Jump to a key chapter
Feedback Linearization Definition
Feedback linearization is a widely used control technique in engineering aimed at transforming nonlinear systems into linear ones. This process simplifies the analysis and design of control systems by eliminating nonlinear dynamics, making it easier to apply linear control methods.
Key Concepts in Feedback Linearization
To understand feedback linearization, you need to grasp the following essential concepts:
- Nonlinear Systems: Systems where the output is not directly proportional to the input. Standard examples include pendulums and nonlinear oscillators.
- Control Laws: Feedback rules applied to the system inputs to achieve desired outputs.
- Linearization: The process of approximating a nonlinear system with a linear one, especially around an operating point.
Consider a simple pendulum. The equations of motion are inherently nonlinear. By applying feedback linearization, you can transform these nonlinear equations into a linear form near the pendulum's stable equilibrium position, thereby making the control design much simpler.
Mathematical Approach to Feedback Linearization
Feedback linearization involves several mathematical steps:
- System Representation: Describe the nonlinear system using differential equations.
- Input Transformation: Find a transformation of input variables to cancel nonlinearities.
- State Feedback: Use state feedback to compute the transformed input, achieving a linear relationship.
- \(\frac{dx}{dt} = f(x) + g(x)u\)
- \(y = h(x)\)
Feedback linearization is particularly useful for systems where conventional linear control methods would otherwise fail due to inherent nonlinear characteristics.
Feedback linearization reveals deep insights into the control of nonlinear systems. For instance, the Lie derivative is instrumental in the procedure. It helps compute the required derivatives of the output function with respect to the input variables. The Lie Brackets and Lie derivatives help express the system's behavior in higher-order dimensions. A proficient understanding of differential geometry and algebra is beneficial when dealing with complex systems. The process offers an elegant solution to controlling robots, vehicles, and aerospace mechanisms, which usually possess nonlinear dynamics due to their mechanical structures.
Feedback Linearization Theory
Feedback linearization is a potent technique employed in control theory to convert nonlinear systems into linear ones. This transformation significantly aids in the application of linear control strategies, which are simpler and well-understood compared to their nonlinear counterparts. By canceling out the nonlinear parts of a system, the control design becomes straightforward.
Nonlinear Systems & Importance in Control
Nonlinear systems are prevalent in many engineering applications, such as robotics, aerospace, and automotive systems. Key characteristics of nonlinear systems include:
- Non-proportional relationship between input and output.
- Possibility of multiple equilibrium points and complex dynamic behaviors.
- Sensitivity to initial conditions.
Steps in Feedback Linearization
The process of feedback linearization encompasses several critical steps:
- Modeling: Define the nonlinear system through differential equations, which describe its dynamic behavior.
- Feedback Law: Calculate the control input that cancels contradictions in the nonlinear segments of the system.
- Transformation: Convert the altered system for which standard linear control methods can be easily applied.
- \(\frac{dx}{dt} = f(x) + g(x)u\)
- \(y = h(x)\)
Suppose you have a robotic arm. The arm's dynamic equations can be expressed as nonlinear due to joint frictions and nonlinear spring effects. By utilizing feedback linearization, you convert these equations into linear ones, which allows for the application of advanced linear control laws to achieve precise movement and position control.
In feedback linearization, understanding the concept of the Lie derivative is crucial. The Lie derivative involves calculating how a function changes along the flows of a vector field. This idea is instrumental in determining which nonlinear elements to eliminate through control input transformation. Specifically, a sequence of Lie derivatives of the output function regarding the dynamics can lead to a connectivity advantage, reducing system complexity.Moreover, understanding the interplay between nonlinear feedback and state variables is vital when implementing more advanced forms of control, such as sliding mode control. Here, methods from differential geometry help analyze and provide a blueprint for systematically designing control laws that linearize these intricate systems.
When implementing feedback linearization, always verify that the resulting linear system meets the desired criteria for controllability and observability before applying standard linear control techniques.
Feedback Linearization of Nonlinear Systems
In the realm of control systems engineering, feedback linearization plays a pivotal role. It serves as a crucial technique to address the complex nature of nonlinear systems by transforming them into linear systems. This facilitates the utilization of linear control methods, which are generally less complex and more predictable than nonlinear approaches.
Understanding Nonlinear Systems
A nonlinear system is characterized by a non-proportional relationship between input and output, often exhibiting complex dynamics such as multiple equilibria or sensitivity to initial conditions. Unlike linear systems, nonlinear systems cannot be easily represented with simple algebraic equations.
Key features of nonlinear systems include:
- Presence of saturation and hysteresis effects.
