Jump to a key chapter
What Is Uniform Distribution?
Uniform distribution represents an important concept in statistics and probability theory, illustrating a scenario where all outcomes are equally likely. This principle is vital across various fields, including computer science, economics, and physical sciences, making it a fundamental topic in mathematics. Understanding uniform distribution helps students grasp the basics of probability and how it applies to real-world situations.
Understanding the Basics of Uniform Distribution
At its core, uniform distribution exists when each member of a set has an equal chance of occurrence. It's like rolling a fair die; each number (1 through 6) has an equal probability of landing up. This concept is crucial for predicting outcomes in various situations, such as simple games or even complex simulations.There are two key formulas associated with uniform distribution that you should know:- For discrete uniform distribution: \(P(X = x) = \frac{1}{n}\), where n is the number of outcomes.- For continuous uniform distribution: The probability density function (pdf) is \(f(x) = \frac{1}{b-a}\) for a ≤ x ≤ b. This means that within the interval from a to b, the probability of any specific point is evenly distributed.
Uniform Distribution: A statistical distribution where all outcomes are equally likely. Encompasses both a discrete and a continuous form, depending on the nature of the random variable involved.
Example of Discrete Uniform Distribution: Consider the scenario of tossing an unbiased coin. There are two possible outcomes, heads or tails, each with an equal probability of \(\frac{1}{2}\).Example of Continuous Uniform Distribution: Imagine measuring the length of parts produced by a machine where the length can vary between 10.00 cm and 10.20 cm. Any specific measurement within this range is equally likely, illustrating continuous uniform distribution.
The uniform distribution, whether discrete or continuous, simplifies probability calculations by assuming all outcomes have the same likelihood.
Differentiating Between Continuous and Discrete Uniform Distribution
Discrete and continuous uniform distributions are two sides of the same coin, each tailored to different types of data. Discrete uniform distribution applies to scenarios with a finite set of outcomes, such as rolling dice or flipping coins. On the other hand, continuous uniform distribution deals with infinite possibilities, often within a certain range, like the aforementioned example of part lengths measured by a machine.Key differences:
- Discrete deals with countable outcomes, while continuous concerns infinitely many outcomes within a range.
- Discrete uses a simple probability formula, while continuous relies on a probability density function.
Continuous Uniform Distribution
In the world of probability and statistics, continuous uniform distribution plays a pivotal role in modelling scenarios where every outcome within a specific range is equally likely. This distribution is particularly useful in simulations, quality control, and various types of analysis across scientific disciplines.
Exploring Continuous Uniform Distribution
Continuous uniform distribution is essential for understanding how probabilities are assigned over a continuous interval. Unlike its discrete counterpart, which deals with distinct outcomes, continuous uniform distribution covers an infinite number of possibilities within a range, such as lengths, weights, or time intervals.This distribution is characterised by its flat probability density function (PDF), meaning the likelihood of observing any value within the defined interval is the same. Consequently, it's a powerful tool for modelling uniform probabilities in continuous spaces.
Uniform Distribution Formula for Continuous Variables
The probability density function (PDF) for continuous uniform distribution is defined for a random variable X that takes values within the interval \[a, b\]. The formula is given by:\[f(x) = \frac{1}{b-a}\] for \(a \leq x \leq b\).This formula is straightforward, indicating that the distribution's density is constant across the interval from a to b. Therefore, the probability of observing a value within any subinterval of \[a, b\] depends solely on the length of that subinterval, not on where the subinterval is located within \[a, b\].
Consider a scenario where a company decides to randomly select a time between 9:00 and 10:00 AM for a daily system reboot. The selection is uniformly distributed over the hour, meaning any second within this timeframe is equally likely to be chosen. Here, \(a = 0\) minutes and \(b = 60\) minutes. Using the formula for continuous uniform distribution, the density function is \[f(x) = \frac{1}{60-0} = \frac{1}{60}\].This means that the probability of the system reboot happening in the first 15 minutes of the hour (i.e., between 9:00 and 9:15 AM) is \[\frac{15}{60} = 0.25\], or 25%.
