Jump to a key chapter
Introduction to Intelligent Systems
An intelligent system is a computational system that mimics aspects of human intelligence. These systems are designed to understand, learn, and make decisions based on a given set of data or information. They play a crucial role in numerous fields such as robotics, data analysis, and artificial intelligence.
What Are Intelligent Systems?
Intelligent systems combine various technologies to simulate cognition and decision-making processes. They are prevalent in applications ranging from simple algorithms in your smartphone to complex networks powering autonomous vehicles. Here are some key characteristics of intelligent systems:
- Autonomy: They can perform tasks without human intervention.
- Adaptivity: They learn and improve over time with new information.
- Collaboration: They can work alongside humans or other systems.
An intelligent system is a computational technology designed to perform tasks by emulating human cognitive abilities such as learning, reasoning, and decision-making.
An example of an intelligent system is a recommendation engine used by streaming services. By analyzing a user's viewing history, it suggests new shows or movies, continually learning from user preferences.
The evolution of intelligent systems has been driven by advancements in machine learning algorithms and computational power. Initially, these systems relied on rule-based logic but have since evolved to adopt neural networks and deep learning. - Machine Learning: This subset of AI forms the core of intelligent systems, enabling them to learn from data without explicit programming. - Neural Networks: Inspired by the human brain, these computational models help in recognizing patterns and complex relationships within data. - Deep Learning: A further refinement of neural networks, it allows intelligent systems to process large volumes of data, learning more abstract features.
Applications of Intelligent Systems
Intelligent systems have a broad range of applications across various industries, revolutionizing how tasks are performed and decisions are made. Here are some notable examples:
Field | Application |
Healthcare | Predictive diagnostics and personalized medicine |
Finance | Fraud detection and automated trading |
Manufacturing | Predictive maintenance and automation |
Automotive | Autonomous driving and intelligent traffic systems |
Keep an eye on how intelligent systems continue to evolve, particularly with emerging technologies like quantum computing, which promises to significantly increase their capabilities.
Intelligent Mechatronic Systems Explained
Intelligent mechatronic systems combine mechanical, electronic, and computational components to create highly adaptive technologies capable of complex tasks. These systems harness sensory data to make informed decisions, thus enhancing automation and precision in engineering applications.
Components of Intelligent Mechatronic Systems
Each intelligent mechatronic system consists of several key components that enable it to function effectively.
- Sensors: These devices collect data from the system's environment, such as temperature, pressure, or motion.
- Actuators: These components convert electrical signals into physical action, allowing the system to interact with its surroundings.
- Controllers: These manage and process the sensory data, making decisions that guide the operation of the actuators.
- Software: Comprising algorithms and control strategies, the software is the brain of the system, executing the decision processes.
A controller within a mechatronic system is a computational unit that processes sensor data and issues commands to the actuators.
Consider a factory automation system. It uses sensors to determine the precise position of an object on a conveyor belt, actuators to move robotic arms for assembly, and controllers to synchronize processes to ensure efficiency and accuracy.
Let's delve deeper into the role of machine learning in intelligent mechatronic systems. Machine learning algorithms are incorporated into the software component to provide adaptability and improve performance over time.For example:
- Pattern Recognition: Systems can identify specific patterns from sensor data, allowing predictive maintenance by analyzing signs of wear and tear.
- Optimization: Algorithms optimize the overall system operation, minimizing energy use and maximizing productivity.
Applications in Modern Engineering
Intelligent mechatronic systems have extensive applications across modern engineering disciplines, transforming traditional processes into optimized solutions. Notable applications include:
Sector | Example |
Automotive | Adaptive cruise control systems |
Healthcare | Surgical robots and diagnostic devices |
Manufacturing | Smart assembly lines and inspection systems |
Aerospace | Stability and navigation systems in aircraft |
Incorporating advancements like the Internet of Things (IoT) into mechatronic systems further broadens their connectivity and interoperability, marking the future of engineering automation.
Intelligent Transportation Systems Overview
Intelligent Transportation Systems (ITS) incorporate technology into transportation infrastructure to enhance the efficiency, safety, and convenience of travel. By utilizing data analysis and digital communication, these systems improve traffic management, reduce congestion, and provide real-time information to travelers.
Benefits of Intelligent Transportation Systems
The implementation of Intelligent Transportation Systems offers numerous benefits for urban and rural areas. Here are some of the key advantages:
- Reduced Traffic Congestion: ITS technologies optimize traffic flow and reduce bottlenecks, decreasing travel time and vehicle emissions.
- Enhanced Safety: Real-time monitoring systems detect accidents and hazardous conditions, enabling quicker emergency response and reducing crash risks.
