Jump to a key chapter
Definition of Moral AI in Engineering
Moral AI refers to the ability of artificial intelligence systems to make decisions that align with ethical and moral principles. In engineering, these systems play a crucial role in ensuring that AI applications operate within acceptable moral boundaries.
Moral AI Definition and Scope
In the realm of engineering, Moral AI is defined as the integration of ethical decision-making capabilities within AI systems. This involves programming AI to consider human values and ensure its actions are morally acceptable. The scope of Moral AI extends beyond merely following rules; it includes understanding the consequences of AI actions and aligning them with societal values.
Moral AI: AI systems imbued with ethical decision-making capabilities to align their actions with human values and societal norms.
Consider an AI system in autonomous vehicles programmed to avoid pedestrians. A decision-making algorithm must be designed to prioritize human safety without causing harm to passengers. This exemplifies the implementation of Moral AI in practical applications.
Moral AI is not limited to avoiding harm; it also involves maximizing positive outcomes in complex ethical scenarios.
Core Concepts of Moral AI
Understanding the core concepts of Moral AI is essential for implementing ethical AI solutions. Here are some fundamental ideas you should consider:
- Ethical Algorithms: Algorithms in Moral AI are designed to evaluate situations based on ethical frameworks. These frameworks guide AI in making moral decisions.
- Value Alignment: The objective is to align AI actions with human values. This requires modeling moral behaviors and incorporating them into AI behavior.
- Accountability: AI systems must be accountable for their decisions. This includes tracing decision-making processes and ensuring they follow ethical guidelines.
The concept of Ethical Algorithms in Moral AI means that the AI must have the ability to weigh various options and decide based on a predetermined set of ethical rules. For example, in healthcare, AI can prioritize patient treatment plans based on urgency and resource availability. This involves complex algorithmic structures and machine learning techniques that can simulate ethical reasoning. The challenge involves not just programming ethical behavior but ensuring the AI can adapt to new ethical dilemmas without human intervention.
Importance of Moral AI in Engineering
The importance of Moral AI in engineering cannot be overstated. It aims to ensure AI technology is used responsibly and ethically across various sectors:
- Safety: By integrating Moral AI, we reduce risks of harm caused by AI systems in high-stakes environments such as autonomous vehicles and healthcare.
- Public Trust: Implementing moral decision-making capabilities engenders trust among users and the public, ensuring broader acceptance and use of AI technologies.
- Regulatory Compliance: Adhering to ethical standards helps meet legal obligations and minimizes regulatory risks.
Developing Moral AI aligns with global initiatives to incorporate ethics in AI, fostering sustainable and fair advancements in technology.
Ethical Implications of AI in Engineering
The integration of AI in engineering introduces a range of ethical implications that need to be addressed. These implications stem from the potential impacts of AI systems on human society and values. Understanding these ethical considerations ensures that AI technologies enhance rather than harm human life.
Challenges and Considerations
As you delve into the challenges posed by AI in engineering, it's crucial to understand that these challenges are multi-dimensional and require careful navigation. Here are a few considerations:
- Bias in AI: Algorithms can inherit biases from their training data, leading to unfair outcomes.
- Privacy Concerns: AI systems often require large amounts of data, raising issues around user privacy.
- Informed Consent: Users may not fully understand how their data is used by AI, making informed consent difficult to obtain.
Imagine an AI system used in recruitment processes. If it's trained on historical data with inherent biases, it may unfairly favor certain demographics over others. This showcases the challenge of bias in AI.
Regular auditing of AI systems can help identify and mitigate bias, ensuring fairer outcomes.
The challenge of Privacy Concerns in AI systems is significant. Engineers must implement robust data protection measures to safeguard user information. This involves encrypting data and ensuring compliance with data protection regulations such as GDPR. Another layer of complexity is added when dealing with cross-border data flows, where varying national regulations may apply. Engineers must stay informed about these regulations to avoid legal pitfalls and ensure that AI innovations respect user privacy.
