Jump to a key chapter
Understanding the Hazard Function
Exploring the Hazard Function enlightens us on evaluating the risk or chance of an event happening over a period. This mathematical concept finds extensive use in various fields, including statistics, engineering, and reliability analysis. Let's delve into its definition, explanation, and key components to grasp its importance in predictive models and risk assessment.
What is the Hazard Function Definition?
The Hazard Function, often denoted as h(t), quantifies the instant rate at which events occur, given no prior occurrence up to time t. It’s a conditional measure focusing on the likelihood of an event within a small time frame, conditional on the event not happening until that point.
An Overview of the Hazard Function Explanation
The Hazard Function is a fundamental tool in survival analysis and reliability engineering, offering insights into time-to-event data analysis. Unlike probability density functions that provide the probabilities of outcomes over an interval, the Hazard Function helps in modelling the rate of change of probability over time. This shift from measuring probabilities to measuring rates of change provides a dynamic view of risk and event occurrence over time.
If you’re familiar with survival curves, think of the Hazard Function as describing the slope of those curves at any point in time.
Key Components of the Hazard Function
To fully understand the Hazard Function, it’s crucial to be aware of its key components. Let’s break them down:
- Baseline Hazard: Represents the risk of event occurrence when all other variables are set to their reference levels.
- Covariates: These are variables that can affect the hazard rate. In statistical models, these are often demographic factors like age or technical specifications in reliability models.
- Survival Function: This function complements the Hazard Function. While the Survival Function quantifies the probability of surviving past a certain time, the Hazard Function focuses on the risk of failure or event occurrence during a given interval.
Consider a study on the reliability of car engines. The Hazard Function can help in identifying at what point in time the risk of an engine failure increases significantly. If a particular model shows a steep increase in the hazard rate after 100,000 miles, proactive maintenance or checks around this mileage could mitigate potential failures.
A deeper dive into the mathematics behind the Hazard Function reveals its direct relationship with the cumulative distribution function (CDF) and the probability density function (PDF) of time-to-event data. The formula for the Hazard Function is as follows:
\[h(t) = rac{f(t)}{S(t)}igg|_{S(t) \neq 0} ight] ight]
Where f(t) is the PDF and S(t) is the Survival Function. This relationship illustrates how the Hazard Function uses information from both PDF and CDF to provide a unique perspective on risk assessment over time.
Applying the Hazard Function in Probability and Statistics
Investigating the Hazard Function offers profound insights into the realms of probability and statistics, especially when assessing the timing and likelihood of certain events. This exploration is not only academically enriching but also practically applicable across diverse scenarios.
Hazard Function Example in Real-Life Scenarios
The Hazard Function illustrates its utility in a variety of real-life scenarios, making abstract statistical concepts tangible. From healthcare to engineering, the applications are wide and varied.
For instance, in medical research, the Hazard Function is pivotal in analysing patient survival times after receiving a treatment. Researchers might want to understand how quickly, if at all, a new drug reduces the risk of illness relapse. By examining the hazard rate, they can identify periods of increased risk and potentially adjust treatment protocols accordingly.
Analysing hazard rates in clinical trials can inform both the effectiveness and potential risks of new treatments.
How to Calculate the Hazard Rate Function
Calculating the Hazard Rate Function involves understanding and applying a specific formula. This process is critical for deriving meaningful insights from data, particularly in survival analysis.
To calculate the Hazard Rate Function, the formula is:
\[h(t) = \frac{f(t)}{S(t)}\]
where h(t) is the hazard function, f(t) is the probability density function, and S(t) is the survival function. This equation essentially divides the probability of an event occurring at time t, by the probability of surviving (i.e., not experiencing the event) up to time t.
Consider a scenario where a study is conducted to measure the failure rate of a specific car part. If the probability of the car part failing at time t is calculated and the survival probability until that time is known, the hazard rate function can offer exact insights into the risk at that specific time.
The Importance of the Cumulative Hazard Function
The Cumulative Hazard Function takes the analysis a step further by summing up the hazard rates over time, giving stakeholders a holistic view of risk across the timeframe of interest.
The Cumulative Hazard Function, denoted as H(t), is defined as the integral of the hazard function over time:
\[H(t) = \int_{0}^{t} h(s) ds\]
This function accumulates the risk of the event happening over time, providing a comprehensive view of the potential for occurrence.
Imagine monitoring a patient's response over time to a long-term treatment plan. The cumulative hazard function can offer insights into whether the patient's risk of adverse reactions is increasing, staying the same, or decreasing. This information is critical in deciding whether to continue, stop, or modify the treatment.
