Jump to a key chapter
What is Feature Engineering
Feature Engineering is a critical process in the realm of machine learning that involves the creation, transformation, and selection of the most relevant features from a dataset to improve the performance of predictive models. By crafting meaningful features, you can enhance the accuracy, efficiency, and interpretability of your models.
Understanding the Basics of Feature Engineering
The goal of feature engineering is to input the maximum amount of information into a machine learning model. This can be achieved through a series of techniques and methodologies:
- Feature Creation: This involves generating new features from existing attributes, usually through mathematical operations or domain-specific knowledge.
- Feature Transformation: This involves altering existing features into different formats or scales, such as through normalization or encoding.
- Feature Selection: This focuses on identifying and selecting only the most relevant features to minimize redundancy and maximize model performance.
Feature Engineering is the process of using domain knowledge to extract features from raw data, which will make predictive models perform better.
Imagine you're tasked with predicting house prices. You have raw data that includes size, number of rooms, location, etc. By creating a new feature like price per square foot, you enhance the dataset's ability to predict more accurately.
For a deeper understanding, let's consider the mathematical transformations often applied in feature engineering: Normalization: Applied to scale the features to a range [0, 1], such as: \[ x' = \frac{x - min(x)}{max(x) - min(x)} \] Encoding: Deals with categorical data by converting it into numerical format for model consumption, such as one-hot encoding.
Remember, well-engineered features can often allow simpler models to outperform more complex ones!
Importance of Feature Engineering in Machine Learning
Feature Engineering plays a crucial role in the effectiveness of machine learning models. By carefully selecting and crafting features, you can significantly enhance the model's ability to learn patterns and make accurate predictions.
How Feature Engineering Impacts Model Performance
The impact of feature engineering on machine learning is profound. It involves multiple steps that contribute to refining the model's learning process. Some benefits include:
- Improved Accuracy: Well-selected features can reduce errors and enhance prediction accuracy.
- Reduced Overfitting: By focusing only on essential features, models are less likely to make overly complex hypotheses that don't generalize well.
- Enhanced Interpretability: Models with clear, relevant features are easier to interpret and explain.
Consider a spam detection task. Raw data includes email content, sender, and time received. By engineering features like the frequency of certain keywords or the length of an email, the model's ability to identify spam can dramatically improve.
Sometimes, feature engineering can convert a nonlinear problem into a linear one, simplifying the model and training process.
Feature engineering often employs mathematical transformations to uncover intricate data patterns. These include:
- Log Transform: Useful for compressing a wide range of values. For example, transforming variable \( x \) is done with \( \log(x + 1) \).
- Polynomial Features: Introducing nonlinearity by expanding features, e.g., creating \( x^2, x^3 \).
- Interaction Features: Capturing the interaction between two variables, such as \( x_1 \times x_2 \).
These transformations can be crucial when the underlying patterns in data possess nonlinear characteristics. By applying such techniques, you can unveil hidden structures, allowing for a more nuanced analysis and model training.
Common Feature Engineering Techniques
There are numerous techniques that one can apply in feature engineering to boost the performance of machine learning models. These techniques aim to refine data into a more usable form, enhancing the model's ability to detect patterns. By learning and applying these methods, you can significantly enhance the accuracy and efficiency of models.
Feature Engineering Definition and Applications
Feature Engineering is the process of selecting, modifying, or creating new data features in order to improve the performance of machine learning algorithms. These features are often derived through expert knowledge in the field of application.
Feature Engineering involves creating and selecting the most relevant features from data to ensure a more efficient and effective machine learning model.
Applications of feature engineering span across various domains, including:
- Finance: Crafting features like average transaction size or spending patterns can aid in better credit score predictions.
- Healthcare: Deriving features such as age or hospitalization frequency can assist in predicting patient outcomes.
- E-commerce: Constructing features based on user purchase history helps in personalized recommendation systems.
In feature engineering, mathematical and statistical transformations often play a critical role. Here are some mathematical methods that are broadly used:
- Standardization: Transforming features to have zero mean and unit variance, mathematically represented as \[ z = \frac{x - \text{mean}(x)}{\text{std}(x)} \].
