Jump to a key chapter
What is Mathematical Statistics?
Mathematical statistics is a branch of mathematics that focuses on the analysis and interpretation of data. It combines principles from both mathematics and statistics to collect, review, summarise, and draw conclusions from data. This discipline is pivotal in various fields such as economics, biology, engineering, and social sciences, providing critical insights and decision-making tools.In this exploration, you'll learn about the foundational concepts of mathematical statistics, a subject rich with applications and methodologies that empower you to navigate and make sense of the vast amounts of data encountered in today's world.
Definition of Statistics in Mathematics
Statistics in mathematics refers to the science of collecting, analysing, interpreting, presenting, and organising data. It provides a framework for making inferences about the characteristics of large groups based on samples.
Statistics serves as the groundwork for empirical research across various scientific disciplines. By applying statistical methods, you can make educated guesses about a population from a sample, understand relationships within data, and estimate the probability of outcomes.
Introduction to Mathematical Statistics
Mathematical statistics is a crucial tool used by statisticians to model and draw conclusions from data. It involves the use of probability theory to interpret the frequency and distribution of data events. With the advent of big data and advanced computing, the importance and relevance of mathematical statistics have grown significantly.This introduction is not just about learning definitions and theories; it's about understanding how mathematical ideas shape the way data is analysed and utilised for practical decisions.
Key Concepts in Mathematical Statistics
Several key concepts form the foundation of mathematical statistics. Understanding these concepts is essential for analysing and interpreting data accurately:
- Probability: The study of how likely an event is to occur.
- Random Variables: Variables whose values result from the outcome of a random phenomenon.
- Probability Distributions: Mathematical functions that describe how probabilities are distributed over the values of a random variable.
- Statistical Inference: The process of using data to make estimates or predictions about a larger population.
- Hypothesis Testing: A method for testing a hypothesised parameter within a statistical model.
Example: Suppose you wish to determine the average height of students in a school. By selecting a sample group of students and measuring their heights, you apply statistical methods to estimate the average height of all students in the school. This process involves principles of both probability (how the sample represents the population) and statistical inference (drawing conclusions about the population based on the sample data).This example illustrates how mathematical statistics facilitates the understanding of data collected from the world around us, despite inherent uncertainties.
Further Exploration: One fascinating aspect of mathematical statistics is the Central Limit Theorem (CLT). It states that, under certain conditions, the sum of a large number of random variables, irrespective of their distributions, will approximately follow a normal distribution. This theorem is significant because it enables statisticians to apply the normal distribution - a well-understood probability distribution - to problems involving other, more complex distributions.For example, even if the individual measurements of height among students are not normally distributed, the average of a sufficiently large number of these height measurements will tend to be normally distributed, thanks to the CLT. This insight greatly simplifies many statistical analyses and interpretations.
Did you know? The term 'statistics' originally denoted the analysis of data about the state, deriving from the word 'state'. Over time, its scope has expanded drastically, encompassing data analysis across nearly every field of study.
Statistical Inference in Mathematical Statistics
Statistical inference is a cornerstone of mathematical statistics, focusing on analysing and interpreting data to make conclusions about a population. This process is fundamental to making decisions based on data samples, as it allows predictions and estimations about larger groups without the need to survey every individual.Through techniques like hypothesis testing and confidence intervals, statistical inference bridges the gap between observed data and practical conclusions, playing a crucial role across various domains like science, engineering, and economics.
Understanding Hypothesis Testing
Hypothesis testing is a statistical method used to decide whether there is enough evidence in a sample of data to infer that a certain condition is true for the entire population. At its core, it involves making an assumption (the hypothesis), then determining whether the data supports or rejects this assumption.The process is typically framed as a test between two competing hypotheses: the null hypothesis (\(H_0\)), which represents a statement of no effect or no difference, and the alternative hypothesis (\(H_A ext{ or }H_1\")), which represents a statement of effect, difference, or relationship.
Hypothesis Testing: A procedure in which sample data is analysed to decide whether to reject a hypothesis about the population from which the data was drawn.
Example: If you want to test the effectiveness of a new drug, the null hypothesis might be that the drug has no effect on patients (\(H_0 ext{: Drug is ineffective}\")). The alternative hypothesis might state that the drug does have an effective impact on patients (\(H_1 ext{: Drug is effective}\")). After collecting data from clinical trials, statistical methods are applied to determine whether the evidence sufficiently supports the alternative hypothesis over the null hypothesis.