- Improved Public Transport: ITS supports real-time tracking and route optimization for buses and trains, ensuring more efficient public transportation services.
- Environmental Impact: By optimizing routes and lowering idle times, these systems contribute to reduced fuel consumption and greenhouse gas emissions.
An example of a benefit of Intelligent Transportation Systems is the adaptive traffic signal control. This system adjusts the timing of traffic lights based on current traffic conditions, improving vehicle flow and reducing idling times.
Delving deeper into the safety enhancements provided by Intelligent Transportation Systems, these solutions often include:
- Collision Avoidance Systems: These systems use sensors and communication technologies to warn drivers of potential collisions or automatically apply brakes.
- Incident Detection Systems: Cameras and sensors monitor road conditions and detect incidents such as accidents or debris.
- Emergency Vehicle Notification Systems: Provide priority signals for emergency vehicles to ensure faster response times.
ITS can significantly reduce the costs associated with traffic congestion, potentially saving billions annually in lost productivity and wasted fuel.
Key Technologies in Transportation Systems
Several cutting-edge technologies underpin Intelligent Transportation Systems, each playing a vital role in their operation. Below is a summary of some essential technologies:
- Vehicle-to-Infrastructure (V2I) Communication: This enables the exchange of information between vehicles and roadside units, improving traffic management and road safety conditions.
- Global Positioning System (GPS): Provides accurate location data essential for navigation and real-time traffic monitoring.
- Traffic Management Software: Optimizes traffic flow by analyzing real-time data and adjusting traffic signals and routes.
- Sensor Networks: Composed of cameras, radars, and other sensing devices, these networks collect data on traffic speed, volume, and road conditions.
A Vehicle-to-Infrastructure (V2I) communication is a technology that facilitates data exchange between vehicles and surrounding infrastructure to enhance traffic management and road safety.
As these technologies mature, expect even greater interoperability and innovation within Intelligent Transportation Systems, further transforming our transportation landscape.
Intelligent Control Systems in Mechanical Engineering
In the field of mechanical engineering, intelligent control systems are pivotal for the advancement of automation and precision. These systems incorporate artificial intelligence techniques and strategies to manage and regulate complex processes.
Working Mechanisms of Intelligent Control Systems
Intelligent control systems utilize a combination of sensors, processors, and actuators to monitor and control mechanical processes. These systems learn from external conditions and experiential data to adapt their functioning over time.The foundation of intelligent control systems typically involves:
- Data Acquisition: Sensors collect accurate data from the environment related to variables such as position, speed, and temperature.
- Data Processing: The collected data is processed by algorithms, often employing approaches like fuzzy logic or neural networks to handle uncertainties.
- Decision-Making: The system makes informed decisions based on processed data to maintain optimal conditions and performance.
- Execution: Actuators implement the control actions determined by the decision-making process.
A sensor in an intelligent control system is a device that detects physical changes in an environment and converts them into data for computational analysis.
An example of an intelligent control system is found in the modern thermostat, which utilizes sensors to monitor room temperature. It then processes this data to adjust the heating or cooling systems for maintaining the desired temperature.
For a deeper understanding, consider the use of neural networks in intelligent control systems. These networks are capable of finding complex, non-linear relationships between inputs and outputs, enhancing system control accuracy. The architecture of a typical neural network in control systems includes:- Input Layer: Where sensor data is introduced.- Hidden Layers: Multiple layers where data transformations and computations occur, identifying patterns.- Output Layer: Produces the control signals for actuation.The power of neural networks lies in their ability to learn and generalize from past data, providing a robust mechanism for handling highly dynamic environments.
Incorporating advanced algorithms like reinforcement learning can further improve the performance of intelligent control systems by optimizing decision-making strategies.
Intelligent Control Systems in Industry
Industrial applications of intelligent control systems are vast, significantly improving efficiency and productivity in various sectors. These systems are essential for automating complex processes and enabling adaptive control strategies.Here are some industry-specific applications:
Sector | Application |
Manufacturing | Robotic arms for assembly lines |
Energy | Smart grid management |
Automotive | Autonomous vehicle navigation systems |
Predictive maintenance, powered by intelligent control systems, significantly reduces downtime and maintenance costs in industrial settings.
Autonomous and Intelligent Aerospace Systems
The aerospace industry is undergoing a significant transformation due to the rise of autonomous and intelligent systems. These systems leverage advanced technologies such as artificial intelligence and machine learning to enhance operational efficiency, safety, and capabilities in aerospace applications.
