Jump to a key chapter
What is Matching Theory?
Matching Theory is a fascinating area of mathematics that revolves around finding suitable pairs from two sets of items, based on specific criteria. It has practical applications in various fields, including economics, where it can determine optimal allocation of resources.
Understanding Matching Theory Definition
Matching Theory is a branch of combinatorial mathematics that deals with the problem of creating pairings between elements of two sets such that the pairings meet specified criteria.
The foundation of Matching Theory lies in its ability to facilitate decision-making processes, ensuring that the outcomes are as favourable as possible for all parties involved. This involves analysing preferences, resources, and other important factors.
The Principals of Matching Theory
The core principles of Matching Theory are centred on optimality and efficiency. Here is a breakdown:
- Stability: A matching is stable if there are no two elements that would prefer each other over their current matches.
- Optimality: A goal of matching is often to find the 'best possible' pairs based on given criteria.
- Complexity: Understanding the computational complexity of different matching problems is crucial for determining feasible solutions.
In real-life applications, achieving a perfect match might be challenging due to the complexity and varying preferences involved.
Matching Theory Explained with Simple Examples
Consider the classic example of matching applicants to jobs. In this scenario, each applicant has a list of preferred jobs, and each job has a list of preferred applicants. Using Matching Theory, one can determine an optimal matching where both applicants and jobs are paired in the most satisfactory manner possible.
A deeper look into Matching Theory reveals the famous Gale-Shapley algorithm, also known as the deferred acceptance algorithm. This algorithm guarantees to find a stable match between two equally sized sets. It operates by one set (say, applicants) proposing to members of the other set (jobs) according to their preferences. If a job receives more than one proposal, it rejects all except its highest-ranked applicant. This process continues until all applicants are matched, ensuring a stable outcome.
Another illustrative example of Matching Theory is the pairing of students to schools. In many countries, sophisticated algorithms based on Matching Theory are employed to allocate school places efficiently. These algorithms take into account the preferences of both students and schools, aiming to create the most satisfactory pairings.
In conclusion, Matching Theory is a powerful mathematical tool with wide-ranging applications. From optimising job placements to streamlining school admissions, it plays a crucial role in solving allocation dilemmas in a fair and efficient manner.
Fundamentals of Matching in Graph Theory
Graph theory, a vital component of discrete mathematics, encompasses the study of graphs, mathematical structures used to model pairwise relations between objects. A significant part of this study is dedicated to matching, which aims at finding a way to pair vertices of a graph under certain conditions.Matching in graph theory is not just an abstract concept; it has significant applications in real-world problems, ranging from organising efficient transport routes to solving allocation issues in various economic sectors.
Introducing Perfect Matching in Graph Theory
In the realm of graph theory, perfect matching represents an ideal state where every vertex in one part of a bipartite graph is connected to exactly one vertex in the other part, with no vertex left unmatched. This concept is crucial in many practical applications, including job assignments, where every person is matched to a job without any overlaps or omissions.The mathematical representation of perfect matching can be expressed as a subset of the graph's edges, ensuring that every vertex is incident to exactly one edge of the subset.
Perfect matching is a special case of matching where the goal is not just to match as many vertices as possible, but to ensure every vertex is included in the matching.
Differentiating Between Matching and Perfect Matching
While both matching and perfect matching are fundamental concepts within graph theory, they serve different purposes and have different constraints. A matching is a set of edges without common vertices, potentially leaving some vertices unmatched. In contrast, a perfect matching includes every vertex of the graph, pairing them in an exclusive one-to-one correspondence.This distinction is crucial in applications where the goal is either to maximise the number of matches (general matching) or to ensure that every participant or resource is optimally allocated (perfect matching).
