Jump to a key chapter
Definition of Convolutional Networks
Convolutional networks, often referred to as CNNs, are a class of artificial neural networks that have revolutionized the field of computer vision. CNNs are particularly well-suited for identifying patterns and structures in images, making them integral to image recognition tasks.
Convolutional Neural Network Basics
A Convolutional Neural Network consists of several layers designed to process visual information in a manner similar to the human brain’s visual cortex. The core components of a CNN include:
- Convolutional Layers: These layers apply a mathematical operation called convolution to the input image, effectively scanning it with a set of filters to highlight specific features.
- Pooling Layers: Often used to reduce the dimensions of the data, pooling layers summarize the results of convolutions, making the network more manageable and efficient.
- Activation Functions: Functions like ReLU (Rectified Linear Unit) are applied to introduce non-linearity into the model, allowing it to learn complex patterns.
- Fully Connected Layers: These layers are similar to those in traditional neural networks and serve to further process the summarized information from the earlier layers, culminating in a classification decision.
Example: Suppose you have a grayscale image of 5x5 pixels and a 3x3 filter. The convolution operation slides over the image, performing element-wise multiplication and summing the results to produce a 3x3 output matrix. Convolutional layers iteratively refine and squeeze information, identifying distinct features like edges or textures.
In more advanced architectures like ResNet and VGG, additional techniques such as skip connections and batch normalization are introduced to enhance performance. Skip connections help in mitigating the vanishing gradient problem by allowing information to bypass one or more layers, while batch normalization normalizes the input of each layer, stabilizing the learning process.
Convolutional Networks vs Other Neural Networks
Convolutional Networks (CNNs) excel in tasks that require analyzing visual data due to their structural design tailored for images. However, compared to other types of Neural Networks, such as:
- Recurrent Neural Networks (RNNs): RNNs are designed for processing sequential data like time series or natural language, retaining internal memory for context analysis.
- Fully Connected Networks: These networks, or dense networks, are used in situations where spatial structure is not a concern. They connect every neuron in one layer to every neuron in the next layer, making them less feasible for image data due to their extensive parameter requirements.
CNNs are generally more data-intensive; having a large dataset helps in harnessing their full potential for accuracy.
Understanding Convolutional Networks
Convolutional Networks, or CNNs, are a powerful type of artificial neural network that specialize in processing data with a grid-like topology, such as images. Their architecture is distinctively composed of multiple layers, each designed to automatically and adaptively learn spatial hierarchies of features from input images. The effectiveness of these networks lies in their ability to detect and recognize intricate patterns and structures.
A Convolutional Network is a type of deep learning model that employs convolutional layers to analyze visual data, aiming to extract sophisticated features from it using specific algorithms like convolution and pooling.
Techniques in Convolutional Networks
CNNs deploy a variety of techniques to process visual data efficiently. Here are the key techniques utilized in convolutional networks:
- Filter Kernels: Small-sized matrices that 'slide' over the input data, creating feature maps that detect specific structures such as edges or textures in images.
- Activation Functions: These introduce non-linearity into the network, with ReLU (Rectified Linear Unit) being the most commonly used due to its simplicity and efficacy.
- Pooling Operations: These operations, such as max pooling, reduce the dimensionality of feature maps while preserving significant information.
- Batch Normalization: This technique normalizes the input of each layer, which can lead to faster convergence and increased performance.
Experimenting with different kernel sizes can significantly influence the performance of a CNN on a particular task.
More sophisticated CNN architectures incorporate additional techniques like Residual Connections, often seen in ResNet models. These bypass pathways connecting non-consecutive layers help in avoiding the vanishing gradient problem by allowing gradients to flow directly through these shortcut pathways.Advanced models also employ Dropout Regularization, which involves randomly setting a portion of activating neurons to zero during training to prevent overfitting. This regularization technique is crucial for improving model robustness.
How Convolutional Networks Process Data
The processing of data in a CNN occurs in several sequential stages, starting with the input layer and culminating in the output layer. The layers are designed to progressively extract higher-level features from the raw input.A typical flow involves:
Input Layer | Takes raw image data, typically resized to match the input dimensions expected by the network. |
Convolutional Layer | Applies multiple filters to create a set of feature maps, capturing various patterns and structures. |
Pooling Layer | Reduces the spatial dimensions of the feature maps, retaining only the most essential features for classification. |
Fully Connected Layer | Collates data from all previous layers, bridging to the final output and aiding in the decision-making process. |
Output Layer | Provides the final class probabilities or regression outputs. |
Consider a CNN trained for digit recognition. For a 28x28 pixel input image of the digit '8', the network's initial layers detect simple patterns such as edges, progressing to more complex shapes until it finally recognizes the digit with high accuracy.