- Discretization: Converting continuous features into discrete buckets or intervals, effectively translating real-valued data into categories.
In many applications, less is often more – selecting fewer, yet meaningful features can lead to a model with greater generality and efficiency.
Examples of Feature Engineering in Machine Learning
Feature engineering is critical in tailoring datasets to enhance model capabilities. Examples in real-world machine learning applications include:
In a weather prediction model, raw data about temperature, humidity, and wind speed can be enriched by features such as heat index and wind chill factor, which convey more nuanced weather conditions.
Here's a simple table to illustrate the transformation of features into more informative representations:
Raw Feature | Engineered Feature |
Temperature (C) | Heat Index |
Wind Speed (km/h) | Wind Chill |
Timestamp | Time of Day |
Advanced Feature Engineering Techniques
In the evolving landscape of machine learning, advanced techniques in feature engineering play a pivotal role in improving model performance and insights. These techniques help extract more meaningful information from data, allowing models to learn and generalize better.
Polynomial and Interaction Features
Polynomial and interaction features provide a way to capture nonlinear relationships in your data. While basic linear models might overlook such complexity, these features can enhance model sophistication by capturing higher-degree relationships.
In a dataset with features like height and weight, creating a polynomial feature like height squared (\( \text{height}^2 \)) or an interaction feature such as height times weight (\( \text{height} \times \text{weight} \)) can allow for better predictions if the target depends on these combinations.
To further understand these techniques, let's explore their mathematical formulation:
- Polynomial Features: By representing a feature \( x \) with powers, such as \( [x, x^2, x^3] \), you enhance the model's ability to fit complex curves.
- Interaction Features: For features \( x_1 \) and \( x_2 \, \( x_1 \times x_2 \) reveals their joint effect, potentially creating new insights.
These transformations are immensely helpful when dealing with data that reveals intricate patterns not easily captured by linear combinations alone.
Feature Encoding and Transformation
To make categorical data suitable for machine learning models, feature encoding and transformation are crucial. These techniques transform non-numeric feature values into numerical ones.
If you have a feature like `day_of_week` with values {Monday, Tuesday, ..., Sunday}, using one-hot encoding transforms it into binary features like {Mon: [1,0,0,0,0,0,0], Tue: [0,1,0,0,0,0,0], etc.}, allowing models to process them effectively.
Some transformation methods, such as using target encoding, can encapsulate the relationship between features and the prediction target itself.
Various encoding techniques to handle categorical features include:
- Label Encoding: Converts categories to integer codes, suitable if the categories have a desirable intrinsic order, represented as
'from sklearn.preprocessing import LabelEncoderencoder = LabelEncoder()encoded_labels = encoder.fit_transform(categorical_feature)'
- One-Hot Encoding: Converts categories into a binary matrix, where each category is represented as a vector.
'from sklearn.preprocessing import OneHotEncoderencoder = OneHotEncoder(sparse=False)encoded_matrix = encoder.fit_transform(categorical_feature)'
feature engineering - Key takeaways
- Feature Engineering Definition: The process of using domain knowledge to extract features from raw data to enhance model performance.
- Importance in Machine Learning: Improves accuracy, reduces overfitting, and enhances interpretability of machine learning models.
- Feature Engineering Techniques: Involves feature creation, transformation, and selection to maximize model performance.
- Common Techniques: Normalization, encoding, log transform, polynomial and interaction features, standardization, and discretization.
- Applications: Used across various fields like finance, healthcare, and e-commerce for better predictions.
- Impact on Models: Allows simpler models to outperform complex ones by crafting meaningful features and revealing hidden data structures.
