Jump to a key chapter
AI Safety Definition
AI Safety refers to the efforts to ensure that Artificial Intelligence systems operate reliably, ethically, and do not pose harm to humans. Understanding AI safety is vital as AI systems continue to integrate into everyday life.
What is AI Safety?
AI Safety encompasses a range of practices and research focused on creating and maintaining AI systems that behave in ways that are predictable and beneficial. Key aspects include:
- Reliability: Ensuring AI operates as expected under various conditions.
- Ethical Standards: Aligning AI behavior with human values and ethics.
- Risk Management: Identifying and mitigating potential harm from AI misuse.
Reliability: The capacity of an AI system to perform consistently and accurately in a variety of environments.
A key area of AI Safety is developing robust AI systems. These systems are designed to handle real-world situations without malfunctioning or producing unexpected results. This includes studying failure modes—ways in which AI can fail—and designing safeguards.
AI Safety Explained
AI safety involves methodologies to prevent AI systems from developing unintended behaviors. This includes:
- Algorithmic Transparency: Making AI decision-making processes understandable.
- Security Measures: Protecting AI from adversarial attacks that manipulate outputs.
- Human Supervision: Keeping human oversight active in critical AI applications.
Concept | Explanation |
Algorithmic Transparency | Understanding the 'why' behind AI decisions. |
Security Measures | Techniques to defend AI systems against threats. |
For instance, a self-driving car equipped with AI must adhere to AI safety principles. It requires:
- Reliable Sensors: To detect obstacles accurately.
- Ethical Decision-Making: To prioritize human safety in split-second choices.
- Continuous Monitoring: To override AI decisions when necessary.
AI Safety is crucial as AI becomes more advanced and autonomous, affecting critical areas such as healthcare, transportation, and finance.
AI Safety Techniques
Ensuring the safe deployment of AI systems involves a comprehensive understanding of various AI safety techniques. These techniques are applied to minimize risks and enhance the reliability of AI applications.
Key AI Safety Techniques
Several key techniques ensure the safety of AI implementations. These include:
- Robustness Testing: Stress testing AI models to perform under diverse and challenging conditions.
- Fail-Safe Mechanisms: Implementing systems that can revert to a safe state in case of unexpected behavior.
- Human-in-the-Loop: Maintaining human oversight in AI decision-making processes to ensure ethical outcomes.
Each technique plays a critical role in mitigating risks and ensuring AI systems are secure and reliable.
Robustness Testing: A method for evaluating an AI system's capability to cope with various conditions and inputs effectively.
Consider an AI used in financial trading platforms. To ensure its safety, the AI undergoes:
- Market Simulation: Testing in simulated environments with variable conditions to predict and handle extreme market fluctuations.
- Error Detection: Algorithms to identify and correct potential transaction errors quickly.
Human-in-the-Loop (HITL) is a vital technique in critical applications like healthcare. In HITL, humans continuously monitor AI systems, allowing for interventions and adjustments to be made in real-time. This technique offers a balance between automation and ethical decision-making.
Implementing AI Safety Techniques
Practical implementation of AI safety techniques involves careful planning and execution. The primary steps include:
- Assessment: Evaluating the scope and potential risks associated with AI applications.
- Integration: Employing techniques such as fail-safe mechanisms and robustness testing.
- Training and Development: Educating AI developers and users about safety protocols.
- Monitoring and Review: Continuous observation of AI behavior and periodic reviews to ensure compliance with safety standards.
Technique | Purpose |
Robustness Testing | Ensure system stability and handling of diverse inputs. |
Human-in-the-Loop | Maintain ethical oversight. |
Regular updates and maintenance are crucial for AI safety as technology and environments evolve continuously.
Importance of AI Safety
As Artificial Intelligence becomes increasingly integrated into critical aspects of society, understanding the importance of AI safety grows more crucial. The aim is to harness AI's capabilities while minimizing potential risks.
Why AI Safety Matters
AI safety matters for several reasons:
- Human Well-being: Ensuring AI applications do not cause harm to humans.
- Trust and Reliability: Building confidence in AI systems through predictable and safe performance.
- Economic Stability: Maintaining market stability in AI-driven applications like finance and retail.
