Jump to a key chapter
Responsible AI - Fundamentals
In a world increasingly populated by intelligent systems, understanding the fundamentals of Responsible AI is essential. AI systems are integrating ever more into our lives, and it's crucial that they operate in ways that are beneficial and ethical. This section delves into the core principles that guide the development and deployment of responsible AI systems.
Core Principles of Responsible AI
Responsible AI is underpinned by several core principles aimed at ensuring fairness, transparency, and accountability in AI systems. These principles serve as guidelines for engineers and developers to align AI technologies with human values and ethical standards. Let’s delve into these principles:
Fairness: This principle aims to eliminate bias and discrimination in AI systems. Fair AI should provide equal opportunities and outcomes for all individuals, regardless of their background or identity.
- Transparency: It’s important for AI systems to be understandable and transparent. This means being able to explain how decisions are made and ensuring the decision-making process is visible to users and regulators.
- Accountability: Implementing accountability ensures that AI systems can be held responsible for their actions. It involves assigning liability and establishing mechanisms for recourse in the case of malfunctions or unintended outcomes.
- Privacy and Security: AI systems must safeguard data privacy and be immune to security breaches. Protecting personal data must be a priority to build trust among users.
Imagine using an AI-based hiring platform. To adhere to the principle of fairness, the platform should be designed to avoid bias based on gender, race, or age. This means ensuring that the algorithm evaluates each candidate based on skills and experience alone, without prejudice.
Delving deeper into the principle of Transparency, it is notable that there are interpretability techniques developed to determine why AI models make their predictions. Methods like Layer-wise Relevance Propagation (LRP) trace the decisions back to input data, helping users understand which parts of the input were most influential. This allows developers and stakeholders to ensure the model processes data in an expected manner. Additionally, these techniques are continuously evolving to match the complexity of modern AI models, promoting better understanding.
Tools and Techniques for Implementing Responsible AI
Implementing Responsible AI requires specific tools and methodologies. Various platforms and frameworks help developers create and maintain AI systems that align with ethical guidelines and standards. Below are some common tools and techniques:
- Fairness Toolkits: Libraries and frameworks like Google’s What-If Tool and IBM’s AI Fairness 360 assist developers in detecting and mitigating bias in AI systems.
- Model Explainability Tools: These tools offer insights into AI decision-making. Examples include SHAP (SHapley Additive exPlanations) and LIME (Local Interpretable Model-agnostic Explanations), which provide explanations of individual predictions.
- Privacy-Preserving Frameworks: Techniques such as Federated Learning and Differential Privacy help protect user data while maintaining the utility of AI models.
Did you know? AI ethics play a central role in protecting human rights and promoting social welfare. Upholding ethical standards in AI ensures technology benefits society widely without causing unintended harm.
Responsible AI in Engineering
Engineering has always sought to incorporate innovative technologies to improve society. As artificial intelligence systems become prevalent within the field, integrating Responsible AI principles is imperative. AI technology should enhance human capabilities while preserving dignity and ethical standards.
Engineering Principles for Ethical AI
To ensure that AI systems are ethically sound, engineers follow a set of core principles during development. These principles help guide the design and deployment of AI systems so they align with societal values.
Ethical Design: This principle focuses on designing AI systems with moral and ethical considerations to avoid harming individuals or society.
Consider self-driving cars equipped with AI technologies. According to the principle of ethical design, these vehicles must prioritize pedestrian safety and follow traffic regulations to minimize accidents.
- Ensuring Equity in Access: AI systems should be accessible and beneficial to all, without discrimination based on socioeconomic status or geographic location.
- Balancing Autonomy and Control: While AI can function autonomously, engineers are responsible for ensuring human oversight to manage potential risks.
- Promoting Accountability: Engineers must develop mechanisms to track AI system outcomes and provide explanations in cases of errors or unintended consequences.
Remember, intentions behind building AI systems are just as important as their technological architecture.
Responsible Machine Learning Practices
Machine learning (ML) practices that uphold responsibility form the backbone of ethical AI deployment. Engineers and ML practitioners focus on aspects such as inclusivity, openness, and risk management to ensure solutions benefit society as a whole.
Bias Mitigation: This technique aims to identify and reduce biases within ML algorithms to achieve fair outcomes.
- Dataset Auditing: Regularly reviewing datasets for representativeness and balance helps prevent unintentional bias in ML models.
- Transparent Algorithms: Creating open-source code and clear documentation fosters trust and allows for community critique and improvement.
- Robustness Testing: Assessing model performance under various conditions ensures reliability and minimizes failure risks.
Imagine an ML model used for loan approvals. By adopting bias mitigation strategies, developers can ensure fair evaluation of applicants based on creditworthiness rather than unrelated demographic factors.
