Jump to a key chapter
Introduction to Geophysical Modeling
Understanding the Earth's physical characteristics and phenomena is vital for resolving environmental challenges. Geophysical modeling is a powerful technique that helps simulate the Earth's processes and system interactions using mathematical models. These models facilitate the analysis and prediction of geological and environmental phenomena. Such predictions are critical in fields like earthquake science, meteorology, and oceanography, impacting disaster management and environmental conservation efforts.
What is Geophysical Modeling?
Geophysical modeling leverages mathematical and computational techniques to represent and study various processes within the Earth. This can include phenomena like tectonic movements, climate dynamics, and ocean currents. These models involve intricate equations that describe physical properties and dynamics at different scales, from the Earth's crust to the atmosphere. A typical geophysical model might solve equations for heat transfer within the Earth's mantle or simulate atmospheric circulation patterns. These equations often involve complex variables and parameters which need to be calibrated against observed data to improve model accuracy.
Geophysical Modeling: The use of mathematical representations to simulate and understand Earth's processes and phenomena, aiding in prediction and analysis.
For example, climate models predict future weather patterns by simulating interactions within the Earth's atmosphere, oceans, and land surface. A basic climate model might solve the energy balance equation: \[Q_{in} - Q_{out} = \frac{dU}{dt}\] where \(Q_{in}\) is the incoming solar energy, \(Q_{out}\) is the outgoing infrared radiation, and \(\frac{dU}{dt}\) represents the change in energy within the system.
Applications of Geophysical Modeling
Geophysical modeling serves numerous practical applications that have a direct impact on human activities:
- Earthquake Modeling: Forecasting seismic activities by analyzing stress accumulation and release in tectonic plates.
- Meteorological Predictions: Enabling accurate weather forecasts through simulations of atmospheric dynamics.
- Oceanography: Understanding currents and their role in climate regulation and marine ecosystems.
Geophysical models become more accurate when robust observational data is incorporated, allowing for better calibration and validation of the simulations.
Delving deeper into geophysical modeling, one finds a variety of modeling methods tailored to specific geological and atmospheric needs. Consider, for instance, the finite element method (FEM), commonly used in simulating complex structures such as the Earth’s crust. This process involves dividing a large system into smaller, more manageable units, making it easier to calculate the properties within each section.Mathematically, FEM transforms a complex physical process into a set of algebraic equations represented by a matrix like: \[K \, u = F\]where \(K\) is the stiffness matrix, \(u\) is the displacement vector, and \(F\) represents the force vector. Further methodologies include Monte Carlo simulations, which randomly sample from probability distributions to provide statistical predictions, especially useful in scenarios like volcanic eruptions or groundwater contamination predictions.Such advanced techniques push the boundaries of what is possible, enabling a deeper understanding of Earth's complex systems.
Geophysical Modelling Techniques Explained
Learning about geophysical modeling techniques is crucial for anyone interested in predicting and understanding Earth's complex processes. Geophysics, as a field of Earth science, covers a wide range of phenomena using various modeling approaches designed to interpret and predict geological and environmental processes.
Forward and Inverse Modelling in Geophysics
In geophysics, two primary modeling strategies are often employed: forward modeling and inverse modeling. Both are vital in interpreting complex data related to Earth's properties and behavior. Forward modeling involves predicting measurable data based on a known geological model. For instance, if the subsurface properties, such as conductivity or density, are known, forward modeling can predict how these properties will affect observations like seismic waves or electromagnetic fields. The goal is to understand how different physical properties affect the results of measurements. In contrast, inverse modeling is utilized to infer unknown parameters in a geological model from observed data. It essentially works backward from the observations to pinpoint the subsurface properties. This is essential for tasks like estimating layer thicknesses in sedimentary basins or identifying potential resource deposits.
Consider an example involving seismic waves. In forward modeling, you might start with known subsurface properties, such as seismic velocities, and use these to compute predicted waves: \[d = G(m)\]where \(d\) is the data (seismic waves), \(G\) is the forward operator, and \(m\) is the model (subsurface properties). In inverse modeling, you start with observed seismic waves and solve for the model parameters: \[m_{\text{est}} = G^{-1}(d)\]where \(G^{-1}\) denotes the inverse of the forward operator, and \(m_{\text{est}}\) is the estimated model.
Think of forward modeling as playing a musical instrument where you know the notes and inverse modeling as figuring out the notes by listening to the music.