Innovations in Aerospace
Recent innovations in aerospace are primarily driven by the integration of intelligent systems. Here are several notable advancements:
- Autonomous Drones: Equipped with AI, these drones perform surveillance, mapping, and environmental monitoring tasks with minimal human intervention.
- Smart Air Traffic Management: Incorporating AI-driven algorithms, air traffic control systems now predict and manage traffic flows, reducing congestion and enhancing safety.
- Intelligent Maintenance Systems: Predictive analytics and machine learning are employed to anticipate maintenance needs, preventing unexpected downtimes.
An autonomous drone is an unmanned aerial vehicle (UAV) that utilizes artificial intelligence to fly, navigate, and perform tasks without direct human control.
One example is the use of smart air traffic control systems which utilize AI to smoothly accommodate increased traffic by optimizing flight paths and schedules, thus minimizing delays and fuel consumption.
Exploring deeper, let's consider the role of AI in cockpit automation. Known as fly-by-wire, this technology replaces conventional manual flight controls with an electronic interface. Here are some components:- The pilot's input is processed by on-board computers that adjust control surfaces to achieve the desired flight path.- Intelligent systems provide real-time feedback, assist in decision-making, and offer corrective actions during flight deviations.This innovation enhances safety, efficiency, and reliability, showcasing the profound impact of intelligent systems on piloting and flight operations.
AI-driven systems are increasingly capable of self-improvement, learning from each flight to optimize performance and safety.
Future of Aerospace Systems
The future of aerospace systems is poised to be shaped by the continued advancement of intelligent technologies. New possibilities include:
- More Autonomous Vehicles: Expect increased autonomy in both commercial and military aircraft, enhancing capabilities while reducing operational costs.
- Efficient Space Exploration: AI systems will play a significant role in deep space missions, handling complex tasks and responding to unforeseen challenges.
- Eco-friendly Aviation: The integration of AI in designing and managing efficient, environmentally sustainable flight operations will be crucial.
An autonomous vehicle in aerospace refers to any aircraft or spacecraft capable of self-operating without human intervention, using intelligent systems to guide and control its operations.
Continuous innovation in AI and data analytics will lead to breakthroughs in sustainable aerospace technology, addressing both economic and ecological challenges.
Role of Intelligent Systems in Applications Engineering
In applications engineering, intelligent systems leverage technologies like machine learning, neural networks, and data analytics to enhance and automate processes. Their role is foundational in improving efficiency, accuracy, and decision-making capabilities across various industries.
Integrating Artificial Intelligence Systems
Integrating Artificial Intelligence (AI) systems into applications engineering involves embedding smart algorithms into existing processes to improve performance. Key steps in integration include:
- Data Collection: Gathering data from various sources such as sensors, historical databases, and real-time tracking systems.
- Algorithm Development: Designing algorithms capable of processing data and making predictions or decisions.
- System Deployment: Integrating AI systems within existing processes and ensuring compatibility with other system components.
- Continuous Monitoring: Adjusting and fine-tuning AI models to align with changing data trends and business objectives.
A neural network is a computational model inspired by the human brain, capable of recognizing complex patterns and relationships within data, used in AI systems for applications engineering.
One application of integrating AI systems is in predictive maintenance across manufacturing industries. These AI systems analyze equipment data to foresee potential breakdowns, thus scheduling timely maintenance and reducing downtime.
To fully understand AI integration, consider the intricate workings of a convolutional neural network (CNN) utilized for visual data processing. Key components include:- Convolutional Layers: Where filters scan through data to detect features such as edges and textures.- Pooling Layers: Reduce the spatial size of representations, lowering computational demands and controlling overfitting.- Fully Connected Layers: Flatten the matrix into a vector, eventually mapping learned features to outputs.The efficacy of a CNN in applications engineering can be represented by its ability to learn from diverse image datasets, enhancing applications such as quality control in manufacturing.
Blockchain technology can be integrated with AI systems to enhance the security and transparency of data handling in applications engineering.
Challenges and Opportunities in Applications Engineering
While integrating intelligent systems in applications engineering presents opportunities for innovation, it also comes with numerous challenges. Here are some critical aspects:Challenges:
- Data Privacy: Ensuring compliance with data protection regulations like GDPR is crucial.
- Interoperability: Achieving seamless integration with existing systems and platforms can be complex.
- Scalability: Adapting systems to cope with growing data volumes and processing demands.
- Enhanced Decision-Making: AI systems provide insights that enhance strategic planning and operational efficiency.
- Cost Reduction: Automation of repetitive tasks leads to significant labor and operational cost savings.
- Innovation Stimulation: Encourages the development of new products and services optimized through intelligent systems.
