Jump to a key chapter
Introduction to Quantum Simulations
Quantum simulations are an exciting area of research poised to revolutionize various fields, from physics to chemistry. They harness the principles of quantum mechanics to simulate complex systems, predicting their behavior with high accuracy.
Quantum Simulation Explained
Quantum simulation involves using quantum computers or quantum systems to simulate other quantum systems, which is often more efficient than classical simulations. It's based on the notion that a quantum computer can naturally represent quantum states and processes, potentially solving these problems much faster.
Quantum Simulation: A process that uses quantum mechanics principles to simulate the behavior of complex quantum systems, typically leveraging quantum computers.
Quantum simulations can solve problems that are nearly impossible for classical computers due to the sheer scale and complexity involved.
Why does quantum simulation matter? Consider molecules and their interactions, which are inherently quantum mechanical in nature. The complexity arises from the many-body problem, described by the Schrödinger equation: \[ H\Psi = E\Psi \]where \( H \) is the Hamiltonian operator, \( E \) are the energy eigenvalues, and \( \Psi \) represents the wave function of the system. Quantum simulation offers a feasible way to study these interactions as they are computationally expensive using classical means.
Quantum Simulation Techniques
There are several techniques utilized in quantum simulations:
- Gate-model quantum computing: Involves using qubits and quantum gates to perform computations analogous to logic gates in classical computing.
- Adiabatic quantum computing: Relies on keeping the quantum system in its ground state while the Hamiltonian evolves over time.
- Quantum annealing: Used primarily for solving combinatorial optimization problems by exploiting quantum tunneling and entanglement.
Example of Gate-model: Consider an algorithm to factor a number, like Shor's algorithm, which leverages quantum mechanics to outperform classical algorithms. The quantum circuit design involves specific gates to enhance the probability amplitude of correct answers.
Examples of Quantum Simulation Problems
Quantum simulations can address several complex problems, from materials science to biology. Here are a few notable examples:
- Drug discovery: Simulating molecular interactions to identify potential drug candidates.
- Condensed matter physics: Investigating superconductors or quantum phase transitions.
- Quantum chemistry: Computing electronic structures to understand chemical reactions.
Example in Drug Discovery: Simulating the interaction between a drug molecule and its target protein to predict efficacy can be afforded by quantum simulations, potentially accelerating the drug development pipeline.
Applications of Quantum Simulation in Engineering
Quantum simulation is emerging as a game-changing tool in the field of engineering. By leveraging the power of quantum mechanics, it enhances the precision of simulations, rendering problems that were previously deemed unsolvable as solvable.
Quantum Simulation for Material Science
In material science, quantum simulations are invaluable for understanding and predicting the properties of new materials. These simulations allow for the exploration of atomic and molecular behaviors that govern material properties. Importantly, using quantum simulations, you can:
- Discover novel materials with specific desirable properties.
- Optimize material composition for strength and durability.
- Assess thermal and electrical conductivity at the atomic level.
E.g., Discovery in Material Science: A recent study utilized quantum simulations to design new polymers with enhanced conductivity for flexible electronic devices, showcasing the potential for innovation directly from simulation results.
Material scientists are often interested in the band structure of materials, which determines their electronic properties. The key equations revolve around calculating the Schrödinger equation for periodic structures, expressed as: \[ H\Psi = E\Psi \] Solving this for different potential energies \( V(x) \) allows us to understand the band gaps, crucial for semiconductors. Quantum simulations offer a pathway to compute these phenomena with high precision.
Quantum Simulation in Electronics
Quantum simulations are also impacting the electronics industry, pushing the frontiers of miniaturization and efficiency in device design. They help in addressing challenges such as:
- Understanding electron transport mechanisms in nanostructures.
- Optimizing semiconductor doping processes.
- Enhancing performance of components like transistors and diodes.
Quantum Transport: Refers to the movement of electrons in a material, significantly affected by quantum tunnelling and interference effects in nanoscale devices.
