Jump to a key chapter
Entropy Function in Engineering Thermodynamics
The entropy function is a fundamental concept in the field of engineering thermodynamics. It's a core tool in understanding the behaviour of energy transformations and in assessing systems' efficiency. To get a solid grip on the topic, knowledge of its definition and application is essential. Additionally, mastering the entropy function technique can greatly enhance your problem-solving skills in thermodynamics.
Understanding the Entropy Function Definition
The science of thermodynamics examines how energy transfers and transforms. The entropy function is a crucial tool in this field, representing the randomness or disorder within a system. The more chaotic the system, the higher the entropy.
Basic Definition of Entropy Function
Entropy function, symbolised by 'S', is the measurement of a system's thermal energy per unit temperature that is unavailable for doing useful work. In thermodynamics, the formula for entropy is typically given as \[dS = \frac{dQ_r}{T}\] where \(dS\) is the infinitesimal increase in entropy, \(dQ_r\) is the infinitesimal amount of heat added to the system at a constant temperature, and \(T\) is the absolute temperature.
Importance and Application of Entropy Function
The entropy function is important in virtually every area of thermodynamic analysis. Its key role includes:
- Elucidating principles of energy conservation
- Understanding energy dissipation and efficiency of systems
- Examining stability and feasiblity of thermodynamic processes
- Quantifying disorder and randomness
Entropy's application in heat engines is a prime example of its importance. In heat engines, the increase in entropy of a system signifies that not all energy can be converted into useful work, leading to heat loss. This forms the basis of the second law of thermodynamics, highlighting entropy's central role in the study of energy transformations.
Mastering the Entropy Function Technique
To utilise the entropy function effectively, you need to grasp key concepts and apply them in practical situations. This involves understanding entropy's relationship with other thermodynamic properties and how to adjust entropy calculations for real-world applications.
Key Concepts in the Entropy Function Technique
There are several key concepts central to calculating entropy:
- The entropy change of a closed system is zero for reversible processes, but increases for irreversible processes.
- Entropy is a state function, meaning its value only depends on the state the system is in, not how it got there.
- The entropy of a pure, perfectly ordered crystalline substance is zero at absolute zero temperature. This is the so-called Third Law of Thermodynamics.
Applying the Entropy Function Technique in Engineering Thermodynamics
Let's consider an example: A 2 kg block of copper at an initial temperature of 100°C is placed in contact with a 1 kg block of aluminium at 20°C. Assuming no heat is lost to the surroundings, we can calculate the final equilibrium temperature and the entropy change in this process using thermodynamic properties and the entropy function technique.
Using integral calculus and data tables, one can solve for final temperature, \( T_f \), and entropy change, \( \Delta S \), in this problem. This gives a clear demonstration of the entropy function technique, showing how it links abstract principles of thermodynamics to real-world applications.
Practical Insights into the Entropy Function
The entropy function isn't confined to textbooks and academic discussions. You'll be amazed to know that its implications are visible in everyday life and professional settings. So, while you may have understood probably the technicalities and conceptual underpinnings of this thermodynamic function, comprehending its practical manifestations can enrich your understanding and boost your skill set in applying this function to solve complex engineering problems.
Delving into Real-Life Entropy Function Examples
From melting ice cream to charging your phone, the entropy function's influence is all around us. These everyday examples provide an exciting, tangible approach to understanding this theoretical concept.
Entropy Function Examples in Everyday Life
Let's consider a few fascinating instances of the entropy function at work in our everyday lives:
- Melting Ice: When an ice cube melts, it changes phase from a well-ordered, crystalline solid to a more chaotic liquid, thereby increasing its entropy. This is reflective of the tendency of natural systems to move towards higher entropy states.
- Coffee Mixing: If you pour milk into coffee and let it mix naturally, the milk's dispersion throughout the coffee is a process of increasing entropy.
- Charging Phones: When you charge your phone, electrical energy is converted into chemical energy stored in the battery. However, not all the energy is usefully converted; some is wasted as heat due to the increase in entropy.
Entropy Function Examples in Professional Settings
In professional realms, the entropy function is frequently employed, specifically in fields like mechanical engineering, chemical engineering, electrical engineering, and even informatics. Below are a few illustrations:
- Power Plants: Engineers at power plants try to minimize entropy increase to maximize power output. They use entropy function calculations to evaluate their systems' efficiency.
- Air Conditioning Systems: In the design and efficiency assessment of HVAC systems, the entropy function plays a crucial role. It helps determine the change in entropy throughout the system to ensure it operates at optimal efficiency.
- Data Compression: In computer science, a variant of entropy is used to evaluate information density and is crucial in designing data compression algorithms. The higher the entropy, the less redundancy is present, and the more efficient the compression.
