Jump to a key chapter
Biomarker Identification Definition
Biomarker identification is the process of detecting and analyzing biological markers, known as biomarkers, which are measurable indicators of biological processes, conditions, or diseases. These markers play a vital role in understanding various physiological states and can be utilized in diagnosing diseases, predicting prognosis, and monitoring therapeutic responses.Biomarker identification is crucial for personalized medicine, as it helps in tailoring treatments to individual patients based on their unique biomarker profiles. The ability to identify specific biomarkers can significantly advance healthcare by providing more accurate diagnoses and effective therapies.In this section, biomarker identification will be explored in detail, covering its definitions, methods of detection, and its significance in modern medical practices.
Biomarker: A biomarker is a distinct biological molecule, gene, or characteristic that is measurable in biological samples like blood, tissues, or bodily fluids. It serves as a sign of a biological process, disease, or pharmacologic response to a therapeutic intervention.
Methods of Biomarker Identification
There are several methods used in the identification of biomarkers, each with its unique attributes and applications. Here is a list of commonly used methods:
- Genomic Approaches: These involve the study of an organism's entire genetic material or specific genes to identify potential biomarkers linked to diseases.
- Proteomic Approaches: These focus on the analysis of the protein composition of cells or tissues to discover disease-related proteins that can act as biomarkers.
- Metabolomic Approaches: This method examines the chemical processes involving metabolites, offering insights into the metabolic changes associated with diseases.
- Imaging Techniques: Advanced imaging technologies are employed to visualize and quantify biomolecular interactions and structures related to disease processes.
Example: An example of biomarker identification in action is the use of the PSA (Prostate-Specific Antigen) test. PSA is a protein produced by the prostate gland, and its levels can be measured in the blood. Elevated levels of PSA can indicate prostate cancer, benign prostate hyperplasia, or prostatitis. Hence, PSA serves as a biomarker for prostate-related health assessments.
Always consider the context and biological significance of a biomarker when evaluating its potential use in diagnosing or treating diseases.
Understanding how biomarkers are discovered involves delving into complex laboratory techniques and analytical processes. For example, in proteomic approaches, sophisticated instruments like mass spectrometers are utilized to analyze complex protein mixtures in biological samples. These instruments can separate and identify thousands of proteins based on their masses and charges, enabling researchers to find proteins uniquely expressed in certain disease states.Genomic approaches often make use of next-generation sequencing technologies, allowing the rapid sequencing of large amounts of DNA, identifying genetic variations that may serve as biomarkers.On the cutting edge, systems biology integrates data from various -omics technologies, combining genomics, proteomics, and metabolomics, to construct comprehensive biological models that enhance biomarker discovery. This integrated approach allows for a more holistic understanding of the complex networks and pathways involved in diseases, leading to better biomarker identification and validation.
Biomarker Identification Methods
Biomarker identification is a critical component of modern medical research and practice. It encompasses both traditional and innovative techniques, which are employed to discover biomarkers that can lead to improved diagnostic tests, treatment plans, and overall patient outcomes. Each method has its characteristics, applications, and advantages in understanding and diagnosing diseases.
Traditional Biomarker Identification Techniques
Traditional biomarker identification techniques have paved the way for modern advancements in medicine. These techniques typically rely on established biological and chemical methods to detect and analyze potential biomarkers. Here are some primary traditional techniques:
- ELISA (Enzyme-Linked Immunosorbent Assay): This is a widely-used technique to measure proteins, hormones, and other substances in biological samples through antigen-antibody interactions.
- Western Blotting: A method used to detect specific proteins in a sample by using gel electrophoresis followed by transfer to a membrane and detection with antibodies.
- Immunohistochemistry: This technique involves staining tissues with antibodies to identify specific protein markers, providing insights into the presence and distribution of biomarkers in tissues.
- Polymerase Chain Reaction (PCR): PCR is used to amplify small segments of DNA, making it easier to identify genetic markers associated with certain diseases.
Example: A classic example of traditional biomarker identification is the use of ELISA to detect the presence of viral antigens, such as hepatitis B surface antigen (HBsAg), in blood samples, helping in the diagnosis of hepatitis B infection.
Traditional techniques are still widely used due to their robustness and established track record in many clinical laboratories.
Innovative Biomarker Identification Techniques
Innovative techniques in biomarker identification leverage new technologies and computational methods to overcome the limitations of traditional approaches. These techniques aim to enhance sensitivity, specificity, and efficiency. Below are some innovative techniques:
- Next-Generation Sequencing (NGS): This technology allows for rapid sequencing of entire genomes, providing a comprehensive analysis of genetic variations that may serve as potential biomarkers.