E.g., Application in Electronics: Using quantum simulations, researchers have developed more efficient algorithms to predict electron paths in graphene and other 2D materials, leading to breakthroughs in flexible electronics.
In electronics, the impact of quantum simulations is profound in the analysis of mesh networks via quantum algorithms. An equation crucial to this is: \[ g(E) = T\times\left(\frac{2e^2}{h}\right) \] where \( g(E) \) is the conductance, \( T \) is the transmission coefficient, and \( \frac{2e^2}{h} \) is the conductance quantum. Quantum simulations can thus predict nanoscale device behaviors more accurately, aiding in optimal component design.
Quantum simulations empower a more sustainable electronic future by reducing the need to physically produce and test every new material configuration.
Quantum Simulation for High-Energy Physics
Quantum simulation stands at the forefront of high-energy physics, offering insights into complex phenomena like particle interactions and cosmological events.
Role of Quantum Simulation in Particle Physics
In particle physics, quantum simulations offer a path to explore the fundamental forces and particles postulated by the Standard Model. The complexity of these systems makes conventional computing challenging, but quantum simulations provide powerful tools for:
- Modeling particle collisions at high energies within accelerators such as the LHC.
- Simulating dark matter interactions, a key unsolved mystery in physics.
- Investigating the quantum chromodynamics (QCD) that govern strong forces.
Quantum Chromodynamics (QCD): A theory describing the strong interaction, one of the fundamental forces in the universe, which impacts the way quarks and gluons interact in protons and neutrons.
Example in QCD: Quantum simulations are used to model gluon interactions at high energies, enabling better understanding of the force binding quarks inside neutrons and protons.
A core challenge in modeling particle interactions is the non-perturbative nature of QCD. The Lattice QCD approach, a computational technique that discretizes space-time into a lattice, is enhanced by quantum simulations to solve the equations of motion for quarks and gluons. This can be described by complex path integrals, where: \[ Z = \int \mathcal{D}\phi \ e^{-S[\phi]} \] Here, \( Z \) is the partition function, and \( S[\phi] \) the action defined for field configurations \( \phi \), representing a key computational focus supported by quantum simulations.
Quantum Simulation for Astrophysics
Quantum simulations also significantly impact astrophysics by allowing analysis of large-scale cosmic phenomena. They are employed to:
- Simulate the behavior of dense stellar objects, like neutron stars, to understand state equations.
- Model early universe conditions, shedding light on primordial nucleosynthesis.
- Analyze quantum fluctuations that could lead to galaxy formation.
Quantum simulations allow the exploration of black hole singularities where classical physics fails.
Example in Astrophysics: The simulation of quantum tunneling within a collapsing star reveals insights into gravitational wave production, an area yielding new observational data from instruments like the LIGO.
In cosmology, quantum simulation assists in understanding the inflationary period of the universe, crucial for explaining its homogeneity and isotropy. One aspect involves modeling scalar field fluctuations as these lead to density perturbations observed in the cosmic microwave background. The corresponding potential \( V(\phi) \) is essential in such simulations and is often described by: \[ V(\phi) = \frac{1}{2}m^2\phi^2 + \frac{\lambda}{4!}\phi^4 \] Here, \( \phi \) is the scalar field, \( m \) its mass, and \( \lambda \) the interaction term, pivotal for probing early universe physics using quantum simulations.
Advances and Future of Quantum Simulation
The field of quantum simulation is rapidly evolving, with new technologies emerging that enhance our ability to perform complex simulations. These advances offer a glimpse into the future possibilities in engineering and scientific research.
Emerging Quantum Simulation Technologies
Recent developments in quantum technologies have significantly boosted the capabilities of quantum simulations. Here are some notable technologies:
- Superconducting Qubits: These qubits leverage superconducting circuits to achieve significant coherence times, suitable for simulations in material science.
