Jump to a key chapter
What Is Queuing Theory?
Queuing Theory explores the dynamics of queues to predict queue lengths and waiting times. This branch of mathematics is crucial for designing and managing systems that involve any form of waiting lines, ensuring efficiency and customer satisfaction.
Understanding the Queuing Theory Definition
Queuing Theory is a mathematical study of queues or waiting lines. It involves the analysis of various queue models and their applications to predict and improve queue performance metrics such as queue length, waiting time, and system utilisation.
In queuing theory, a basic model consists of three fundamental components: arrivals, queue, and service mechanism. The goal is to optimise these components to enhance efficiency and reduce costs. Key parameters like arrival rate (\( ext{λ} \)) and service rate (\( ext{μ} \)) are analysed to achieve this.
The arrival rate and service rate are critical in determining the performance of a queueing system.
Example: Consider a small coffee shop where the arrival rate of customers is 10 per hour (\( ext{λ} = 10 ext{/hour} \)), and each barista can serve 12 customers per hour (\( ext{μ} = 12 ext{/hour} \)). Using queuing theory, the shop can predict waiting times and adjust staffing to improve customer service.
An interesting aspect of queuing theory is its assumption models. For instance, the Poisson distribution often models the arrival of entities, while service times might follow an Exponential distribution. These assumptions help in simplifying and solving complex queue models to obtain practical solutions.
The Real-World Applications of Queuing Theory
Queuing theory finds its application across various sectors. From telecommunications to healthcare, understanding queues helps optimise resource allocation and customer experience. Below are some sectors where queuing theory is extensively applied:
- Telecommunications: To manage network traffic and reduce congestion.
- Banking: For optimising teller numbers and reducing wait times.
- Healthcare: To decrease patient wait times and improve service delivery.
- Manufacturing: In scheduling production lines to minimise downtime.
- Retail: To improve checkout processes and enhance customer satisfaction.
- Airport security: For managing queue lengths and reducing waiting times for security checks.
The versatility of queuing theory allows its principles to be applied in both service and manufacturing sectors.
Example: An airport planning to redesign its security checkpoint can use queuing theory to determine the optimal number of open lanes and staff allocation to minimise passenger wait times, even during peak travel periods.
The Math Behind Queuing Theory
Queuing theory offers a quantitative framework for analysing and predicting the behaviour of queueing systems. This mathematical approach enables the design of more efficient systems, whether you are managing a busy restaurant or optimising network traffic.
Breaking Down the Formula for Queuing Theory
The fundamental queuing formula, often at the heart of queuing theory, is based on the relationship between the arrival rate of entities to a system (\( ext{λ} ext{/hour} \) ), and the service rate at which these entities can be processed (\( ext{μ} ext{/hour} \) ).
The stability of any queueing system hinges on the balance between these rates. Ideally, the service rate should exceed the arrival rate to prevent indefinite queue growth. This balance is encapsulated in the formula: \[ ext{Stability: } ext{μ} > ext{λ} \].
Key Queuing Theory Equations Explained
Several key equations are central to queuing theory, each serving a different purpose in the analysis of queue dynamics:
- Little’s Law (\( L = ext{λ}W \) ): Connects the average number of items in a system (\( L \) ), the arrival rate (\( ext{λ} \) ), and the average time an item spends in the system (\( W \) ).
- The Traffic Intensity (\( ho = rac{ ext{λ}}{ ext{μ}} \) ): Offers a measure of how busy a system is, relative to its capacity to serve.
- Probability of Queue Length and Waiting Times: These equations predict the likelihood of certain queue lengths and waiting times based on the arrival and service rates.
Example: In a healthcare setting, if a clinic receives 60 patients per hour (\( ext{λ} = 60 \) ), and each doctor can treat 10 patients per hour (\( ext{μ} = 10 \) ), the traffic intensity (\( ho \) ) can be calculated as \( rac{60}{10} = 6 \), indicating the system is highly overburdened.
Probability and Queuing Theory: The Connection
Probability plays a key role in queuing theory, providing the foundation for predicting queue lengths and waiting times. It helps in modeling the random arrival of entities and variability in their service times.
A fascinating aspect of probability in queuing theory is the use of probability distributions, such as the Poisson distribution for arrivals and the Exponential distribution for service times. These distributions are utilised because of their memoryless properties, making them suitable for modelling events that occur independently.
The management of queueing systems often involves balancing the probabilities of service times and arrivals to optimise for efficiency and satisfaction.
Exploring Different Queuing Theory Models
In the study of queuing theory, various models are used to represent and solve real-world queueing problems. These models, each with their unique assumptions and characteristics, offer insight into how queues function and how best to manage them. By understanding these models, you can apply the most appropriate strategies to minimise waiting times and enhance system efficiency.A deep understanding of single-queue and multi-queue models, as well as how service and non-service systems are modelled in queuing theory, provides a solid foundation for anyone looking to optimise operations or study this interesting mathematical field.
