Jump to a key chapter
Definition of Adversarial Networks
Adversarial Networks have become an essential concept in the field of machine learning. At their core, they are set up to simulate the interaction between two neural networks, namely the generator and the discriminator, to improve the performance and robustness of models. This interaction aims to enhance the model's ability to handle unexpected or novel inputs.
What Are Adversarial Networks?
Adversarial Networks can be understood as a framework where two neural networks engage in a battle of wits. The generator tries to create data that can fool the discriminator, while the discriminator works to distinguish between real data and the generated data. This competitive process continues until the generator produces data indistinguishable from the real data.
Adversarial Networks are machine learning models composed of two neural networks in a constant process of learning to improve data generation and validation.
Here's how the interaction generally functions:
- The Generator crafts fake data from random noise.
- The Discriminator evaluates data authenticity, determining if it's real or generated.
Imagine an art forger and an art expert. The forger creates counterfeit art, while the expert tries to identify fake pieces. As both improve their skills, the forger becomes better at creating indistinguishable replicas, similar to how the generator improves in adversarial networks.
In adversarial networks, especially the popular Generative Adversarial Networks (GANs), the generator can be universally represented by a differentiable function \( G(z; \theta_g) \). Here, \( \theta_g \) signifies the parameters adjusted during the training process to minimize the maximum loss. The discriminator is represented as \( D(x; \theta_d) \), where \( \theta_d \) are its respective parameters. The primary goal in training these networks is the minimization of the adversarial loss function:\[ \text{min}_G \text{max}_D V(D, G) = \mathbb{E}_{x \sim p_{data}(x)} [\log D(x)] + \mathbb{E}_{z \sim p_z(z)} [\log (1 - D(G(z)))] \]This formula illustrates the dynamic balancing act between the generator and discriminator's objectives, where the generator aims to minimize the equation and the discriminator seeks its maximization.
Generative Adversarial Networks in Engineering
Generative Adversarial Networks (GANs) are revolutionizing various fields of engineering. Their ability to generate realistic data from seemingly random inputs can be applied in processes like image synthesis, data augmentation, and more, opening new horizons for innovation.
Applications of Adversarial Networks in Engineering
Adversarial Networks hold immense potential in engineering, primarily because of their capacity to simulate and model complex environments. Here are some key applications:
- Image Generation: GANs are used to create high-resolution images, which can be useful in designing prototypes and virtual reality applications.
- Data Augmentation: They provide additional simulated data that help improve the machine learning models' accuracy and robustness.
- Anomaly Detection: Generative models excel in spotting anomalies in datasets, crucial for quality control in engineering processes.
Consider an engineering project aimed at designing new automotive body parts. GANs can generate multiple design variations that maintain aerodynamics and style, accelerating the development process and reducing costs.
A Generative Adversarial Network (GAN) is a class of machine learning frameworks where two neural networks contest with each other to generate realistic outputs from random inputs.
Mathematically, GANs aim to solve a two-player minimax game. Hardcoding your neural networks in a language like Python, you might start by defining the generator and discriminator as follows:
'import torch' 'import torch.nn as nn' 'class Generator(nn.Module):' ' def __init__(self, z_dim):' ' super(Generator, self).__init__()' ' # Define model layers here' 'class Discriminator(nn.Module):' ' def __init__(self):' ' super(Discriminator, self).__init__()' ' # Define model layers here'GANs seek to minimize the function:\[ \min_G \max_D V(D, G) = \mathbb{E}_{x \sim p_{data}(x)} [\log D(x)] + \mathbb{E}_{z \sim p_z(z)} [\log (1 - D(G(z)))] \]This expresses the competition between the generator G and the discriminator D, where the generator tries to optimize its parameters to produce as realistic data as possible, fooling the discriminator.
GANs are particularly efficient when there is limited data availability, as they can artificially increase the dataset with generated examples.
Generative Adversarial Network Architecture
The Generative Adversarial Network (GAN) architecture consists of two primary components: the generator and the discriminator. This architecture enables a dynamic interaction between these components to create and verify data authenticity effectively.
Key Components of GAN Architecture
Understanding the GAN architecture involves breaking down its essential parts:
- The Generator Network attempts to produce realistic data from seemingly random input, known as latent space.
- The Discriminator Network, on the other hand, evaluates the data it receives to categorize it as authentic or fake, guiding the generator's learning process.
Think of the GAN architecture as a competitive game between two artificial intelligence agents. The generator is like a painter trying to create realistic paintings, while the discriminator acts as an art critic, distinguishing between genuine artwork and fake paintings.