Variance of Continuous Uniform Distribution
Understanding the variance of a continuous uniform distribution is crucial for assessing the spread of the data within the interval. The formula for calculating the variance (\( ext{Var}(X)\)) of a continuous uniform distribution defined over the interval \[a, b\] is:\[\text{Var}(X) = \frac{(b-a)^2}{12}\].This equation reveals how the span of the interval \[a, b\] influences the variability of the distribution. A wider interval means greater variance, indicating a broader spread of potential values within the range. Knowing the variance helps in risk assessments, quality control, and prediction accuracy in various applications.
The derivation of the variance formula for continuous uniform distribution starts with understanding its moment about the mean. The second moment about the mean for any continuous distribution is integral to deriving its variance. Given the uniform nature of the distribution and the linear behaviour of integration, the formula simplifies elegantly to \[\frac{(b-a)^2}{12}\]. This balance between simplicity and mathematical profundity highlights the elegance inherent in statistical theory and its application to understanding real-world phenomena.The implications of this formula extend beyond academia. For instance, in financial risk management, understanding the variance of returns on investments with uniform outcomes within specified ranges can offer insights into expected volatility. Similarly, in engineering, calculating the variance of uniformly distributed measurements can enhance quality control protocols.
The uniform distribution, by offering constant probability across an interval, simplifies the conceptualisation of randomness in continuous spaces.
Discrete Uniform Distribution
Discrete uniform distribution is a cornerstone concept in statistics and probability theory that applies when all outcomes of a discrete random variable are equally likely. This distribution is fundamental in understanding how randomness operates within a finite set of possibilities, such as rolling a fair die or drawing cards from a deck where each outcome has an identical chance of occurring.
Introduction to Discrete Uniform Distribution
The discrete uniform distribution is defined for scenarios where there are a finite number of outcomes that are equally probable. It's one of the simplest probability distributions available, serving as a model for idealised situations where each event in a sample space occurs with the same frequency. This distribution is not only fundamental in theoretical studies but also has practical applications in various fields, including computer science, game theory, and operations research.Recognising discrete uniform distribution situations helps in the accurate calculation of probabilities, making it a crucial concept in introductory statistics and probability courses.
Uniform Distribution Formula for Discrete Variables
The probability formula for a discrete uniform distribution where each of the n outcomes is equally likely is given by:\[P(X = x) = \frac{1}{n}\]Here, n represents the total number of outcomes, and \(P(X = x)\) denotes the probability of any specific outcome x. This formula is integral to calculating the likelihood of any single event occurring within a set of equally likely events.For example, in a fair dice roll with six possible outcomes, the probability of rolling any particular number is \(\frac{1}{6}\). This formula is straightforward yet powerful, providing a clear understanding of how to assess probabilities in discrete uniform settings.
Consider a fair six-sided die. This is a classic example of a discrete uniform distribution as each of the six faces—numbered 1 through 6—has an equal chance of landing up. Using our formula, the probability of rolling a 4, or any other specific number, is:\[P(X = 4) = \frac{1}{6}\].Similarly, if you were to randomly select a card from a standard deck of 52 playing cards, the probability of picking any specific card would be \(\frac{1}{52}\), assuming each card has an equal chance of being selected.
Calculating the Variance of Discrete Uniform Distribution
Variance measures the spread of the outcomes around the mean in a distribution. For a discrete uniform distribution, the variance provides insight into how the spread of all possible outcomes relates to their average value. The formula to calculate the variance (\( ext{Var}(X)\)) of a discrete uniform distribution with n equally likely outcomes is:\[ ext{Var}(X) = \frac{n^2 - 1}{12}\]This equation reflects the fact that as the number of outcomes increases, the variance grows, indicating a wider spread of possible outcomes around the mean. Understanding this relationship is key in statistical analysis to assess the predictability and reliability of the outcomes.
The derivation of the variance formula for discrete uniform distribution involves mathematical concepts such as the computation of the mean and the second moment about the mean. The formula \( ext{Var}(X) = \frac{n^2 - 1}{12}\) elegantly summarises the essence of variability within a discrete uniform set of outcomes. It highlights a fascinating aspect of uniform distributions: despite all outcomes being equally likely, the distribution of their deviations from the mean still follows a predictable pattern.This aspect of the variance calculation underscores the deeper principles underpinning probability distributions and their significance in understanding randomness and predictability in statistical data. The discrete uniform distribution, with its simplicity, serves as a gateway to these fundamental concepts in statistics.
