Jump to a key chapter
Autonomous Decision Making Definition
Autonomous decision making refers to the capability of a system to make decisions independently, usually without human intervention, by utilizing complex algorithms and artificial intelligence. This process mimics human decision-making by analyzing data, learning from experiences, and even predicting potential outcomes.
Autonomous Decision Making Explained
Autonomous decision making plays a crucial role in modern technology, particularly in systems requiring speedy and accurate decision capabilities, such as self-driving cars, robotic systems, and smart grids. These systems rely on a combination of sensors, data processing, machine learning, and algorithms to interpret and respond to real-time environments effectively.
Machine Learning: A subset of artificial intelligence where systems learn and improve from experience without being explicitly programmed, often used in autonomous decision-making systems.
The process involved in autonomous decision making typically comprises the following steps:
- Data Collection: Gathering input from various sensors and sources.
- Data Processing: Analyzing the collected data using algorithms.
- Decision Making: Selecting the best option based on analysis.
- Actuation: Implementing the decision with minimal time delay.
Consider a self-driving car approaching a crosswalk:1. Data Collection: The car uses cameras and sensors to detect pedestrians near the crosswalk.2. Data Processing: Algorithms analyze the speed and distance of the pedestrians.3. Decision Making: The system decides to slow down and stop to allow pedestrians to cross.4. Actuation: The car applies the brakes gently to come to a stop.
Autonomous systems are increasingly being developed in sectors like healthcare and agriculture, offering substantial advancements in service delivery and productivity.
Exploring further into autonomous decision making reveals the intricacy involved in moral and ethical decision-making. For example, autonomous vehicles face complex scenarios where decisions are not just about efficiency but also encompass ethical considerations. Instances such as deciding between braking or swerving to avoid an accident require deep ethical programming. This is often referred to as the 'trolley problem,' highlighting the moral dilemma where every decision has potential consequences, and the system needs to choose the lesser evil. Engineers and philosophers work together to equip autonomous systems with guidelines that ethically align with human values.
Techniques in Autonomous Decision Making
Techniques in autonomous decision making are crucial in enabling machines to learn, adapt, and operate without human intervention. These techniques combine advanced algorithms and machine learning models to ensure decisions are made optimally and efficiently.
Machine Learning in Autonomous Decision Making
Machine learning is a vital component of autonomous decision making, enabling machines to improve their decision-making capabilities over time. This process involves using algorithms to parse data, learn from it, and make actionable decisions without explicit programming.There are several types of machine learning that can be used, including:
Supervised Learning: A machine learning technique where the system learns from a labeled dataset, allowing predictions or classifications based on new data inputs.
- Unsupervised Learning: The system explores input data without labeled responses to detect patterns and structures.
- Reinforcement Learning: The system learns to make decisions by receiving rewards or penalties based on actions, ideal for systems requiring continuous decision-making like autonomous vehicles.
Consider a self-driving car using reinforcement learning:1. Environment: Roads with various obstacles and traffic signals.2. Actions: Accelerate, decelerate, or turn.3. Rewards: Successfully navigating a route without accidents.4. Penalties: Collisions or traffic violations.
To further clarify using math, consider a basic decision model for a machine learning algorithm. Given datasets, a model can be denoted as:\[f(x) = \text{decision}(x)\]where \(x\) is the input data, often represented by features such as road conditions.
As machine learning models continue to evolve, they increasingly outperform traditional decision-making models, especially in complex situations.
Neural Networks and Autonomous Decision Making
Neural networks mimic the human brain's architecture, playing a crucial role in autonomous decision-making systems. These networks consist of interconnected neurons (or nodes) that process data to identify patterns or make decisions.The primary advantages of neural networks in decision making include:
Deep Learning: A subset of neural networks with multiple layers that allow the model to learn complex patterns and features, often used in tasks such as image and speech recognition.
- Ability to handle large volumes of data and complex computations.
- Capability to generalize across different tasks once trained appropriately.
- Improved accuracy and performance compared to simpler algorithms.