- Mass Spectrometry-Based Proteomics: A highly sensitive method used to detect and quantify proteins in complex biological matrices, identifying new protein biomarkers.
- Microarray Analysis: Utilizes chips that contain thousands of microscopic DNA spots, enabling the analysis of gene expression patterns linked to various diseases.
- Bioinformatics and Machine Learning: Computational tools and algorithms are employed to analyze large biological datasets, identifying patterns and potential biomarkers with high accuracy.
- CRISPR-Cas Technology: An innovative genome editing tool that facilitates precise manipulation and identification of genetic markers related to diseases.
With the rise of personalized medicine, innovative biomarker techniques are continuously evolving. Mass spectrometry-based proteomics has revolutionized the way proteins are studied, enabling the quantification of thousands of proteins simultaneously, which is essential for identifying subtle changes in protein expression linked to disease states.Next-generation sequencing has made it feasible to perform whole-genome sequencing in a matter of hours, offering a comprehensive view of genetic alterations across populations.Moreover, bioinformatics and machine learning are increasingly integrated with laboratory techniques to interpret multi-dimensional data efficiently. Computational models predict outcomes by looking for patterns within vast datasets, guiding researchers towards the identification of clinically relevant biomarkers. These advancements promise a future where diagnostics and treatments are custom-tailored to the individual patient’s biological profile.
Biomarker Identification and Validation
Biomarker identification and validation are critical processes in medical research and diagnostics. Identification involves discovering potential biomarkers, while validation ensures these biomarkers are reliable and relevant for clinical applications, such as disease diagnosis, treatment monitoring, and therapeutic breakthroughs.
Steps in Biomarker Identification and Validation
Conducting successful biomarker identification and validation involves several key steps. These steps are integral to developing biomarkers that are not only scientifically sound but also clinically useful.
- Discovery Phase: The initial phase in which potential biomarkers are identified through various techniques, such as genomic, proteomic, and metabolomic approaches.
- Analytical Validation: Involves confirming the test’s capability to measure the biomarker accurately and consistently, ensuring its technical reliability.
- Clinical Validation: Assesses the biomarker’s effectiveness in the clinical setting, focusing on its role in disease detection, monitoring, or prognosis.
- Clinical Utility Evaluation: Confirms the biomarker's applicability in real-world clinical practice, demonstrating its benefit to patients' health management.
- Regulatory Approval: Biomarkers need approval from regulatory bodies such as the FDA or EMA, ensuring they are safe and effective for clinical use.
Example: The process of identifying and validating the BRCA1 and BRCA2 gene mutations as biomarkers for breast and ovarian cancer risk involved extensive discovery, validation, and regulatory approval processes. These biomarkers now help in genetic screening and risk assessment.
Always consider the sensitivity, specificity, and reproducibility of a biomarker during the validation process to ensure its clinical relevance.
The process of biomarker validation can be complex and resource-intensive. During the discovery phase, researchers often use high-throughput technologies, which allow the analysis of thousands of potential biomarkers simultaneously. The emphasis in analytical validation lies in developing assays that are both sensitive and specific to the biomarker, often utilizing sophisticated analytical methods like liquid chromatography-mass spectrometry (LC-MS). During clinical validation, statistical analyses are conducted to evaluate the relationship between the biomarker and the clinical outcome, employing large cohort studies to establish clinical relevance. Regulatory approval involves submitting comprehensive documentation to bodies such as the FDA, including clinical trial data and performance metrics, highlighting the biomarker’s safety and efficacy.
Challenges in Biomarker Identification and Validation
The journey from biomarker discovery to clinical application is fraught with numerous challenges. These hurdles often arise due to the complexity of biological systems and regulatory requirements.
- Biological Variability: Variations in biomarker levels due to age, gender, ethnicity, or environmental influences complicate identification and validation processes.
- Technical Limitations: The sensitivity and specificity of current detection technologies might not be adequate for certain biomarkers, affecting their reliability.
- Cost: The extensive investment in time, money, and resources may hinder the exploration and validation of novel biomarkers.
- Regulatory Hurdles: Strict regulatory requirements can delay the approval and implementation of new biomarkers in clinical settings.
- Translational Challenges: Difficulties in translating laboratory findings into clinical applications prevent the effective use of potential biomarkers.
Robust bioinformatics tools can help analyze complex data more effectively, overcoming some challenges in biomarker discovery.