Diving deeper, the Cumulative Hazard Function is instrumental in non-parametric models, such as the Nelson-Aalen estimator. This estimator is particularly advantageous in scenarios where the data is censored. Censored data occurs frequently in survival analysis, where the outcome (e.g., death, failure) might not be observed within the study period for all subjects. The Nelson-Aalen estimator provides a way to estimate cumulative hazard rates despite these challenges.
Variations of the Hazard Function
In the study of predictive models, understanding the variations of the Hazard Function proves crucial. These variations, including the baseline hazard function and the cumulative hazard function, offer distinct perspectives on event occurrence over time. Let's delve into these to enhance comprehension.
Exploring the Baseline Hazard Function
The Baseline Hazard Function plays a pivotal role in survival analysis, serving as a reference point from which variations due to specific factors can be measured. This section explores this crucial component.
The Baseline Hazard Function, denoted as h_0(t), represents the hazard rate when all covariates in a model are set to zero. It shows the default risk of an event occurring at any time t, independent of any influencing factors.
In a clinical trial studying the effect of a new medication on extending survival time after surgery, the baseline hazard function could represent the survival rate without considering the drug's impact. Here, any deviations from this baseline could be attributed to the medication's effectiveness.
Think of the baseline hazard function as a starting point that allows researchers to isolate and examine the effects of specific variables on the hazard rate.
Differences between Hazard Function and Cumulative Hazard Function
While the Hazard Function and Cumulative Hazard Function are closely related, distinguishing between them is vital for accurate analysis. This segment highlights their differences.
The Hazard Function, h(t), focuses on the instantaneous risk of an event at any given time. In contrast, the Cumulative Hazard Function compiles the risk over a time period, providing a comprehensive risk assessment.
The Cumulative Hazard Function, represented as H(t), is the integral of the hazard function over time t, given by:
\[H(t) = \int_0^t h(s) ds\]
This function accumulates the risk of the event happening across a specified timeframe, offering a broader perspective on risk dynamics.
Suppose a study aims to evaluate the risk of developing a particular disease among different age groups over a decade. While the hazard function could reveal the immediate risk at each year of age, the cumulative hazard function would illustrate the total risk accumulated by each age group over the decade.
One fundamental difference lies in the interpretation and use of these functions. The hazard function is crucial for understanding immediate risks and their variations over time. On the other hand, the cumulative hazard function is instrumental in evaluating the overall impact of risk over a longer period, often used in creating survival curves and in methods such as the Nelson-Aalen estimator for handling censored data.
Practical Applications of the Hazard Function
The Hazard Function is a critical tool in the fields of survival analysis, risk assessment, and statistical decision making. By quantifying how the risk of an event changes over time, this function can help predict future occurrences and inform strategic decisions in various sectors such as healthcare, engineering, and finance.
Utilising the Hazard Function in Survival Analysis
In survival analysis, the Hazard Function plays a pivotal role in understanding the time until an event of interest, such as death or failure, occurs. It is particularly useful in healthcare for assessing patient outcomes following treatments or in engineering for predicting when a machine part might fail.
Survival analysis typically involves datasets where the time to event is known for some subjects, but not for others (censored data). Here, the Hazard Function helps in dealing with such complexities by providing a rate at which the event occurs, conditional on survival until that time.
An example involves studying the effect of a new cancer treatment. The Hazard Function would allow researchers to determine the treatment's efficacy over time, highlighting moments when risk of relapse diminishes or increases. This is crucial for understanding long-term patient survival and tailoring follow-up care.
Remember, in survival analysis, the lower the hazard rate, the better the survival prospects.
Hazard Function in Risk Assessment
Risk assessment is another vital application area of the Hazard Function. Industries such as insurance, finance, and public health use it to evaluate the likelihood of an event’s occurrence and its potential impact.
By determining the rate at which specific risks evolve over time, organisations can allocate resources more efficiently, develop risk mitigation strategies, and improve safety protocols.
In public health, the Hazard Function can help predict disease spread within a community. It quantifies not just the risk of infection over time, but how changing conditions—like the introduction of vaccines—alter that risk. This information is critical for public health planning and response strategies.
In risk assessment, a sudden increase in the hazard rate can signal a need for immediate action to prevent potential disasters.
Impact of the Hazard Function on Decision Making in Statistics
The Hazard Function significantly influences decision making in statistics by providing a temporal dimension to risk assessment. It allows for dynamic analysis of risk, which can support more informed, evidence-based decisions.
Furthermore, the Hazard Function’s ability to isolate the impact of different variables over time enhances its utility in developing targeted intervention strategies and in predictive modelling.
- Identifying critical time periods for intervention
- Assessing the effectiveness of policy changes
- Optimising resource allocation based on risk levels over time
For instance, in statistical models used for predicting customer churn in telecoms, the Hazard Function can identify periods when a customer is more likely to leave. This enables companies to proactively engage with these customers, perhaps offering promotions or addressing service issues, thereby potentially reducing churn rates. The use of the Hazard Function here illustrates how time-dependent risk assessments can directly influence strategic business decisions, demonstrating its wide-ranging implications beyond traditional fields.