Exploring the depths of Bias Mitigation, you find techniques like Adversarial Debiasing, where adversarial networks learn to predict protected attributes inaccurately, thereby reducing model bias. Another approach is Re-weighting Data, where data points are assigned weights inversely proportional to their frequency, ensuring minority representation during training. With continuous advancements in bias mitigation, engineers can build fairer AI systems while preserving user trust.
Ethical AI Development
The development of AI systems comes with significant responsibilities to adhere to ethical practices. Ensuring that AI is developed with consideration for ethical standards is crucial for creating systems that respect human rights and promote social good. This section delves into the nuances of ethical AI standards and their implementation.
AI Ethics and Standards
AI ethics involve a comprehensive framework that guides developers in building systems that are beneficial, fair, and respectful of human values. These ethics usually encompass areas such as:
- Informed Consent - Ensuring users are aware of and agree to how their data is used by AI systems.
- Data Privacy - Protecting sensitive information against unauthorized access.
- Algorithmic Accountability - Assigning responsibility for decisions and outcomes attributed to AI models.
Algorithmic Transparency: This refers to the clarity and openness in how algorithms operate and make decisions, allowing stakeholders to understand and verify their functioning.
Consider a medical AI system that assists in diagnosing diseases. To meet ethical standards, it should be transparent in its decision-making process and provide comprehensive insights into data handling procedures.
Transparency is not only about revealing how AI works but also about communicating limitations and potential risks to users.
Responsible AI Explained
Responsible AI represents a commitment to harness AI technologies in ways that are ethical, accountable, and aligned with societal values. It emphasizes:
- Ensuring fairness and non-discrimination in AI applications.
- Consistent monitoring and evaluation of AI impact on society.
- A proactive approach to identifying and mitigating risks associated with AI deployment.
Responsible Use of AI: Involves operating AI technologies in ways that prioritize human welfare and ethical norms, often requiring active participation from various stakeholders such as developers, policymakers, and users.
In a smart city scenario, responsible AI could be employed to manage energy consumption efficiently, ensuring minimal environmental impact while respecting citizen privacy and autonomy.
Exploring deeper into Responsible AI, we find practices like Impact Assessments, which evaluate potential societal impacts before deploying AI systems. These assessments consider several factors:
- Short and long-term societal effects.
- Potential biases and their mitigation strategies.
- Feedback mechanisms for continuously improving AI systems.
Responsible AI Challenges in Engineering
As AI technologies evolve within the field of engineering, addressing challenges becomes increasingly important. Ensuring that AI systems are fair, transparent, and aligned with ethical values requires careful consideration and innovative solutions. Let's explore two primary challenges: addressing AI bias and ensuring transparency in AI systems.
Addressing AI Bias
AI bias emerges when algorithms reproduce or exacerbate existing prejudices present in data. This can lead to unfair outcomes, impacting decision-making processes in critical areas such as hiring, medical diagnosis, and more. Addressing AI bias involves several strategies:
- Data Diversification: Ensuring diverse and representative data sets help minimize bias in AI training.
- Algorithm Testing: Regularly testing algorithms against varied datasets can highlight potential biases and allow for timely adjustments.
- Bias Audits: Systematic reviews that examine AI systems to identify and mitigate bias in their outputs.
For example, an automated resume screening tool should accurately assess applicants based on their qualifications, free from bias related to name, ethnicity, or gender. By diversifying the training data and regularly auditing the system, developers can reduce bias and promote fair hiring practices.
In tackling AI bias, advanced methods such as Adversarial Debiasing have been developed. This approach involves training AI with a secondary model that attempts to predict sensitive attributes, thereby forcing the main model to minimize its reliance on biased data patterns. Techniques like this are helping to pave the way for more equitable AI systems across different sectors.
Ensuring Transparency in AI Systems
Transparency in AI systems is paramount to building trust and understanding among users. It involves highlighting how AI systems make decisions and exposing the logic behind these processes to users and stakeholders.
To achieve transparency, consider these strategies:
- Clear Documentation: Providing comprehensive information about AI algorithms, their development, and usage assists stakeholders in understanding decision-making processes.
- Explainable AI: Developing AI models that expound on their decisions enhances transparency and user trust. Techniques like SHAP and LIME are often utilized.
- Open-source Platforms: Making code and models publicly available invites community engagement and allows for external reviews and improvements.
In healthcare applications, it's essential that AI systems used for patient diagnosis are transparent and explainable. This allows healthcare professionals to understand the AI's recommendations, leading to more informed and confident decisions.