To delve deeper into the GAN architecture, consider the mathematical formulation of their objectives. The generator aims to transform random noise \( z \) into data \( G(z) \), creating outputs that deceive the discriminator. Meanwhile, the discriminator uses an input \( x \) to calculate the probability \( D(x) \) that \( x \) is real versus generated.The generator and discriminator work through a minimax game described by the loss function:\[ \min_G \max_D V(D, G) = \mathbb{E}_{x \sim p_{data}(x)} [\log D(x)] + \mathbb{E}_{z \sim p_z(z)} [\log (1 - D(G(z)))] \]This loss function ensures a balance between the networks, with the generator improving its ability to fool the discriminator over time.
GANs can be prone to instability. Adjustments in the architecture, such as adding noise or using batch normalization, often help stabilize training.
The significance of using these two networks lies in their respective abilities to reinforce and challenge each other. Within a feedback loop, the generator improves by producing better fake samples, while the discriminator gets better at detecting fakes. This loop continues until the generator creates outputs that are indistinguishable from real data, indicating a successful training process.
Conditional Generative Adversarial Network
The Conditional Generative Adversarial Network (cGAN) expands on the traditional GAN framework by conditioning the generation process on additional information. This added layer of data enables more tailored and context-specific outputs compared to the generic scope of standard GANs, making it particularly effective in fields requiring detailed customization.
A Conditional Generative Adversarial Network (cGAN) is a type of GAN where both the generator and discriminator receive additional information, enabling data generation based on specific conditions.
Imagine building personalized avatars in a video game. By leveraging cGANs, you can generate avatars that match specific user characteristics, such as hair color or style, by conditioning on these features.
The work of a cGAN involves augmenting both the generator and discriminator inputs with conditional information \( y \). The generator aims to create data \( G(z|y) \), tailored to the condition \( y \). Likewise, the discriminator checks real data \( x \) conditioned by \( y \), calculating the probability \( D(x|y) \) that \( x \) is real given \( y \).The conditional GAN loss function is expressed as: \[ \min_G \max_D V(D, G) = \mathbb{E}_{x \sim p_{data}(x|y)} [\log D(x|y)] + \mathbb{E}_{z \sim p_z(z)} [\log (1 - D(G(z|y)))] \] This reformulation ensures that the generator learns to synthesize data coherent with the context provided by \( y \), producing more relevant and useful outputs.
Wasserstein Generative Adversarial Networks
Wasserstein Generative Adversarial Networks (WGANs) introduced a significant change in how GANs are trained by adopting a different approach to measure the distance between the distributions of real and generated data. This method yields more stable training processes and allows for improved evaluation of model convergence beyond binary classification.
Consider the challenge of aligning the generation of financial data to meet market patterns. WGANs help maintain this alignment by consistently adjusting the difference in distributions, improving the model's ability to mimic real-world data.
Unlike traditional GANs, WGANs replace the simple classification loss with the Wasserstein distance, often referred to as the Earth Mover's distance. This metric provides a more meaningful measure of how generated data diverges from real data. The core purpose is to optimize the model with:\[ \min_G \max_{D \in \mathcal{D}} \mathbb{E}_{x \sim p_{real}} [D(x)] - \mathbb{E}_{z \sim p_z(z)} [D(G(z))] \]Here, \( \mathcal{D} \) denotes the set of 1-Lipschitz functions, imposing a constraint to ensure the discriminator's gradients to remain within certain bounds—addressed through careful design of the discriminator's architecture, including weight clipping and gradient penalty techniques.
WGANs require fewer hyperparameter tuning, making them more accessible compared to traditional GANs in practical applications.
Applications of Adversarial Networks in Engineering
Adversarial Networks have garnered attention across disciplines within engineering due to their ability to innovate data generation methods. They have been particularly impactful in areas requiring high-fidelity data simulation, rare-event scenario modeling, and process optimization.
In engineering contexts, Adversarial Networks utilize competitive neural network structures to generate, enhance, and validate complex models effectively.
One of the pivotal roles of adversarial networks is in enhancing simulation models. Standard engineering simulations can benefit significantly from adversarial approaches by:
- Enhancing Predictive Maintenance: By generating synthetic time series data, GANs can improve early detection of component failures. The expanded dataset helps train machine learning models with higher accuracy in predicting maintenance needs.
- Augmenting Structural Analysis: In civil engineering, adversarial networks simulate stress-testing scenarios on materials, assisting in assessing structural integrity under various conditions.
adversarial networks - Key takeaways
- Adversarial Networks: Machine learning models composed of two neural networks engaging in competition to improve data generation and validation.
