Jump to a key chapter
Computational Microstructure Definition and Examples
The concept of computational microstructure involves the use of computational techniques to study and simulate the structures found in materials. These structures are often on a microscopic scale, influencing the material properties immensely. By employing modeling and simulation methods, you can gain insights into material behavior without the exhaustive need for physical experiments.
Computational Microstructure Explained for Students
To truly grasp the idea of computational microstructure, it's essential to first understand what a microstructure is. A microstructure refers to the small-scale structure of a material, typically observed through a microscope. The arrangement and size of these structures determine the material's mechanical and physical properties.Computational microstructure involves using computers to simulate these microscopic structures. Through this simulation, you visualize and predict how materials behave under different conditions. This process often involves complex algorithms and heavy computations, but they offer significant advantages, such as:
- Avoiding expensive and time-consuming experimental setups.
- Able to explore theoretical materials that can't currently be produced.
- Providing detailed insights into the role different microstructures play in material properties.
Computational microstructure: It is the study and simulation of materials' microscopic structures using computational methods.
When exploring computational microstructures, remember that changes at the microscopic level can significantly impact macroscopic properties.
In computational microstructure analysis, models often use the finite element method (FEM) to solve complex equations. FEM divides a large system into smaller, simpler parts called finite elements, which are easier to analyze. These calculations help predict how a microstructure might deform, heat up, or react under specific conditions. This insight is crucial for applications across aerospace, automotive, and biomedical industries.
Practical Examples of Computational Microstructure
Computational microstructures are vital in various applications. Let's delve into some practical examples:1. **Materials Design**: Engineers use simulation to design new alloys with specific properties, such as corrosion resistance or increased strength. For instance, by adjusting parameters within a simulated microstructure, you can predict the response of a new alloy under stress.2. **Battery Development**: In optimizing battery materials, like lithium-ion batteries, simulations can predict ion movement and arrangement within the material. This aids in designing batteries with better charge capacity and lifespan.3. **Biomaterials**: In developing materials safe for implants, computational simulations help analyze how microstructures impact material compatibility within the human body.These simulations provide a virtual testing ground, effectively minimizing risks and improving material efficacy. Remember, these examples are just the tip of the iceberg; numerous industries are benefiting from computational microstructure research.
Imagine you're developing a lightweight but strong alloy for a new car model. Using computational microstructure, you simulate the alloy's microstructure and its response to forces like torsion and compression. By tweaking the virtual microstructure, you find a composition that strikes the right balance of strength and weight, avoiding costly prototyping and testing stages.
The precision of simulations heavily depends on the mathematical models you use and the computing power available.
Applications of Computational Microstructure in Engineering
Computational microstructure plays a vital role in modern engineering by enabling the simulation of material behavior without the necessity for physical testing. This technology contributes significantly to advancing various engineering fields.Its applications extend across numerous sectors, providing profound insights into material properties and aiding in innovative design solutions.
Real-World Engineering Applications
In engineering, computational microstructure is instrumental in reducing costs and accelerating development cycles. Key applications include:
- Aerospace Engineering: Simulations assess high-strength, lightweight materials vital for constructing aircraft components capable of withstanding extreme conditions.
- Automotive Industry: The prediction of wear and tear on various car parts through computational microstructures leads to longer-lasting and safer vehicles.
- Energy Sector: Wind turbines and solar panels benefit from materials optimized through simulations, boosting efficiency and durability.
Consider the design of a jet engine turbine blade. By utilizing computational microstructure, engineers simulate the behavior of different metals under high temperatures and stresses. Through this method, they can choose a material with optimal properties, avoiding costly real-world testing and development setbacks.
Computational microstructures allow engineers to test extreme theoretical scenarios, which might be impossible physically due to safety or practical limitations.
Impact on Mechanical Engineering
The influence of computational microstructure in mechanical engineering is transformative. It provides detailed insights that guide the design and analysis of mechanical systems.Among its impacts are:
- Enhanced Material Performance: Through simulation, you can predict and improve the performance of materials under various environmental conditions.
- Stress Analysis: Detailed models simulate micro-level behaviors to predict stress distribution in components, improving reliability.
- Optimization of Manufacturing Processes: Computational microstructure aids in optimizing techniques like casting and welding by predicting defects and minimizing waste.
In the realm of mechanical engineering, computational microstructure uses advanced mathematical models like phase-field methods to simulate microstructure evolution. These methods employ complex differential equations, such as the Cahn-Hilliard equation, to model phase transitions within materials. The benefits include:- **Predictive Modeling**: Simulating microstructures over time offers predictions on component lifespans.- **Virtual Prototyping**: Engineers visualize and iterate designs quickly, shortening product development cycles and fostering innovation.