A Closer Look at Single-Queue and Multi-Queue Models
Queuing theory models are broadly categorised into single-queue and multi-queue models, each with its specific context of application and analysis. Understanding the nuances between these models is essential for effectively managing queues in different scenarios.A single-queue system involves one line that feeds into one or many servers. This model is common in settings where ensuring fairness and first-come, first-served (FCFS) service is vital. In contrast, multi-queue systems consist of several lines, each leading to its server. This model finds application in scenarios where specialisation of service or parallel processing can significantly reduce waiting times.
Deep Dive Into Multi-Queue Models: Multi-queue models often incorporate sophisticated algorithms to manage queue dynamics, such as routing customers to the shortest line or balancing loads across servers. Retail checkouts, airport security screenings, and call centres frequently use multi-queue systems to optimise service efficiency. The challenge lies in the careful design and management of these systems to avoid common pitfalls such as unequal queue lengths and server idle times.
Example: In a grocery store using a multi-queue system, each cashier operates an individual queue. Advanced systems might employ software to direct customers to the shortest queue or open new cashier lanes as queue length exceeds a certain threshold, thus aiming to minimise the overall waiting time for customers.
Service and Non-Service Systems in Queuing Theory
While queuing theory traditionally focuses on service systems—where the primary concern is the efficient delivery of services to customers—it also extends to non-service systems, such as manufacturing and computer networks. The principles remain constant, but the application and impact can vary significantly between these contexts.In service systems (e.g., banks, hospitals), the aim is to reduce waiting times and improve customer satisfaction. In contrast, in non-service systems (e.g., manufacturing lines, network routers), the focus shifts towards maximising throughput and optimising resource allocation.
Example: In a manufacturing context (a non-service system), queuing theory might be used to determine the optimal number of machines in a series to maximise production throughput while minimising the time components spend waiting to be processed at each stage.
Queuing theory, through its diverse models, serves as a versatile tool applicable across different sectors, encompassing both service and non-service systems. Its adoption can significantly enhance operational efficiency and customer experience.
Little's Law in Queuing Theory
In the realm of queuing theory, Little's Law stands out for its simplicity and profound practical significance. This law offers a powerful tool for analysing a wide range of queuing systems, from customer service desks to sophisticated manufacturing processes. By providing a clear mathematical relationship between key queuing metrics, Little's Law enables efficient system management and optimisation.Understanding Little's Law is fundamental for anyone looking to delve into queuing theory or improve the efficiency of systems characterised by waiting lines.
The Essence of Little's Law in Simplifying Queuing Theory
Little's Law is elegantly simple, stating that the long-term average number of customers in a stable system (L) is equal to the long-term average effective arrival rate (\( ext{λ} \)), times the average time a customer spends in the system (W). Represented mathematically as: \[ L = ext{λ}W \].
This law applies under steady-state conditions without any assumptions about the statistical distribution of arrival or service processes. It offers a direct method to evaluate queuing systems, simplifying the analysis by focusing on these three interconnected variables. Little's Law essentially highlights the trade-off between wait times and system capacity, demonstrating that to achieve lower wait times, either decrease the arrival rate or increase the service capability.The application of Little's Law extends beyond theory, providing practical insights into the dynamics of real-world queuing systems.
Little's Law illustrates that in a stable system, to maintain service quality, you must balance the relationship between arrivals, their service duration, and total in-system numbers.
Applying Little's Law in Various Queuing Scenarios
The versatility of Little's Law allows for its application across a broad spectrum of queuing scenarios. From customer service centres and healthcare facilities to production lines and computer networks, understanding and applying Little's Law can significantly enhance operational efficiency and customer satisfaction.The following list provides a snapshot of where Little's Law can make a noticeable impact:
- Customer Service: Predicting wait times and adjusting staff levels to meet demand.
- Healthcare: Managing patient flow to reduce waiting times for treatment.
- Retail: Streamlining checkout processes to improve shopping experience.
- Manufacturing: Balancing production lines to minimise bottlenecks.
- IT and Networks: Optimising data flow to prevent congestion and improve system responsiveness.
Example: In a retail environment, if the arrival rate of customers at a checkout is 30 customers per hour (\( ext{λ} = 30 \)), and customers spend an average of 10 minutes in the system (\( W = 10 ext{ minutes} \)), Little's Law can be applied to predict that, on average, there will be 5 customers in the system at any time (\( L = 30 ext{ customers/hour} imes rac{10}{60} ext{ hour} = 5 ext{ customers} \)).
A deeper exploration of Little's Law reveals its application in complex system analysis, such as in queueing networks with interrelated nodes and processes. In these environments, Little's Law helps to identify system-wide bottlenecks, guiding the reallocation of resources or the redesign of processes to enhance overall efficiency.Moreover, its application is not just limited to physical systems. Little's Law is equally valuable in virtual environments, such as in the design of efficient software algorithms and architecture to manage digital queues and workflows, indicating its broad relevance across fields.