Entropy Function Formula Derivation in Detail
To confidently apply the entropy function in practice, you need an in-depth understanding of how its formula is derived. Doing so not only reinforces your comprehension of the function itself but also gives you a foundation to tackle more complex problems relating to entropy.
Understanding the Concept Behind the Entropy Function Formula Derivation
Before stepping into the derivation process, it's vital to grasp the fundamental notion behind the entropy function's formula. As discussed, entropy is about the degree of disorder or randomness within a thermodynamic system. It quantifies the part of a system's energy that is unable to do work, and it adheres to the principles of the second law of thermodynamics. In essence, the entropy function's formula encapsulates these principles mathematically.
Steps to Perform an Entropy Function Formula Derivation
While there are several ways to derive the entropy function formula, one of the most common methods uses the principles of heat transfer and the concept of a reversible process.
- Imagine a small amount of heat \(dQ\) being added to a system at a uniform temperature \(T\).
- For a reversible process, the entropy change \(dS\) is given by \[dS = \frac{dQ_{rev}}{T}\] where \(dQ_{rev}\) is the heat added reversibly to the system.
- To find the total entropy change for a process, integrate \(dS\) from the initial to the final state. This gives the formula for the change in entropy, \[ \Delta S = \int_{S1}^{S2} dS = \int_{Q1}^{Q2} \frac{dQ_{rev}}{T} \]
In conclusion, mastering the theory and practical implications of the entropy function not only aids your understanding of thermodynamics but also prepares you for real-world applications, which we trust, would bolster your future engineering endeavours.
Bringing Together the Aspects of Entropy Function
Having discussed the myriad facets of the entropy function, now is a good time to draw together these threads and solidify understanding of them. From the concept's definition and technique to its practical applications and formula derivation, you've covered a vast set of entropy-related knowledge.
Recap: Entropy Function Definition and Technique
The entropy function is central to thermodynamics, measuring a system's thermal energy per unit temperature that is unavailable for doing work. It represents the quantity of randomness or disorder within a system. The mathematical technique to calculate entropy hinges on understanding its incremental change \(\Delta S\) which, for a reversible process, is formulated as \(dS = \frac{dQ_{rev}}{T}\).
Key Takeaways: Definition and Technique
Briefly summarising, entropy, denoted by 'S', quantifies a system's disorder or randomness. It's a pivotal concept in thermodynamics and sheds light on the principles of energy conservation, system efficiency, and process feasibility:
- The entropy change, \( \Delta S \), is zero for a reversible process, but increases for any irreversible activity.
- Entropy is a state function that depends solely on the current state of the system, not the path taken to reach there.
- The Third Law of Thermodynamics posits that a perfectly ordered pure crystalline substance possesses zero entropy at absolute zero temperature.
Challenges and Solutions in Entropy Function Technique
Certain complications could arise in applying the entropy function technique. A common hurdle is the practical understanding and application of entropy's definition to real-world problems. Another challenge may be interpreting entropy changes in a system and linking these changes to the function's core principles.
Addressing these challenges primarily involves deep dives into the theoretical principles and numerous practice sessions with different types of entropy problems. Another approach could be studying real-world systems and their entropy changes to understand the practical implications of the entropy function. Working on diverse entropy-related calculations will help you build comprehension and hone problem-solving skills.
Recap: Entropy Function Examples and Formula Derivation
In terms of practical manifestations of the entropy function, examples can be found everywhere — from the melting of ice to the charging of phones or in professional settings like power plants or air conditioning systems. The mathematical derivation of the entropy function formula delivers solid knowledge about how the formula encapsulates the principles of the second law of thermodynamics.
Key Takeaways: Examples and Formula Derivation
Practical examples of entropy function not only provide a slogan comprehension of the topic but also make it interesting. As we dealt with, entropy is observable in daily events like melting ice, mixing coffee, or charging phones. Its professional implicants include energy efficiency assessments in power plants or HVAC systems.
The entropy function formula is derived from the fundamental heat transfer principles and the concept of reversible processes. It's expressed as \( \Delta S = \int_{S1}^{S2} dS = \int_{Q1}^{Q2} \frac{dQ_{rev}}{T} \), signifying the part of the system's energy that is unusable for work due to increase in randomness or disorder.
Potential Hurdles and Coping Strategies in Using Entropy Function Formula Derivation
Understanding the derivation of the entropy function formula could pose significant challenges, particularly in handling the integral calculus part or in grasping the contextual sense of the formula. On that note, a problem-focused study coupled with consistent practice can help simplify the learning process.
Take small, steady steps moving from simpler to more complex derivations. Turn to digital learning resources, simulation modules, and educational software to visualise entropy in action in different thermodynamic processes. Also, don't hesitate to seek guidance from experienced tutors or peers when obstacles arise — remember, even the most complex concepts can be mastered with patience and perseverance.