Convolutional Networks in Engineering Applications
Convolutional Networks, or CNNs, have become instrumental in many fields of engineering, contributing to advancements in automation, robotics, and more. They function by analyzing visual data, making them vital for applications that require image interpretation and pattern recognition.
Real-world Examples in Engineering
In the engineering domain, convolutional networks have enabled several innovative applications. These networks help in automating complex tasks and enhancing precision in various processes.Some real-world examples include:
- Automated Quality Inspection: CNNs are deployed in manufacturing lines to detect defects in products by analyzing high-resolution images of components.
- Infrastructure Monitoring: Engineers use CNNs to monitor structures like bridges or buildings, detecting cracks or deformations from camera feeds.
- Robotics and Automation: In robotics, these networks assist in visual guidance, helping robots to interpret and interact with dynamic environments.
Example: Consider a CNN-based system in a solar panel manufacturing plant. It analyzes images of solar panels to ensure there are no micro-cracks before they are shipped. The CNN is trained on thousands of images of both defect-free and flawed panels, enabling it to spot even the tiniest cracks without human intervention.
Training CNNs with augmented datasets enhances their ability to perform accurately in varied manufacturing scenarios.
Benefits of Using Convolutional Networks in Engineering
Convolutional networks offer numerous benefits in the field of engineering by making processes more efficient and precise. Here are some notable advantages:
- Enhanced Accuracy: Their ability to recognize subtle patterns and anomalies helps in improving the accuracy of engineering solutions.
- Scalability: CNNs can be scaled to handle large amounts of data with high-dimensional inputs, making them ideal for complex engineering projects.
- Cost Efficiency: By reducing the need for manual inspection and intervention, CNNs lower operational costs.
- Real-time Processing: These networks excel at handling real-time data, providing instant analysis and feedback which is crucial in dynamic environments.
An advanced CNN application in engineering is predictive maintenance, where these networks are used to predict equipment failures before they occur. This involves analyzing streams of image data and other sensor inputs to forecast potential issues. This predictive capability allows engineers to perform maintenance precisely when needed—avoiding downtime and optimizing repair cycles.The incorporation of hybrid models, which combine CNNs with other machine learning models like Reinforcement Learning, creates robust systems capable of making decisions based on visual and contextual data. These hybrid models bring about improvements in fields like autonomous driving and smart grid management.
Educational Resources on Convolutional Networks
Exploring educational resources on Convolutional Networks is essential for gaining a comprehensive understanding of this field. These resources range from online courses to insightful books and articles, offering guidance for both beginners and advanced learners.
Online Courses for Convolutional Networks
Enrolling in online courses provides structured learning experiences to deepen your knowledge of convolutional networks. Here are some recommended options:
- Coursera's Deep Learning Specialization: Offered by DeepLearning.AI, this course covers the fundamentals of neural networks, including CNNs, and provides hands-on projects for practical understanding.
- Udacity's Computer Vision Nanodegree: This program dives into CNNs, teaching you how to implement these networks effectively for computer vision tasks using platforms like TensorFlow and PyTorch.
- edX's Introduction to Artificial Intelligence with Python: Developed by Harvard University, this course introduces AI concepts with an emphasis on image recognition using CNNs.
import tensorflow as tfX = tf.placeholder(tf.float32, shape=[None, 28, 28, 1])conv_layer = tf.layers.conv2d(X, filters=32, kernel_size=(5, 5), activation=tf.nn.relu)
Participating in forums and discussion groups related to these courses can significantly improve your understanding of complex topics.
Recommended Books and Articles
For those who prefer reading, books and articles offer in-depth perspectives and theoretical foundations for convolutional networks. Here are some highly recommended texts:
- Deep Learning by Ian Goodfellow, Yoshua Bengio, and Aaron Courville: This comprehensive book covers the fundamentals of deep learning, exploring techniques, including CNNs, through detailed explanations and case studies.
- Hands-On Machine Learning with Scikit-Learn, Keras, and TensorFlow by Aurélien Géron: This practical guide provides examples and hands-on exercises to build real-world applications using CNNs.
- Research Articles in Journals: Journals like the IEEE Transactions on Neural Networks and Learning Systems publish cutting-edge articles demonstrating the latest advances in CNN research and applications.
For those interested in the cutting-edge developments of convolutional networks, journals and conference proceedings such as NeurIPS, CVPR, and ICCV are invaluable. They offer access to peer-reviewed papers on the latest theoretical advancements and novel CNN architectures, pushing the boundaries of current understanding. Researchers frequently introduce new concepts like dilated convolutions and attention mechanisms, which further enhance CNN capabilities and are essential reads for those wishing to stay at the forefront of this field.
convolutional networks - Key takeaways
- Definition of Convolutional Networks: A type of deep learning model employing layers like convolutional and pooling layers to process visual data.