Mathematical Matching Theory Fundamentals
Understanding the core principles of Mathematical Matching Theory involves a dive into its foundational formulas and algorithms. One such fundamental theorem is Hal’s Marriage Theorem, which provides a condition for when a perfect matching exists in bipartite graphs.The theorem states: For a bipartite graph \(G = (X, Y, E)\), a necessary and sufficient condition for a perfect matching is that for every subset \(S\) of \(X\), the size of the neighbourhood \(N(S)\) is at least as large as the size of \(S\). Mathematically, this is expressed as \(|N(S)| \geq |S|\) for all subsets \(S\) of \(X\).This criterion is instrumental in determining the feasibility of perfect matchings and guides the development of algorithms aimed at finding such matchings.
Delving deeper into the complexities of Mathematical Matching Theory reveals sophisticated algorithms like the Edmonds’ matching algorithm, also known as the blossoms algorithm. This algorithm efficiently finds a maximum matching or maximum weight matching in a graph, dealing adeptly with the challenges posed by odd-length cycles, known as blossoms, within non-bipartite graphs.Edmonds' algorithm is a testament to the rich interplay between theoretical insights and practical applications in graph theory. It underscores the importance of rigorous mathematical underpinnings in solving complex real-world problems through sophisticated computational methods.
Applications of Matching Theory
Matching Theory extends beyond the confines of mathematics and finds applications in various real-life scenarios. This theory aids in resolving complex pairing problems, ensuring optimal matches between elements from different sets based on specific criteria. These applications span across numerous fields such as economics, computer science, and healthcare, demonstrating the versatility and significance of Matching Theory.
Real-Life Applications of Matching Theory
Real-life applications of Matching Theory often involve scenarios where there is a need to match items or individuals from two distinct groups without necessarily having a direct one-to-one correspondence. This includes examples like organ donor and recipient matching, student allocations to schools, and matching drivers with passengers in ride-sharing platforms. The efficient solutions provided by Matching Theory in these contexts significantly improve outcomes for all parties involved.
The Nobel Prize in Economic Sciences 2012 was awarded to Alvin E. Roth and Lloyd S. Shapley for the theory of stable allocations and the practice of market design, a testament to the profound impact of Matching Theory in real-world applications.
Matching Theory Application Examples in Various Fields
Matching Theory’s versatility allows for its application across a wide range of fields, each with unique challenges and requirements:
- In healthcare, Matching Theory is used in allocating organs to patients in a way that maximises compatibility and optimises outcomes.
- In education, algorithms based on Matching Theory help in assigning students to schools or universities according to preferences and criteria, ensuring fairness and efficiency.
- The labour market benefits from Matching Theory by optimally pairing job seekers with available positions, considering both the employers’ requirements and the job seekers' preferences.
- In online platforms, from ride-sharing to dating apps, Matching Theory guides the pairing of users based on preferences and availability, enhancing user satisfaction and service efficiency.
A compelling example of Matching Theory in action is the organ donation system used in many countries. This system relies on sophisticated algorithms to match donors with recipients based not only on medical criteria such as blood type and tissue compatibility but also on urgency and geographical location. This matching process increases the chances of success for transplants and saves lives by efficiently allocating available organs.
Exploring further into the application in the labour market, Matching Theory underpins the design of many job matching platforms and career services. Platforms utilise complex algorithms developed from Matching Theory principles to assess the fit between job descriptions provided by employers and the profiles or resumes submitted by job seekers. These algorithms analyse skills, experience, location preferences, and other vital factors to facilitate the best possible matches, thus streamlining the hiring process for both parties. This application underlines the significant impact of Matching Theory on improving labour market efficiency and job satisfaction rates.
Advancements and Challenges in Matching Theory
Advancements in Matching Theory have played a crucial role in the development of algorithms that optimise resource allocation and pairing in a variety of fields. This journey from theoretical mathematics to practical applications has not been without challenges, reflecting the dynamic nature of this domain.This exploration captures both the evolution of Matching Theory and the current hurdles faced by researchers and practitioners, providing insight into the future trajectory of this fascinating area of study.