Hazard function - Key takeaways
- Hazard Function Definition: Quantifies the instant rate at which events occur at a specific time t, conditional on no previous occurrence.
- Hazard Function Explanation: Unlike probability density functions, the hazard function models the rate of change of probability over time, offering a dynamic view of risk and event occurrence.
- Baseline Hazard Function: Represents the hazard rate when all covariates in a model are at their reference levels, serving as a starting point to measure the effect of specific variables.
- Cumulative Hazard Function: The integral of the hazard function over time, it accumulates the risk of an event occurring across a specified timeframe and is used for comprehensive risk assessment.
- Hazard Rate Function Calculation: The hazard function h(t) is calculated as the probability density function f(t) divided by the survival function S(t), representing the risk of event occurrence at time t.
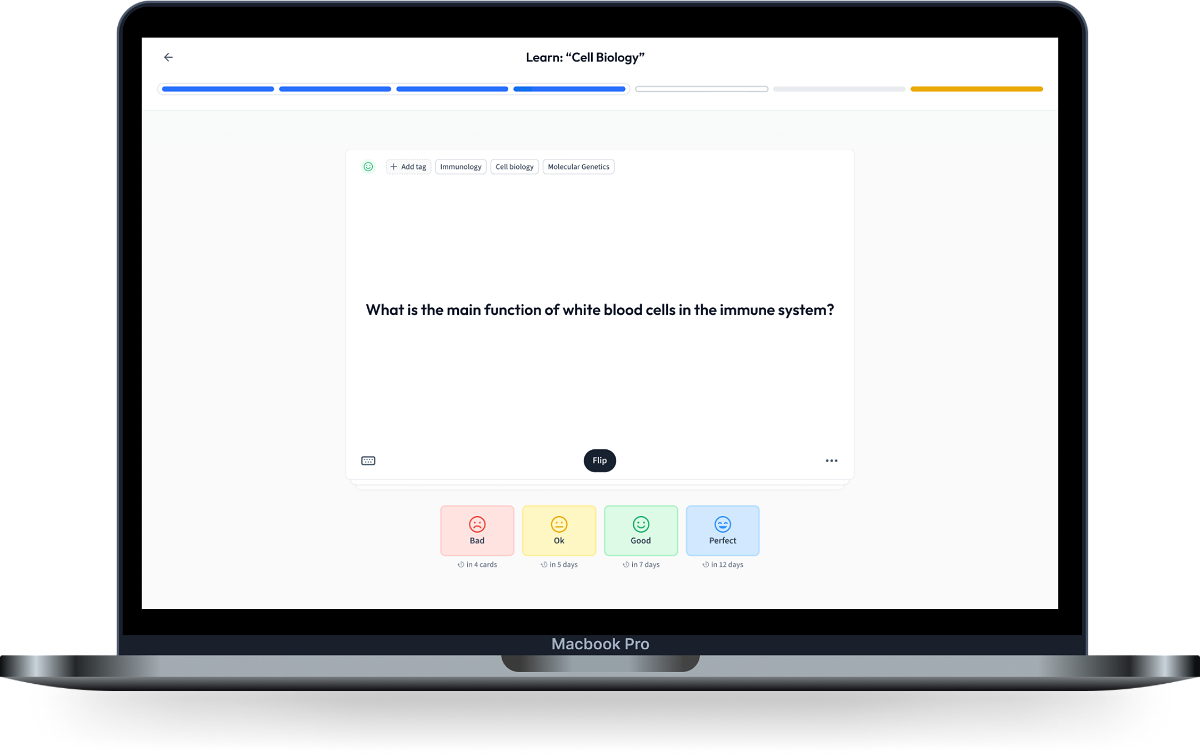
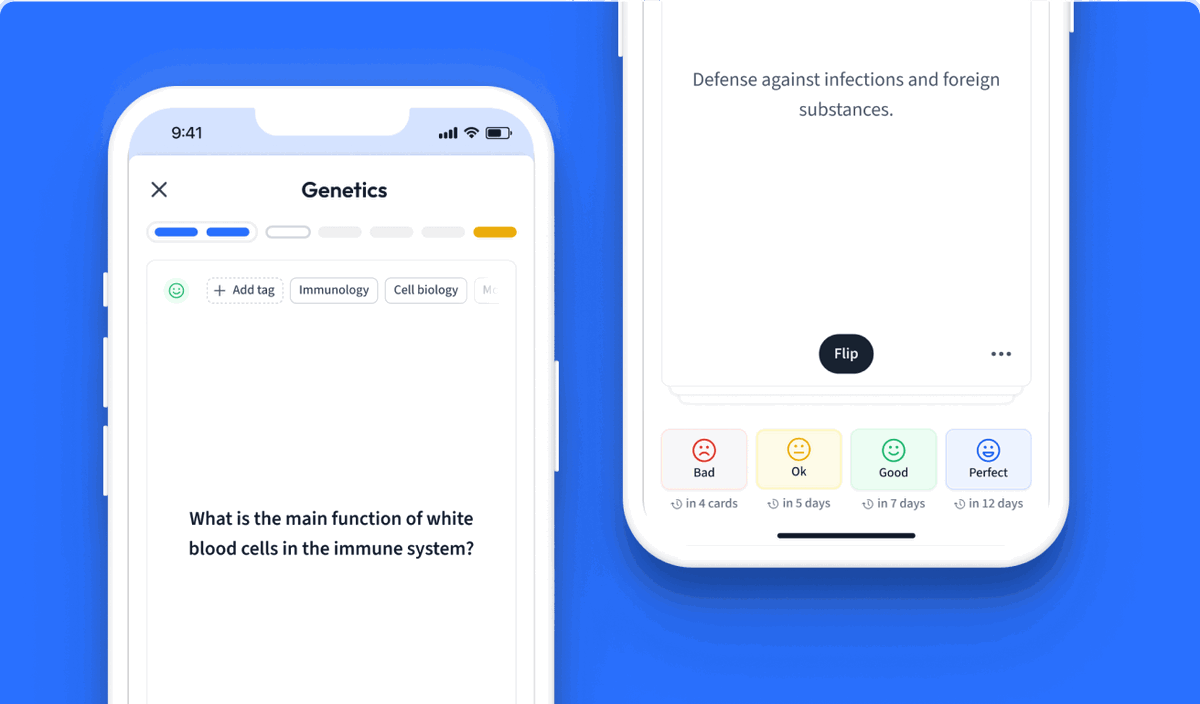