For example, in facial recognition software:1. Input Layer: Raw pixel data from an image.2. Hidden Layers: Layers that extract features such as edges and shapes.3. Output Layer: Probabilities of the image matching different pre-learned faces.
A fascinating aspect of neural networks is the use of convolutional neural networks (CNNs) in image processing for autonomous systems. CNNs have been instrumental in advancements in autonomous drones and vehicle operations by allowing real-time processing and decision-making. They utilize convolutional layers to automatically and adaptively learn spatial hierarchies of features from low- to high-level patterns.Consider the math behind a basic neural network model:\[y = f(Wx + b)\]where \(y\) is the output, \(W\) are the weights, \(x\) is the input, and \(b\) is the bias. Each layer running computations creates a decision map for the input data, enabling real-time, autonomous decision making.
Autonomous Decision Making Process
The autonomous decision making process involves a system making choices independently, often in complex or unpredictable environments. These systems utilize advanced technologies like artificial intelligence and machine learning to interpret data and make decisions without human oversight.
Steps in Autonomous Decision Making
Autonomous decision making generally follows a structured process composed of several key steps:1. Data Collection: This involves gathering relevant data through sensors and external sources. For instance, a self-driving car collects data using cameras and LIDAR.2. Data Processing: The collected data is then processed using algorithms that interpret and analyze it. This step may involve employing machine learning models which adjust and improve over time.3. Decision Making: At this stage, the system makes a choice based on processed data, often by evaluating possible outcomes and employing predictive models.4. Actuation: Finally, the decision is implemented, which could mean adjusting speed, direction, or another action essential to the system’s operation.
In a smart thermostat system:
- Data Collection: Sensors measure indoor temperature and user preferences.
- Data Processing: The system analyzes temperature variations and external weather forecasts.
- Decision Making: It decides when to turn the heating on or off.
- Actuation: Adjusts the thermostat settings automatically.
Always consider that noise in data collection can affect accuracy in decision-making, necessitating robust data processing and handling mechanisms.
Challenges in the Autonomous Decision Making Process
Although autonomous systems boast impressive capabilities, they also face several challenges:1. Data Noise: Inaccuracies and inconsistencies in data can lead to faulty decisions. For example, fog affecting a camera's ability to capture clear images.2. Dynamic Environments: Rapid changes in an environment might require continuous learning or real-time decision recalibration to ensure accuracy and safety.3. Ethical Considerations: Autonomous decision-making systems must align with human values, especially when faced with moral dilemmas (e.g., choosing actions in accident scenarios).4. Security Risks: Systems are prone to cyber-attacks that may manipulate data inputs, leading to erroneous decisions.
In the realm of autonomous decision making, concepts like moral agency introduce philosophical inquiries into the accountability and ethics of machine systems. For example, if a drone carrying deliveries encounters a potential collision situation, should it prioritize cargo protection, or safety of individuals? Engineers must program these systems to simulate humane decision-making procedures, ensuring algorithmic behavior aligns with ethical expectations. Moreover, the system might use reinforcement learning to refine decision-making strategies by evaluating prior outcomes and enhancing future responses.Considering the mathematical complexity involved in optimizing decisions, a simple reward function in a reinforcement learning model can be represented as:\[ R = \text{Sum} \times \text{outcome value} \times \text{probability} \times \text{discount factor} \]Where Reward \( R \) is a function of outcome values associated with potential decisions, each scaled by their probabilities and discounted over time.
Autonomous Decision Making Examples
Autonomous decision making is increasingly prevalent in various technological domains, showing remarkable progress in fields that require swift and independent decision abilities. Below, explore how autonomous decision making is applied in real-world scenarios, highlighting its significance and implications.