Cloud computing offers a robust solution for scaling AI systems, providing the necessary computational power and data storage.
intelligent systems - Key takeaways
- Intelligent systems: Computational technologies that emulate human intelligence for decision-making and learning.
- Intelligent mechatronic systems: Systems that integrate mechanical, electronic, and computational elements for automation and adaptive tasks.
- Intelligent transportation systems: Use of technology in transport infrastructure for enhanced safety, efficiency, and communication.
- Intelligent control systems: Systems in mechanical engineering employing AI for monitoring and control of processes.
- Autonomous and intelligent aerospace systems: Aerospace innovations leveraging AI for enhanced efficiency, safety, and operations.
- Intelligent systems applications engineering: Integration of AI and machine learning to optimize processes across various industries.
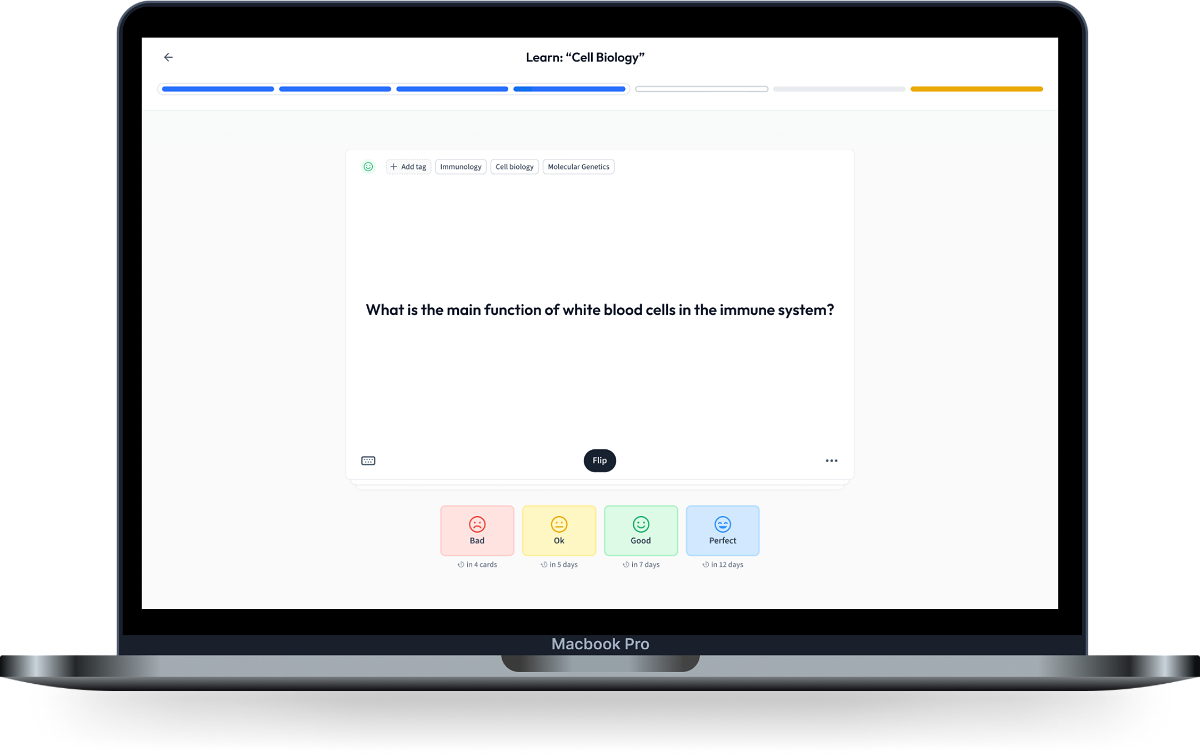
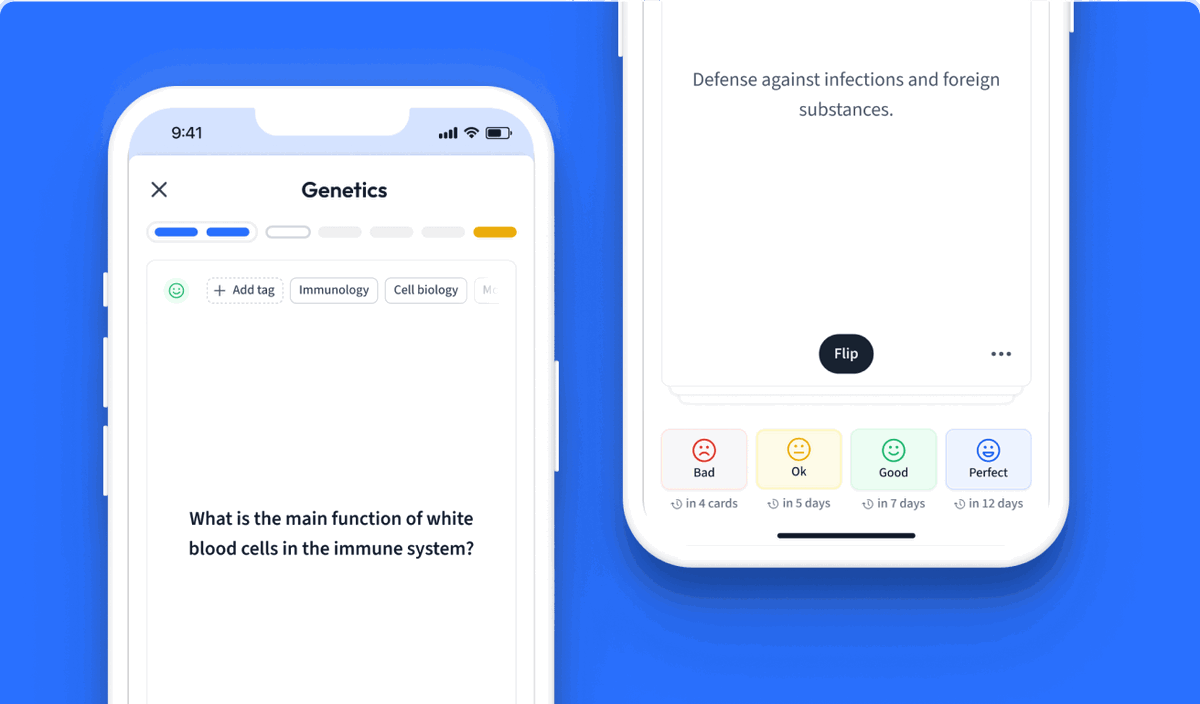