The simplicity of the discrete uniform distribution's variance formula underscores the predictability within randomness, offering insights into how data might spread around a mean value.
Uniform Distribution Examples
Examples of Continuous Uniform Distribution in Real Life
Continuous uniform distribution finds its application in a myriad of real-life situations where the outcomes within a certain range are equally likely. Understanding these examples helps in grasifying the concept's practicality beyond theoretical aspects.One common example is the distribution of birth times throughout a day. Assuming each moment in a day is equally likely for a baby to be born, the time of birth from 00:00 to 23:59 follows a continuous uniform distribution. Likewise, measurements of manufactured items that are supposed to adhere to a specific length or size, such as metal rods cut to be two metres long with a permissible error margin, would also demonstrate this distribution, given the actual length can be any value within the defined range.
Consider the process of filling bottles with water, where the target volume is 500ml, but due to machine precision, the actual volume might range between 495ml and 505ml. Assuming the filling process is uniform and controlled:\[f(x) = \frac{1}{b-a}\]for \(a = 495\), \(b = 505\), and \(f(x)\) represents the probability density function. This scenario vividly illustrates how each outcome within the specified range (495ml to 505ml) is equally probable, embodying the essence of continuous uniform distribution.
Continuous uniform distribution is often used in simulations to model equally likely outcomes over an interval, making theoretical approximations more tangible in real-world scenarios.
Examples of Discrete Uniform Distribution in Everyday Scenarios
Discrete uniform distribution is equally prevalent in everyday life, particularly in scenarios where there are a finite number of outcomes that are all equally likely. This concept underpins many common experiences, from simple games to choosing random items from a set without bias.Classic examples include rolling a fair dice where each of the six sides has an equal chance of facing up, or drawing a card from a well-shuffled standard deck, where any of the 52 cards is equally likely to be picked. Such examples demonstrate the foundation of discrete uniform distribution in making predictions based on equal likelihoods of discrete outcomes.
Drawing a Student's Name from a Hat: Imagine a teacher decides to select a student at random for a classroom activity. If there are 25 students in the class, and each student's name is written on an identical piece of paper placed in a hat, the chance of any particular student's name being drawn is:\[P(X = x) = \frac{1}{n}\]where \(n = 25\). This setup perfectly showcases a discrete uniform distribution at play, with each outcome (i.e., each student's name) having an equal probability of \(\frac{1}{25}\).
The concept of discrete uniform distribution extends beyond mere theoretical or classroom examples into complex decision-making processes and simulations. For instance, in cybersecurity, generating random numbers that follow a discrete uniform distribution is crucial for encryption algorithms to ensure every possible key choice is equally likely, enhancing the robustness of cryptographic systems. This application underscores the importance of achieving genuine randomness, foundational to secure communications and data protection.Thus, while examples like drawing names from a hat or rolling dice are straightforward, they lay the groundwork for understanding the impact of uniformly distributed discrete outcomes in high-stakes fields such as information security and cryptography.
Uniform distribution - Key takeaways
- Uniform distribution is a statistical principle where all outcomes are equally likely, applicable to both discrete and continuous random variables.
- Discrete uniform distribution formula is P(X = x) = 1/n, whereas continuous uniform distribution uses a probability density function f(x) = 1/(b-a).
- Continuous uniform distribution is useful in modelling scenarios with infinite possible outcomes within a range, such as time intervals or measurements.
- The variance of a continuous uniform distribution over an interval [a, b] is calculated using the formula Var(X) = (b-a)^2/12.
- Discrete uniform distribution applies to scenarios with a finite set of outcomes, characterised by the variance formula Var(X) = (n^2 - 1)/12.