Autonomous Vehicles and Decision Making
Autonomous vehicles, like self-driving cars, represent a significant technological advancement that utilizes autonomous decision making. These vehicles rely on a complex array of sensors, cameras, and machine learning algorithms to make real-time decisions. The decision-making process in autonomous vehicles involves analyzing vast amounts of data to navigate roads safely and effectively.1. Perception: Sensors detect and classify objects such as pedestrians, cyclists, and other vehicles.2. Localization: GPS and onboard sensors determine the vehicle's precise location.3. Path Planning: Algorithms calculate the safest and most efficient route.4. Control: The vehicle executes maneuvers such as steering, acceleration, and braking.
Consider a scenario where an autonomous car approaches an intersection:
- Data Collection: Cameras and LIDAR sense surrounding traffic and pedestrians.
- Decision Making: Algorithms decide to proceed, stop, or yield based on traffic light status and pedestrian presence.
- Actuation: The car adjusts its speed or stops accordingly.
Autonomous vehicles must continuously update and refine their decision-making algorithms to address unique and evolving road conditions.
One of the biggest challenges in autonomous vehicles is safely navigating unpredictable urban environments. For instance, when a child suddenly runs onto the road, the system must calculate options in milliseconds to take protective measures. Engineers implement reinforcement learning systems to allow vehicles to adapt to such situations through extensive simulations. Mathematical models are crucial here. For instance, a basic predictive model might be:\[P(t) = f(T(t-1), T(t-2), ...)\]Where \(P(t)\) is the probability of an action at time \(t\), depending on past events. This formula helps in forecasting possible actions quickly and effectively.
Robotics and Autonomous Decision Making in Engineering
In the field of robotics, autonomous decision making is an essential component that enables machines to perform complex tasks autonomously, often in diverse and dynamic environments. From industrial robots to service robots, autonomous decision-making systems enhance the efficiency and functionality of these machines. Key processes include:
Logistics Robots | Sort and distribute packages autonomously using vision and motion sensors. |
Healthcare Robots | Assist in surgeries by making precise decisions based on real-time data. |
Exploration Robots | Navigate terrains autonomously, crucial for mars rovers. |
For a warehouse robot tasked with autonomously sorting parcels:
- Data Collection: Uses cameras to scan barcodes and RFID tags.
- Decision Making: Determines the correct chute for each parcel based on destination data.
- Actuation: Directs parcels using robotic arms to corresponding chutes.
Robotics in agriculture is a rapidly growing field, where robots use autonomous decision making to optimize yield and manage resources.
Integration of machine learning with robotics has revolutionized various sectors by greatly enhancing precision, efficiency, and safety. Take medical robots, for instance, which are capable of executing delicate tasks such as neural surgeries. These robots utilize deep learning to process images and identify regions of interest. Consider their reliance on mathematical optimization:\[J(\theta) = \frac{1}{2m} \times \text{Sum of errors for predicted vs actual outcomes}\]where \(J(\theta)\) represents the cost function optimizing robot's actions, with \(\theta\) indicating decision parameters. Such equations are pivotal in minimizing errors and ensuring desired outcomes, showcasing how essential autonomous decision making is in robotic engineering.
autonomous decision making - Key takeaways
- Autonomous Decision Making Definition: The ability of a system to make decisions independently, often using AI and algorithms, without human intervention.
- Techniques in Autonomous Decision Making: Involves machine learning, algorithms, and data processing to ensure optimal and efficient decisions.
- Autonomous Decision Making Process: Consists of steps like data collection, data processing, decision making, and actuation.
- Machine Learning in Autonomous Decision Making: Utilizes supervised, unsupervised, and reinforcement learning to improve decision-making capabilities over time.
- Neural Networks: Mimic the brain's architecture in autonomous systems to process data and enhance decision-making precision and efficiency.
- Autonomous Decision Making Examples: Includes applications in autonomous vehicles, robotics, and various sectors requiring swift, independent decisions.
Learn faster with the 12 flashcards about autonomous decision making
Sign up for free to gain access to all our flashcards.