- Exhibition of oscillatory behavior without external periodic input.
- Complex dynamics that may lead to chaotic behavior.
Imagine controlling a spacecraft. The dynamics of the spacecraft are nonlinear due to gravitational forces and thruster inputs. By applying feedback linearization, you can achieve linear-like behavior in the control system, enabling precise maneuvers.
Implementing Feedback Linearization
The process of applying feedback linearization involves several careful steps:
- System Modeling: Represent the nonlinear system using differential equations that describe its state dynamics.
- Design Control Laws: Develop a feedback control law to compensate for nonlinear behavior.
- Input Transformation: Apply transformations that linearize the equations of motion.
Feedback linearization can greatly simplify the controller design but requires accurate modeling of system dynamics.
In-depth understanding of Lie derivatives and partial derivatives is essential in feedback linearization. The Lie derivative provides a framework to evaluate how a function changes along the flow of a vector field, which is crucial for canceling nonlinearity. Being adept at utilizing these mathematical tools allows you to effectively design control inputs that transform an intricate, nonlinear system into a linear equivalent. This understanding extends to the manipulation of computational algorithms in dynamic systems, rendering them more accessible to standard linear feedback control approaches. Mastery in such areas can significantly enhance system efficiency and robustness in real-world applications, such as robotics and aerospace engineering.
Feedback Linearization Control
Feedback linearization is a crucial method for transforming nonlinear systems into linear systems to facilitate the application of linear control techniques. In engineering control systems, eliminating nonlinear components ensures the predictability and simplicity of system behavior, enabling efficient design and implementation of control strategies.
Input Output Feedback Linearization
Input-output feedback linearization involves canceling nonlinear effects through the feedback of input signals, allowing the linearization of the system around a desired trajectory or equilibrium.This approach targets the output function directly, ensuring that the output adheres to a specified linear dynamics model by adjusting the inputs accordingly. The process involves the following steps:
- Identify the nonlinear dynamics governing the system.
- Develop a feedback law that negates these nonlinear dynamics.
- Transform the non-linear equations to yield linear dynamics.
The main objective of feedback linearization is to determine a control law \(u\) such that the relationship \(y = h(x)\) behaves in a linear fashion, where:\[\frac{dx}{dt} = f(x) + g(x)u\]with \(y = h(x)\) representing system output.
Effective feedback linearization requires a comprehensive understanding of differential equations and transformations to accurately determine and apply control laws.
Lie derivatives play a critical role in the process of input-output feedback linearization. By employing Lie derivatives, you can compute the derivative of the output function along the vector field defined by the system dynamics. This aids in formulating the appropriate feedback law that will cancel out nonlinearities in the system.For instance, in the computation of relative degree, a series of Lie derivatives are used to determine the order of derivatives of the output function with respect to time before the input appears explicitly. Efficient computation and understanding of these derivatives allow for better control design and improved system performance. Such methods are vital for implementing robust feedback control in systems like robotic manipulators and autonomous vehicles, where maintaining stability and predictability is paramount even in complex operational environments.
Feedback Linearization Example
To illustrate feedback linearization, let's consider a practical example involving a robotic arm, where the challenge is to control the arm's position and trajectory precisely.Suppose the robotic arm's motion is described by a nonlinear dynamic equation \(\frac{d^2q}{dt^2} = f(q, \frac{dq}{dt}) + g(q, \frac{dq}{dt})u\), where \(q\) represents angular position, and \(u\) is the control input. The goal is to transform this into a simpler linear form, such as \(\frac{d^2q}{dt^2} = a+ bu\), where \(a\) and \(b\) are constants.
By selecting an appropriate feedback law \(u = v - f(q, \frac{dq}{dt})\)/\(g(q, \frac{dq}{dt})\), we can derive an input-output relation that simplifies the nonlinearities. Such a transformation facilitates the application of linear control methods, resulting in efficient path tracking and positional accuracy.This process is valuable in fields requiring precision and responsiveness, such as robotics, where autonomous systems must adapt to varying conditions while maintaining control reliability.
feedback linearization - Key takeaways
- Feedback Linearization Definition: A control technique used to transform nonlinear systems into linear ones to simplify the control system design by eliminating nonlinear dynamics.
- Feedback Linearization Theory: Converts nonlinear systems into linear ones, enabling the use of simpler linear control strategies.
- Feedback Linearization Control: Employing feedback linearization to transform systems for applying linear control techniques, ensuring predictability and simplicity of system behaviors.
- Input Output Feedback Linearization: Involves the cancellation of nonlinear effects through feedback to linearize a system's output around a desired trajectory or point.
