Jump to a key chapter
What is Smart Manufacturing
Smart Manufacturing refers to the integration of advanced technologies, such as IoT (Internet of Things), Artificial Intelligence (AI), and robotics, to create efficient and adaptable manufacturing processes. This approach allows manufacturers to streamline operations, reduce costs, and improve product quality by enabling real-time data monitoring and automated control systems.
Definition of Smart Manufacturing
Smart Manufacturing is a method of optimizing manufacturing processes by leveraging digital technologies, data analytics, and connected machinery to enhance production efficiency and flexibility.
Incorporating advanced tools, Smart Manufacturing transforms traditional production lines into a network of interconnected systems. It involves the following core elements:
- IoT Devices: Sensors and devices used to gather real-time data across the manufacturing floor.
- Data Analytics: The use of big data analytics to gain insights and optimize the production process.
- AI and Machine Learning: Algorithms that improve decision-making and predictive maintenance capabilities.
- Automation: The integration of robotics to handle repetitive tasks efficiently.
An example of Smart Manufacturing is the use of smart sensors in automotive factories. These sensors monitor every aspect of production, from assembly line speed to temperature control, ensuring that each car is manufactured to precise specifications. This level of oversight helps to prevent defects and reduce waste.
Did you know that Smart Manufacturing helps in reducing the environmental impact of industries by minimizing waste and optimizing resources?
Smart Manufacturing in Engineering
The integration of Smart Manufacturing in engineering represents a transformative approach that blends advanced technologies with traditional manufacturing processes. This fusion aims to create more efficient, cost-effective, and adaptable production systems.
Smart Manufacturing Technology
Smart Manufacturing Technology encompasses a broad range of innovative tools and systems designed to enhance manufacturing capabilities. By leveraging technologies such as connectivity, data analytics, and automation, manufacturers can optimize their operations and improve decision-making processes.
Smart Manufacturing Technology is an advanced platform combining digital tools, real-time data, and intelligent automation to enhance the adaptability and efficiency of manufacturing operations.
The core components of Smart Manufacturing Technology include:
- Cyber-Physical Systems (CPS): Integration of computation, networking, and physical processes.
- Industrial Internet of Things (IIoT): Connects sensors, devices, and networks to capture data from manufacturing environments.
- Cloud Computing: Provides scalable computing resources and data storage for analytics and applications.
- Big Data Analytics: Analyzes vast amounts of data to drive evidence-based decision-making.
An example of smart manufacturing technology in action is the adoption of predictive maintenance systems. These systems utilize IoT sensors to constantly monitor equipment conditions, analyzing data for signs of wear or failure. The collected data enables timely maintenance activities, reducing downtime and increasing efficiency.
Exploring the applications of smart manufacturing technology, it's interesting to note that some factories have implemented digital twins - virtual models that mirror physical assets. These allow for simulations and analyses that can test changes in production without disrupting actual processes. Digital twins offer insights into potential improvements and can predict outcomes of various operational strategies before they are applied in real-life contexts.
Smart Manufacturing Technologies empower companies to keep up with rapidly changing market demands through agile and responsive production methodologies.
Smart Manufacturing Processes
Smart Manufacturing Processes are characterized by their ability to incorporate various advanced technologies to streamline production and enhance efficiency. This involves the use of digital tools and data analytics to facilitate more informed decision-making and real-time interventions within manufacturing activities.
Smart Manufacturing Examples
Understanding smart manufacturing examples can provide insight into how different industries adopt and implement advanced technologies. Below are some notable instances:
- Automotive Industry: Utilizes robotics for assembling vehicle parts, improving assembly speed and accuracy.
- Pharmaceutical Industry: Employs automation in packaging and inspection processes to ensure product quality.
- Food and Beverage Industry: Implements IoT for monitoring storage conditions to maintain freshness and safety.