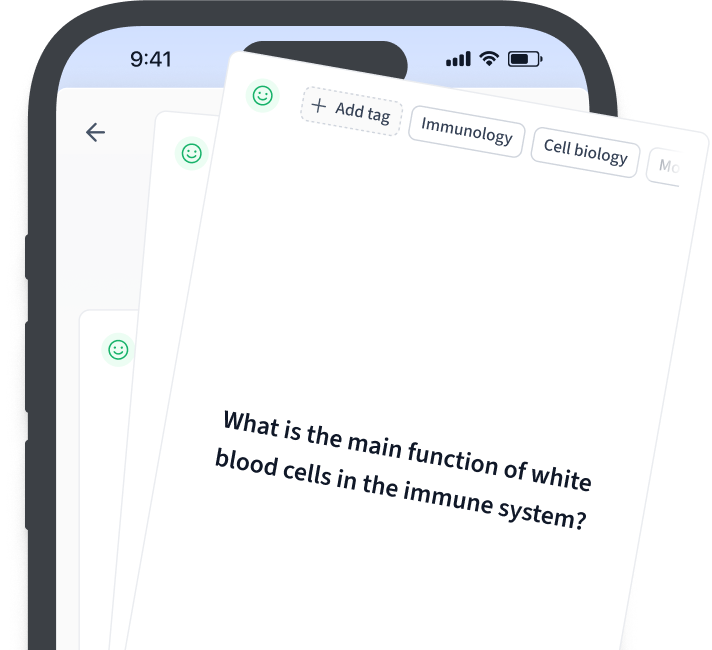
Frequently Asked Questions about autonomous decision making
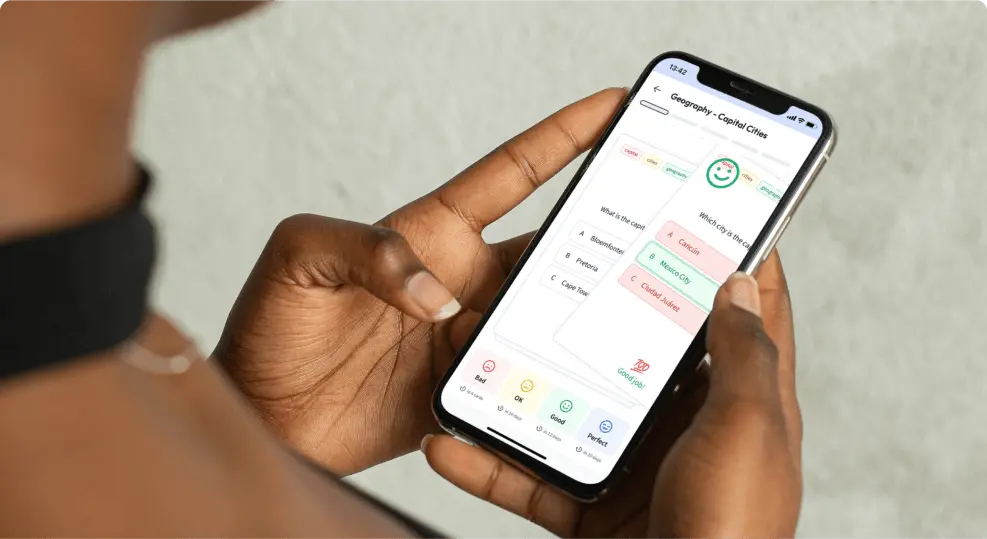
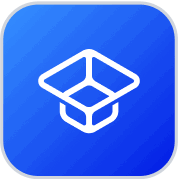
About StudySmarter
StudySmarter is a globally recognized educational technology company, offering a holistic learning platform designed for students of all ages and educational levels. Our platform provides learning support for a wide range of subjects, including STEM, Social Sciences, and Languages and also helps students to successfully master various tests and exams worldwide, such as GCSE, A Level, SAT, ACT, Abitur, and more. We offer an extensive library of learning materials, including interactive flashcards, comprehensive textbook solutions, and detailed explanations. The cutting-edge technology and tools we provide help students create their own learning materials. StudySmarter’s content is not only expert-verified but also regularly updated to ensure accuracy and relevance.
Learn more