Jump to a key chapter
Data Carving Definition
In the realm of digital forensics, data carving is an essential process used to recover files and evidences from digital media. This technique becomes particularly useful when a file system is damaged or data is missing, making file retrieval through conventional methods impossible.
Understanding Data Carving
Data carving does not rely on file system metadata, such as directory entries or partition tables, to locate files. Instead, it involves the process of scanning the raw binary data of digital storage devices and using known file signatures to retrieve files. These file signatures, often referred to as headers and footers, define the starting and ending points of a file type and are crucial in identifying and extracting data. The primary stages of data carving include:
- Identifying the file signature of the target files.
- Searching the binary data for matching patterns.
- Extracting the data between known headers and footers.
Data Carving: A data recovery technique that bypasses file system metadata and uses file signatures to retrieve files from damaged, formatted, or corrupt storage media.
Imagine a situation where you accidentally deleted photos from your digital camera. A software that utilizes data carving techniques could potentially recover these images by identifying the specific file signature common to JPEG files.
Advanced Techniques in Data Carving Beyond file signature analysis, advanced carving techniques integrate content-based approaches, which involve inspecting actual data within a file to improve accuracy. This can include pattern recognition and statistical analysis methods that understand structure-specific data patterns or keywords. Additionally, machine learning algorithms are being applied to predict file signatures dynamically and adapt to complex file structures, enabling higher precision in reconstructing files even with partial data loss.
Data carving can be computationally intensive and time-consuming, depending on storage size and the complexity of data structures.
Data Carving in Digital Forensics
Data carving is a critical technique used in digital forensics to extract and recover information from digital media when accessing file systems is unsuccessful. This technique is particularly beneficial in scenarios where data has been corrupted, deleted, or segmented across various parts of a storage device.
The Process of Data Carving
Data carving involves scanning the raw binary data present on a device's storage media, seeking file signatures that mark the beginning and end of recognizable file types. These signatures, often located in the headers and footers of files, assist in precise identification and extraction of data without relying on the file system's metadata.The stages of data carving typically include:
- File Signature Identification: Recognizing the binary patterns associated with specific file types.
- Signature Scanning: Searching the entirety of storage media for matching file signatures.
- Data Extraction: Pulling data between identified file segments to reconstruct files.
Data Carving: A method of recovering deleted or corrupted files by identifying and extracting data based on file signatures directly from raw disk data.
Consider using data carving software to recover MP3 files you've accidentally deleted from a USB drive. By recognizing the specific signature associated with MP3 files, the software searches the raw data for similar patterns and extracts these segments, reconstructing the deleted files.
As forensic methods advance, data carving has evolved beyond simple signature matching. Techniques now include content-based analysis, which inspects the internal structure of data to improve recovery accuracy. This approach, involving pattern recognition or statistical methods, helps in identifying files with partial data or unique formats.Furthermore, integrating machine learning into data carving allows adaptive learning of file signatures, even predicting unknown file patterns, and enhances recovery effectiveness when metadata is compromised or absent.
Although powerful, data carving can be a lengthy process, especially for large storage media, due to the exhaustive nature of scanning and data extraction.
Examples of Data Carving in Law
In legal scenarios, data carving becomes a pivotal tool for uncovering evidence from digital media, particularly when traditional data recovery methods fall short. It plays a significant role in legal investigations and court proceedings where access to hidden or deleted data can influence the outcome of a case.
Recovering Deleted Emails in Legal Cases
Legal cases often require the retrieval of emails that may have been intentionally deleted to conceal evidence. Data carving allows legal teams to reconstruct emails by identifying the unique file signatures of email formats such as .eml or .pst. This process can uncover:
- Concealed email correspondences relevant to the case at hand
- Evidence of tampering or deletion during litigation
- Critical timestamps verifying the sequence of events
Consider a case where an employee is suspected of sharing confidential company information. Using data carving techniques, legal investigators can recover deleted emails from the employee’s device, revealing critical evidence of breach of confidentiality agreements.
