeling
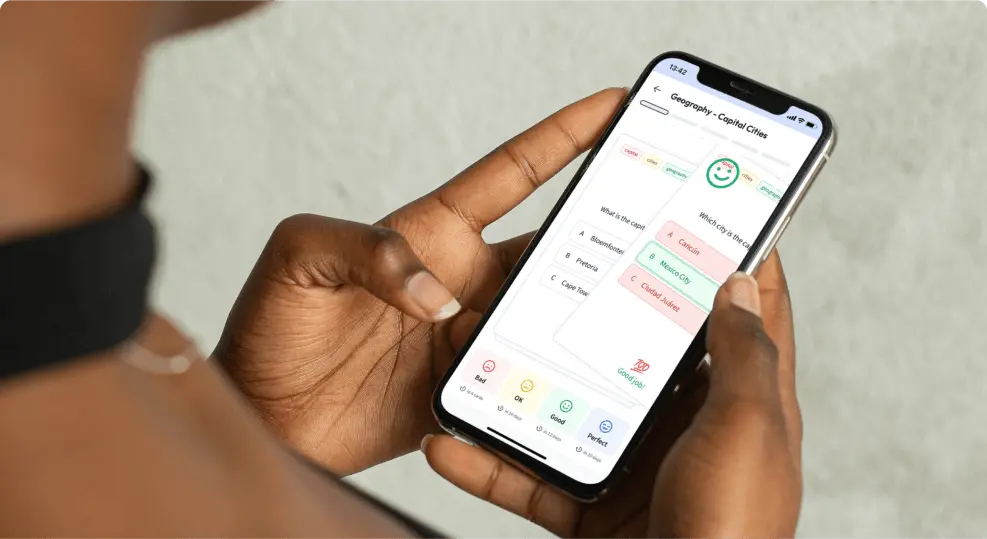
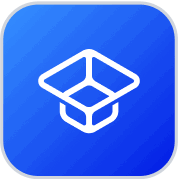
About StudySmarter
StudySmarter is a globally recognized educational technology company, offering a holistic learning platform designed for students of all ages and educational levels. Our platform provides learning support for a wide range of subjects, including STEM, Social Sciences, and Languages and also helps students to successfully master various tests and exams worldwide, such as GCSE, A Level, SAT, ACT, Abitur, and more. We offer an extensive library of learning materials, including interactive flashcards, comprehensive textbook solutions, and detailed explanations. The cutting-edge technology and tools we provide help students create their own learning materials. StudySmarter’s content is not only expert-verified but also regularly updated to ensure accuracy and relevance.
Learn more