The outcome of hypothesis testing is not binary (true/false), but rather a statement about the evidence: there's enough evidence to reject the null hypothesis, or not enough to do so, leaving it standing by default.
Confidence Intervals Explained
Confidence intervals provide a range of values within which the true value of a population parameter is expected to fall, with a certain level of confidence. They are based on the sample data and reflect the uncertainty around the estimate of the parameter. Unlike a single-point estimate, a confidence interval gives an interval that likely contains the true parameter, along with a measure of how confident we are in this range.The level of confidence (commonly set at 95% or 99%) indicates the degree to which the data supports the interval as containing the true parameter value. A 95% confidence interval means that if the experiment were repeated many times, approximately 95% of the confidence intervals calculated in this way would expect to contain the true parameter.
Confidence Interval: An interval estimate, derived from the statistics of the observed data, that likely contains the true parameter of the population with a specified level of confidence.
Example: Suppose a study aims to estimate the average height of men in a country. After measuring a sample of men, it calculates an average height of 175cm. It then calculates a 95% confidence interval of 173cm to 177cm. This means that, based on the sample data, it can be 95% confident the true average height of all men in the country falls within this range.This concept not only provides an estimate but also encapsulates the uncertainty inherent in sampling, offering a more comprehensive understanding of the data.
Confidence intervals and hypothesis testing are closely linked in that both are derived from understanding the variability inherent in sample data and using probabilistic models to make inferences about population parameters. However, their interpretations and applications can differ significantly. Where hypothesis testing is often used to make decisions or test theories, confidence intervals provide a range for where a parameter lies, offering insights into the precision of estimates and the possible values that parameter can take, based on the data at hand.Furthermore, the 'wideness' of a confidence interval can indicate the reliability of an estimate: narrower intervals suggest more precise estimates, whereas wider intervals suggest less certainty. Understanding both concepts is foundational in navigating statistical data analysis confidently.
Mathematical Statistics and Data Analysis
In the realm of mathematical statistics, the two components that stand out for their critical importance are probability and data analysis. These elements are interdependent and serve as the foundation for making informed decisions based on data.Through the application of statistical theories and methodologies, you're equipped to navigate through complex data, interpret various outcomes, and establish predictive models that are beneficial in a multitude of academic and professional fields.
The Role of Probability in Data Analysis
Probability plays a pivotal role in data analysis by providing a theoretical framework underpinning statistical inference. It allows for the measurement and quantification of uncertainty, enabling statisticians and data analysts to make sense of the data through the lens of likelihood and chance.Understanding probability principles is essential for interpreting data correctly, crafting robust statistical models, and minimising errors in predictive analyses.
Probability: A branch of mathematics concerning the analysis of random phenomena. The central objects of probability theory are random variables, stochastic processes, and events, which are mathematical abstractions of non-deterministic events or measured quantities that may either occur or not, depending on chance.
Example: If you flip a fair coin, the probability of observing a head or a tail is equal, represented as 0.5 for either outcome. This simple example illustrates the foundational concept of probability as the likelihood of occurring for a specific event.
Techniques for Effective Data Analysis in Mathematical Statistics
In the context of mathematical statistics, effective data analysis is contingent on the proper selection and application of techniques tailored to specific data types and intended outcomes. Among these, descriptive statistics, inferential statistics, and predictive models are paramount.Applying these techniques allows for the summarisation of big data into interpretable forms, estimation of population parameters from sample data, and forecasting of future observations.
Descriptive Statistics: These are brief descriptive coefficients that summarise a given data set, which can be either a representation of the entire population or a sample of it. Descriptive statistics are broken down into measures of central tendency and measures of variability (spread).
Example: Measures of central tendency include the mean, median, and mode, which detail the central point of a data set. Measures of variability include the standard deviation, variance, and range, which describe the spread of data points around the central point.For instance, calculating the mean age of students in a classroom gives a single value representing the average age, while the standard deviation indicates how spread out the ages are from this average.