Learn with 0 Hazard function flashcards in the free StudySmarter app
Already have an account? Log in
Frequently Asked Questions about Hazard function
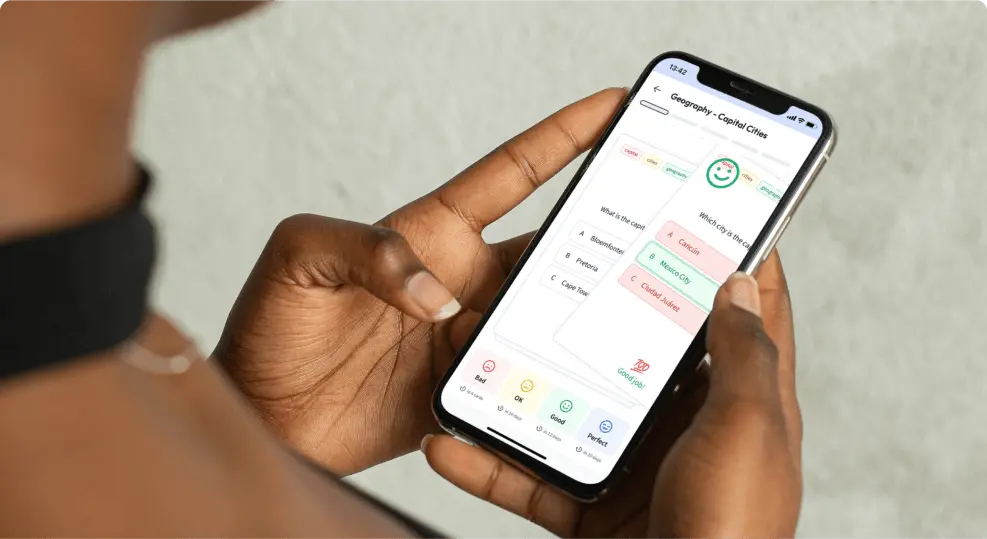
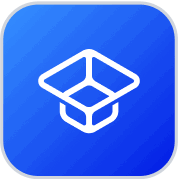
About StudySmarter
StudySmarter is a globally recognized educational technology company, offering a holistic learning platform designed for students of all ages and educational levels. Our platform provides learning support for a wide range of subjects, including STEM, Social Sciences, and Languages and also helps students to successfully master various tests and exams worldwide, such as GCSE, A Level, SAT, ACT, Abitur, and more. We offer an extensive library of learning materials, including interactive flashcards, comprehensive textbook solutions, and detailed explanations. The cutting-edge technology and tools we provide help students create their own learning materials. StudySmarter’s content is not only expert-verified but also regularly updated to ensure accuracy and relevance.
Learn more