Jump to a key chapter
Process Variability Definition
Process variability refers to the natural tendency of any process to exhibit changes over time, leading to variations in output. This is a critical concept in engineering and quality management, as it highlights the importance of consistently maintaining product quality and performance. Understanding and controlling process variability can help ensure that the quality of products remains within acceptable limits, which is crucial for both customer satisfaction and compliance with industry standards.
Importance of Process Variability in Engineering
In engineering, addressing process variability is vital to maintaining the reliability of a product. Variability can arise from several sources, including:
- Material properties - Differences in raw materials can lead to variations in product quality.
- Environmental factors - Changes in temperature or humidity can affect the dimensions of components.
- Human factors - Inconsistent handling or operation may introduce variability in the process.
Statistical Process Control (SPC) is a technique used to monitor, control, and reduce process variability by employing statistical methods to analyze process performance.
Consider a manufacturing process that involves assembling electronic components. If the soldering temperature varies, it may result in poor electrical connections, affecting the performance of the final product. By using Statistical Process Control (SPC) charts, engineers can monitor the soldering temperature, identify trends or abnormalities, and make necessary adjustments to maintain an optimal range, reducing process variability.
The analysis of process variability can be further explored using mathematical models and simulations. For instance, engineers use a method called Design of Experiments (DOE) to systematically change variables and observe the effects on the output. This approach provides insights into which factors significantly impact process variability.Furthermore, advanced statistical analyses, such as Analysis of Variance (ANOVA), can be conducted. This technique involves comparing the variance in a group of data points to distinguish meaningful differences between them. ANOVA tests the hypothesis that the means of different groups are equal, helping engineers determine if observed variabilities are statistically significant. The mathematical expression for ANOVA can be represented as:\[F = \frac{MS_{between}}{MS_{within}}\]where
- F: F-ratio
- MS_{between}: Mean square variance between samples
- MS_{within}: Mean square variance within samples
Causes of Process Variability
Understanding the causes of process variability is essential in managing and controlling it effectively. Identifying these factors can help in implementing strategies to minimize their impact on the process. Common causes include variations in materials, environmental conditions, human error, machine performance, and measurement techniques.
Material Variability
Materials used in any process can be a source of variability. Variations in the properties of raw materials, such as density, moisture content, or tensile strength, can significantly affect the final product.
- Density fluctuations may alter how materials are processed, impacting dimensional accuracy.
- A change in moisture content may lead to expansion or contraction, affecting the fit of assembled parts.
- Diverse tensile strengths could influence the durability and reliability of the product.
In the textile industry, variation in the thickness of yarn can lead to defects in the fabric. If the yarn becomes too thin, it may break during the weaving process, resulting in uneven textures. Monitoring yarn consistency through regular testing can help mitigate this type of process variability.
Environmental Factors
Environmental conditions can affect the stability and efficiency of processes. For instance, changes in temperature and humidity can cause:
- Expansion or contraction of materials - Components may not fit together correctly if they expand or contract beyond acceptable ranges.
- Alteration of chemical reactions - Reaction rates may increase or decrease with temperature fluctuations, affecting product quality.
Did you know? Ambient temperature can affect the viscosity of fluids used in hydraulic systems, leading to altered system responses.
Human Error
Human errors are prevalent causes of variability. They may result from:
- Lack of training - Inadequately trained personnel might misinterpret instructions or operate machinery incorrectly.
- Fatigue - Prolonged work hours can lead to decreased focus and increased error rates.
Machine Performance Variability
Machine performance variability arises due to wear and tear over time, leading to:
- Inconsistent outputs - This can stem from mechanical failure or imbalance.
- Calibration drift - Instruments may lose accuracy, affecting measurements.
Examining machine performance variability involves understanding the probabilistic nature of machine failures. Reliability engineering often uses the Weibull distribution to model the time until a machine component fails. This distribution helps in predicting failures and planning maintenance: The formula for the Weibull probability density function is given by:\[f(t; \lambda, k) = \frac{k}{\lambda} \left(\frac{t}{\lambda}\right)^{k-1} e^{-(t/\lambda)^k}\]where:
- t: time
- \lambda: scale parameter
- k: shape parameter
Examples of Process Variability
Examining examples of process variability in various scenarios helps you understand how it manifests and can be managed effectively. These examples demonstrate key challenges in different industries and showcase the practical application of control techniques.