Mitigating Ethical Risks
To mitigate ethical risks associated with AI in engineering, a proactive approach is needed. This involves:
- Transparent AI Development: Maintain transparency in AI's decision-making processes to build trust.
- Ethical Guidelines: Adhere to established ethical guidelines across all stages of AI deployment.
- Stakeholder Engagement: Involve diverse stakeholders in AI development to ensure ethical considerations are addressed.
Transparent AI Development: The process of making AI's decision-making logic accessible and understandable to users and stakeholders.
An effective way to achieve transparency is through clear documentation and explanation of AI algorithms. Additionally, utilizing visual tools to demonstrate how AI makes decisions can further aid understanding. A holistic ethical framework can serve as a foundation for these practices, keeping engineering teams accountable and aligned with societal values.
Developing a culture of ethical awareness in engineering teams can enhance compliance with ethical guidelines.
Moral AI Examples in Engineering
Exploring examples of Moral AI in engineering provides valuable insights into how ethical decision-making is integrated into AI systems. These examples highlight the practical application of Moral AI principles across varying contexts.
Case Studies of Moral AI
Case studies offer a deep look into how Moral AI operates within specific engineering projects. They demonstrate how ethical frameworks are applied to real-world problems and how AI systems are designed to align with moral values.
- Autonomous Transportation: One notable case study is the implementation of autonomous vehicles. Here, Moral AI algorithms are programmed to make quick decisions in potential collision scenarios, prioritizing human life over property damage.
- Healthcare Diagnosis: AI systems in healthcare are designed to assist in diagnoses. Moral AI ensures that these systems base their recommendations on accurate data and ethical considerations, avoiding biases against any patient group.
Consider an autonomous vehicle required to choose between damaging the car itself or avoiding a pedestrian on the road. Moral AI helps the vehicle to prioritize actions that preserve human life.
Incorporating Moral AI in decision-making processes helps autonomous systems align with human ethics.
In the healthcare sector, Moral AI is especially critical due to the sensitive nature of medical data and the potential impacts of AI-driven decisions on patients' lives. A noteworthy implementation is in AI-enhanced diagnostic tools, which can assist doctors by suggesting possible diagnoses. These tools are designed to avoid biases by training on diverse datasets, thus ensuring fairness. Moreover, AI systems must comply with strict privacy laws, safeguarding patient information. This requires engineers to balance AI's data needs with ethical data handling practices.
Real-World Applications
The application of Moral AI in engineering is evident in various real-world scenarios. These applications demonstrate the potential of AI systems to adhere to ethical norms across multiple industries.
- Robotics and Automation: In manufacturing, robots equipped with Moral AI can optimize production lines while ensuring worker safety.
- Smart City Solutions: AI systems managing urban infrastructures utilize Moral AI to balance resource distribution fairly, enhancing the quality of life for residents.
Smart City Solutions: Systems that integrate AI to manage urban resources efficiently and ethically, ensuring equitable access for all city residents.
In a smart city, traffic management systems with Moral AI prioritize emergency vehicles during congestion, minimizing response times and potentially saving lives.
Moral AI in smart cities aids in fair resource distribution, thus addressing social inequalities.
Developing Moral AI Techniques
Developing Moral AI involves creating AI systems that can perform decision-making processes aligned with ethical and moral principles. This section explores the techniques and tools required to achieve such capabilities.
Techniques for Implementing Moral AI
Implementing Moral AI requires a strategic approach that includes various technologies and methodologies. Below are some techniques to integrate moral decision-making capabilities into AI systems:
- Ethical Rule Programming: Design AI algorithms to adhere to specific ethical rules. This could involve creating a set of ethical guidelines that the AI must follow.
- Machine Learning and Neural Networks: Use machine learning techniques to train AI models to recognize ethical dilemmas and respond appropriately based on learned inputs.