Learn with 12 intelligent systems flashcards in the free StudySmarter app
We have 14,000 flashcards about Dynamic Landscapes.
Already have an account? Log in
Frequently Asked Questions about intelligent systems
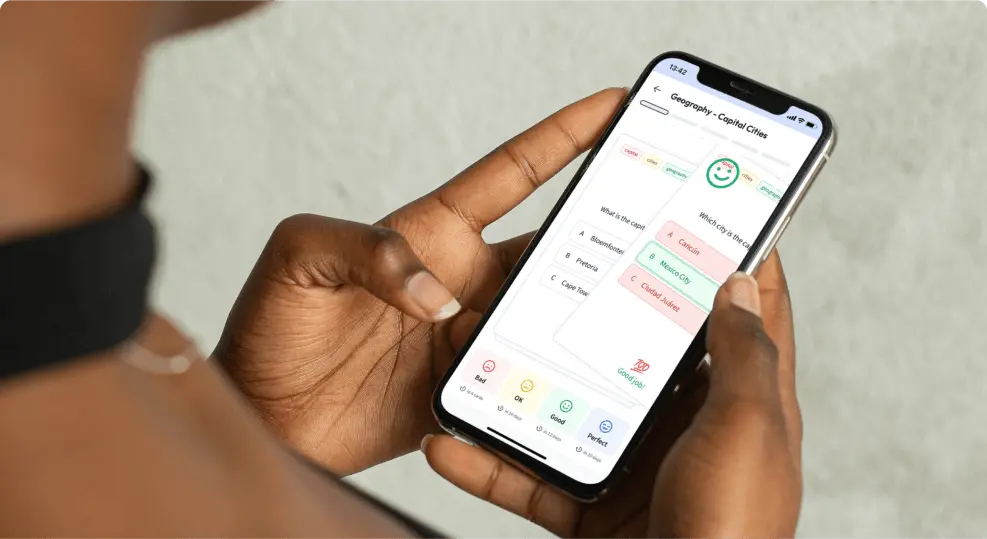
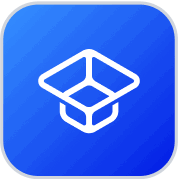
About StudySmarter
StudySmarter is a globally recognized educational technology company, offering a holistic learning platform designed for students of all ages and educational levels. Our platform provides learning support for a wide range of subjects, including STEM, Social Sciences, and Languages and also helps students to successfully master various tests and exams worldwide, such as GCSE, A Level, SAT, ACT, Abitur, and more. We offer an extensive library of learning materials, including interactive flashcards, comprehensive textbook solutions, and detailed explanations. The cutting-edge technology and tools we provide help students create their own learning materials. StudySmarter’s content is not only expert-verified but also regularly updated to ensure accuracy and relevance.
Learn more