Biomarker Identification Machine Learning
Machine learning plays an instrumental role in the field of biomarker identification, offering advanced techniques to manage and analyze the vast amounts of biological data required to discover meaningful biomarkers. By leveraging algorithms and computational models, machine learning aids in identifying patterns and relationships within complex datasets that would be difficult or impossible to detect manually. This enhances the accuracy of biomarker predictions, improving diagnosis and treatment strategies.
Biomarker Identification Algorithms
Several machine learning algorithms are pivotal in biomarker discovery, each providing distinct capabilities in analyzing data. Here are some key algorithms frequently used:
- Support Vector Machines (SVM): An algorithm that classifies data by finding the optimal hyperplane that separates different classes. SVMs are effective in high-dimensional spaces and are frequently used in genomics.
- Random Forest: This ensemble learning method constructs multiple decision trees to improve classification accuracy and is particularly useful for handling large datasets with mixed data types.
- Neural Networks: Inspired by the human brain, neural networks learn complex patterns through layers of interconnected nodes. They excel in capturing non-linear relationships in data.
- k-Nearest Neighbors (k-NN): A simple, yet powerful algorithm that classifies data based on the proximity of data points to one another and is used in identifying biomarkers associated with diseases.
Example: In the context of cancer research, Support Vector Machines (SVM) have been used to develop models that predict the presence of specific mutations based on gene expression profiles, effectively identifying biomarkers indicative of cancerous states.
Understanding how machine learning algorithms work in biomarker discovery can provide deeper insights into their potential. Support Vector Machines (SVM) operate by mapping input data into a high-dimensional space where a linear separator is constructed. Given a set of training samples from two classes, an SVM aims to find the hyperplane that maximizes the margin between these classes.Mathematically, the function for the hyperplane in a two-dimensional space can be written as:\[w \times x + b = 0\]where w is the weight vector, x is the feature vector, and b is the bias. The choice of this hyperplane is crucial for effective classification.Random Forest works by creating a multitude of decision trees during training and outputting the mode of the classes (classification) or mean prediction (regression) of the individual trees. This method is extremely apt for datasets with a lush array of features, ensuring lower chances of overfitting.In complex biological datasets, these algorithms, along with computational power, enable the distillation of vast amounts of data into meaningful patterns that can flag potential biomarkers.
Remember, machine learning models require vast and well-curated datasets to truly harness their predictive potential.
Future of Biomarker Identification with Machine Learning
The future of biomarker identification using machine learning is promising, with advancements expected to refine processes and deliver even greater insights into disease mechanisms.Here are some evolving trends that could shape the future:
- Integration of Multi-Omics Data: Combining genomics, transcriptomics, proteomics, and metabolomics will provide a comprehensive view of biological pathways and improve biomarker discovery.
- Deep Learning Applications: Deep learning models, with their capacity to handle extreme data complexities, will likely lead to the identification of novel biomarkers through advanced pattern recognition.
- Automated Model Building: Automated machine learning (AutoML) could streamline the model development process, making it more efficient and accessible for researchers without extensive expertise in coding.
- Improved Interpretability: New algorithms focusing on model transparency will allow better understanding and trust in biomarker predictions, critical for clinical acceptance.
- Real-time Data Analysis: Enhanced computational power and algorithms could enable real-time analysis of data streams, helping in immediate biomarker identification and monitoring.
Continuous innovation in computational techniques will drive new discoveries in biomarker identification, paving the way for more individualized therapeutic approaches.
The integration of deep learning in biomarker identification is particularly exciting. Deep learning networks, such as Convolutional Neural Networks (CNNs) and Recurrent Neural Networks (RNNs), possess unique architectures that are capable of detecting intricate patterns in large datasets, something particularly suitable for imaging and sequential data.For instance, CNNs, with their convolutional layers, excel at processing input data with grid-like topology (such as 2D images) and can thus assist in identifying disease biomarkers from medical images. Their architecture, consisting of layers such as convolutional, pooling, and fully connected layers, allows for adaptive space transformation, enhancing feature extraction.Furthermore, the convergence of machine learning with cloud computing and data sharing platforms could democratize access to sophisticated analytical tools, empowering more researchers worldwide to contribute to biomarker discovery.In summary, as machine learning technologies continue to evolve, so too will their influence on the efficiency and effectiveness of biomarker identification.
biomarker identification - Key takeaways
- Biomarker Identification Definition: The process of detecting and analyzing measurable biological indicators (biomarkers) to assess biological processes or diseases, essential for personalized medicine.
- Biomarker Identification Methods: Includes genomic, proteomic, metabolomic approaches, and imaging techniques to discover disease-linked biomarkers.