Consider an AI system for loan approval that needs to evaluate applications fairly. By using machine learning, the AI can learn from historical data to detect and reduce biases, promoting fair decisions.
Regularly updating and retraining AI models is essential to adapt to new ethical standards and societal norms.
A significant aspect of implementing Moral AI is the use of reinforcement learning, a subset of machine learning. It involves training AI models through feedback from their actions. In a moral context, the AI receives 'rewards' for making ethically correct decisions and 'penalties' for unethical actions. This technique allows AI systems to improve their ethical decision-making over time, adapting to more complex ethical dilemmas as they arise. Reinforcement learning helps in setting up a dynamic framework where AI continuously learns and refines its understanding of ethical behavior without human intervention.
Tools and Frameworks
Tools and frameworks are critical for developing Moral AI, as they provide the necessary resources and environments to test and implement ethical decision-making. Notable tools and frameworks include:
- AI Fairness 360 (IBM): This toolkit offers a comprehensive framework for developing unbiased AI systems, with methods to evaluate and mitigate bias in machine learning models.
- OpenAI Baselines: These are high-quality implementations of reinforcement learning algorithms, facilitating the integration of moral decision-making in AI solutions.
Using AI Fairness 360, an engineering team can assess a financial AI system for bias and apply corrections to ensure ethical lending practices.
Choosing the right tools is crucial, as they determine the efficiency and effectiveness of ethical AI implementation.
Moral AI and How We Get There
Getting to Moral AI is an ongoing process that involves continuous learning and adaptation. Several steps are essential in this journey:
- Research and Development: Ongoing R&D in AI ethics helps to identify emerging ethical challenges and solutions.
- Interdisciplinary Collaboration: Engaging experts from various fields, such as ethics, law, and computer science, fosters comprehensive solutions to moral challenges.
Collaboration between disciplines is particularly valuable when considering the cultural dimensions of ethics. What is considered moral can vary significantly across different cultures and societies. Thus, moral AI must be adaptable to cultural contexts, requiring input from sociologists and anthropologists. By leveraging diverse perspectives, AI systems can be more culturally sensitive, increasing their acceptance and efficacy worldwide. Researchers are exploring computational models that factor in cultural norms, ensuring AI respects local ethical standards while maintaining its foundational moral principles.
moral ai - Key takeaways
- Definition of Moral AI in Engineering: Refers to AI systems capable of making decisions that align with ethical and moral principles, ensuring they operate within acceptable moral boundaries.
- Scope of Moral AI: Involves integrating ethical decision-making capabilities into AI, considering human values and societal norms beyond just rule-following.
- Ethical Implications in Engineering: Moral AI in engineering aims to ensure AI systems enhance rather than harm human society through responsible and ethical applications.
- Moral AI Examples in Engineering: Includes AI in autonomous vehicles prioritizing human life and healthcare systems avoiding biases in diagnosis.
- Moral AI Techniques: Encompasses ethical rule programming and machine learning to handle ethical dilemmas and support moral decision-making.
- Path to Moral AI: Requires continuous R&D, interdisciplinary collaboration, and cultural adaptability to achieve moral AI systems worldwide.