- Ethical Responsibility: Aligning AI operations with human ethics and laws.
The deployment of AI technology across industries highlights the need for safety protocols to ensure positive outcomes.
Human Well-being: The state of being comfortable, healthy, or happy; ensuring AI supports this for all individuals.
Consider an AI system used for medical diagnoses. AI safety is crucial because:
- It must provide accurate diagnostics to avoid misinformation.
- It requires oversight to allow healthcare professionals to confirm AI findings.
- AI's integration needs strict compliance with medical regulations to ensure patient safety.
When AI fails to operate safely, it might cause disruptions that range from minor inconveniences to major calamities.
Impact of AI Safety on Society
AI safety has far-reaching impacts on society:
- Cultural Influence: The perception of AI's role in society changes with its safety and reliability.
- Technological Progress: Safe practices encourage innovation and adoption of AI technologies.
- Social Practice: AI systems supplement and enhance human activities when verified safe.
Aspect | Impact |
Cultural Influence | Increases society's acceptance of AI marvels. |
Technological Progress | Promotes safe and innovative AI development. |
A profound societal impact of AI safety comes in the form of employment transformation. As AI systems enhance or replace certain jobs, ensuring these systems follow stringent safety protocols helps minimize adverse effects on employment, providing a secure transition for affected workers.
AI Safety Risks
Understanding AI safety risks is essential in anticipating and preventing potential adverse outcomes associated with AI technology. These risks can arise from various aspects of AI systems and their interactions with human environments.
Common AI Safety Risks
Common safety risks in AI stem from several factors:
- Algorithm Bias: AI systems can inadvertently learn biased patterns from data, leading to unfair outcomes.
- Data Privacy: The collection and use of data by AI systems can compromise individual privacy.
- Autonomous Decision Making: Fully autonomous AI systems might make decisions without human context or ethics.
These risks pose significant challenges to developers and policymakers in ensuring safe AI deployment.
Algorithm Bias: The presence of systematic and unfair discrimination in AI algorithms that arises from training data or model assumptions.
For example, if an AI system is used in hiring processes, biased algorithms might favor candidates of a certain demographic unintentionally. This can happen if the training data reflects historical biases.
Regular audits of AI systems can help identify and mitigate biases early in development.
Autonomous Decision Making in critical fields like medicine or transportation requires stringent safety protocols. Unsupervised AI can theoretically lead to decisions that are efficient but lack human empathy or justification, potentially causing ethical dilemmas or safety hazards.
Mitigating AI Safety Risks
Several strategies can effectively mitigate AI safety risks:
- Bias Detection and Correction: Implementing checks to identify and eliminate bias from AI systems.
- Strong Data Encryption: Protecting data to prevent breaches and ensure privacy.
- Ethical Guidelines: Establishing clear ethical guidelines for AI to follow, especially in autonomous systems.
Strategy | Purpose |
Bias Detection | Recognize and adjust unfair biases in AI outputs. |
Data Encryption | Ensure the privacy and security of sensitive user data. |
In finance, AI systems could utilize:
- Fairness Assessment Tools to continuously check algorithms for bias.
- Advanced Cryptography to protect transaction data against cyber attacks.
AI safety guidelines should evolve with technology, considering new applications and potential risks.
Building AI safety frameworks often involves interdisciplinary approaches, combining insights from technology, ethics, and law. These frameworks guide not only AI developers but also policymakers as they create legislation balancing innovation with public safety and ethics.
ai safety - Key takeaways
- AI Safety Definition: Ensures AI operates reliably, ethically, without harming humans.
- AI Safety Techniques: Include robustness testing, fail-safe mechanisms, and human-in-the-loop oversight.
- Importance of AI Safety: Essential for human well-being, trust, economic stability, and ethical responsibility in AI applications.
- AI Safety Explained: Prevents unintended AI behaviors with transparency, security measures, and human supervision.
- AI Safety Risks: Include algorithm bias, data privacy concerns, and risks from autonomous decision-making.
- Mitigating AI Safety Risks: Involves bias detection, data encryption, and following ethical guidelines.