- Generative Adversarial Networks (GANs): A framework where a generator creates fake data to fool a discriminator, which classifies data as real or fake.
- Wasserstein Generative Adversarial Networks (WGANs): Improve GAN training by using the Wasserstein distance to gauge the variance between real and generated data distributions.
- Generative Adversarial Network Architecture: Comprises a generator creating data from latent space and a discriminator evaluating data authenticity.
- Conditional Generative Adversarial Network (cGAN): A type of GAN where generation is influenced by additional context-related information for tailored outputs.
- Adversarial Networks in Engineering: Employed in image generation, data augmentation, and anomaly detection for enhanced simulations and process optimization.
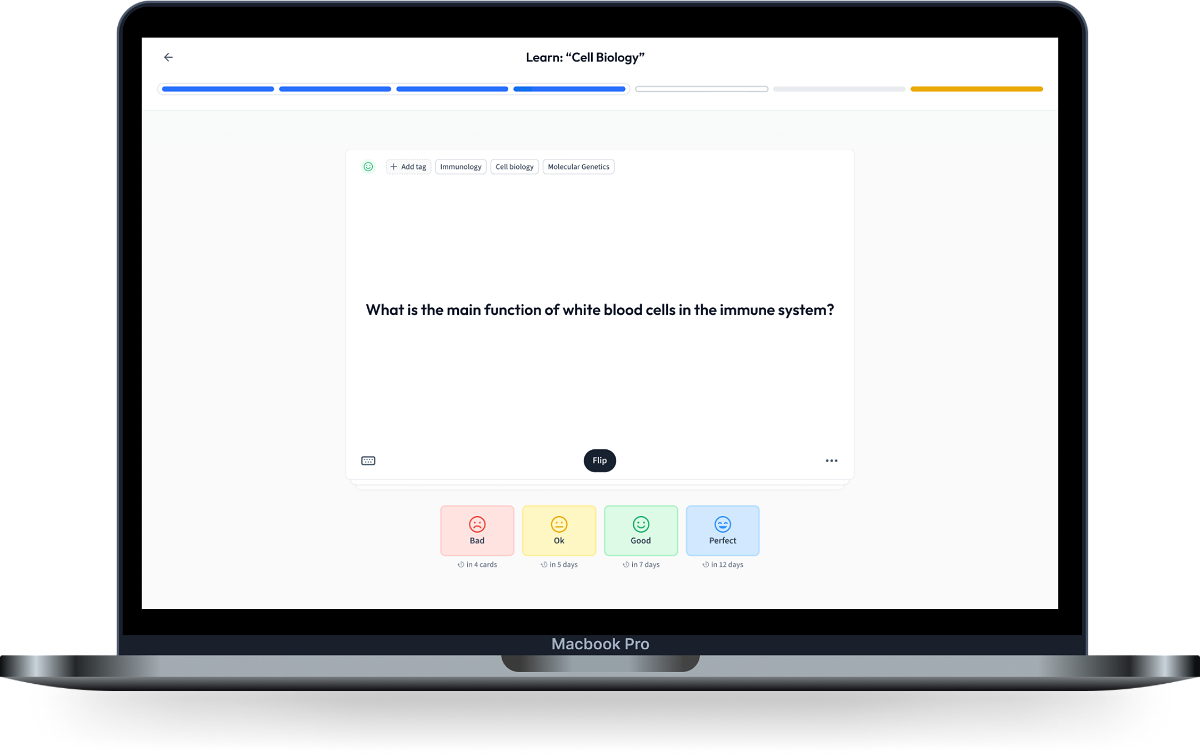
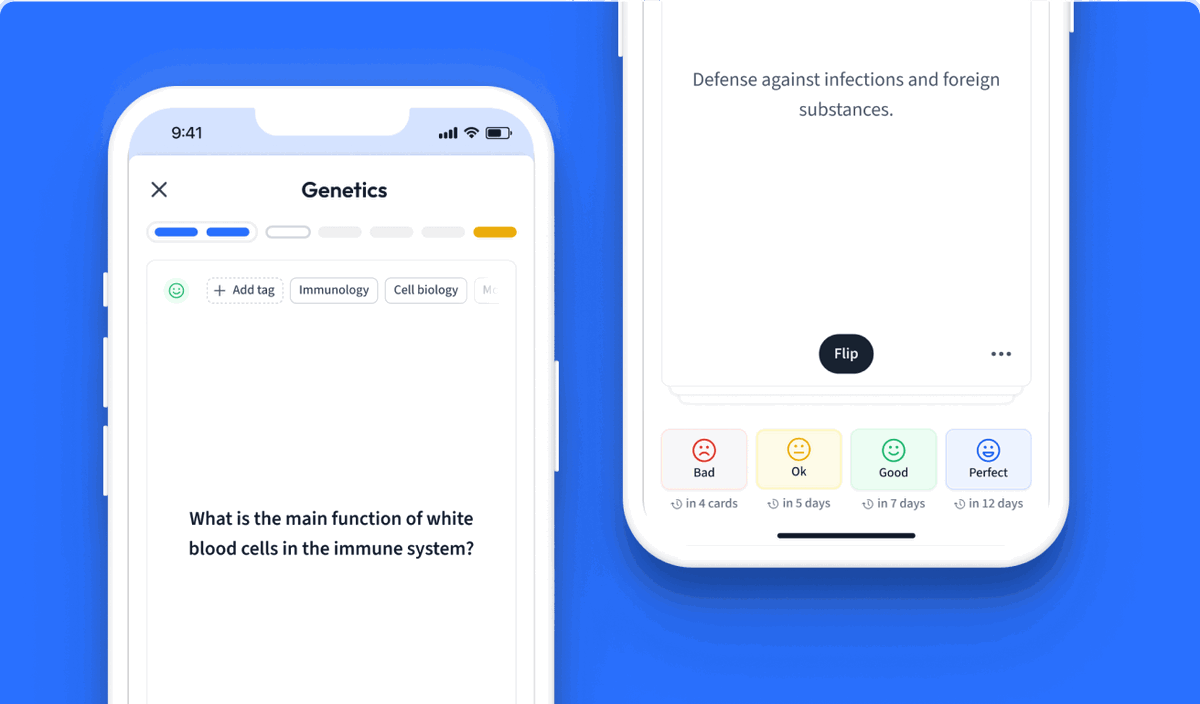
Learn with 12 adversarial networks flashcards in the free StudySmarter app
We have 14,000 flashcards about Dynamic Landscapes.