Regression Analysis: A predictive modelling technique that assesses the relationship between two or more variables. The goal is to understand how the typical value of the dependent variable changes when any one of the independent variables is varied. This is especially powerful in forecasting scenarios where predicting future trends based on current data is crucial.Consider housing prices: a regression analysis might explore how various factors like size, location, or age affect the price. By inputting data from current sales, it can help predict the price of a house based on its attributes, providing valuable insights for buyers, sellers, and realtors alike.
The application of these statistical techniques not only aids in the analysis of past and current data but also in the prediction of future trends, making it a powerful tool in decision-making processes across disciplines.
Practical Applications of Mathematical Statistics
Mathematical statistics plays a vital role in various fields, extending far beyond the realm of academia. It provides tools and methodologies crucial for analysing data, drawing inferences, and making informed decisions. Through real-world examples and exercises for beginners, you're invited to explore the practicality of mathematical statistics in everyday scenarios.From health research to market analyses, this exploration underscores the indispensable nature of mathematical statistics in addressing complex problems and enhancing our understanding of the world around us.
Mathematical Statistics Examples in Real Life
Mathematical statistics applications permeate numerous aspects of daily life and professional fields. Here are a few examples where statistical methods play a crucial role:
- Healthcare: In medical research, statistical analyses help in understanding the effectiveness of treatments or drugs through clinical trials. Data from these trials are analysed to confirm if a new treatment performs better than standard treatments or a placebo.
- Economics: Economists rely on statistical models to predict market trends, assess economic policies' impact, and make forecasts about economic behaviours.
- Quality Control: Industries use statistical methods to monitor and maintain product quality. This includes using sample testing to infer the overall quality of goods produced.
- Environmental Science: Statisticians analyse environmental data to track climate change, study wildlife populations, and manage resources efficiently.
Example: Consider a study aimed at determining whether a new diet plan reduces blood pressure. Participants are divided into two groups: one follows the new diet, while the other continues with their regular diet. After a specified period, the blood pressure of participants from both groups is measured and compared. Statistical analysis of the collected data would involve hypothesis testing to evaluate the diet plan's effectiveness.In this scenario, statistical methods enable researchers to draw conclusions based on empirical data, illustrating how mathematical statistics aids in health-related decision-making.
Social media platforms use mathematical statistics to analyse user data and trends, helping improve user experiences through personalised feeds and targeted advertisements.
Mathematical Statistics Exercises for Beginners
For those new to mathematical statistics, starting with basic exercises is crucial for building a strong foundation. These exercises revolve around core concepts such as probability, descriptive statistics, and basic inferential statistics. Beginners can progress from simple probability exercises to more complex data analysis problems, reinforcing their understanding through practice.Here are beginner-friendly exercises designed to introduce fundamental statistical principles and techniques:
- Determine the probability of rolling a six on a fair die.
- Calculate the mean, median, and mode of a given set of data points.
- Create a simulated data set and plot it using basic graphing techniques.
- Conduct a simple hypothesis test using a small sample of data to determine if the average value differs from a known population mean.
Example: Suppose you have a dataset representing the ages of 50 randomly selected attendees at a conference. The data are as follows:
22 | 29 | 35 | 42 | 27 | 34 | 28 | 45 |
26 | 33 | 31 | 38 | 24 | 36 | 30 | 39 |
When embarking on statistical exercises, understanding the concept of a population versus a sample is key. A population includes all members of a defined group, whereas a sample is a subset of that population, chosen for analysis. The distinction is crucial in inferential statistics, where the goal is to draw conclusions about the population based on sample data. Through exercises, beginners can explore how sample size, variability, and sampling methods impact the accuracy and reliability of statistical inferences. These are fundamental aspects that form the bedrock of more advanced statistical studies and analyses.
Mathematical statistics - Key takeaways
- Mathematical Statistics: A branch of mathematics focused on data analysis and interpretation, combining principles from both mathematics and statistics.
- Definition of Statistics in Mathematics: The science of collecting, analysing, interpreting, presenting, and organising data to make inferences about large groups based on samples.
- Statistical Inference: Using data to make estimates or predictions about a larger population, fundamental in decision-making across various fields.
- Hypothesis Testing: A statistical method used to determine whether there is enough evidence in a sample of data to infer a condition for the entire population.
- Confidence Intervals: Range of values within which the true value of a population parameter is expected to fall, with a specified level of confidence.