A fascinating development in AI transparency is the concept of Model Cards. These are documents that accompany AI models to detail their intended use, performance across different scenarios, ethical considerations, and limitations. Model Cards offer essential insights into AI systems, promoting informed deployment and usage decisions.
responsible ai - Key takeaways
- Responsible AI: Emphasizes fairness, transparency, accountability, and ethical alignment in AI systems, ensuring they operate beneficially and ethically.
- Fairness, Transparency, Accountability: Core principles of responsible AI ensuring minimal bias, system understandability, and mechanisms for liability.
- Responsible Machine Learning: Incorporates practices like bias mitigation, dataset auditing, and model transparency for ethical AI deployment.
- Engineering Principles for Ethical AI: Encourage equity, balance between autonomy and control, and promoting accountability in AI systems.
- AI Ethics and Standards: Involve informed consent, data privacy, and algorithmic accountability to create systems that respect human rights.
- Tools for Responsible AI: Include fairness toolkits, model explainability tools, and privacy-preserving frameworks to align AI with ethical standards.
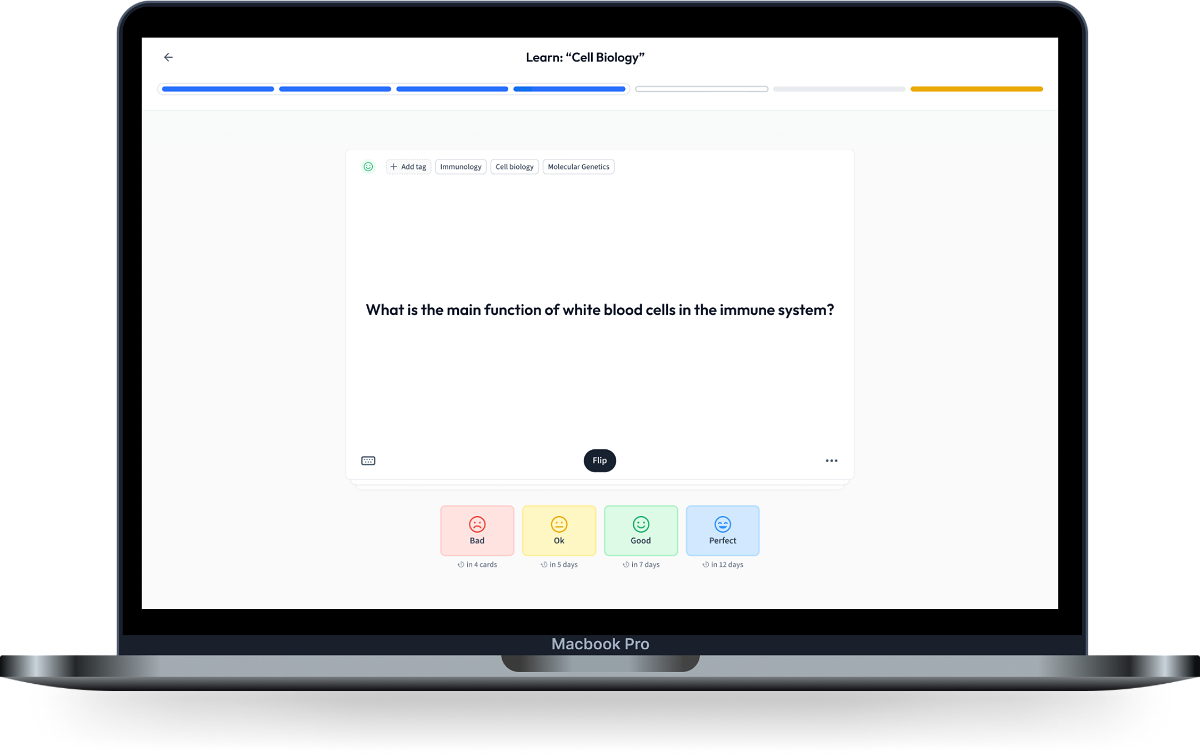
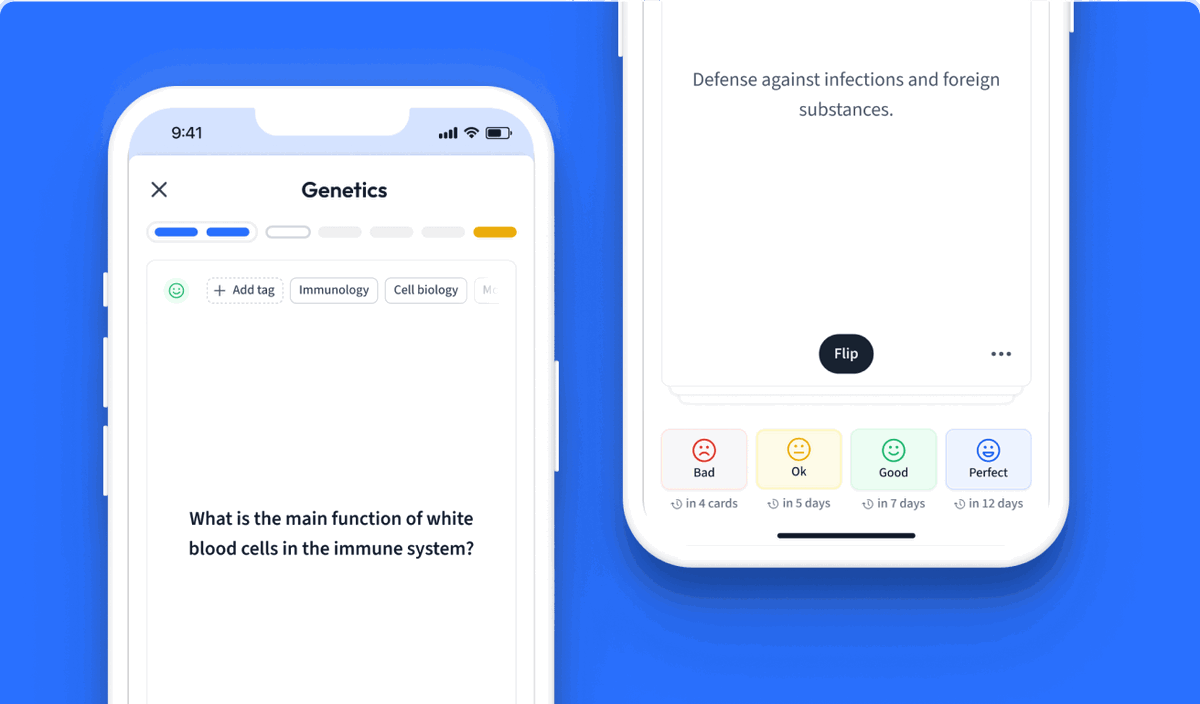
Learn with 12 responsible ai flashcards in the free StudySmarter app
We have 14,000 flashcards about Dynamic Landscapes.
Already have an account? Log in
Frequently Asked Questions about responsible ai
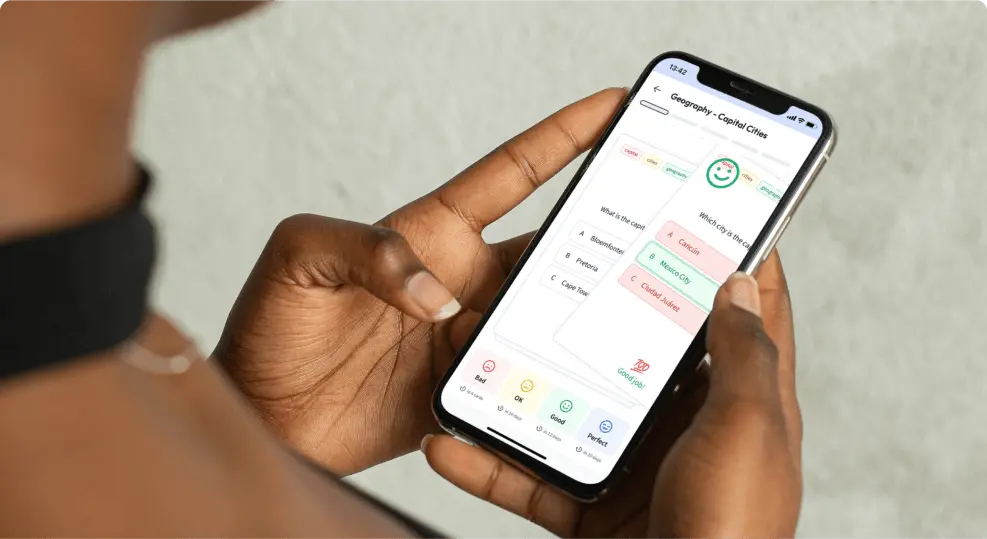
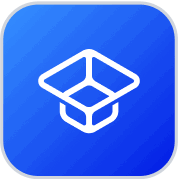
About StudySmarter
StudySmarter is a globally recognized educational technology company, offering a holistic learning platform designed for students of all ages and educational levels. Our platform provides learning support for a wide range of subjects, including STEM, Social Sciences, and Languages and also helps students to successfully master various tests and exams worldwide, such as GCSE, A Level, SAT, ACT, Abitur, and more. We offer an extensive library of learning materials, including interactive flashcards, comprehensive textbook solutions, and detailed explanations. The cutting-edge technology and tools we provide help students create their own learning materials. StudySmarter’s content is not only expert-verified but also regularly updated to ensure accuracy and relevance.
Learn more