- Traditional Techniques: Techniques like ELISA, Western Blotting, and PCR are foundational in identifying biomarkers but may lack modern sensitivity or throughput.
- Innovative Techniques: Employs next-generation sequencing, mass spectrometry, bioinformatics, machine learning, and CRISPR-Cas for enhanced biomarker discovery.
- Machine Learning in Biomarker Identification: Uses algorithms like SVM, Random Forest, Neural Networks for pattern recognition in complex datasets, improving diagnostics and therapeutic strategies.
- Biomarker Identification and Validation: Involves discovery, analytical and clinical validation, regulatory approval, and clinical utility assessment to ensure biomarker efficacy.
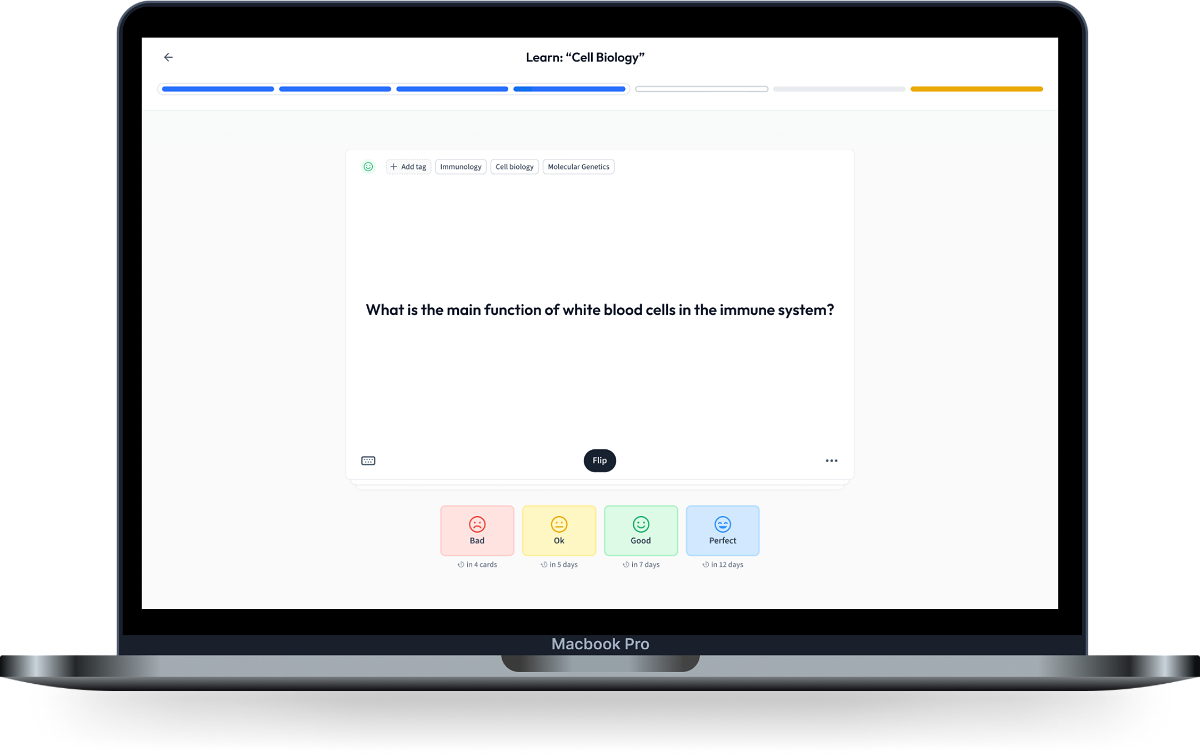
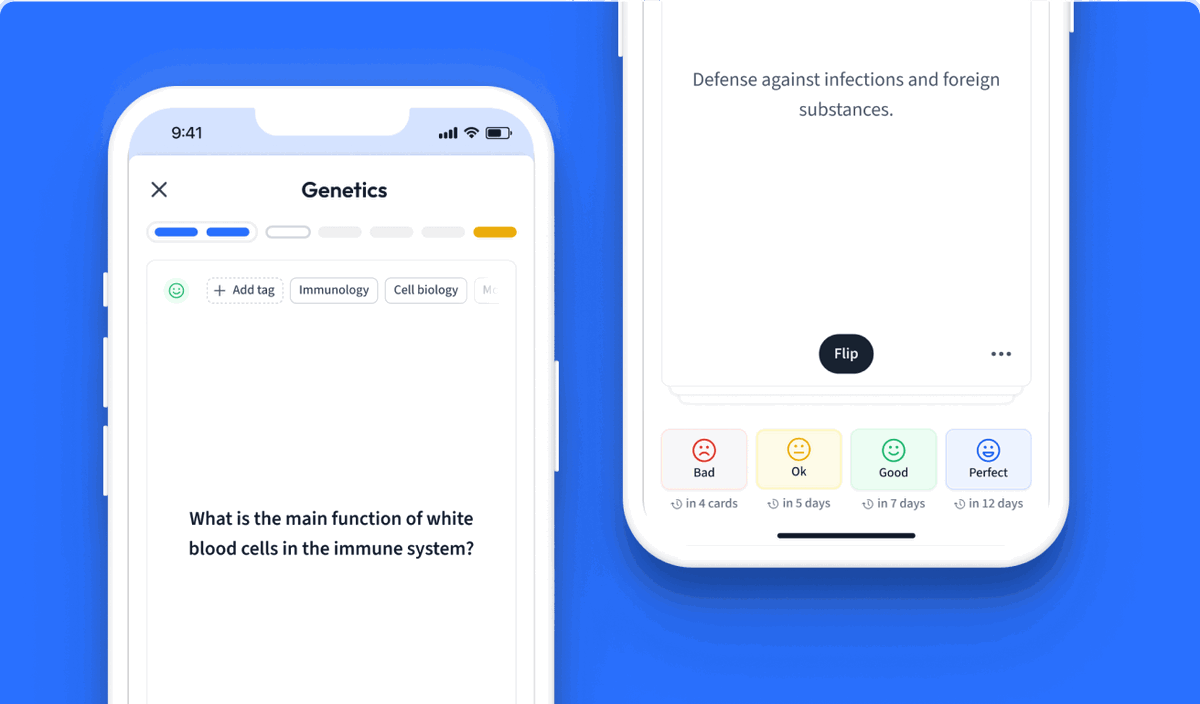
Learn with 12 biomarker identification flashcards in the free StudySmarter app
Already have an account? Log in