- Ion Trap Quantum Computers: Using ions trapped in electromagnetic fields, these computers offer precise control for quantum simulations of large systems.
- Topological Qubits: These hypothetical qubits promise robustness against errors, potentially increasing the scalability and reliability of quantum simulations.
Superconducting Qubits: These are qubits that utilize circuits with zero electrical resistance, enabling more stable quantum computations.
Example of Application: Superconducting qubits have been integral in simulating quantum phase transitions in exotic materials, revealing insights into potential new states of matter.
The effectiveness of these technologies is often quantified by their quantum volume, a metric that considers qubit count, coherence time, gate fidelity, and connectivity. For instance, a superconducting qubit system's performance is described by: \[ QV = (2^n) \times r \times \text{fidelity} \times \text{depth} \] where \( n \) is the number of qubits, \( r \) is the error rate, and \( \text{depth} \) refers to gate depth capability. Quantum volume reflects the overall power of quantum systems and their potential in simulation tasks.
Future Prospects in Engineering with Quantum Simulations
Quantum simulations are poised to transform various engineering disciplines by addressing complex challenges in ways classical tools cannot. Here are some promising prospects:
- Energy Optimization: Quantum simulations can optimize energy grids and improve the efficiency of renewable energy resources.
- Nanotechnology: Simulating quantum effects in nanomaterials, leading to the development of smaller, more efficient devices.
- Supply Chain Management: Enhancements in logistics algorithms through quantum simulations could streamline operations and reduce costs.
Future breakthroughs in quantum error correction will drastically improve simulation accuracy and reliability, paving the way for widespread adoption.
Impact in Energy Optimization: Quantum simulations could accurately predict fluctuations in solar panel efficiencies across regions with variable weather patterns, optimizing energy distribution strategies.
One long-term vision for quantum simulations is their role in creating quantum internet. Key to this is the distribution of entangled qubits across vast distances, facilitating instantaneous information exchange. Central to this vision lies the challenge of maintaining entanglement, represented by the entanglement entropy \( S \) for a system, calculated as: \[ S = - \sum_{i}^n \lambda_i \log(\lambda_i) \] where \( \lambda_i \) are the proportions of each quantum state in the superposition. Advances in quantum simulations could support better understanding and realization of such entangled systems, revolutionizing communication technologies.
quantum simulation - Key takeaways
- Quantum Simulation: A process that uses quantum mechanics principles to simulate the behavior of complex quantum systems, often utilizing quantum computers.
- Quantum Simulation Techniques: Includes gate-model quantum computing, adiabatic quantum computing, and quantum annealing, each exploiting quantum mechanical properties.
- Applications in Engineering: Enhances precision in simulations, impacts material science, electronics, and energy optimization through the power of quantum mechanics.
- Examples of Quantum Simulation Problems: Tackles complex issues like drug discovery, condensed matter physics, and quantum chemistry that classical computing struggles to simulate.
- Quantum Simulation for High-Energy Physics: Offers insights into particle interactions and cosmological events, supporting studies in particle physics and astrophysics.
- Emerging Technologies: Superconducting qubits, ion trap quantum computers, and topological qubits are advancing the capabilities and reliability of quantum simulations.