- Feedback Linearization of Nonlinear Systems: Essential for transforming nonlinear systems like robotic arms or spacecraft to linear equivalents for efficient control.
- Feedback Linearization Example: Demonstrates methods of simplifying robotic arm dynamics through feedback transformation into linear equations for precise control.
Learn faster with the 12 flashcards about feedback linearization
Sign up for free to gain access to all our flashcards.
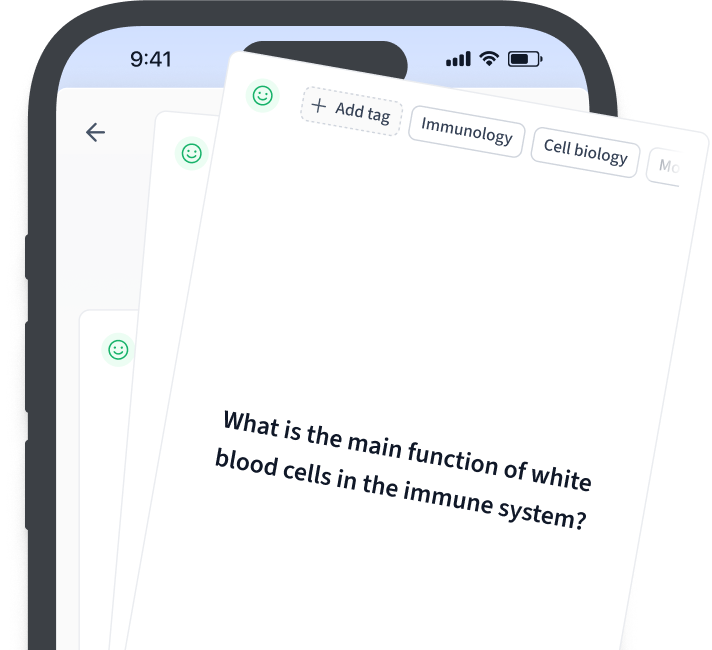
Frequently Asked Questions about feedback linearization
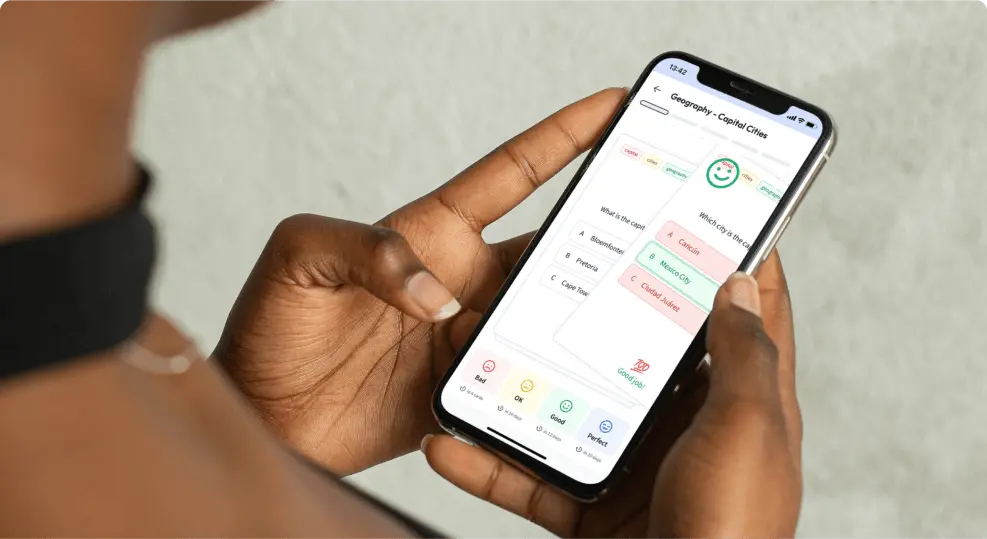
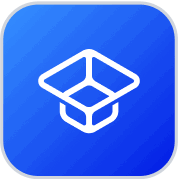
About StudySmarter
StudySmarter is a globally recognized educational technology company, offering a holistic learning platform designed for students of all ages and educational levels. Our platform provides learning support for a wide range of subjects, including STEM, Social Sciences, and Languages and also helps students to successfully master various tests and exams worldwide, such as GCSE, A Level, SAT, ACT, Abitur, and more. We offer an extensive library of learning materials, including interactive flashcards, comprehensive textbook solutions, and detailed explanations. The cutting-edge technology and tools we provide help students create their own learning materials. StudySmarter’s content is not only expert-verified but also regularly updated to ensure accuracy and relevance.
Learn more