Recovery of Contracts from Damaged Devices
In situations where digital storage media is physically damaged, such as through fire or water damage, data carving can be instrumental in retrieving essential documents like contracts. This process involves:
- Analyzing raw data segments to locate recognizable document signatures like .docx or .pdf
- Reconstructing text data that has been corrupted or partially destroyed
- Providing documented evidence in disputes where original contracts are required
While data carving is highly effective, always ensure that the process respects privacy laws and proper authorization, as unauthorized data recovery can breach legal and ethical standards.
Legal teams are increasingly utilizing advanced algorithms in data carving to improve precision and reliability in recovering encrypted or byte-aligned data. These algorithms can enhance the understanding and reconstruction of files that standard carving techniques might miss. By deploying machine learning models, these processes learn to predict and align data patterns dynamically, offering better results, even in complex datasets often encountered in legal cases.
Data Carving Legal Aspects
Within the legal field, data carving is applied to extract pivotal evidence from digital sources, a task performed without reliance on high-level metadata. Valuable in scenarios ranging from intellectual property disputes to criminal investigations, it aids in uncovering information otherwise inaccessible, forming the backbone of evidence-based arguments in court.
Data Carving Methods in Legal Studies
Data carving methods in legal studies employ strategic approaches to retrieve necessary data from digital evidence. These methods typically focus on:
- Signature-based carving: Utilizing predefined signatures to identify file types, particularly useful when dealing with common document formats.
- Content-based approaches: Involving deeper inspection of data content to reconstruct files when signatures are absent.
- Advanced tools: Deployment of sophisticated tools like Scalpel to automate and refine the data carving process for efficiency and accuracy.
Consider researchers working on a study regarding corporate fraud investigations. Utilizing data carving methods, they can retrieve and analyze past corrupted email archives, providing insights on communication patterns used as evidence of fraudulent activity.
The evolution of data carving techniques in legal studies is seeing the integration of artificial intelligence to enhance recovery accuracy. AI-driven bots can dynamically identify data patterns, predict missing signatures, and adaptively reconstruct file systems. These bots increase the reliability of data recovery from heavily damaged sources, offering robust support in complex legal investigations.
Data Carving Challenges in Law
Despite its potential, data carving faces several challenges within the legal context. These include ethical considerations, technical limitations, and procedural constraints:
- Ethical and legal issues: Ensuring data privacy and compliance with relevant legislation is paramount, requiring strict adherence to data protection laws to prevent unauthorized recovery.
- Technical hurdles: Involves dealing with fragmented data and heavily encrypted files, which demand advanced techniques beyond traditional carving methods.
- Procedural adherence: Maintaining the integrity of retrieved evidence within legal standards to ensure admissibility in court without compromising the chain of custody.
When recovering data for legal purposes, engage with qualified forensic experts who are well-versed in the legalities and technical nuances of data carving.
data carving - Key takeaways
- Data Carving Definition: A data recovery technique in digital forensics that retrieves files from damaged or corrupt storage media by using file signatures, bypassing file system metadata.
- Data Carving Process: Involves stages like file signature identification, signature scanning, and data extraction, employing tools such as Foremost and Scalpel for automation.
- Data Carving in Law: Used for recovering evidence from digital media when conventional methods fail, crucial in legal investigations and court cases.
- Legal Methods: Signature-based and content-based carving, along with advanced tools, aid in reconstructing documents for legal research and case studies.
- Data Carving Legal Aspects: Focuses on extracting evidence for legal purposes, emphasizing compliance with privacy laws and maintaining data integrity.
- Challenges in Law: Ethical and technical challenges include ensuring data privacy, dealing with fragmented/encrypted data, and maintaining procedural integrity for legal admissibility.