Learn faster with the 12 flashcards about Mathematical statistics
Sign up for free to gain access to all our flashcards.
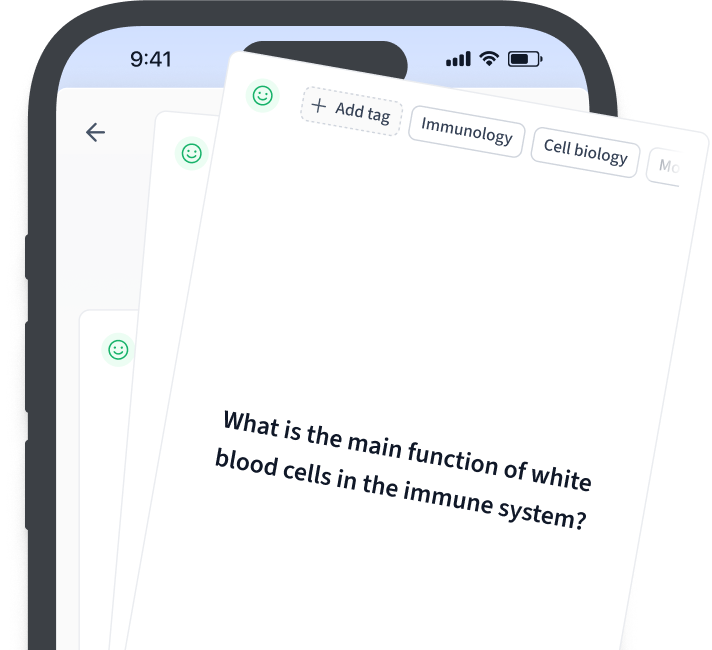
Frequently Asked Questions about Mathematical statistics
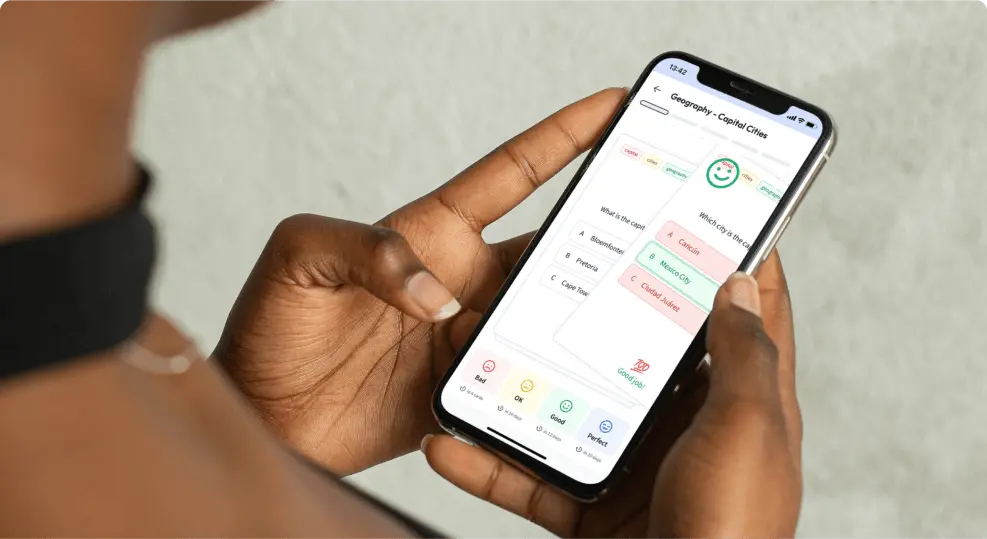
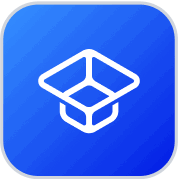
About StudySmarter
StudySmarter is a globally recognized educational technology company, offering a holistic learning platform designed for students of all ages and educational levels. Our platform provides learning support for a wide range of subjects, including STEM, Social Sciences, and Languages and also helps students to successfully master various tests and exams worldwide, such as GCSE, A Level, SAT, ACT, Abitur, and more. We offer an extensive library of learning materials, including interactive flashcards, comprehensive textbook solutions, and detailed explanations. The cutting-edge technology and tools we provide help students create their own learning materials. StudySmarter’s content is not only expert-verified but also regularly updated to ensure accuracy and relevance.
Learn more