The Evolution of Matching Theory Over the Years
The progression of Matching Theory is a testament to the adaptability and depth of mathematical exploration. Early concepts of matching found in the works of mathematicians like Leonhard Euler paved the way for a more structured approach, epitomising the potential of mathematical theory in solving real-world problems.Fast-forward to the 20th century, the introduction of the Gale-Shapley algorithm marked a turning point, providing a concrete methodology for addressing the stable marriage problem. This breakthrough laid the groundwork for further developments in both theory and application.
The stable marriage problem and the Gale-Shapley algorithm are pivotal in understanding the basic principles and challenges of Matching Theory.
Recent advancements have broadened the scope of Matching Theory, incorporating complex models like networked markets and multi-agent systems. These developments have introduced new dimensions to the field, allowing for the exploration of more intricate and nuanced matching scenarios.Notably, the integration of Matching Theory with other areas such as machine learning and data analytics has unlocked innovative approaches to tackle allocation problems, ensuring that the evolution of Matching Theory is inextricably linked with technological progress.
Current Challenges in the Field of Matching Theory
Despite significant advancements, the field of Matching Theory faces several challenges that stem from the growing complexity of its applications and the dynamic nature of real-world systems. One of the foremost challenges is designing algorithms that can efficiently process large datasets without compromising on the quality of the match.Addressing issues of fairness and equity in matching, particularly in sensitive areas such as organ donation and school admissions, presents another layer of complexity. These problems require balancing mathematical optimality with ethical considerations, a task that is as challenging as it is crucial.
A noteworthy challenge is adapting Matching Theory to the ever-changing landscape of the digital economy, where platform-based markets (e.g., ride-sharing apps, online freelance job platforms) introduce unique constraints and preferences. The dynamic nature of these platforms, coupled with the diverse and sometimes conflicting interests of users, demands sophisticated matching algorithms that can continually adapt to real-time changes and user feedback.Moreover, the integration of privacy-preserving techniques into matching algorithms — to protect sensitive personal data in applications like online dating or job matching — is becoming increasingly important. This integration necessitates a delicate balance between the integrity of the matching process and the privacy concerns of participants.The resolution of these challenges will likely require interdisciplinary approaches, drawing from fields such as economics, computer science, and ethics, to develop matching algorithms that are not only efficient and practical but also fair and respectful of individual privacy.
In summary, the future of Matching Theory hinges on addressing these challenges through innovative research and cross-disciplinary collaboration. As the field continues to evolve, it remains an area ripe for exploration and discovery.The developments to come will undoubtedly push the boundaries of what algorithms can achieve, further cementing the role of Matching Theory in solving some of the most complex and impactful problems faced by society today.
Matching Theory - Key takeaways
- Matching Theory Definition: A branch of combinatorial mathematics focusing on creating pairs from two sets based on specific criteria, applicable in economics, resource allocation, etc.
- Stability and Optimality: Core principles of Matching Theory emphasising stable and 'best possible' pairings considering preferences and resources.
- Gale-Shapley Algorithm: A deferred acceptance algorithm which ensures stable matches between two equally sized sets by handling proposals and rejections based on ranked preferences.
- Perfect Matching in Graph Theory: A state in which each vertex of one part of a bipartite graph is exclusively paired with one vertex of the other part, with no vertices left unmatched.
- Application Examples: Matching Theory is widespread in healthcare for organ allocations, in education for student school placements, and in labour markets for job matchmaking.
Learn faster with the 0 flashcards about Matching Theory
Sign up for free to gain access to all our flashcards.