- Convolutional Neural Network (CNN) Basics: Structures like convolutional and pooling layers, with ReLU activation functions and fully connected layers to learn visual features.
- Techniques in Convolutional Networks: Usage of filter kernels, pooling operations, batch normalization, and dropout regularization to enhance network learning.
- Convolutional Networks in Engineering Applications: Used in automated quality inspection, infrastructure monitoring, and robotics for image analysis.
- Understanding Convolutional Networks: CNNs specialize in identifying patterns in images, making them ideal for computer vision tasks due to their architecture.
- Educational Resources on Convolutional Networks: Online courses, books, and research articles provide a comprehensive understanding of CNN concepts and applications.
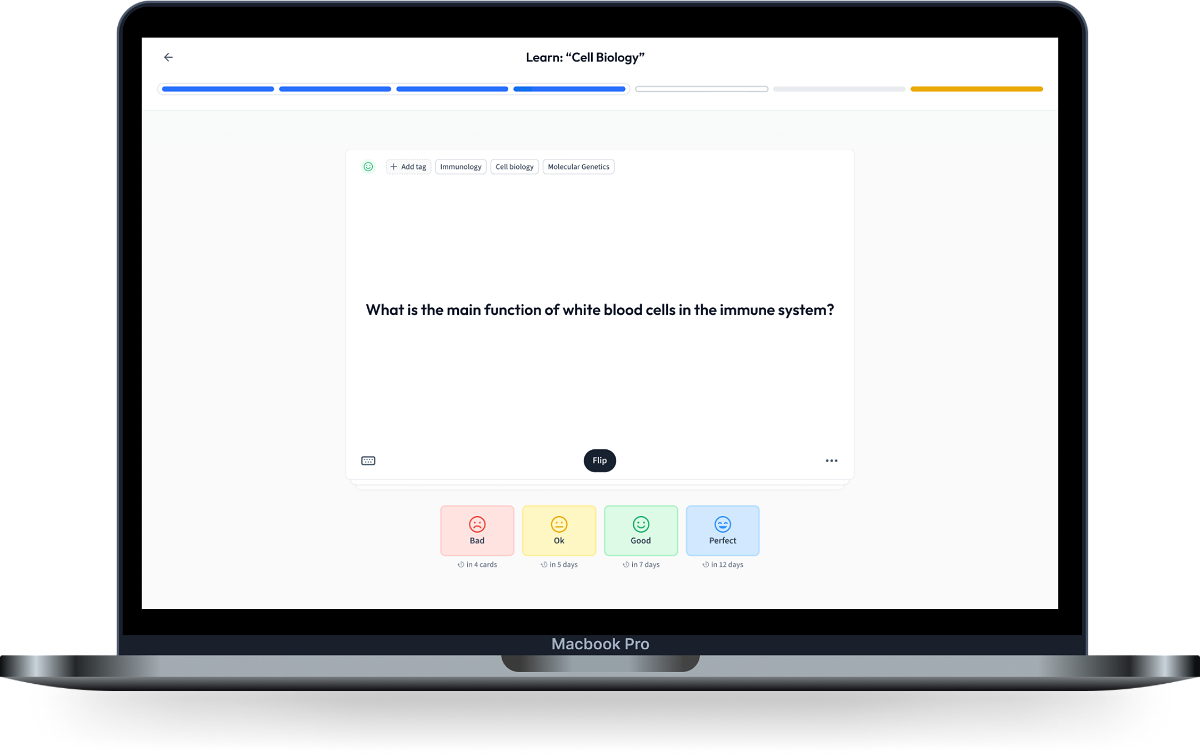
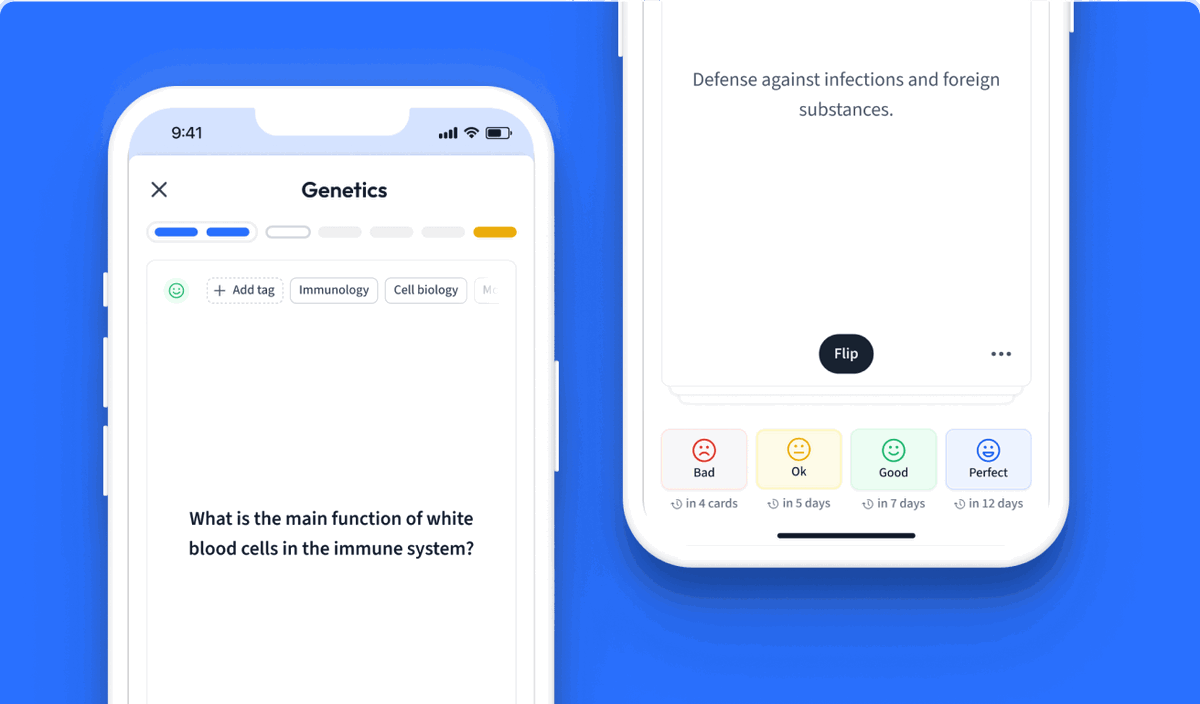
Learn with 12 convolutional networks flashcards in the free StudySmarter app