Techniques in Geophysical Modeling
Various techniques in geophysical modeling are employed to reveal and predict different subsurface features. These techniques are critical in different fields such as oil exploration, earthquake studies, and environmental monitoring. Some common techniques include:
- Finite Difference Method (FDM): Used to explore seismic wave propagation by transforming differential equations into algebraic equations solved in discrete time and space.
- Finite Element Method (FEM): Applied for simulating complex geological formations, using mesh generation to solve partial differential equations over polygons.
- Monte Carlo Simulations: Engaged for probabilistic assessments where uncertainty in geological models is represented by running numerous simulations.
- Ray Tracing: A popular method in seismic tomography used to estimate travel times by tracing the paths of seismic waves through the Earth's layers.
Diving deeper into Finite Element Method (FEM), we see it is particularly useful when dealing with the Earth's crust's complex structures. FEM divides the area of study into smaller sections called finite elements and solves simple equations within these elements. Its flexibility makes it suitable for irregular shapes and heterogeneous properties. The basic idea is to approximate field values at nodes of the elements, then establish equations for each element and systematically assemble a global stiffness matrix. Mathematically, the global equation often takes the form: \[K \, u = F\]where \(K\) is the global stiffness matrix, \(u\) is the displacement vector, and \(F\) is the force vector. This technique is crucial in simulating how stress and strain distribute within tectonic plates and for predicting potential fault lines. Moreover, FEM is compatible with computer simulations, enabling extensive calculations that would be otherwise impossible by hand, facilitating detailed models that significantly advance our understanding of seismic activities and geological stability.
Inverse Modeling Geophysics Overview
Inverse modeling in geophysics plays a crucial role in interpreting complex geophysical data by enabling the determination of subsurface properties from observed measurements. This method is imperative for understanding the Earth's structure and dynamics, guiding industries like oil exploration and natural disaster management to make informed decisions.
Applications of Inverse Modeling in Geophysics
Inverse modeling finds multiple applications within geophysics, allowing researchers to extract vital information about the Earth's substructure. Key applications include:
- Seismic Tomography: Through the inversion of seismic data, you can construct 3D models that reveal variations in Earth's velocity structure, helping in earthquake research and volcanic activity monitoring.
- Magnetotellurics: Used for exploring conductivity variations within the Earth's crust and mantle by analyzing electromagnetic fields, assisting in mineral and hydrocarbon exploration.
- Gravity Inversion: Helps in mapping density variations beneath the surface by interpreting gravity field measurements, crucial for mineral exploration and determining sedimentary basins.
For example, in seismic tomography, the process involves using inverse modeling on travel-time data from seismic waves. The fundamental equation is: \[t = \int_{path} \frac{1}{v(s)} \, ds\]where \(t\) is the travel time, \(v(s)\) is the velocity along the path, and \(ds\) is the path differential. The aim is to reconstruct the velocity structure \(v(s)\) from observed travel times \(t\).
An intriguing aspect of inverse modeling in geophysics is its use in full-waveform inversion (FWI). This advanced technique leverages the entire seismic waveform to extract subsurface properties, unlike traditional methods that focus solely on travel times. FWI attempts to minimize the difference between observed and modeled waveforms by adjusting model parameters, using an optimization algorithm. The mathematical objective function can be expressed as: \[\phi(m) = \frac{1}{2} \sum_{i=1}^{N} (d_i^{obs} - d_i^{calc}(m))^2\]where \(d_i^{obs}\) is the observed data, \(d_i^{calc}(m)\) is the calculated data from the model \(m\), and \(N\) is the number of data points. This iterative process results in high-resolution models that are invaluable for interpreting complex geological formations and behaviors.
Benefits of Inverse Modeling Geophysics
The adoption of inverse modeling in geophysics offers numerous advantages, greatly impacting scientific and industrial applications:
- Precision: The technique provides highly accurate subsurface models by effectively incorporating and analyzing diverse datasets.
- Resource Efficiency: By refining exploration and assessment processes, inverse modeling reduces the need for extensive drilling and surveying.
- Predictive Power: Facilitates robust hazard assessments, such as landslide or earthquake predictions, enabling preparedness and risk mitigation.
Inverse modeling is often computationally intensive, requiring powerful software and resources to solve the complex equations involved in geophysical data processing.
Geophysical Model Development Process
The creation of geophysical models is a complex process that integrates mathematical frameworks with empirical data to simulate Earth’s intricate systems. Each step in this process is crucial for ensuring that the models accurately represent the real-world phenomena they seek to depict.
Steps in Geophysical Model Development
The development of geophysical models involves several systematic steps, each contributing to the model's accuracy and applicability:
- Problem Definition: Clearly identify the geophysical process or phenomenon to be modeled, ensuring the objectives are well-defined.