Queuing theory - Key takeaways
- Queuing Theory Definition: A branch of mathematics analysing queues or waiting lines to predict and improve performance metrics such as queue length, waiting time, and system utilisation.
- Basic Components: A queuing model includes arrivals, queue, and service mechanism, with the arrival rate (λ) and service rate (μ) as key parameters.
- Stability Formula: For a stable system, the service rate (μ) should exceed the arrival rate (λ), encapsulated in the formula μ > λ.
- Little's Law: A key equation in queuing theory stating that the average number of items in the system (L) equals the arrival rate (λ) times the average time an item spends in the system (W), expressed as L = λW.
- Real-World Applications: Queuing theory is applied in various sectors like telecommunications, banking, healthcare, manufacturing, retail, and airport security to optimise systems and improve efficiency.
Learn faster with the 0 flashcards about Queuing theory
Sign up for free to gain access to all our flashcards.
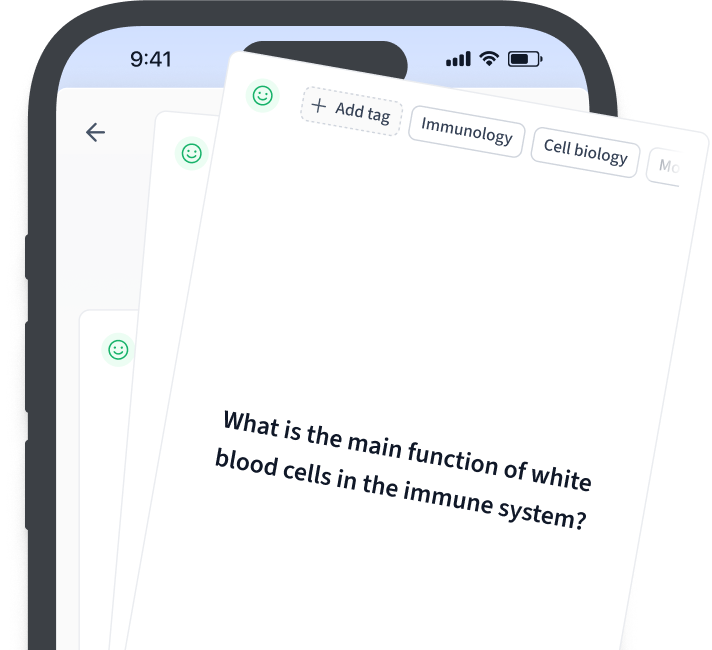
Frequently Asked Questions about Queuing theory
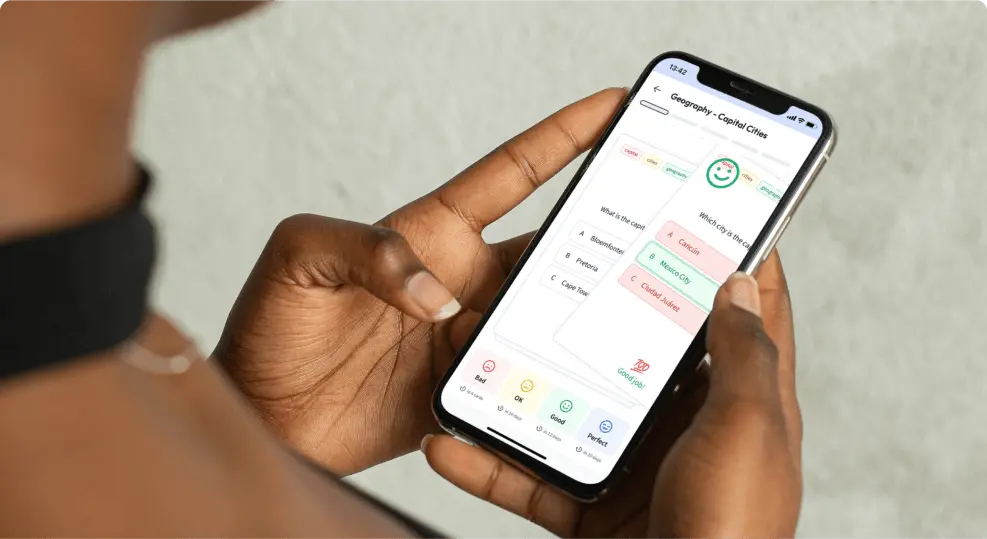
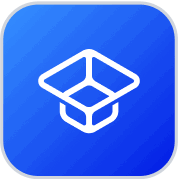
About StudySmarter
StudySmarter is a globally recognized educational technology company, offering a holistic learning platform designed for students of all ages and educational levels. Our platform provides learning support for a wide range of subjects, including STEM, Social Sciences, and Languages and also helps students to successfully master various tests and exams worldwide, such as GCSE, A Level, SAT, ACT, Abitur, and more. We offer an extensive library of learning materials, including interactive flashcards, comprehensive textbook solutions, and detailed explanations. The cutting-edge technology and tools we provide help students create their own learning materials. StudySmarter’s content is not only expert-verified but also regularly updated to ensure accuracy and relevance.
Learn more