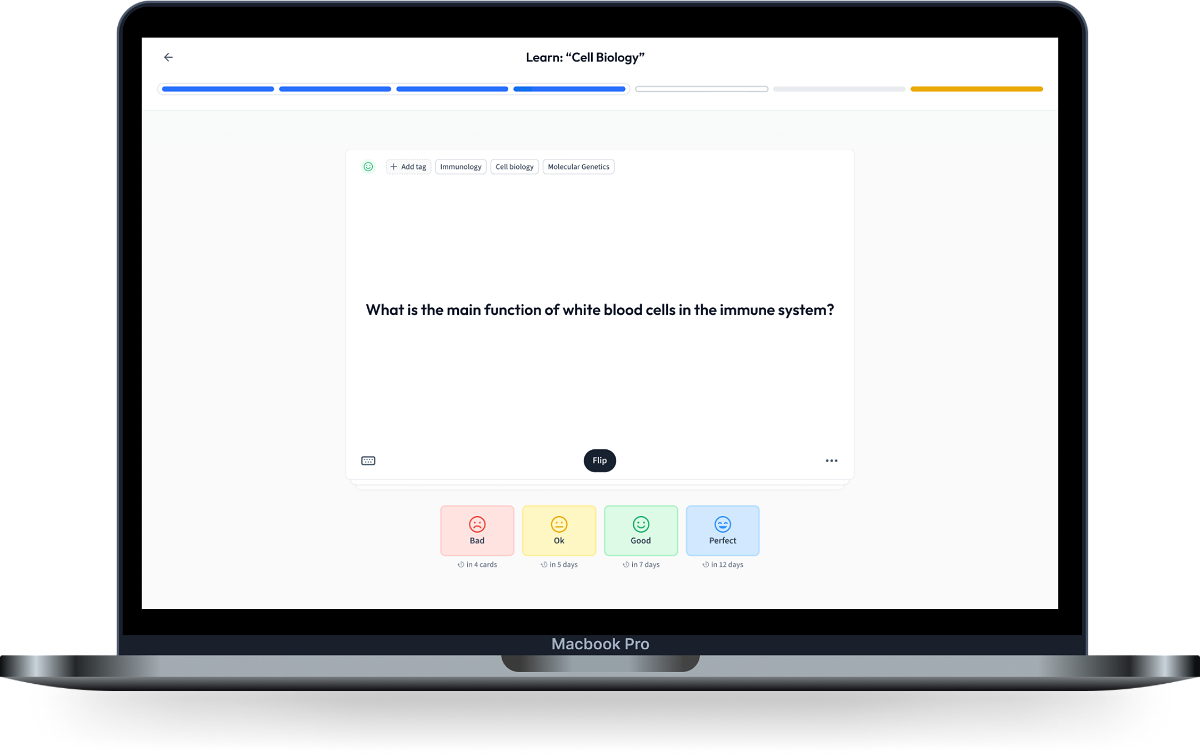
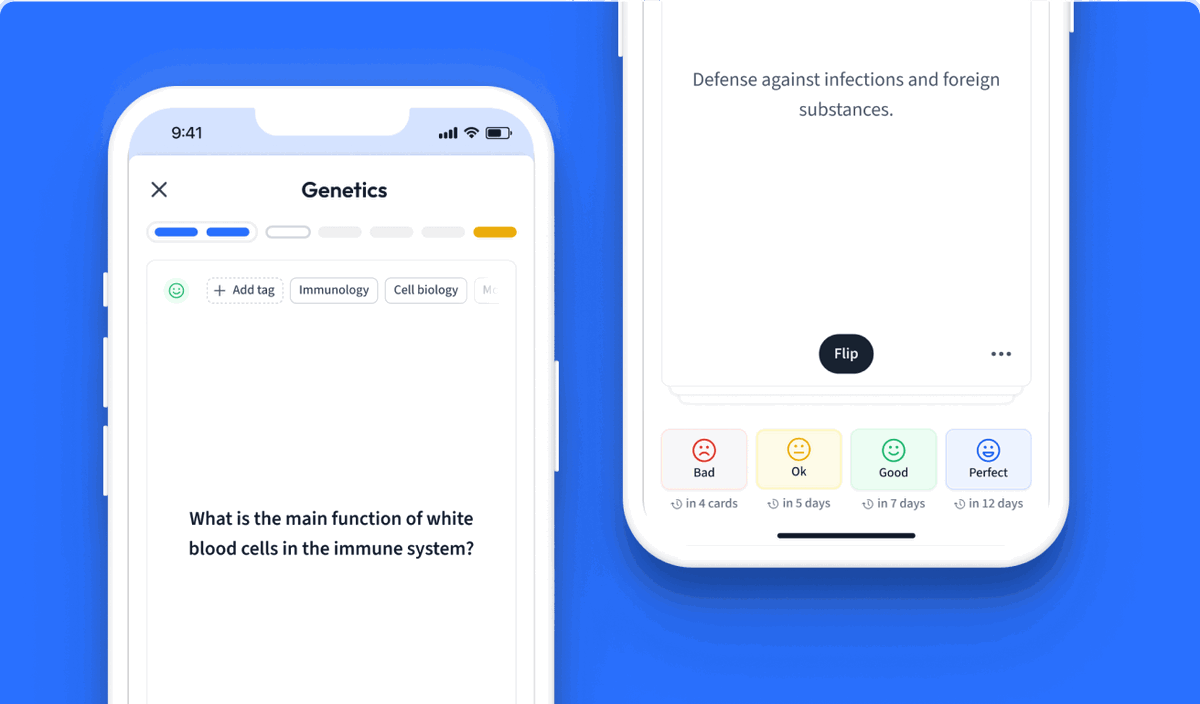
Learn with 12 feature engineering flashcards in the free StudySmarter app
We have 14,000 flashcards about Dynamic Landscapes.
Already have an account? Log in
Frequently Asked Questions about feature engineering
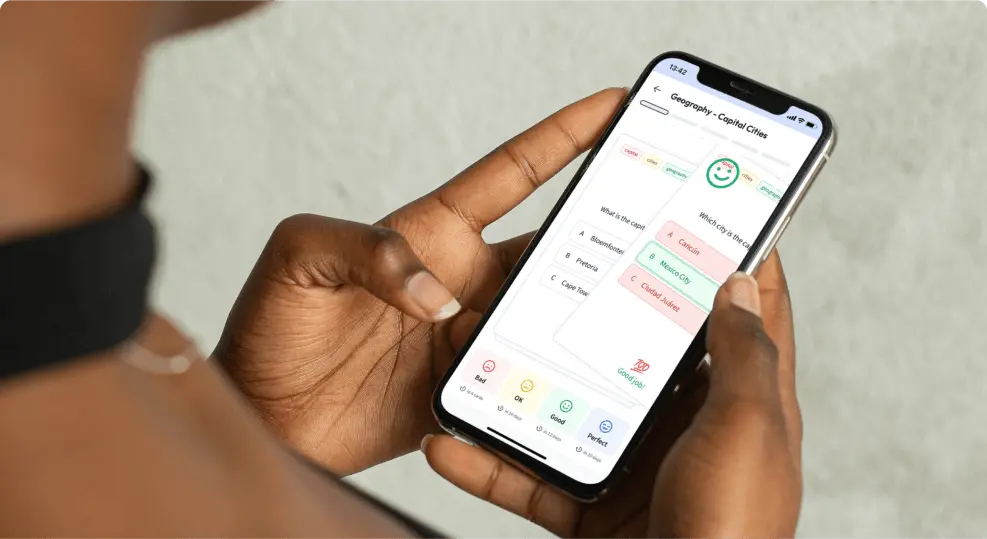
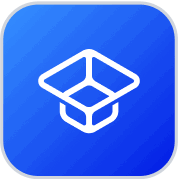
About StudySmarter
StudySmarter is a globally recognized educational technology company, offering a holistic learning platform designed for students of all ages and educational levels. Our platform provides learning support for a wide range of subjects, including STEM, Social Sciences, and Languages and also helps students to successfully master various tests and exams worldwide, such as GCSE, A Level, SAT, ACT, Abitur, and more. We offer an extensive library of learning materials, including interactive flashcards, comprehensive textbook solutions, and detailed explanations. The cutting-edge technology and tools we provide help students create their own learning materials. StudySmarter’s content is not only expert-verified but also regularly updated to ensure accuracy and relevance.
Learn more