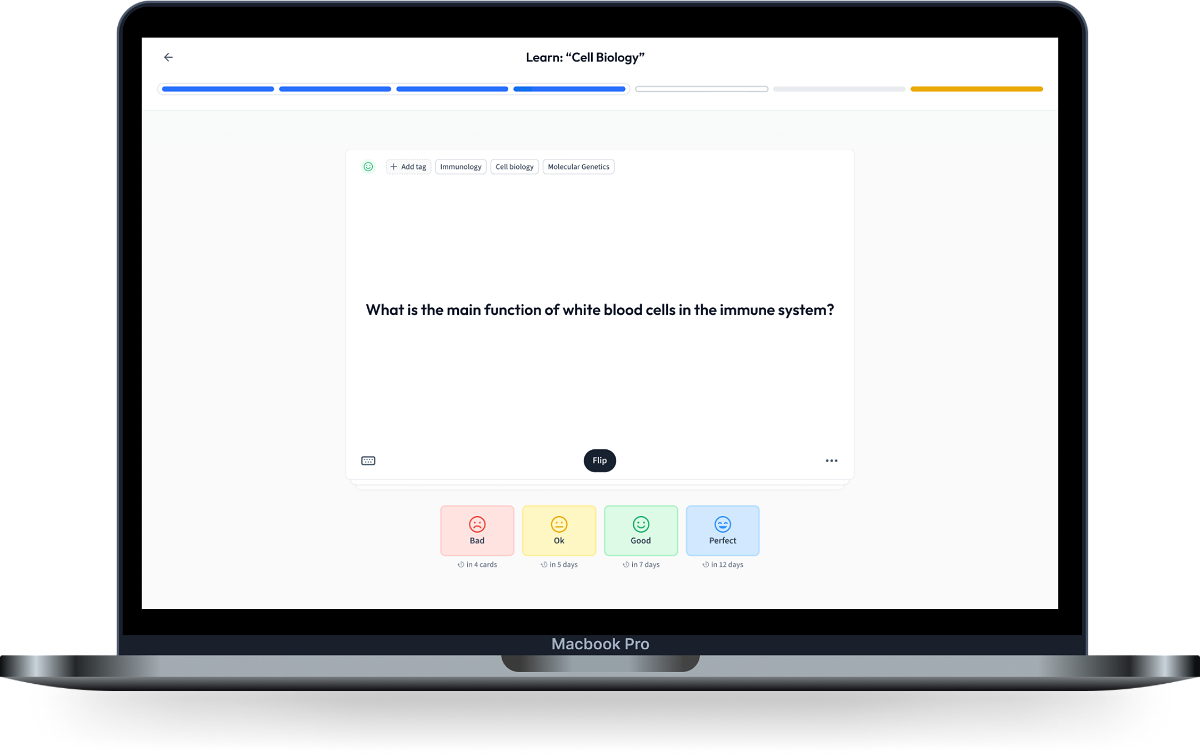
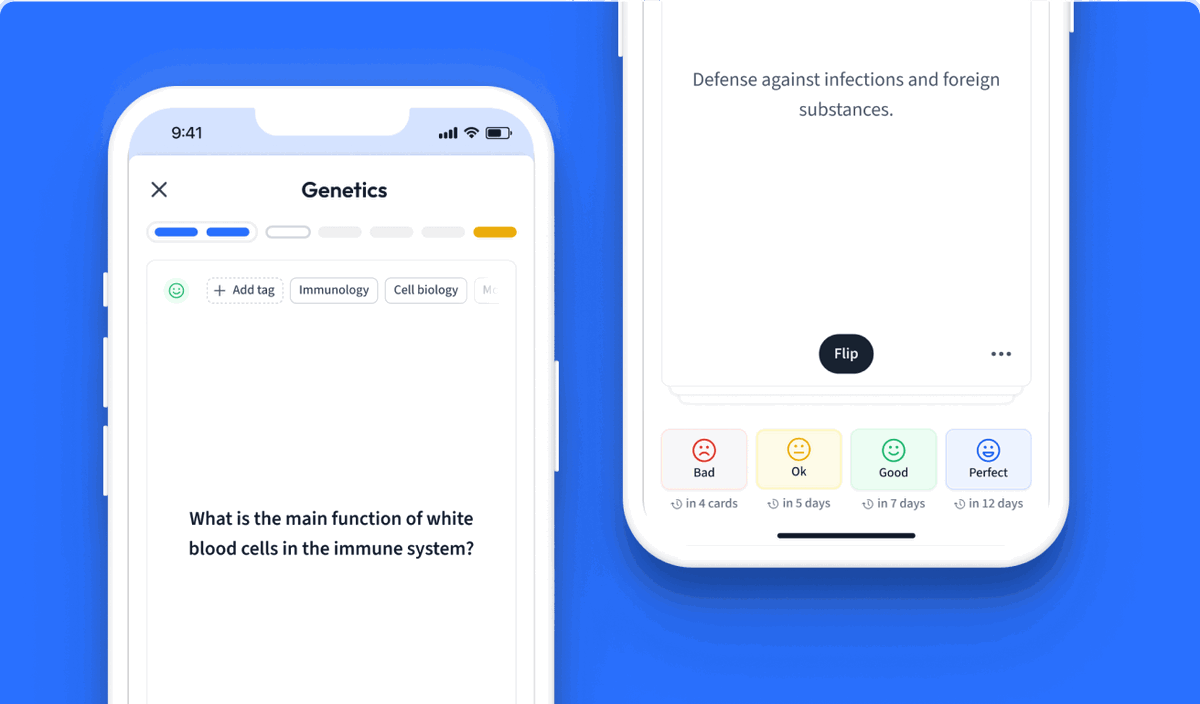
Learn with 12 data carving flashcards in the free StudySmarter app
We have 14,000 flashcards about Dynamic Landscapes.
Already have an account? Log in
Frequently Asked Questions about data carving
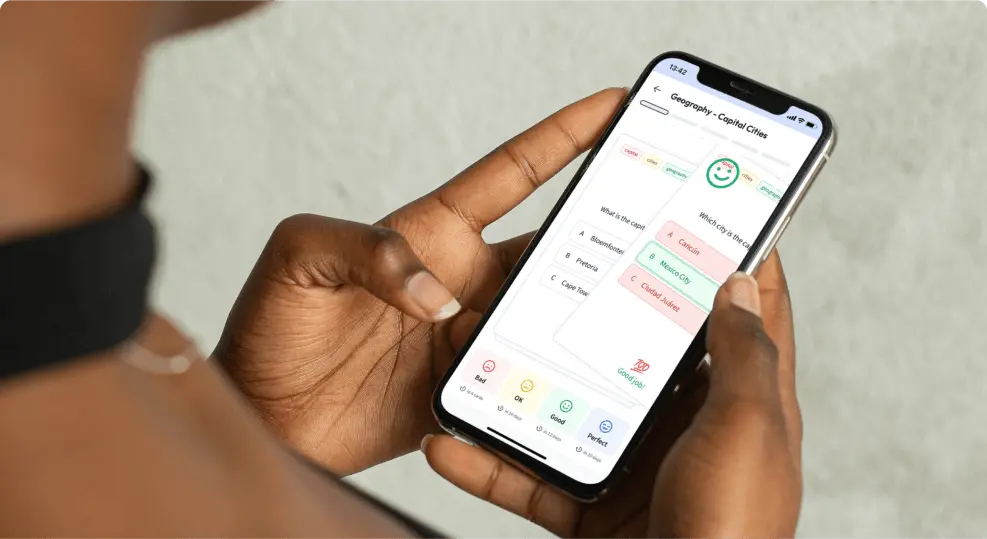
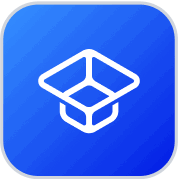
About StudySmarter
StudySmarter is a globally recognized educational technology company, offering a holistic learning platform designed for students of all ages and educational levels. Our platform provides learning support for a wide range of subjects, including STEM, Social Sciences, and Languages and also helps students to successfully master various tests and exams worldwide, such as GCSE, A Level, SAT, ACT, Abitur, and more. We offer an extensive library of learning materials, including interactive flashcards, comprehensive textbook solutions, and detailed explanations. The cutting-edge technology and tools we provide help students create their own learning materials. StudySmarter’s content is not only expert-verified but also regularly updated to ensure accuracy and relevance.
Learn more