Industry | Technology Used |
Automotive | Robotics |
Pharmaceutical | Automation |
Food & Beverage | IoT Monitoring |
An example in the manufacturing landscape is the use of AI-driven quality control systems. By incorporating machine learning algorithms, these systems can detect defects in products at various stages of production, ensuring that only high-quality items reach consumers.
Smart manufacturing often leads to reduced energy consumption and waste, contributing to more sustainable industrial practices.
Diving deeper into how smart manufacturing functions, consider the role of real-time data analytics. By leveraging this technology, factories can optimize their supply chain management. Data from suppliers, production lines, and logistics can be analyzed to predict potential delays and optimize inventory levels, ensuring seamless operations. This level of predictive analysis contributes to a more resilient manufacturing process, capable of adapting to market fluctuations swiftly.
Smart Manufacturing and Future Trends
As you delve into the realm of Smart Manufacturing, it's crucial to explore how future trends could shape this dynamic field. With technological advancements continually evolving, the manufacturing industry stands on the brink of a transformative era.
Emerging Technologies in Smart Manufacturing
Several emerging technologies are playing a pivotal role in the evolution of smart manufacturing, offering manufacturers numerous opportunities to innovate and improve efficiency. Key trends include:
- 5G Connectivity: Facilitates faster and more reliable communication between devices, supporting real-time data transmission.
- Edge Computing: Places data processing closer to the source, reducing latency and improving decision-making speed.
- Augmented Reality (AR) and Virtual Reality (VR): Enhances training and troubleshooting by providing immersive and interactive environments.
Taking a deeper look at 5G technology, it’s noteworthy that its implementation in manufacturing can support up to one million devices per square kilometer. This high-density connectivity enables seamless data flow across smart factories, allowing for unprecedented levels of automation and control. This connectivity paves the way for innovations like autonomous vehicles within the factory floor, optimizing logistics and productivity.
Data-Driven Decision Making
Data-driven decision-making has become a cornerstone of smart manufacturing, enabling manufacturers to utilize vast amounts of data to gain insights and improve processes. The role of data analytics in manufacturing encompasses:
- Predictive Analytics: Uses historical data to predict future outcomes, assisting in proactive maintenance and demand forecasting.
- Real-time Analytics: Provides instant feedback on operations, enabling quick adjustments and optimization.
- Descriptive Analytics: Offers insights into past operations and performance patterns.
An example of data-driven decision-making is the use of real-time analytics in production lines. For instance, manufacturers can adjust production speeds and resource allocation based on current demand and machine performance, optimizing efficiency and reducing downtime.
The integration of data analytics in manufacturing not only boosts operational performance but also enhances the quality of the final product.
smart manufacturing - Key takeaways
- Smart Manufacturing Definition: Integration of advanced technologies like IoT, AI, and robotics to enhance manufacturing efficiency and adaptability.
- Core Elements: Involves IoT devices, data analytics, AI, and automation to optimize production processes.
- Examples: Smart sensors in automotive factories for monitoring and reducing defects; predictive maintenance systems using IoT sensors.
- Technologies in Engineering: Connects digital tools with traditional processes for efficient production, including CPS, IIoT, and cloud computing.
- Industry Applications: Automotive uses robotics; pharmaceuticals employ automation; food industry implements IoT for quality control.
- Emerging Trends: Includes 5G for enhanced connectivity, edge computing for faster processing, and AR/VR for training in manufacturing.