- Selection of Model Framework: Determine the appropriate mathematical and physical principles, such as conservation of mass and energy, which form the foundation of the model.
- Data Acquisition and Processing: Gather relevant observational data, including seismic, magnetic, and gravitational measurements, ensuring high data quality and resolution.
- Model Formulation: Translate the physical and mathematical principles into equations. For example, in climate modeling, one might use: \[\frac{\partial T}{\partial t} = -u \frac{\partial T}{\partial x} - v \frac{\partial T}{\partial y} + \kappa abla^2 T\]where \(T\) is the temperature, \(u, v\) are velocity components, and \(\kappa\) is thermal diffusivity.
- Model Implementation: Use software platforms to input equations and data, running simulations to generate output.
- Calibration and Validation: Compare model outputs with observational data to ensure accuracy, adjusting parameters as necessary.
- Interpretation and Application: Analyze model results to draw conclusions and guide decision-making in applications such as resource management and disaster prediction.
Consider developing a volcanic eruption model which involves simulating the movement of magma. The model formulation step might use the Navier-Stokes equations to simulate magma flow: \[\rho \left( \frac{\partial \mathbf v}{\partial t} + (\mathbf v \cdot abla) \mathbf v \right) = -abla p + \mu abla^2 \mathbf v + \rho \mathbf g\]where \(\rho\) is the density, \(\mathbf v\) is the velocity vector, \(p\) is pressure, \(\mu\) is the viscosity, and \(\mathbf g\) represents the gravitational force.
It's essential to check that models remain computationally feasible without compromising on accuracy. Simplifying assumptions may sometimes be necessary.
Exploring deeper into the calibration and validation phase, this step is often the most challenging due to the complexities of the real-world data it engages with. Calibration involves adjusting model parameters until the model mimics real-world behavior accurately. This often requires iterative testing and may involve techniques like cross-validation where the dataset is divided, using part for calibration and another for confirmation. Validation is crucial, ensuring predictions made by the model stand up in different scenarios. This often employs various metrics to test the model's reliability, such as: - Root Mean Square Error (RMSE): Provides a measure of the differences between values predicted by the model and the values observed. - Nash-Sutcliffe Efficiency: Used to assess the predictive power of hydrological models, given by: \[NSE = 1 - \frac{\sum_{i=1}^{n}(Q_o - Q_m)^2}{\sum_{i=1}^{n}(Q_o - \overline{Q_o})^2}\]where \(Q_o\) is the observed value, \(Q_m\) is the modeled value, and \(\overline{Q_o}\) is the mean of observed values. Successful calibration and validation ensure the model's results can be trusted for predictive applications, ultimately enhancing scientific understanding and decision-making capabilities.
Challenges in Developing Geophysical Models
Creating geophysical models entails various challenges that require skilled handling:
- Data Limitations: Access to high-quality, extensive data is crucial but often scarce due to geographic and financial constraints.
- Complexity in Processes: Many Earth processes are nonlinear and chaotic, demanding sophisticated algorithms to capture their dynamics accurately.
- Computational Demands: High-resolution models can be computationally intensive, requiring significant time and resources to run effectively.
- Parameter Uncertainty: Natural systems contain inherent uncertainties; hence models must be robust enough to handle variability and provide reliable forecasts.
An interesting challenge is handling nonlinearity, which is prevalent in many geophysical phenomena. Nonlinear systems, such as weather patterns or tectonic movements, can exhibit unpredictable behavior and sensitivity to initial conditions. To manage this, ensemble modeling is often used. This involves running multiple simulations with varied initial conditions and averaging the results to improve accuracy and offer insight into potential outcomes.Mathematically, this relates to finding solutions that balance these dynamic systems, often optimizing equations under changing conditions. Advanced mathematical techniques, such as bifurcation theory, are sometimes employed to understand these nonlinear systems better.Addressing these challenges involves a holistic approach, combining robust data handling, advanced algorithm implementation, and continuous model testing and revision to ensure reliability.
geophysical modeling - Key takeaways
- Geophysical Modeling: Utilizes mathematical models to simulate Earth's processes, aiding in prediction and analysis of geological phenomena.
- Forward and Inverse Modeling in Geophysics: Forward modeling predicts data based on known models; inverse modeling deduces model parameters from observations.
- Geophysical Modeling Techniques: Techniques include Finite Difference Method, Finite Element Method, Monte Carlo Simulations, and Ray Tracing.
- Inverse Modeling in Geophysics: Used to interpret data and determine subsurface properties, supporting industries like oil exploration and disaster management.