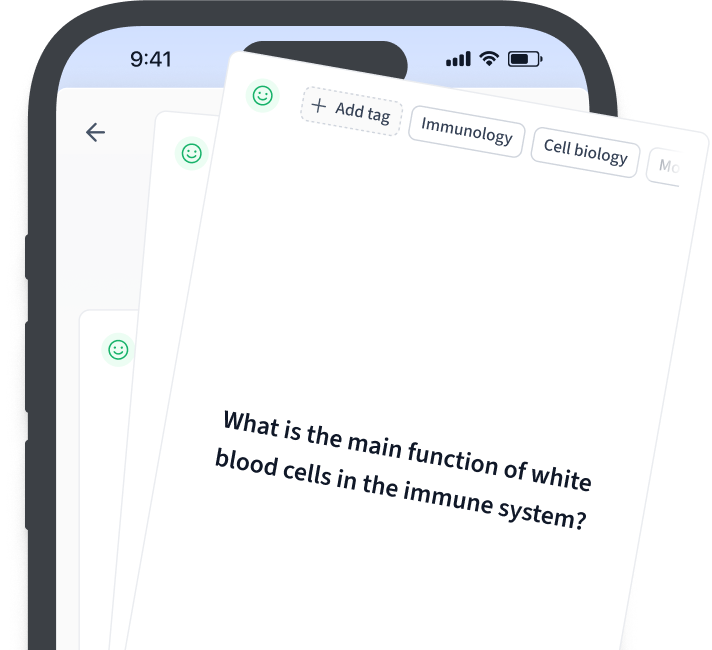
Frequently Asked Questions about Matching Theory
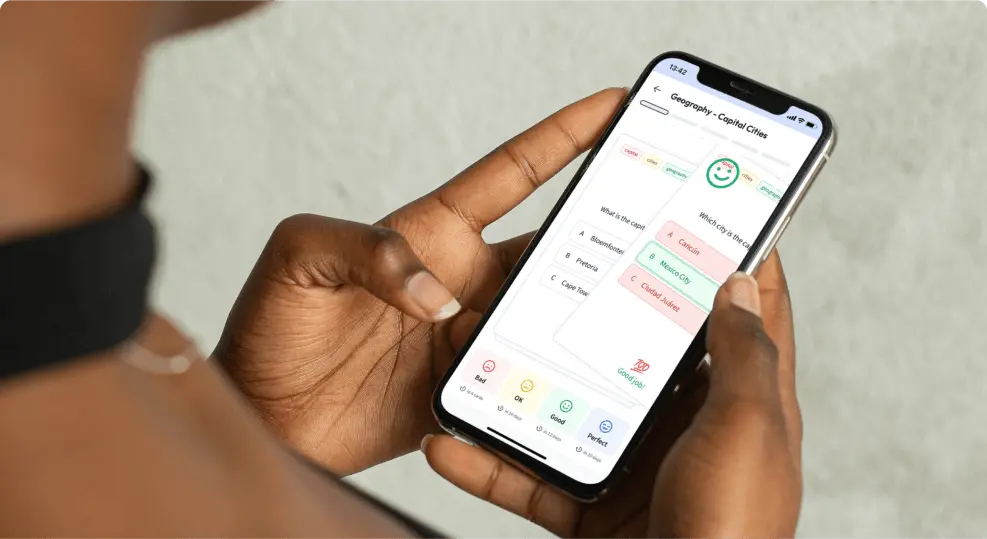
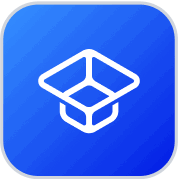
About StudySmarter
StudySmarter is a globally recognized educational technology company, offering a holistic learning platform designed for students of all ages and educational levels. Our platform provides learning support for a wide range of subjects, including STEM, Social Sciences, and Languages and also helps students to successfully master various tests and exams worldwide, such as GCSE, A Level, SAT, ACT, Abitur, and more. We offer an extensive library of learning materials, including interactive flashcards, comprehensive textbook solutions, and detailed explanations. The cutting-edge technology and tools we provide help students create their own learning materials. StudySmarter’s content is not only expert-verified but also regularly updated to ensure accuracy and relevance.
Learn more