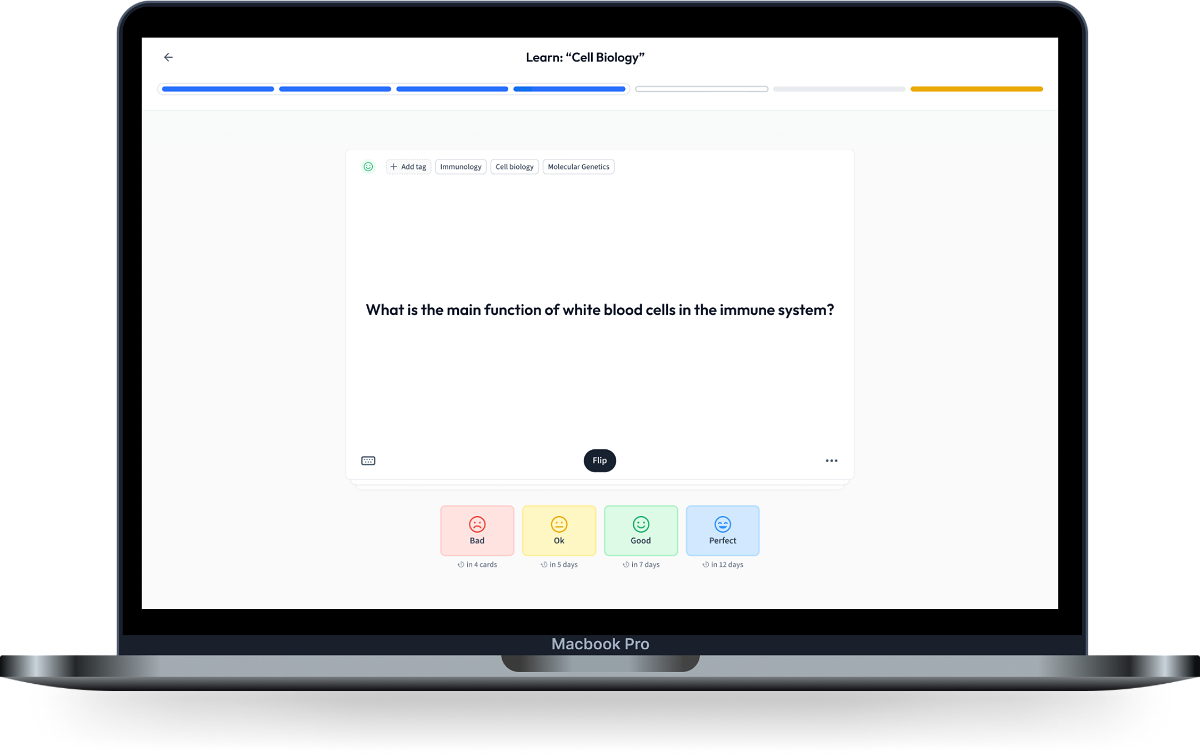
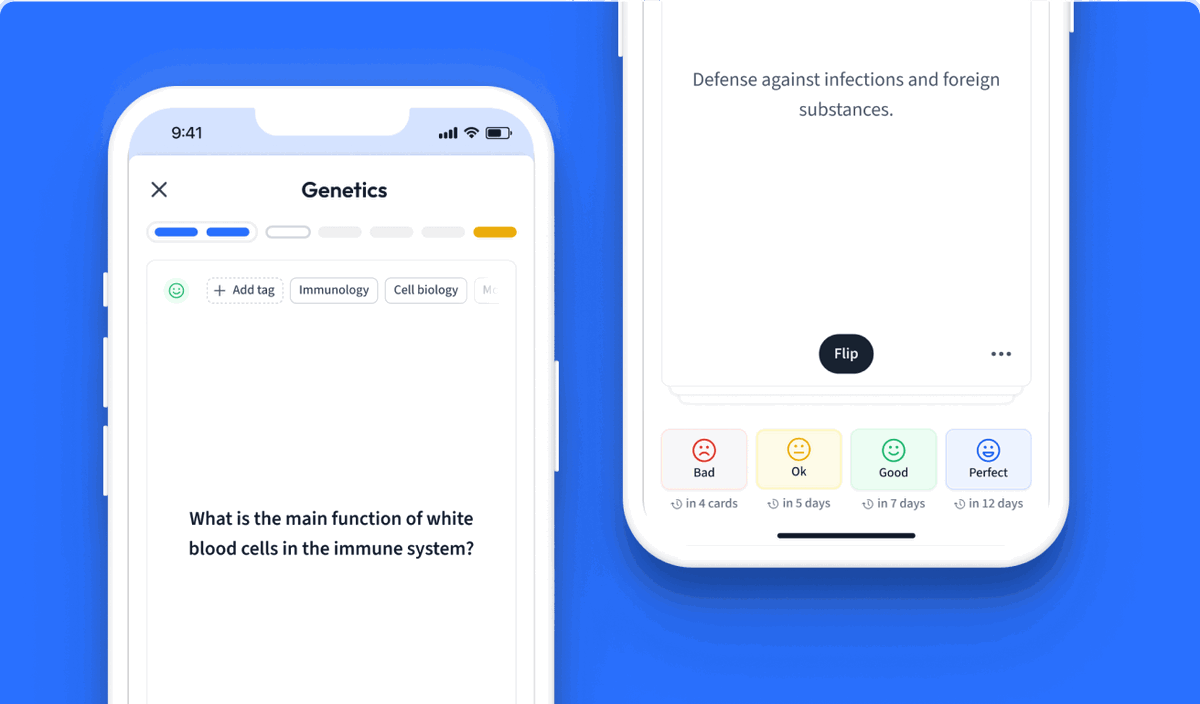
Learn with 12 smart manufacturing flashcards in the free StudySmarter app
We have 14,000 flashcards about Dynamic Landscapes.
Already have an account? Log in
Frequently Asked Questions about smart manufacturing
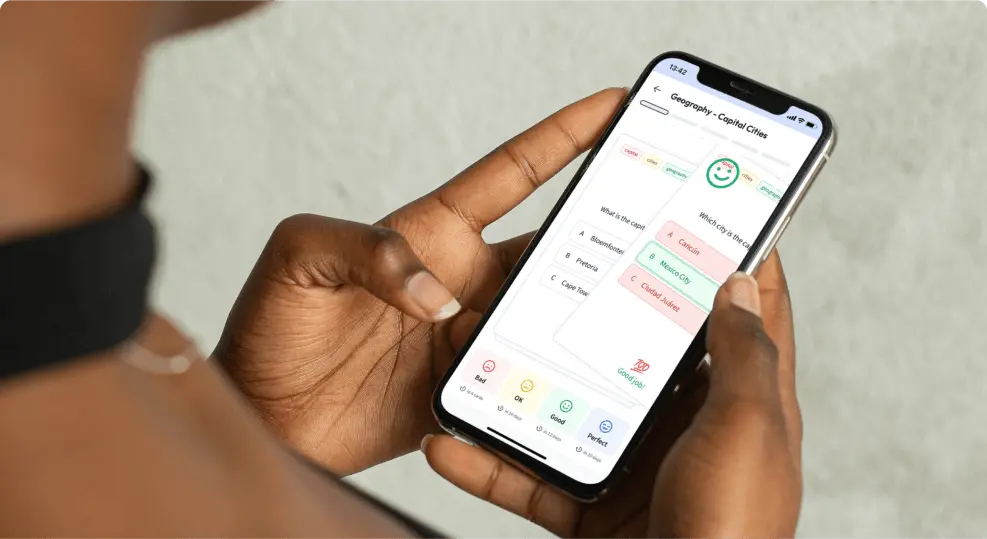
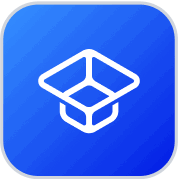
About StudySmarter
StudySmarter is a globally recognized educational technology company, offering a holistic learning platform designed for students of all ages and educational levels. Our platform provides learning support for a wide range of subjects, including STEM, Social Sciences, and Languages and also helps students to successfully master various tests and exams worldwide, such as GCSE, A Level, SAT, ACT, Abitur, and more. We offer an extensive library of learning materials, including interactive flashcards, comprehensive textbook solutions, and detailed explanations. The cutting-edge technology and tools we provide help students create their own learning materials. StudySmarter’s content is not only expert-verified but also regularly updated to ensure accuracy and relevance.
Learn more