Manufacturing Process Variability
In the context of manufacturing, process variability can lead to differences in product dimensions and performance. Consider a scenario where the assembly line speed in an automobile factory fluctuates due to unstable power supply. This can impact:
- The fit of vehicle components.
- The finish quality of paint applications.
A common example of manufacturing variability is seen in the production of plastic components. During the injection molding process, variations in the temperature of the mold can lead to inconsistencies in the thickness of the final product. By employing sensors to monitor and adjust mold temperature, manufacturers can effectively manage this variability.
Healthcare Process Variability
In healthcare, process variability might occur in the administration of medication due to slight differences in dosage or timing. This can affect patient outcomes, requiring:
- Consistent training of healthcare staff to ensure correct dosing.
- Implementation of automated dispensing systems to minimize human error.
The time of day at which medication is administered can influence its effectiveness, as metabolic rates vary throughout the day.
Service Industry Process Variability
Process variability in the service industry often revolves around customer experience. Variability can arise due to:
- Inconsistent customer service skills among employees.
- Variation in service delivery times.
A detailed analysis of variability often involves statistical modeling and hypothesis testing. In manufacturing, variance between sample products is evaluated to ensure quality control. The standard deviation, a key statistic in this context, measures variability in a dataset.The standard deviation (\
Techniques to Reduce Process Variability
Reducing process variability is crucial in ensuring consistent product quality and operational efficiency. Various techniques can be employed to manage these variations and maintain processes within desired specifications.
Process Capability Compares Process Variability to the Tolerances
Process capability analysis is a statistical method used to determine how well a process can produce output that meets specified limits. It compares the variability of the process output to the specified tolerances. Key metrics used in process capability analysis include:
- Cp (Process Capability Index) - Measures how well the process width fits within the tolerance limits. It is calculated as: \[Cp = \frac{USL - LSL}{6\sigma}\]where
- USL: Upper Specification Limit
- LSL: Lower Specification Limit
- \(\sigma\): Standard deviation of the process
- Cpk (Process Capability Index taking into account the process mean) - Indicates how centered the process is within the limits. Its formula is \[Cpk = \min \left(\frac{USL - \mu}{3\sigma}, \frac{\mu - LSL}{3\sigma}\right)\]where
- \(\mu\): Process mean
Imagine a process designed to fill bottles with 500ml of liquid. If the standard deviation is 5ml, the USL is 510ml, and the LSL is 490ml, the process capability \[Cp = \frac{510 - 490}{6 \times 5} = 0.67\]indicating the process may not meet requirements, prompting improvements.
Further exploration into process capability involves analyzing the distribution of process data and how it impacts variability perception.Engineers may also use control charts, such as X-bar and R charts, to detect shifts and trends over time. These charts help visualize the variability and pinpoint specific times when the process goes out of control.Another statistical tool, the Cumulative Distribution Function (CDF), shows the probability that a process measurement is within a certain limit. This is important for assessing the likelihood of producing units within the specified range. The general formula for CDF is \[F(x) = P(X \leq x)\] for random variable X.Understanding these techniques allows for proactive management of process variability, leading to enhanced control and stability in manufacturing and service settings.
Impact of Process Variability on Product Quality
Process variability can have a profound impact on product quality, potentially resulting in non-conforming products and affecting customer satisfaction. Key impacts include:
- Reduced Product Consistency - Variations in the process can lead to fluctuations in product dimensions and attributes.
- Increased Scrap Rates - High variability may result in more defects, leading to wastage and increased production costs.
- Customer Dissatisfaction - Inconsistent product performance can tarnish brand reputation, leading to a loss of market share.
A successful approach in reducing product variability involves adopting a Lean Six Sigma methodology to systematically eliminate sources of variation and improve process outcomes.
process variability - Key takeaways
- Process Variability Definition: The natural tendency of a process to change over time, affecting output consistency, crucial in maintaining product quality and performance.
- Causes of Process Variability: Variability arises from material properties, environmental factors, human errors, machine performance, and measurement techniques.
- Examples of Process Variability: Variations in manufacturing, such as fluctuating temperatures affecting mold thickness, and healthcare dosing impacting patient outcomes.
- Techniques to Reduce Process Variability: Use of tools like Statistical Process Control (SPC), Design of Experiments (DOE), and control charts to monitor and minimize variability.
- Process Capability Compares Process Variability to the Tolerances: Statistical analysis using indexes like Cp and Cpk to measure and ensure process output meets specification limits.