- Geophysical Model Development Process: Involves problem definition, model formulation, data processing, calibration, and validation to ensure model accuracy.
- Challenges in Geophysical Modeling: Include data limitations, complexity of processes, computational demands, and parameter uncertainty.
Learn faster with the 12 flashcards about geophysical modeling
Sign up for free to gain access to all our flashcards.
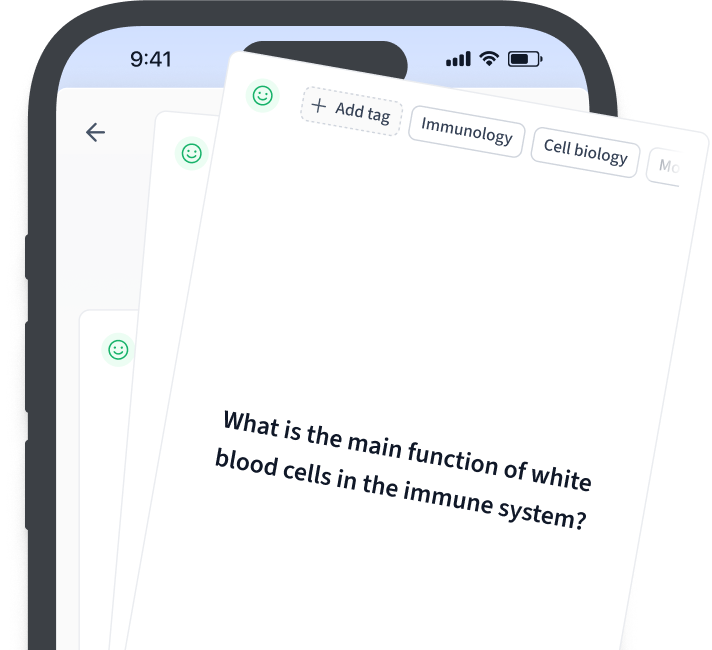
Frequently Asked Questions about geophysical modeling
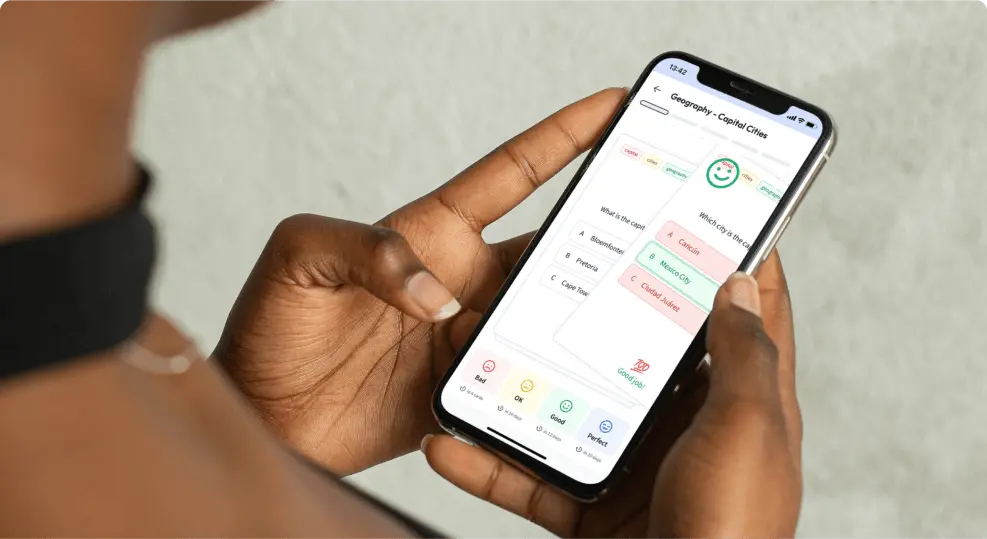
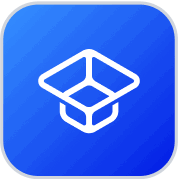
About StudySmarter
StudySmarter is a globally recognized educational technology company, offering a holistic learning platform designed for students of all ages and educational levels. Our platform provides learning support for a wide range of subjects, including STEM, Social Sciences, and Languages and also helps students to successfully master various tests and exams worldwide, such as GCSE, A Level, SAT, ACT, Abitur, and more. We offer an extensive library of learning materials, including interactive flashcards, comprehensive textbook solutions, and detailed explanations. The cutting-edge technology and tools we provide help students create their own learning materials. StudySmarter’s content is not only expert-verified but also regularly updated to ensure accuracy and relevance.
Learn more