Already have an account? Log in
Frequently Asked Questions about adversarial networks
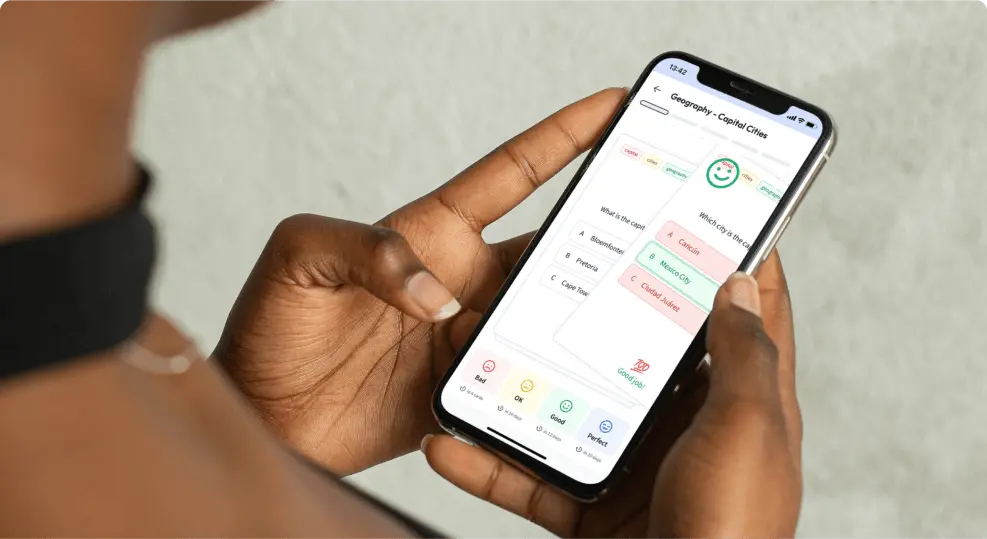
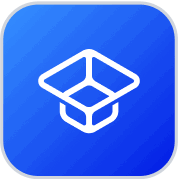
About StudySmarter
StudySmarter is a globally recognized educational technology company, offering a holistic learning platform designed for students of all ages and educational levels. Our platform provides learning support for a wide range of subjects, including STEM, Social Sciences, and Languages and also helps students to successfully master various tests and exams worldwide, such as GCSE, A Level, SAT, ACT, Abitur, and more. We offer an extensive library of learning materials, including interactive flashcards, comprehensive textbook solutions, and detailed explanations. The cutting-edge technology and tools we provide help students create their own learning materials. StudySmarter’s content is not only expert-verified but also regularly updated to ensure accuracy and relevance.
Learn more