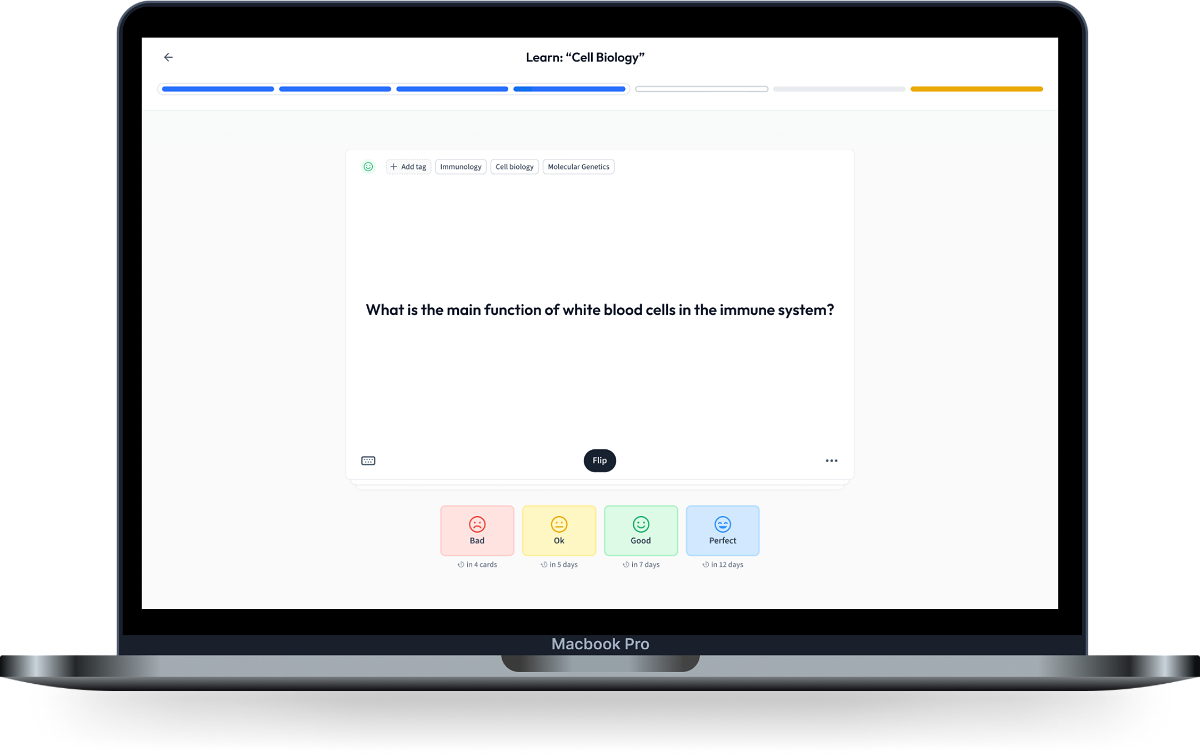
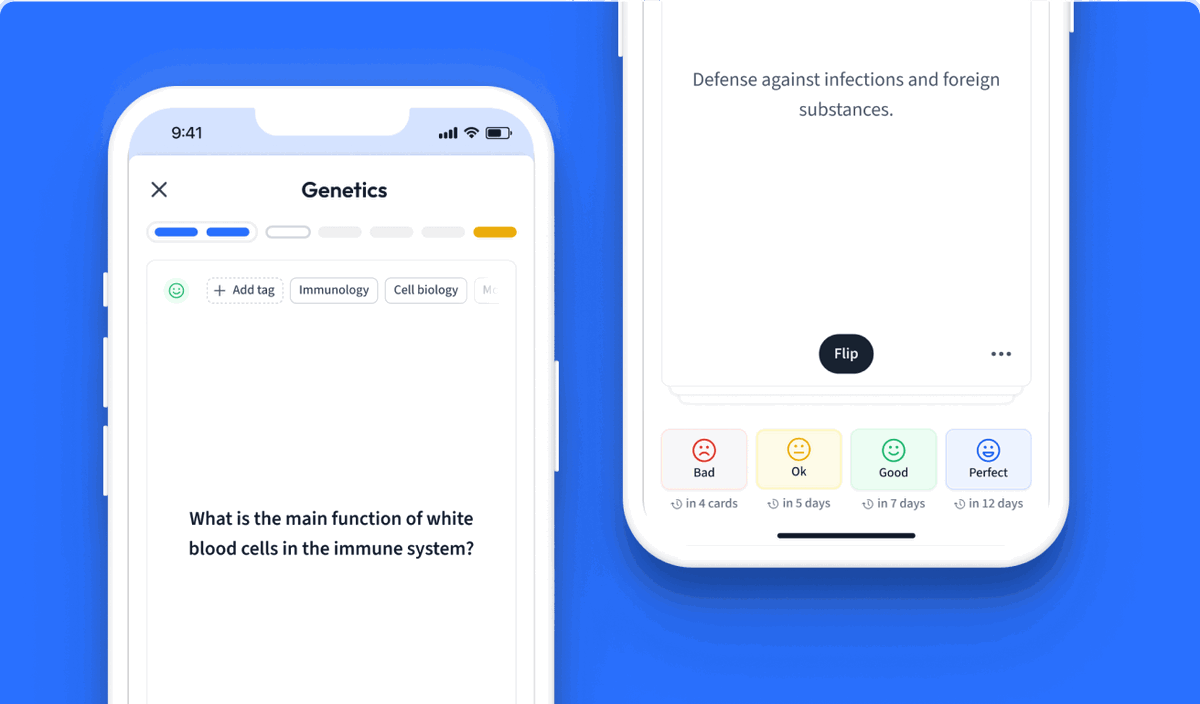
Learn with 12 quantum simulation flashcards in the free StudySmarter app
Already have an account? Log in
Frequently Asked Questions about quantum simulation
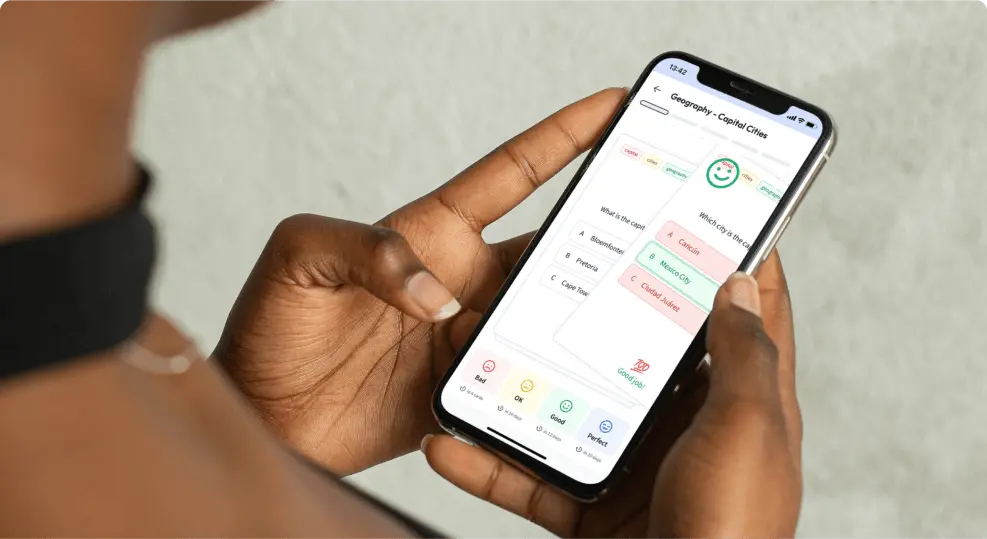
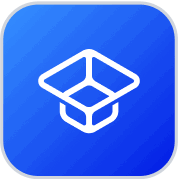
About StudySmarter
StudySmarter is a globally recognized educational technology company, offering a holistic learning platform designed for students of all ages and educational levels. Our platform provides learning support for a wide range of subjects, including STEM, Social Sciences, and Languages and also helps students to successfully master various tests and exams worldwide, such as GCSE, A Level, SAT, ACT, Abitur, and more. We offer an extensive library of learning materials, including interactive flashcards, comprehensive textbook solutions, and detailed explanations. The cutting-edge technology and tools we provide help students create their own learning materials. StudySmarter’s content is not only expert-verified but also regularly updated to ensure accuracy and relevance.
Learn more