Entropy Function - Key takeaways
- The entropy function is a key concept in the field of engineering thermodynamics essential for understanding the behaviour of energy transformations.
- Entropy function, represented by 'S', measures a system's thermal energy per unit temperature unavailable for doing useful work, with the formula in thermodynamics given as \[dS = \frac{dQ_r}{T}\]
- Entropy change of a closed system is zero for reversible processes and increases for irreversible processes. It is a state function dependent only on the state of the system and is zero at absolute zero temperature for a pure, perfectly ordered crystalline substance (Third Law of Thermodynamics).
- Practical examples of the entropy function occur in everyday life and professional settings: from melting ice, and coffee mixing to power plants and air conditioning systems.
- The entropy function formula is derived using the principles of heat transfer and the concept of a reversible process, expressed as \[ \Delta S = \int_{S1}^{S2} dS = \int_{Q1}^{Q2} \frac{dQ_{rev}}{T} \]
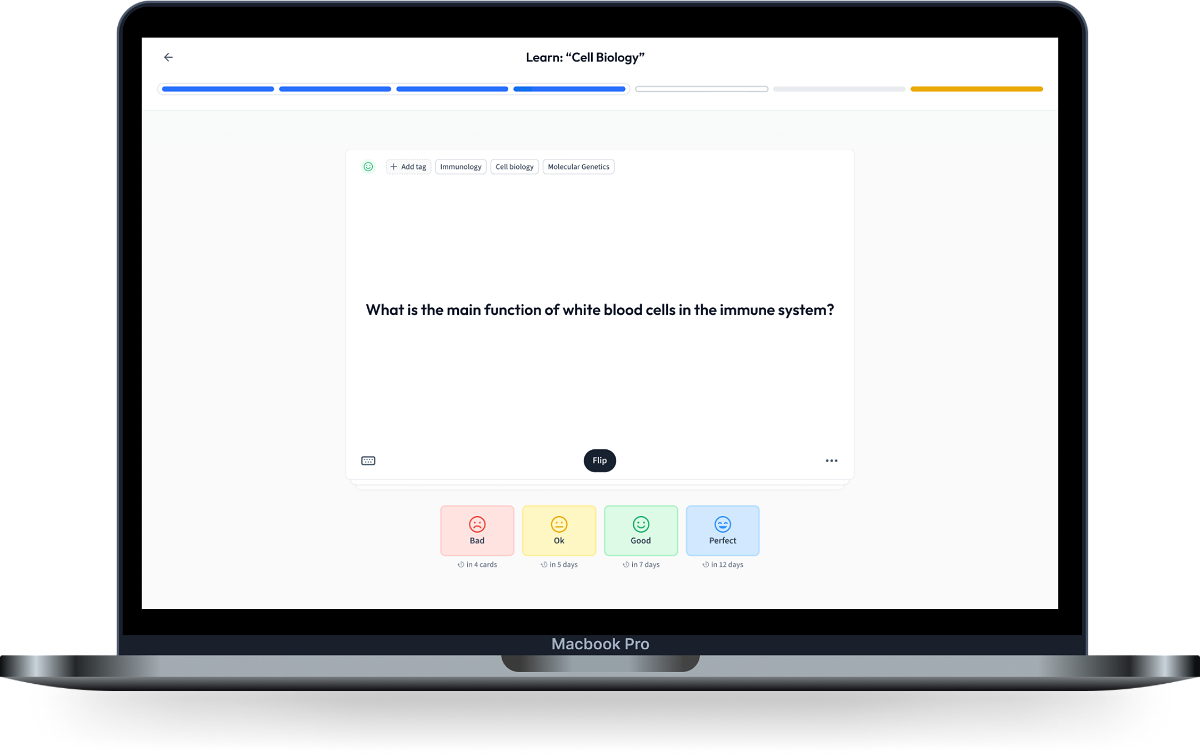