We have 14,000 flashcards about Dynamic Landscapes.
Already have an account? Log in
Frequently Asked Questions about convolutional networks
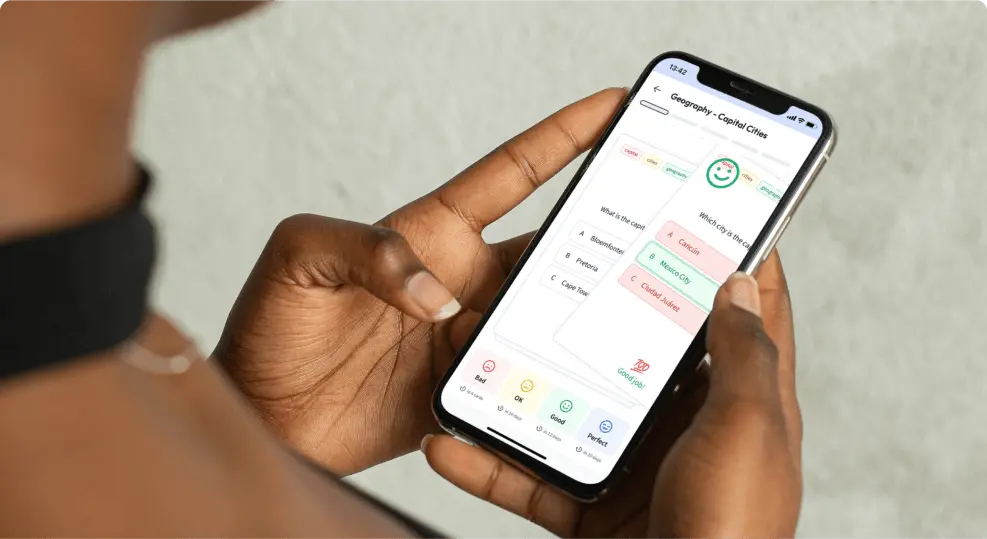
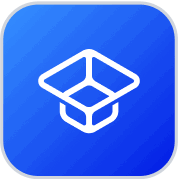
About StudySmarter
StudySmarter is a globally recognized educational technology company, offering a holistic learning platform designed for students of all ages and educational levels. Our platform provides learning support for a wide range of subjects, including STEM, Social Sciences, and Languages and also helps students to successfully master various tests and exams worldwide, such as GCSE, A Level, SAT, ACT, Abitur, and more. We offer an extensive library of learning materials, including interactive flashcards, comprehensive textbook solutions, and detailed explanations. The cutting-edge technology and tools we provide help students create their own learning materials. StudySmarter’s content is not only expert-verified but also regularly updated to ensure accuracy and relevance.
Learn more