Jump to a key chapter
Understanding Statistical Process Control: A Comprehensive Overview
Before diving into the complex world of Statistical Process Control (SPC), you must first lay a strong foundation of understanding.SPC is a method used within the engineering field, particularly in manufacturing, to monitor and control quality during the production process.
Defining the Concept: Statistical Process Control Meaning
For you to grasp the Statistical Process Control meaning, let's break it down into two parts.The 'statistical' part refers to statistical analysis tools, including descriptive stats such as means and standard deviations, and inferential stats like trend analysis.
- Control Charts
- Variations
- Process Capability
A central character in this play of numbers is the control chart. It visually depicts whether a process is under statistical control. That is, no unusual fluctuations indicating problems are detected.
Discovering Statistical Process Control: Real Life Examples
To truly understand Statistical Process Control, real-world examples can be a valuable tool.Example: A beverage company using SPC to monitor the volume of soda in its bottles. If the filling machine is working correctly — within its specified limits, the volumes will follow a normal distribution. Utilizing control charts, the company can quickly identify if the machine starts overfilling or underfilling the bottles, indicating a problem with the machine.
Diving Into the Core: Components of Statistical Process Control Charts
A significant element of SPC is the control chart. It consists of several components:- Centre Line
- Upper Control Limit (UCL)
- Lower Control Limit (LCL)
- Data Points
- \( \overline{X} \) is the process mean,
- \( S \) is the standard deviation,
- \( UCL \) is the Upper Control Limit, and
- \( LCL \) is the Lower Control Limit.
Analysing the Advantages and Disadvantages of Statistical Process Control
In this section, we shall part the curtains on the advantages and disadvantages of implementing Statistical Process Control, aiming to clarify your understanding on the practical worth of this invaluable tool.The Upside: Benefits of Implementing Statistical Process Control
Implementing SPC offers benefits that enhance productivity, improve product quality and streamline manufacturing processes. Let's delve deeper into these benefits: Boosts Efficiency: SPC pinpoints inefficient processes, allowing you to quickly rectify these inefficiencies and improve your overall process efficiency. Enhances Product Quality: By continually monitoring your process with SPC, you can quickly identify and prevent errors. Reduces Costs: SPC assists in minimising production defects and reducing scrap and rework, subsequently saving cost. Customer Satisfaction: Superior product quality attained using SPC invariably leads to high customer satisfaction.The Downside: Limitations and Challenges of Statistical Process Control
While SPC offers several advantages, you should note that there can be challenges and limitations in implementation as well: Time and Resource Intensive: The implementation of SPC can be time-consuming and resource-intensive, as you may have to train the staff, collect and analyse data, and maintain the SPC system. Statistical Knowledge: Successful use of SPC requires a good understanding of statistical principles and methods, something not everyone possesses. Resistance to Change: Like with any change, you might encounter resistance from staff who are comfortable with existing processes. Misinterpretation: Not understanding the statistical methods used in SPC can lead to misinterpretation of data, causing incorrect decisions to be made. Despite these challenges, with appropriate planning, training, and execution, the adoption of SPC can significantly enhance your manufacturing process control. However, tailoring the application of SPC to fit your specific industrial needs could be necessary for optimal results.Uncovering the Application of Statistical Process Control in Various Fields
Let's shine a light on the versatility of Statistical Process Control (SPC) and uncover its significance in various industries, including manufacturing, quality control, and the service sector. This statistical method has not only created waves in the manufacturing industry but has also found its niche in other areas.Statistical Process Control in Manufacturing: A Close Look
In manufacturing, SPC is hailed as a hero. It can be the difference between a defunct product assembly line and a well-oiled machine. It serves as an invaluable tool for monitoring production processes and maintaining product quality. Application of SPC in manufacturing involves creating a control chart to monitor a particular process. Let's consider a company manufacturing car engines. The diameter of a piston hole — an integral parameter that requires critical precision for efficient working — can be monitored using SPC. Control limits are established, and measurements from the production process are plotted on the control chart. If the data points fall within these predefined control limits, the process is deemed to be in control. However, an indication of potential trouble arises when these points move outside of the control limits or exhibit unnatural patterns within the limits, which is a sign of special cause variation. This critical information allows manufacturers to investigate issues and fix them, often before significant problems occur. The result is a decrease in defects and an increase in product reliability.Application of Statistical Process Control in Quality Control