- Impact of Process Variability on Product Quality: Can lead to reduced product consistency, increased scrap rates, and customer dissatisfaction, highlighting the need for variability management.
Learn faster with the 12 flashcards about process variability
Sign up for free to gain access to all our flashcards.
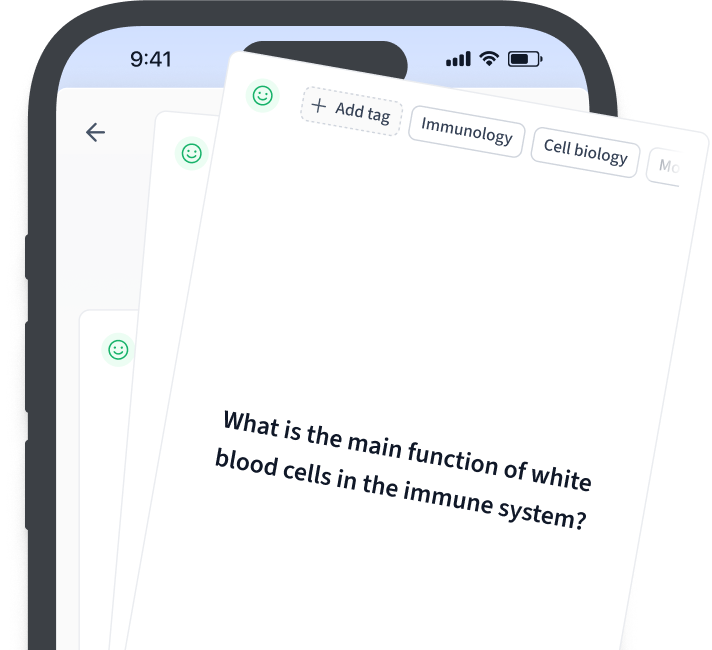
Frequently Asked Questions about process variability
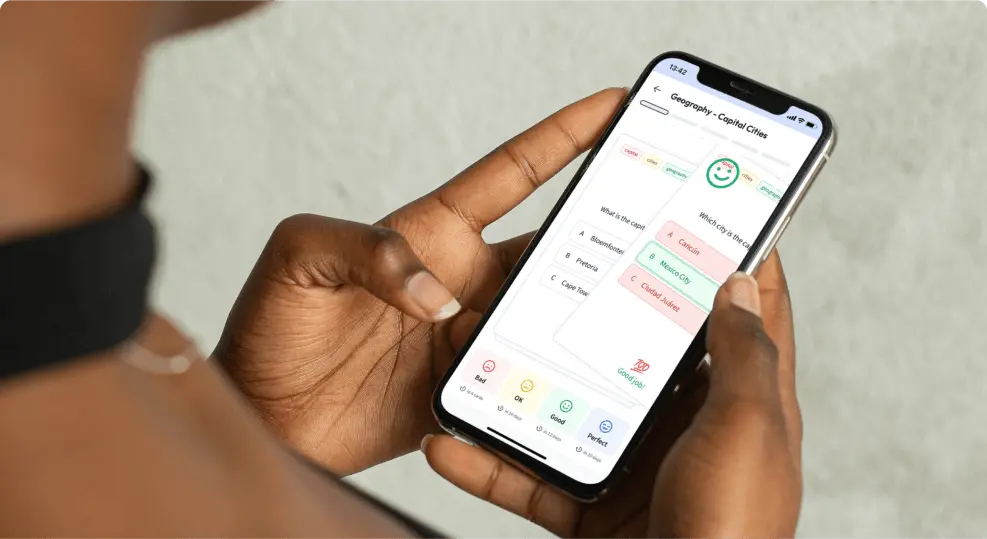
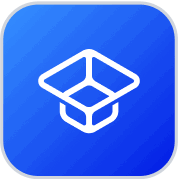
About StudySmarter
StudySmarter is a globally recognized educational technology company, offering a holistic learning platform designed for students of all ages and educational levels. Our platform provides learning support for a wide range of subjects, including STEM, Social Sciences, and Languages and also helps students to successfully master various tests and exams worldwide, such as GCSE, A Level, SAT, ACT, Abitur, and more. We offer an extensive library of learning materials, including interactive flashcards, comprehensive textbook solutions, and detailed explanations. The cutting-edge technology and tools we provide help students create their own learning materials. StudySmarter’s content is not only expert-verified but also regularly updated to ensure accuracy and relevance.
Learn more