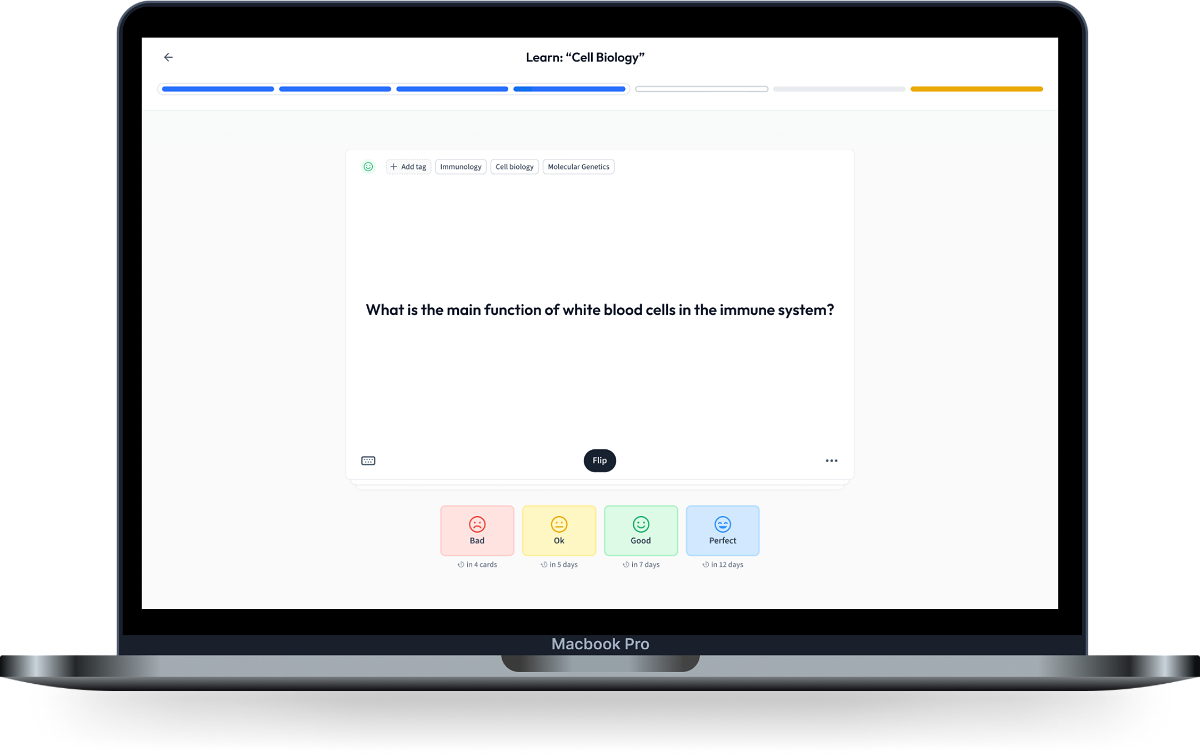
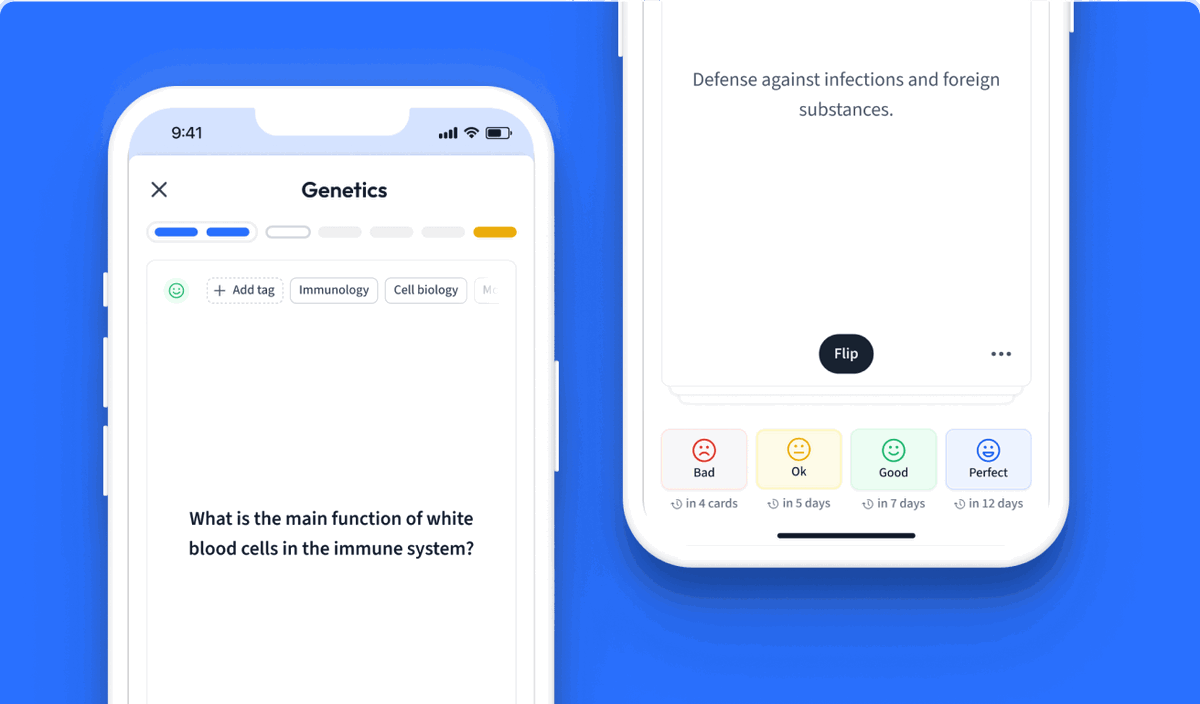
Learn with 12 moral ai flashcards in the free StudySmarter app
We have 14,000 flashcards about Dynamic Landscapes.
Already have an account? Log in
Frequently Asked Questions about moral ai
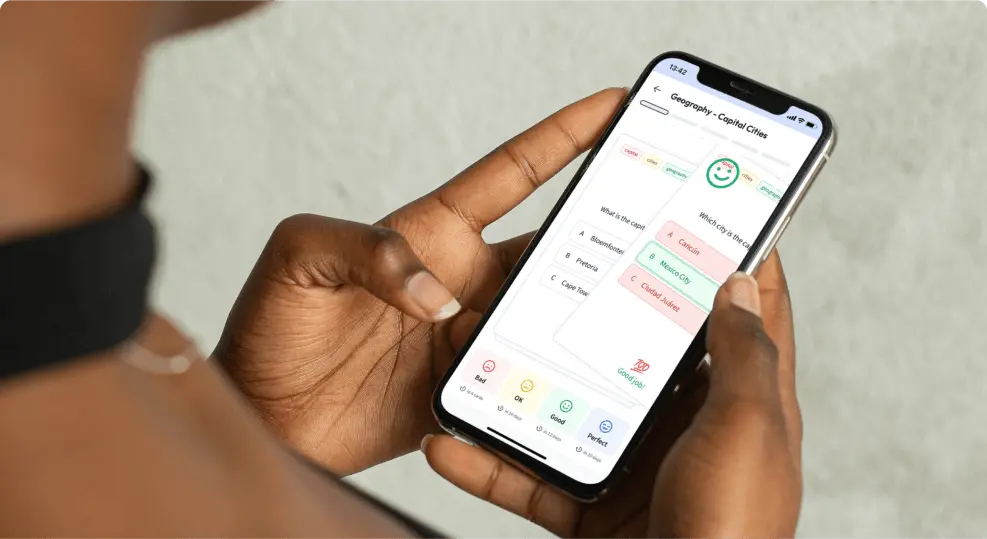
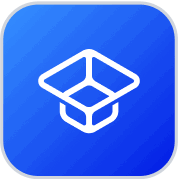
About StudySmarter
StudySmarter is a globally recognized educational technology company, offering a holistic learning platform designed for students of all ages and educational levels. Our platform provides learning support for a wide range of subjects, including STEM, Social Sciences, and Languages and also helps students to successfully master various tests and exams worldwide, such as GCSE, A Level, SAT, ACT, Abitur, and more. We offer an extensive library of learning materials, including interactive flashcards, comprehensive textbook solutions, and detailed explanations. The cutting-edge technology and tools we provide help students create their own learning materials. StudySmarter’s content is not only expert-verified but also regularly updated to ensure accuracy and relevance.
Learn more