Computational Microstructure Analysis Techniques
In the world of computational microstructure, there are several techniques used to analyze the complex structures of materials. These techniques enable scientists and engineers to simulate and understand the intrinsic properties of materials at a microscopic level.Exploring these techniques involves advanced methods and state-of-the-art tools, enhancing the ability to predict and optimize material performance.
Advanced Analytical Methods
Among the various analytical methods used to examine microstructures, some are particularly noteworthy for their advanced capabilities:
- Finite Element Analysis (FEA): Used to solve complex structural problems by dividing the material into a mesh of smaller, manageable finite elements. This technique helps predict how microstructures will respond to physical forces.
- Phase-Field Modeling: A method that uses partial differential equations, like the Cahn-Hilliard equation, to simulate phase transformations in materials. This modeling helps in understanding the evolution of microstructures over time.
- Molecular Dynamics (MD): Involves calculating the interactions between atoms in a material to simulate material properties at a molecular level. MD is crucial for predicting thermal and mechanical behaviors.
For effective analysis using FEA, selecting an appropriate mesh size is critical, as it balances the trade-off between computational cost and result accuracy.
In deeper analysis, computational microstructure utilizes the concept of homogenization, which simplifies complex microstructures into equivalent homogeneous models. This approach involves:
- Applying mathematical techniques to average out the heterogeneous properties.
- Using scales comparison to ensure that the model's behavior matches with the actual material behavior.
Tools and Software Used in Analysis
The realm of computational microstructure is supported by numerous tools and software that facilitate detailed analysis and simulations:
- ABAQUS: A widely used software for finite element analysis, allowing users to model both simple and complex microstructures.
- COMSOL Multiphysics: Offers multi-physics simulations that incorporate various interrelated physical phenomena to accurately represent microstructures.
- Materials Studio: A suite of modeling and simulation tools tailored for material science applications, ideal for molecular dynamics simulations.
- OpenFOAM: An open-source software used for computational fluid dynamics, beneficial for simulating fluid interactions within porous microstructures.
When using ABAQUS for finite element analysis, engineers can model a component with a complex microstructure, apply loads, and study the stress distribution. This example demonstrates how the software helps visualize potential failure points before manufacturing.
Open-source tools like OpenFOAM are ideal for researchers who want flexibility and customization in their simulations.
Computational Microstructure Theory and Practice
The study of computational microstructure encompasses both theoretical and practical aspects, aiming to provide a deeper understanding of the internal characteristics of materials. By simulating the microstructural evolution of materials, significant strides can be made in anticipating their behavior under various conditions.Bridging theory and practice in this field involves not only fundamental research but also the application of simulation tools to model microstructural changes accurately.
Understanding Microstructure Evolution
To comprehend microstructure evolution, it's crucial to analyze how microstructures change over time due to external influences like temperature and pressure. These changes can be modeled computationally to predict material properties.Some critical factors influencing microstructure evolution include:
- Thermal Dynamics: Heat treatment processes, such as annealing, alter grain structures affecting material strength.
- Mechanical Stress: Forces applied to materials can cause microstructural deformation over time.
- Chemical Environment: Chemical reactions on the surface or within the material contribute to structural changes.
Consider the growth of grains within a metal, which can be described by the equation for grain boundary velocity: \[V_b = M_b \frac{\text{d} G}{\text{d} t}\]where \(V_b\) is the velocity of the boundary, \(M_b\) is mobility, and \(G\) is driving force.
In practice, if you're developing a high-temperature alloy for jet engines, understanding the microstructure evolution during thermal cycling is vital. By simulating these conditions, you can predict changes in grain structure and optimize the alloy's composition accordingly.
When simulating microstructure evolution, consider incorporating real-world constraints like manufacturing tolerances and material availability.
Theoretical Approaches in Computational Materials Engineering
Theoretical approaches in computational materials engineering provide frameworks for simulating and understanding microstructures at a fundamental level. These methods often include:
- Density Functional Theory (DFT): Used to calculate electronic structure and atomic interactions.
- Monte Carlo Simulations: A stochastic method for simulating the behavior of complex systems and predicting the probabilistic outcomes of microstructure evolution.
- Phase-Field Models: Employ differential equations to simulate phase transitions and microstructure evolution, accounting for advantageous energy minimization paths.
In detail, let's explore the Monte Carlo method employed in computational microstructure. This technique uses random sampling to obtain numerical results, often applied to the study of phase changes in materials. Here's how it works:
- Define the System: Set the initial conditions and parameters for the microstructure.
- Random Sampling: Use probability distributions to randomly perturb the system.
- Iterate and Analyze: Gradually progress the system through probable states, analyzing the resulting microstructure and driving transformations towards equilibrium.