Quality control is an essential branch of manufacturing where SPC is extensively used. The primary goal is to ensure that the end product aligns with the specified quality standards. With SPC, you can continually monitor your process and take corrective action before defects occur. This proactive approach is far more productive than a reactive methodology, which only uncovers problems after they've happened. For example, in a chocolate factory, SPC can be used to monitor the weight of each chocolate bar produced, ensuring that it meets the advertised weight. Any variation signalling underweight or overweight bars can be swiftly identified and dealt with, resulting in less waste and enhanced customer satisfaction. Moreover, SPC in quality control isn't limited merely to product characteristics. It also applies to monitoring workflow timelines and adherence to schedules, significantly reducing the chances of oversights and major setbacks during production.Exploring the Impact of Statistical Process Control in the Service Industry
Despite its strong roots in manufacturing, SPC has also found valid applications in the service industry. In a service-oriented business, quality can be more subjective and harder to quantify than in manufacturing, yet is undeniably important. Many service industries use SPC to monitor various performance metrics. For example, in a call centre, SPC can be used to track the length of calls, waiting times or number of calls dropped. This data is valuable in assessing productivity and customer service. Consequently, improvements can be implemented based on these insights. In the hospitality industry, key performance indicators such as reservation errors, check-in and check-out times and room readiness can be monitored using SPC, significantly improving customer service standards and overall operational efficiency. In healthcare, SPC acts as a vital sign monitor, tracking aspects like admission rates, patient waiting times, and infection rates. By identifying trends and variations in these data, hospitals can optimise their processes, resulting in improved patient care and saving lives. By exploring the implementation of SPC in these varied sectors, it's clear that this process monitoring tool carries with it tremendous significance, having far-reaching implications in enhancing service delivery, quality control, and manufacturing.Tackling the Basics of Statistical Process Control in Engineering
In the realm of engineering, Statistical Process Control (SPC) conveys paramount significance. It's a problem-solving tool that uses statistics to understand process capability and performance, thereby driving effective decision-making. SPC is based on process data, making it an objective, defensible standard that can underpin all engineering decisions.Role of Statistical Process Control in Engineering Decisions
The primary role of Statistical Process Control in engineering is to provide a systematic, measurable, and transparent method for optimising the engineering process. It accomplishes this by using statistical techniques to understand, measure, and control variation in the process. An engineering process can be anything from the assembly of a device to the creation of a complex system. The phenomena that generate the data, the sources of variation, and their interplay in the process are all important. When the process is under control, you understand the pattern and nature of variation and are less likely to make wrong decisions based on randomness or perceived effects that are merely noise.Variation is a term used in statistics and process control to describe how far data points in a monitored process vary from the expected value (mean).
Engineering Success with Statistical Process Control: Useful Approaches
A successful implementation of SPC in engineering relies on various statistical techniques, appropriate selection of control charts, understanding the process and its variation, and integrating these insights into the decision-making process. Let's delve deeper into the main steps involved: Data Collection: Before you jump straight into the statistics, you need to collate meaningful and relevant data representing the process you wish to control. This could be measurements from a specific machine, the features of a product, or the time it takes to complete a process. Identifying Key Metrics: It's critical to identify the key processes and their input and output variables that need to be monitored. These could include parameters like cycle times, defect rates, costs, wastage, and customer satisfaction. Selecting the Right Control Chart: The next step involves choosing the appropriate control chart for your data. These charts can differ based on the type of data you're collecting (attributes or variables) and what aspect of the process you're measuring.Code to generate Control Charts in Python: import matplotlib.pyplot as plt import numpy as np def generate_control_chart(data, UCL, LCL, CL): plt.plot(data, marker="o") plt.axhline(y=UCL, color='r', linestyle='--', label='UCL') plt.axhline(y=CL, color='g', linestyle='-', label='CL') plt.axhline(y=LCL, color='r', linestyle='--', label='LCL') plt.legend(loc='upper right') plt.title("Control Chart") plt.show() sample_data = np.random.normal(0, 1, 100) generate_control_chart(sample_data, 1.96, -1.96, 0)Analyzing the Control Chart: Once your control chart is ready, you'll begin to monitor your process, identifying any points outside the control limits, or non-random patterns within the control limits, signifying potential problems with your process. Making Decisions: Armed with the derived insights, you can then make well-informed decisions on whether potential changes must be implemented or an ongoing change has been successful. By encapsulating these methods into the broader engineering cycle, SPC provides a pivotal pathway to optimise workflows, mitigate risks, enhance efficiency, and ultimately, bolster the quality of products or services delivered to the end-user.