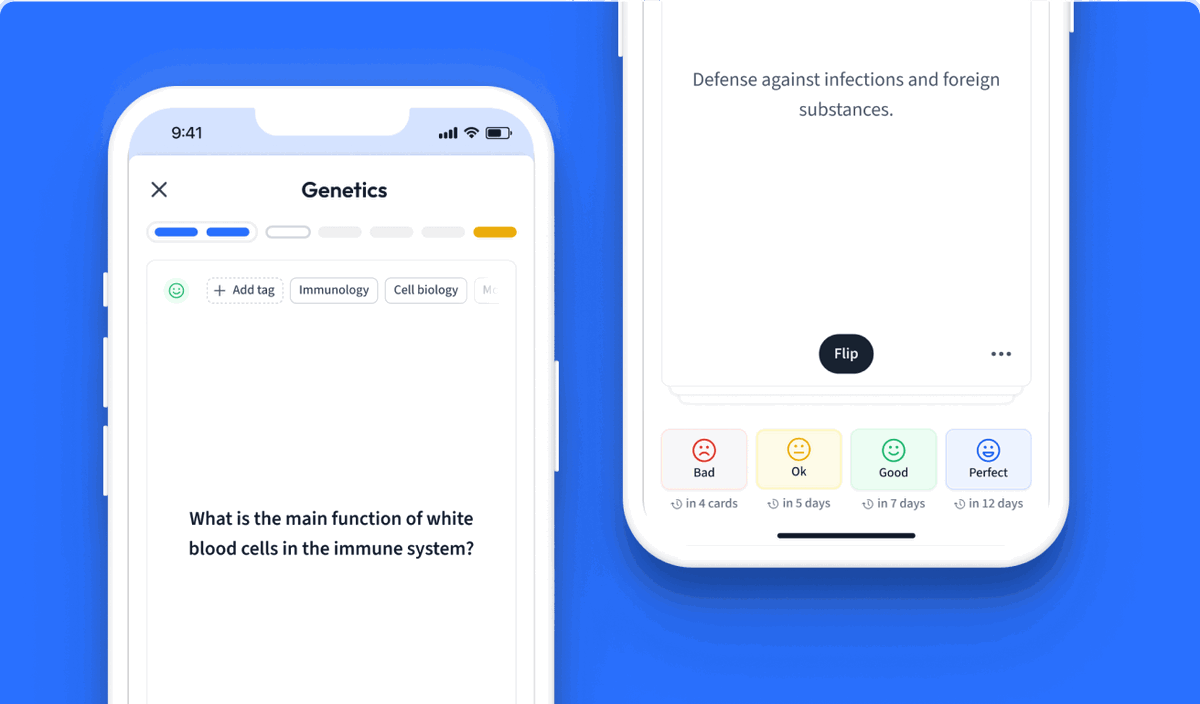
Learn with 12 Entropy Function flashcards in the free StudySmarter app
Already have an account? Log in
Frequently Asked Questions about Entropy Function
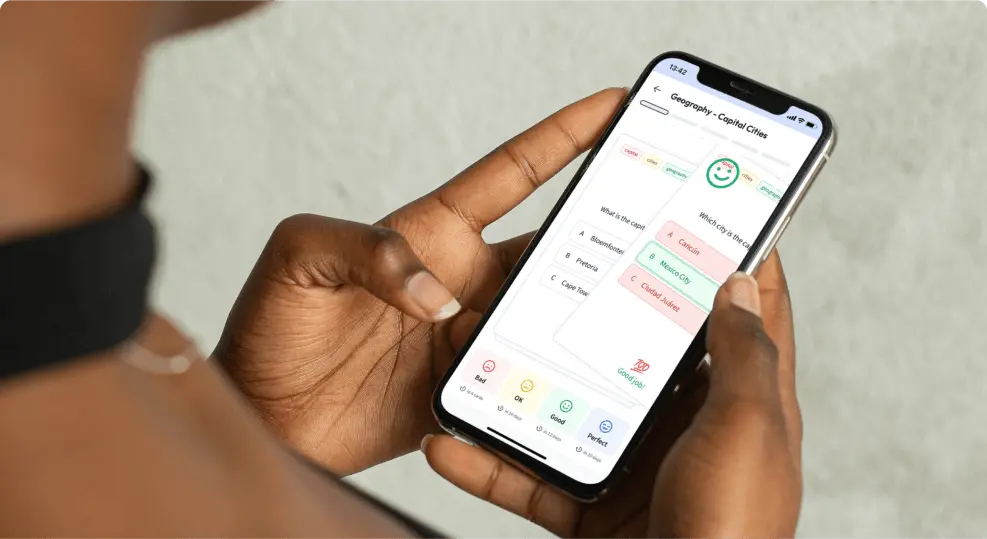
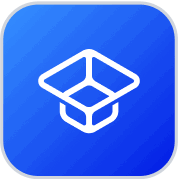
About StudySmarter
StudySmarter is a globally recognized educational technology company, offering a holistic learning platform designed for students of all ages and educational levels. Our platform provides learning support for a wide range of subjects, including STEM, Social Sciences, and Languages and also helps students to successfully master various tests and exams worldwide, such as GCSE, A Level, SAT, ACT, Abitur, and more. We offer an extensive library of learning materials, including interactive flashcards, comprehensive textbook solutions, and detailed explanations. The cutting-edge technology and tools we provide help students create their own learning materials. StudySmarter’s content is not only expert-verified but also regularly updated to ensure accuracy and relevance.
Learn more