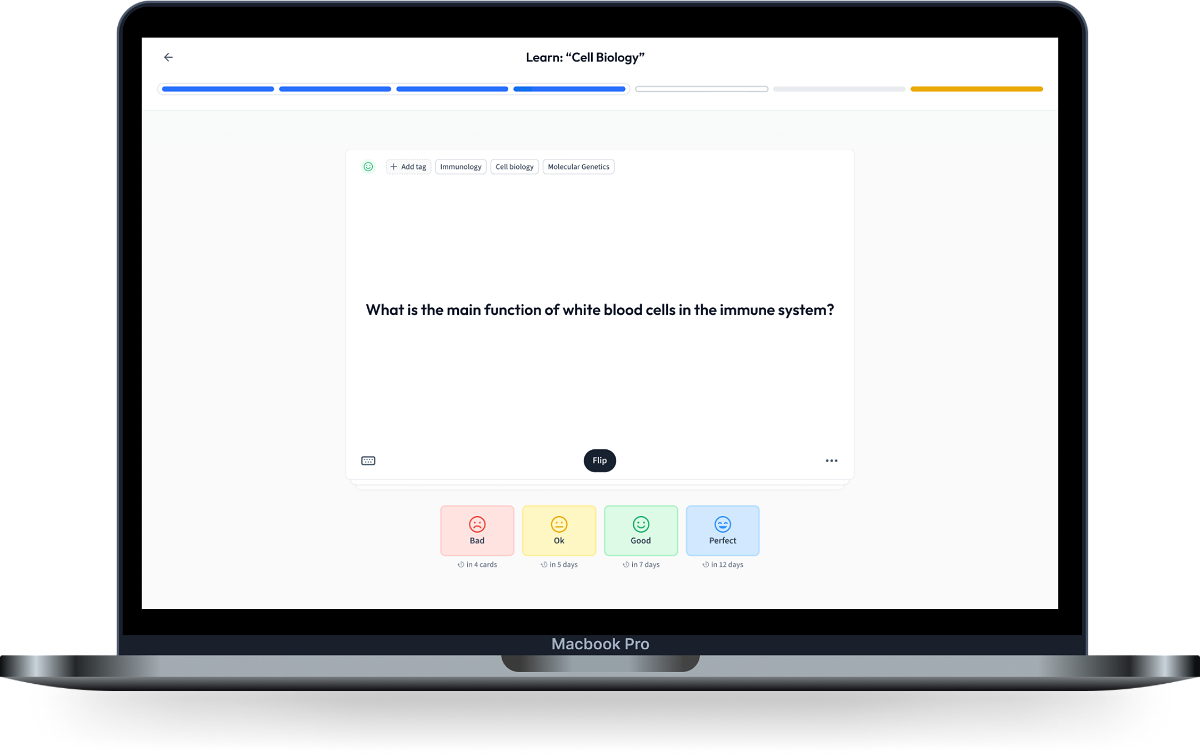
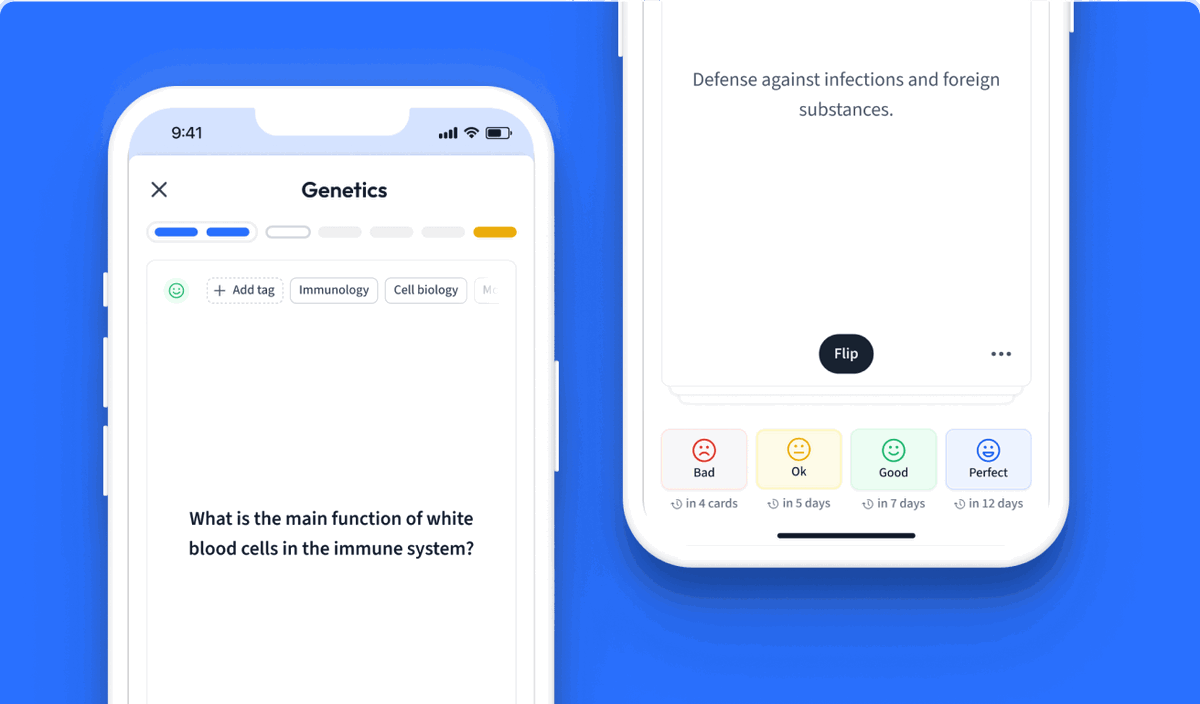
Learn with 12 ai safety flashcards in the free StudySmarter app
We have 14,000 flashcards about Dynamic Landscapes.
Already have an account? Log in
Frequently Asked Questions about ai safety
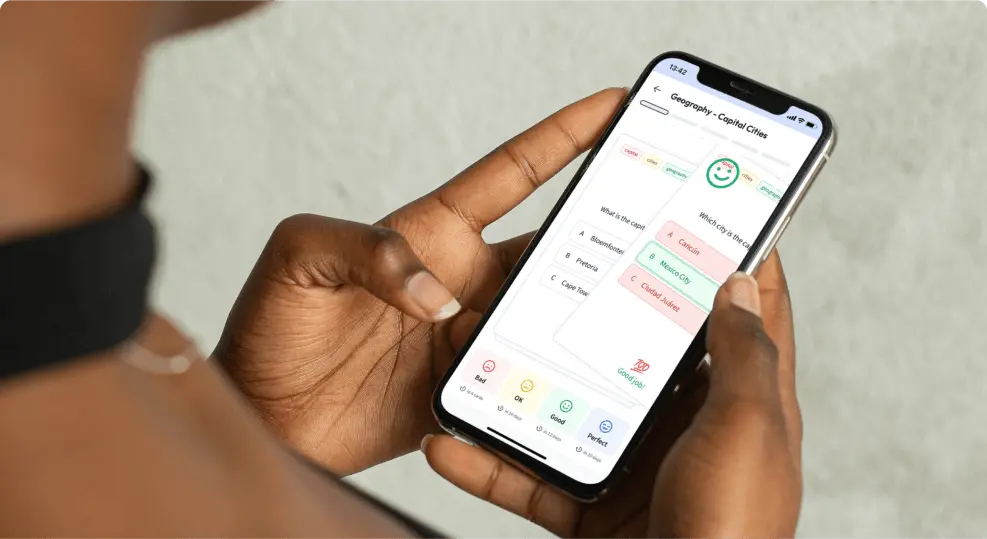
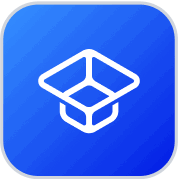
About StudySmarter
StudySmarter is a globally recognized educational technology company, offering a holistic learning platform designed for students of all ages and educational levels. Our platform provides learning support for a wide range of subjects, including STEM, Social Sciences, and Languages and also helps students to successfully master various tests and exams worldwide, such as GCSE, A Level, SAT, ACT, Abitur, and more. We offer an extensive library of learning materials, including interactive flashcards, comprehensive textbook solutions, and detailed explanations. The cutting-edge technology and tools we provide help students create their own learning materials. StudySmarter’s content is not only expert-verified but also regularly updated to ensure accuracy and relevance.
Learn more