When employing theoretical approaches, ensuring that computational demands align with the expected accuracy is crucial for efficient simulations.
computational microstructure - Key takeaways
- Computational microstructure definition: It involves the use of computational techniques to study and simulate microscopic structures in materials.
- Applications in engineering: Used in aerospace, automotive, and energy sectors to predict how materials behave under specific conditions without physical testing.
- Analysis techniques: Includes finite element analysis (FEA), phase-field modeling, and molecular dynamics for predicting material behaviors.
- Theory and practice: Involves both theoretical frameworks like Density Functional Theory and practical applications such as predicting microstructure evolution.
- Tools and software: Notable tools include ABAQUS, COMSOL Multiphysics, and Materials Studio for simulating and analyzing microstructures.
- Explained for students: Computational microstructure helps visualize and anticipate material responses, avoiding costly experiments and improving material design.
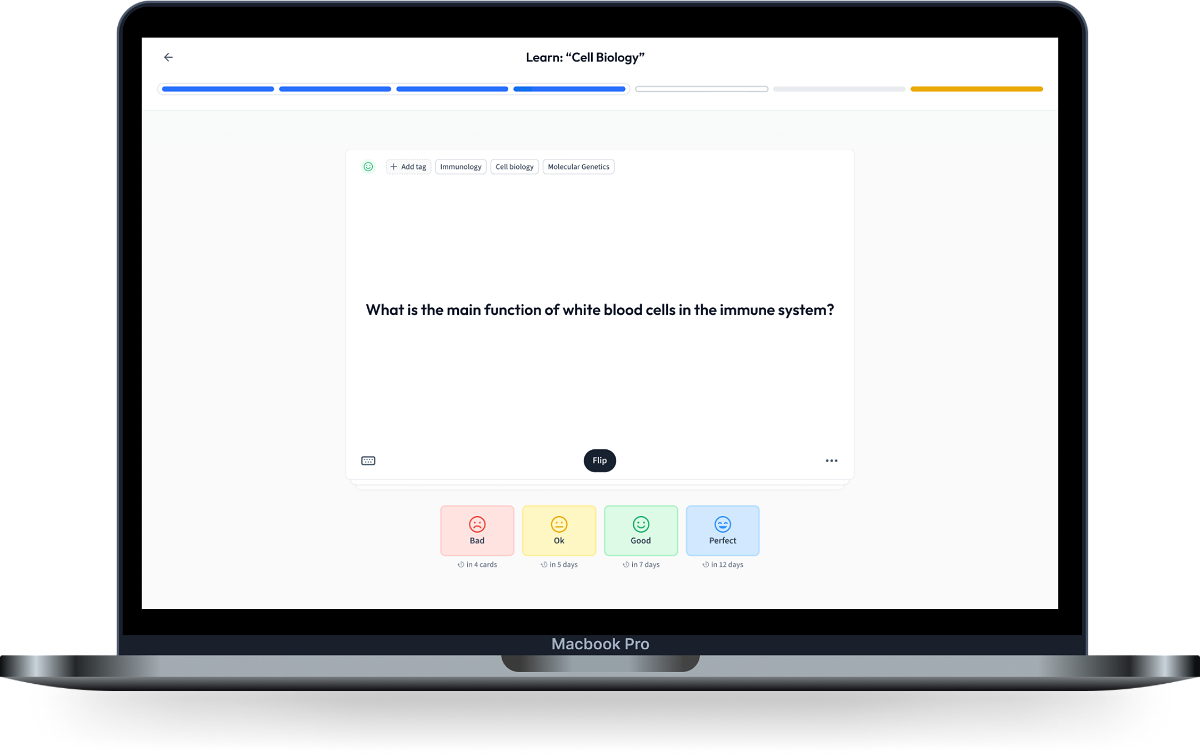
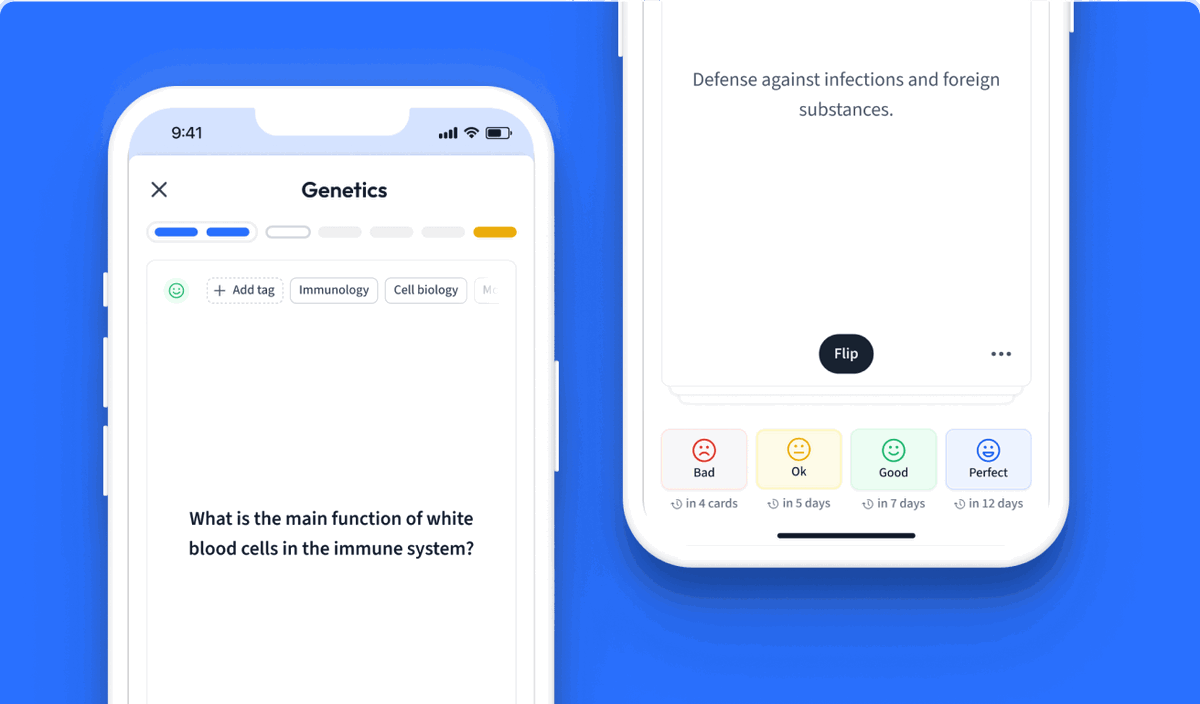
Learn with 12 computational microstructure flashcards in the free StudySmarter app
Already have an account? Log in
Frequently Asked Questions about computational microstructure
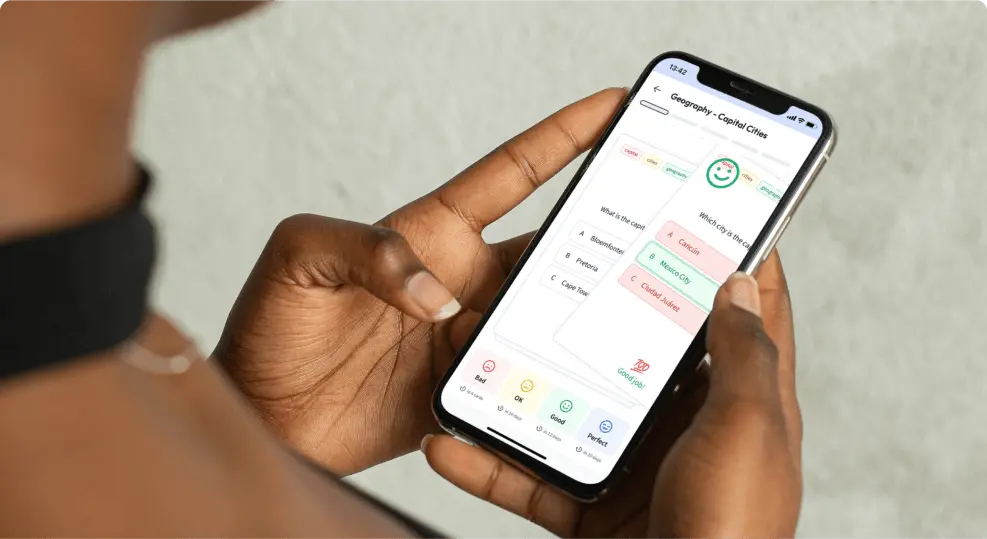
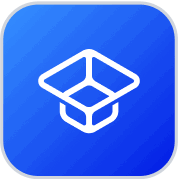
About StudySmarter
StudySmarter is a globally recognized educational technology company, offering a holistic learning platform designed for students of all ages and educational levels. Our platform provides learning support for a wide range of subjects, including STEM, Social Sciences, and Languages and also helps students to successfully master various tests and exams worldwide, such as GCSE, A Level, SAT, ACT, Abitur, and more. We offer an extensive library of learning materials, including interactive flashcards, comprehensive textbook solutions, and detailed explanations. The cutting-edge technology and tools we provide help students create their own learning materials. StudySmarter’s content is not only expert-verified but also regularly updated to ensure accuracy and relevance.
Learn more