Mastering Statistical Process Control: Comprehensive Guide for Students
Statistical Process Control (SPC) is a beneficial tool for maintaining and improving process quality in various industries. As a student learning this fundamental methodology, understanding its concepts and utilising them proficiently enriches your skillset and opens diverse avenues in the engineering world.Step by Step: Interpreting Statistical Process Control Charts
Before diving into the interpretation of Statistical Process Control Charts (also known as control charts), let's briefly define what they are. Control charts are statistical tools used to differentiate between common and special causes of variation in a process. By using these charts, you can understand if your process is predictable and stable or unpredictable and unstable. A control chart displays data points plotted over time and includes three horizontal lines - the centre line, and the upper and lower control limits (UCL and LCL). The centre line represents the average, while the control limits depict the allowable variation. An important concept in SPC charts is the use of control limits, calculated as:UCL = \( \overline{X} + 3\sigma\)
LCL = \( \overline{X} - 3\sigma\)
- Within Control Limits: If all data points are within the control limits and display a random pattern, the process is stable and predictable. It is then a process displaying only common cause variation.
- Outside Control Limits: If one or more data points fall outside the control limits, this indicates an unstable process. The variation observed is likely due to special causes, warranting further investigation.
- Non-random patterns within Control Limits: Even if all the points lie within the control limits, certain patterns – like seven points in a row on one side of the centre line or a steady upward or downward trend – can indicate an unstable process.
Landmark Study: Significant Examples of Statistical Process Control
Statistical Process Control has been used across various sectors to make significant improvements in quality and efficiency. Let's address a few notable examples: 1. The Healthcare Sector: In the United Kingdom, the NHS implemented SPC to monitor A&E waiting times with a goal to reduce delays. By plotting the patient waiting times on control charts, NHS could identify when significant changes occurred and investigate any special causes of variation. This led to the steady improvement of their system and improved patient turnaround times. 2. The Automotive Industry: Toyota, a leader in automotive manufacturing, has been known to incorporate SPC extensively. One case is their use of SPC in monitoring the thickness of brake pads. Deviations from the norm, identified through control charts, led them to investigate and uncover machine wear and tear, which was then promptly fixed. 3. The Electronics Industry: IBM implemented SPC in its manufacturing of circuit boards to control soldering quality. Control charts were used to monitor the process, reduce variation and yield significant savings and improved product quality. These profound examples emphasise the effectiveness of SPC in continuous quality improvement and optimisation in diverse sectors.Improving Your Understanding of Statistical Process Control: Useful Tips
Mastering Statistical Process Control takes practice, comprehension, and a curiosity to delve into its depths. Here are some tips to enhance your understanding:- Understand the Basics: Start by focusing on understanding the foundational concepts of SPC such as control charts, variation, standard deviation, process capability, and so on.
- Hands-on Practice: Theory is indispensable, but practice helps reinforce it. Use relevant tools or software to simulate basic SPC processes. Create and interpret control charts with different sets of data.
- Real-life Application: Look for real-life examples/evidence of SPC. Reading case studies and reports on how SPC is utilised in various sectors can provide invaluable insights and deepen your understanding.
- Participate in Workshops/Courses: There are multiple online and offline workshops or courses available that can provide a structured learning path for mastering SPC. Enrolling in one could prove beneficial.
- Peer Learning: Studying in a group often aids understanding. Discussing doubts, debating over interpretations, and working on projects with peers can enhance your learning experience.
Statistical Process Control - Key takeaways
- 'Statistical Process Control (SPC)' uses 'control charts' to identify variations in a process, where main components are 'Centre Line', 'Upper Control Limit (UCL)', 'Lower Control Limit (LCL)', and 'Data Points'. The centre line is the process mean or median, while UCL and LCL denote the range in which the process variations are considered normal.
- Advantages of Statistical Process Control include boosting efficiency by identifying and rectifying inefficiencies, enhancing product quality, reducing costs, and raising customer satisfaction.
- Disadvantages of Statistical Process Control include the time and resources required for implementation, need for understanding of statistical principles and methods, resistance to change from staff, and risk of data misinterpretation.
- Statistical Process Control finds application across various fields, from manufacturing and quality control to the service industry, helping to monitor production processes, maintain product quality and improve operational efficiency.
