Jump to a key chapter
What is Frequency Domain Analysis
Frequency Domain Analysis is a fundamental concept in engineering and signal processing. It involves analyzing signals or systems based on frequency, rather than time.
Understanding the Frequency Domain
In the frequency domain, signals are represented by their frequency components. Instead of dealing with waveforms in the time domain, you analyze spectral content. This is crucial in fields such as telecommunications, audio processing, and electronics.
- Time Domain: Involves observing signal changes over time.
- Frequency Domain: Focuses on signal composition in terms of sinusoidal components.
The transition from the time domain to the frequency domain is accomplished using mathematical transformations like the Fourier Transform.
Fourier Transform: A mathematical technique that transforms a time-domain signal into its frequency-domain representation.
For instance, consider a simple sine wave in time domain, represented by A sin(ωt). Applying Fourier Transform will showcase a peak at the frequency ω in the frequency domain, as the frequency content is concentrated at this point.
Mathematical Representation
The Fourier Transform of a continuous time signal x(t) is given by:
\[X(f) = \int_{-\infty}^{\infty} x(t) e^{-j2\pi ft} dt\]
This transformation expresses the signal x(t) as a superposition of complex exponentials or sinusoids.
Variable | Meaning |
f | Frequency Variable |
X(f) | Frequency Domain Representation |
Frequency domain analysis is particularly useful in identifying periodicities in signals or determining how a system responds to different inputs.
In practical applications, the Discrete Fourier Transform (DFT) is widely used. It allows computational processors to efficiently transform digital data into the frequency domain. One key algorithm for computing DFT is the Fast Fourier Transform (FFT). The FFT reduces the computational complexity from O(n²) in direct calculation to O(n log n), making real-time signal processing feasible even with large datasets.
The implementation of FFT is highly optimized for computers, allowing devices to analyze audio signals, decode telecommunications data, and process images efficiently. Engineers often prefer using libraries in programming languages like Python or MATLAB, due to their highly optimized versions of FFT, for digital signal processing tasks.
Frequency Domain Analysis in Control System
The concept of Frequency Domain Analysis plays a vital role in understanding control systems. It helps you in analyzing how different frequencies affect system stability and performance.
Frequency domain concepts are essential in control engineering for systems that operate across a range of frequencies. They allow you to assess system behavior without solving differential equations directly.
Benefits of Frequency Domain Analysis in Control Systems
Frequency domain analysis is beneficial because:
- Stability: Gain insight into system stability by observing resonance frequencies and damping factors.
- Performance: Assess how a system responds to different frequency inputs, aiding in filter design and noise suppression.
- Efficiency: Simplifies complex system behavior into manageable parts using tools like Bode plots and Nyquist plots.
Frequency Domain Stability Analysis
In control systems, Frequency Domain Stability Analysis is essential for determining how systems respond to inputs of various frequencies. This analysis helps ensure a system is stable and functions correctly across its operational frequency range.
Stability in the Frequency Domain
Stability in the frequency domain can be analyzed using methods like Bode plots or Nyquist criteria. These techniques allow you to visualize the effect of changing frequencies on a system's stability.
- Bode Plot: Graphical representation showing the frequency response of a system.
- Nyquist Plot: Illustrates how the frequency response encircles the critical point \text{-1,0}, providing insights into system stability.
Frequency Domain Analysis of RLC Circuits
Frequency Domain Analysis is crucial for evaluating RLC circuits. By observing how these circuits behave over a range of frequencies, you gain insights into their resonant properties and impedance variations.
RLC circuits consist of resistors (R), inductors (L), and capacitors (C), each introducing their own frequency-dependent characteristics.
Techniques in Frequency Domain Analysis
Various techniques are utilized in frequency domain analysis to understand RLC circuits:
- Impedance Analysis: Calculate the total impedance, considering the frequency-dependent impedance of capacitors and inductors.
- Resonance: Identify the frequency at which the circuit naturally oscillates, known as the resonant frequency.
- Bode Plots: Graphical representation outlining how the magnitude and phase of the circuit’s impedance change with frequency.
Calculating the impedance of an RLC circuit involves summing the impedances of its components:
\[Z = R + j\left(\omega L - \frac{1}{\omega C}\right)\]
Component | Impedance |
Resistor | R |
Inductor | \(j\omega L\) |
Capacitor | \(-\frac{j}{\omega C}\) |
Remember, \(j\) represents the imaginary unit, often used in analysis related to phasors and complex numbers.