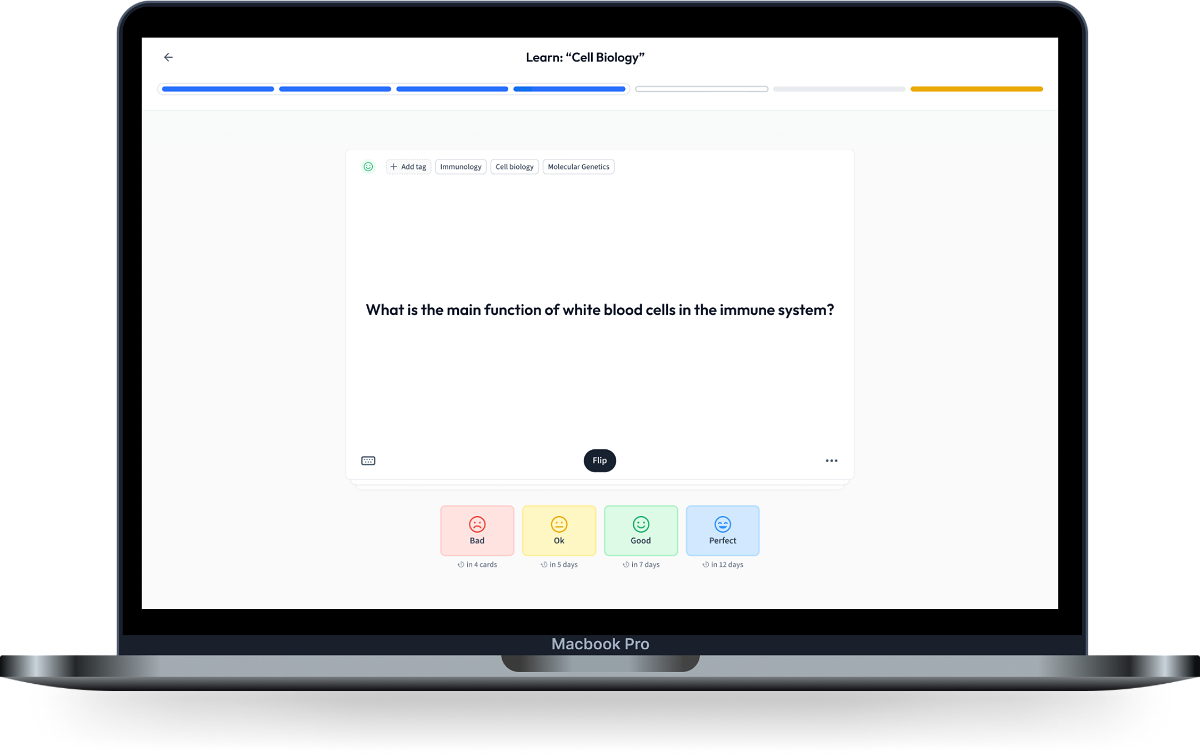
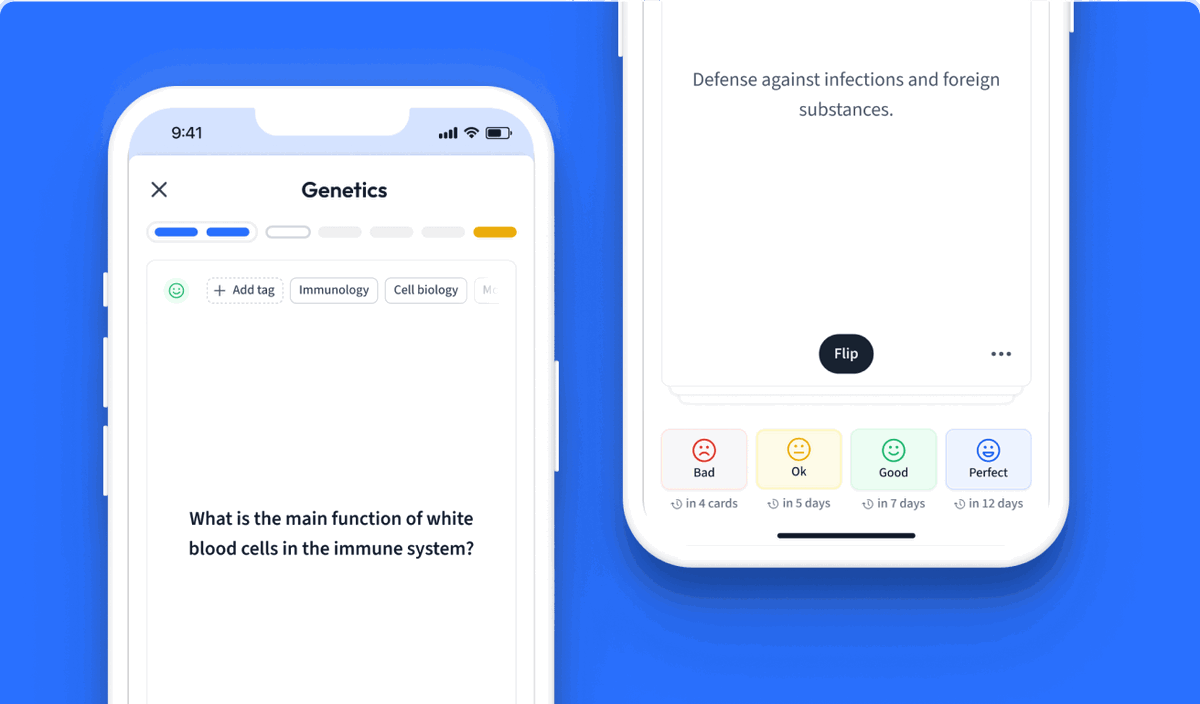
Learn with 0 Uniform distribution flashcards in the free StudySmarter app
Already have an account? Log in
Frequently Asked Questions about Uniform distribution
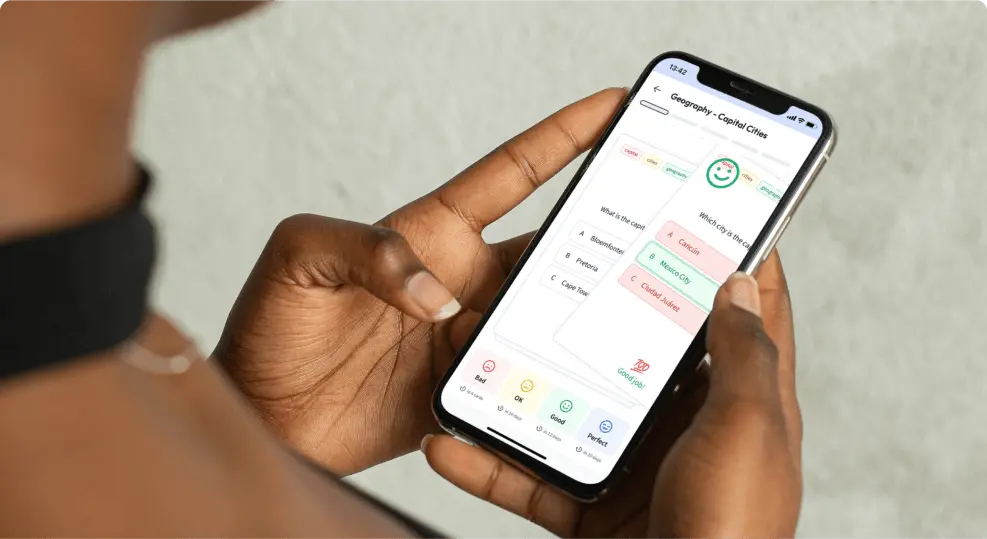
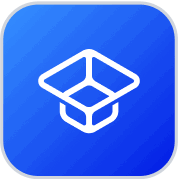
About StudySmarter
StudySmarter is a globally recognized educational technology company, offering a holistic learning platform designed for students of all ages and educational levels. Our platform provides learning support for a wide range of subjects, including STEM, Social Sciences, and Languages and also helps students to successfully master various tests and exams worldwide, such as GCSE, A Level, SAT, ACT, Abitur, and more. We offer an extensive library of learning materials, including interactive flashcards, comprehensive textbook solutions, and detailed explanations. The cutting-edge technology and tools we provide help students create their own learning materials. StudySmarter’s content is not only expert-verified but also regularly updated to ensure accuracy and relevance.
Learn more