- In engineering, Statistical Process Control offers a systematic method for optimising processes, understanding and controlling variation using statistical techniques, and making informed decisions based on data analysis.
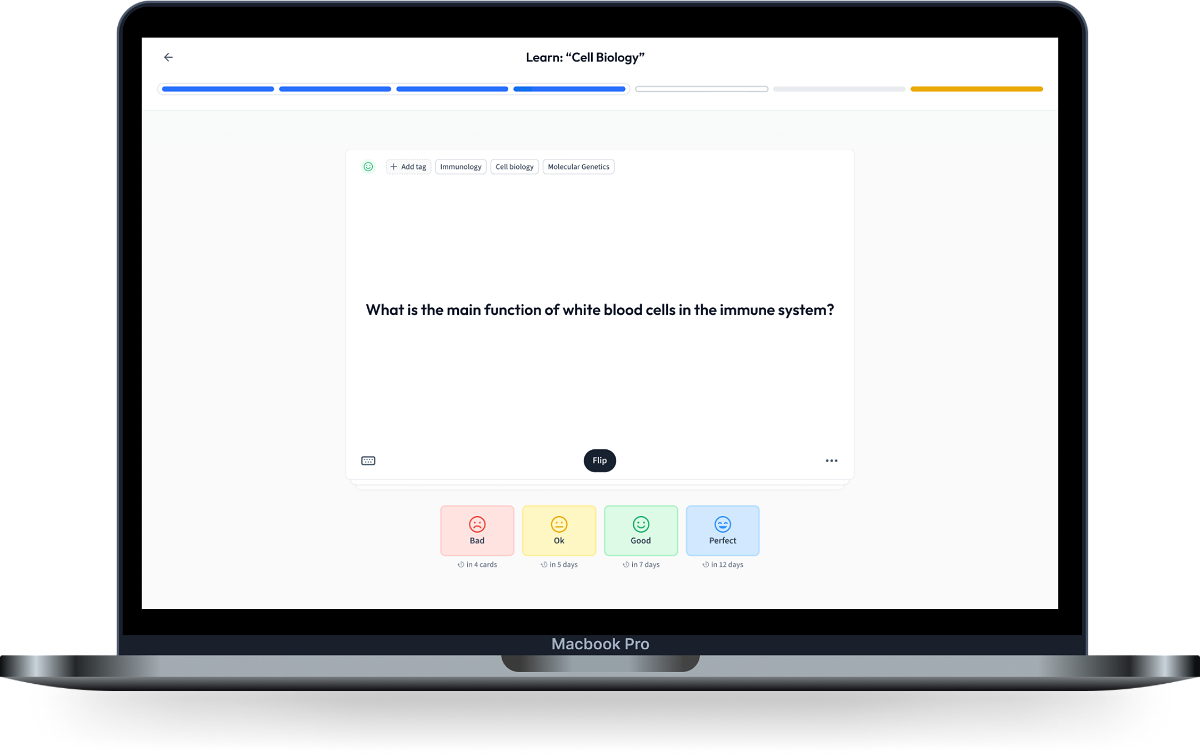
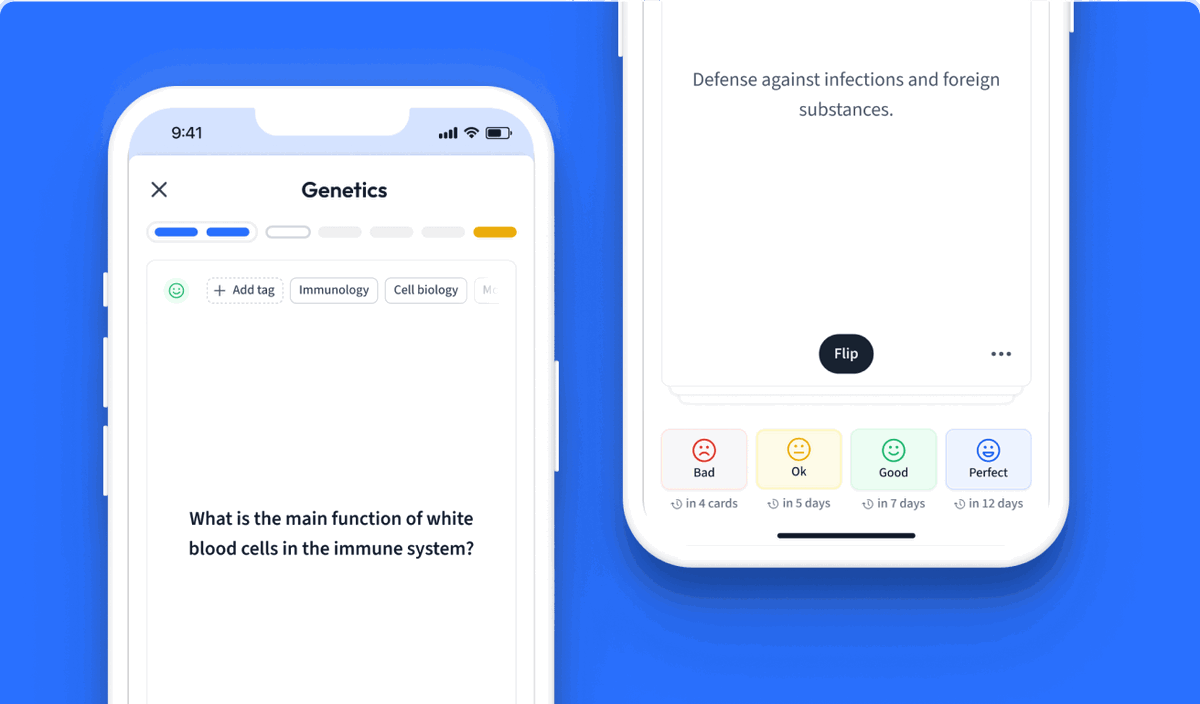
Learn with 15 Statistical Process Control flashcards in the free StudySmarter app
We have 14,000 flashcards about Dynamic Landscapes.
Already have an account? Log in
Frequently Asked Questions about Statistical Process Control
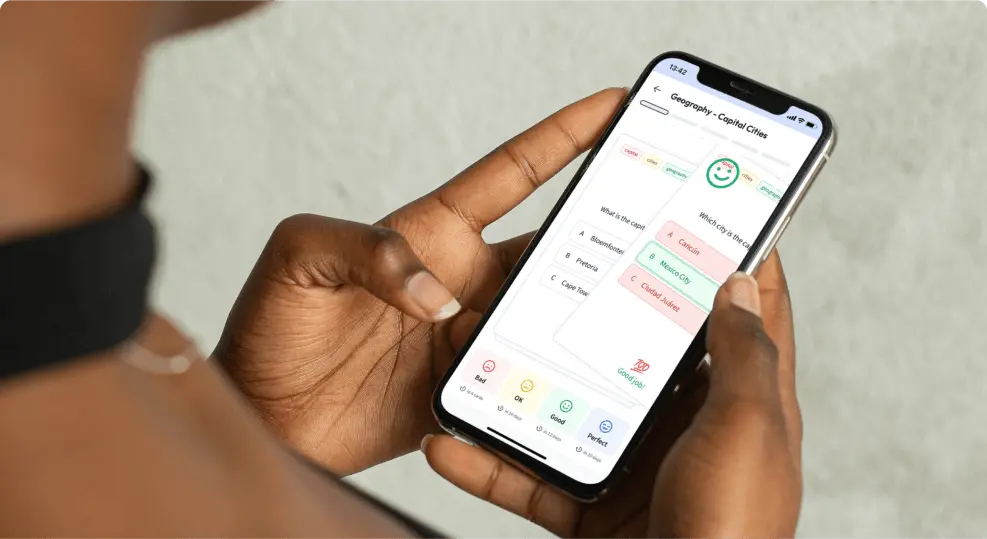
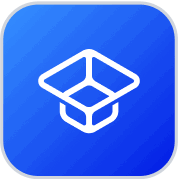
About StudySmarter
StudySmarter is a globally recognized educational technology company, offering a holistic learning platform designed for students of all ages and educational levels. Our platform provides learning support for a wide range of subjects, including STEM, Social Sciences, and Languages and also helps students to successfully master various tests and exams worldwide, such as GCSE, A Level, SAT, ACT, Abitur, and more. We offer an extensive library of learning materials, including interactive flashcards, comprehensive textbook solutions, and detailed explanations. The cutting-edge technology and tools we provide help students create their own learning materials. StudySmarter’s content is not only expert-verified but also regularly updated to ensure accuracy and relevance.
Learn more