Frequently Asked Questions about biomarker identification
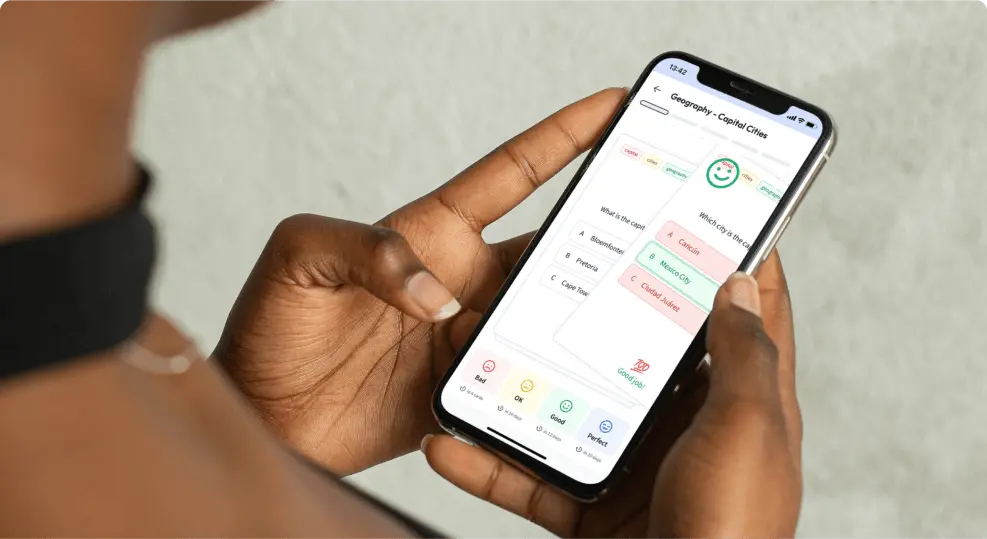
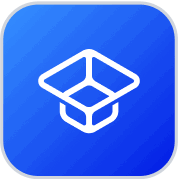
About StudySmarter
StudySmarter is a globally recognized educational technology company, offering a holistic learning platform designed for students of all ages and educational levels. Our platform provides learning support for a wide range of subjects, including STEM, Social Sciences, and Languages and also helps students to successfully master various tests and exams worldwide, such as GCSE, A Level, SAT, ACT, Abitur, and more. We offer an extensive library of learning materials, including interactive flashcards, comprehensive textbook solutions, and detailed explanations. The cutting-edge technology and tools we provide help students create their own learning materials. StudySmarter’s content is not only expert-verified but also regularly updated to ensure accuracy and relevance.
Learn more