Understanding resonance in RLC circuits is fundamental to frequency domain analysis. At resonance, the inductive and capacitive reactances cancel each other out, leading to a purely resistive impedance at a specific frequency. This frequency, \(f_r\), can be calculated using:
\[f_r = \frac{1}{2\pi\sqrt{LC}}\]
This equation highlights that the resonant frequency depends only on the inductor and capacitor values. The concept of resonance is critical in applications such as tuning circuits, where maximum voltage or current response at a particular frequency is desired.
frequency domain analysis - Key takeaways
- Frequency Domain Analysis: Analyzes signals/systems based on frequency rather than time; crucial for fields like telecommunications.
- Fourier Transform: Mathematical technique to convert time-domain signals into frequency-domain representation.
- Frequency Domain Stability Analysis: Uses methods like Bode and Nyquist plots to ensure the stability of control systems.
- Benefits in Control Systems: Provides insights into system stability, performance evaluation, and efficiency improvements.
- RLC Circuit Analysis: Evaluates circuit behavior over frequencies, focusing on impedance and resonance.
- Techniques in Frequency Domain Analysis: Includes impedance analysis, resonance identification, and Bode plots for RLC circuits.
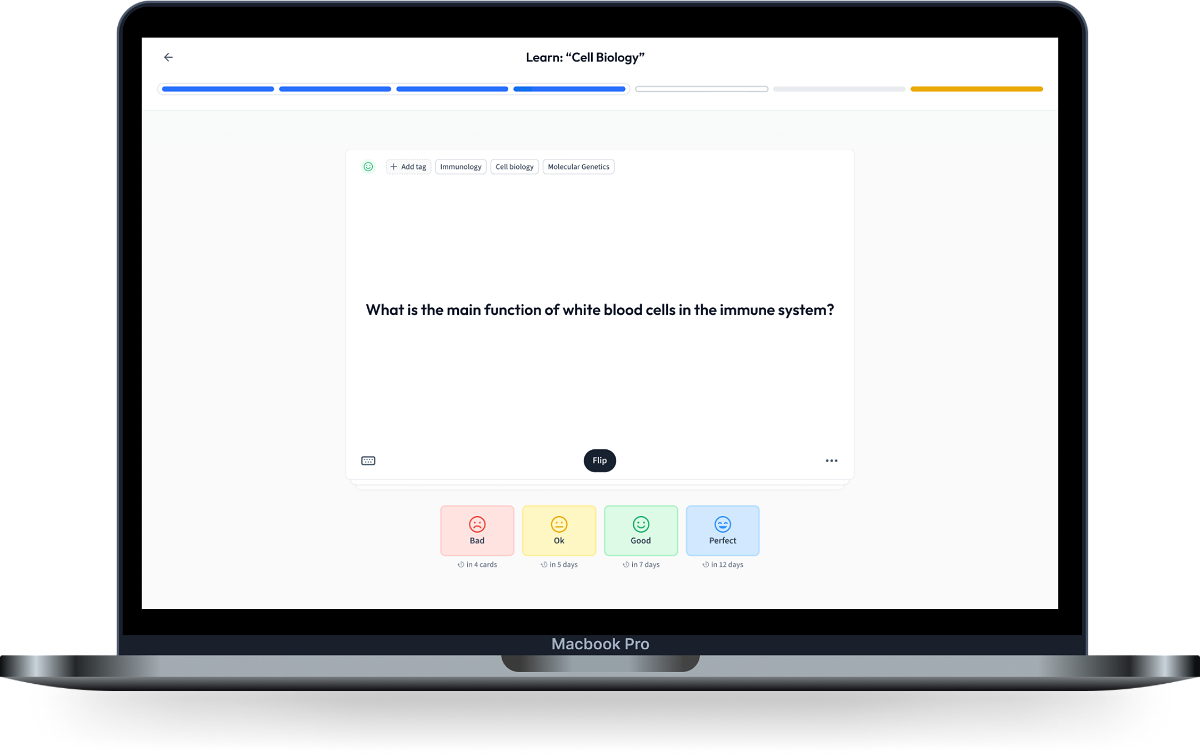
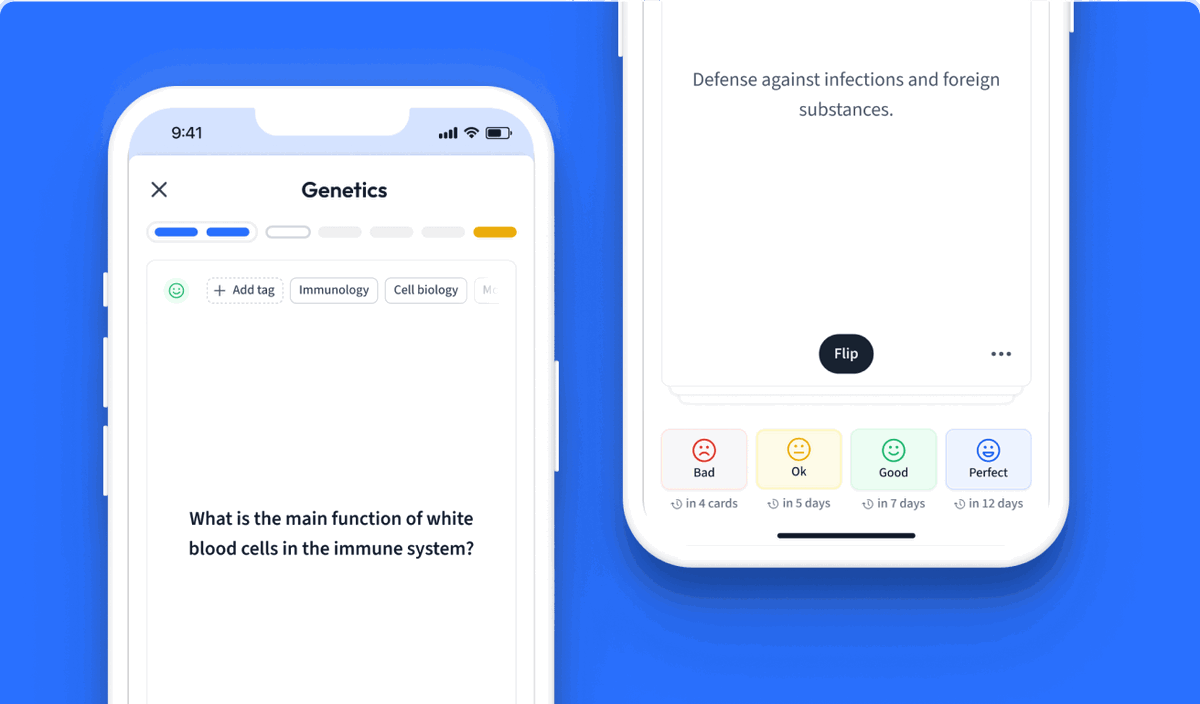
Learn with 12 frequency domain analysis flashcards in the free StudySmarter app
Already have an account? Log in
Frequently Asked Questions about frequency domain analysis
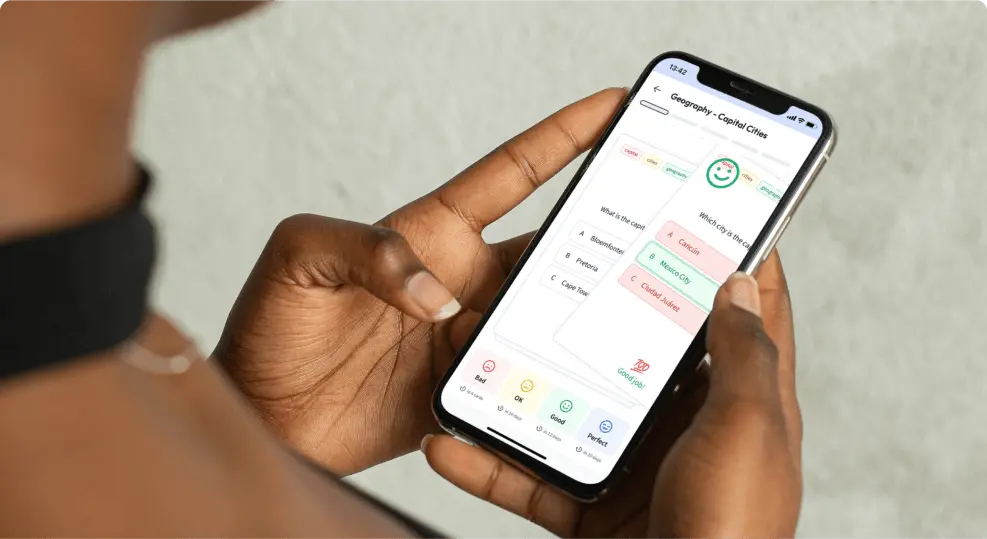
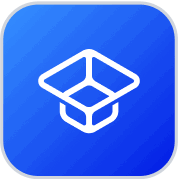
About StudySmarter
StudySmarter is a globally recognized educational technology company, offering a holistic learning platform designed for students of all ages and educational levels. Our platform provides learning support for a wide range of subjects, including STEM, Social Sciences, and Languages and also helps students to successfully master various tests and exams worldwide, such as GCSE, A Level, SAT, ACT, Abitur, and more. We offer an extensive library of learning materials, including interactive flashcards, comprehensive textbook solutions, and detailed explanations. The cutting-edge technology and tools we provide help students create their own learning materials. StudySmarter’s content is not only expert-verified but also regularly updated to ensure accuracy and relevance.
Learn more