Jump to a key chapter
What Is Biostatistics?
Biostatistics plays a pivotal role in the world of health sciences, combining the fields of biology and statistics to analyse and interpret data that can lead to breakthroughs in medical research and healthcare. This integration of disciplines enables professionals to address pressing health-related issues, making biostatistics a cornerstone in the advancement of medical knowledge and public health practices.
Defining Biostatistics: Understanding Its Role and Importance
Biostatistics is a branch of statistics that applies mathematical and statistical techniques to biological data to help solve problems in public health, medicine, and biology. It encompasses the design of biological experiments, the collection and analysis of data from those experiments, and the interpretation of the results.
Biostatistics: A field of science that applies quantitative methods to answer questions, test hypotheses, and make predictions in biology and health sciences.
Biostatistics is essential for the development of public health guidelines and for making informed medical decisions.
Consider the COVID-19 pandemic; biostatisticians played a crucial role in modelling the spread of the virus, evaluating the effectiveness of treatments, and analysing the impact of public health interventions. Their work helped in making data-driven decisions to control the pandemic.
Real-World Applications of Biostatistics in Medical and Health Sciences
The applications of biostatistics in the medical and health sciences are vast and varied. They range from designing clinical trials to understand the efficacy of new drugs, to epidemiological studies that seek to understand the patterns, causes, and effects of health and disease conditions in defined populations.
Example: In clinical trials, biostatistics is used to ascertain if a new drug is more effective than existing treatments. This involves the creation of control groups, the random assignment of subjects, and the use of statistical methods to analyse the outcome.
Moreover, biostatistics is integral in genomics, where it helps in associating genetic markers with specific diseases, and in public health, guiding the formulation of strategies to manage chronic diseases like diabetes and cancer. The following are key areas where biostatistics is applied in medical and health sciences:
- Design and analysis of clinical trials
- Epidemiological research
- Genetic and genomic studies
- Health services research
- Survival analysis and predictions
The use of biostatistics in health policy development enables authorities to make evidence-based decisions that can significantly improve healthcare outcomes.
Biostatistics Definition and Basics
Biostatistics offers an invaluable lens through which biological data are analysed, providing insights crucial for advancing medical and health sciences. It encompasses a unique blend of statistical methods tailored to address biological questions, making it indispensable for researchers and healthcare professionals alike.
Biostatistics: A discipline that involves the application of statistical techniques to scientific research in health-related fields, including biology, public health, and medicine. This field focuses on the development and application of data analysis methods to gather, summarise, and interpret complex biological data.
Key Concepts in Biostatistics for Beginners
To navigate the field of biostatistics, understanding its foundational concepts is crucial. These key concepts include probability distributions, hypothesis testing, and p-values, among others. Each plays a vital role in the interpretation and analysis of data within the context of health and medicine.
For instance, probability distributions help in understanding how data are spread out or distributed across different values. This is crucial in determining the likelihood of specific outcomes in biological experiments or health studies.
Hypothesis testing is another fundamental concept, where statisticians use data to test assumptions or hypotheses about the nature of health phenomena or the efficacy of treatments.
Consider a scenario where a new medication is introduced to lower blood pressure. Biostatisticians might set up a hypothesis that this medication does not result in a significant difference in blood pressure levels (null hypothesis) versus the alternative hypothesis that it does. By analysing collected data through controlled experiments, they use hypothesis testing to accept or refute these hypotheses.
Utilising these concepts allows for the robust design of experiments, as well as the accurate collection and interpretation of data, ultimately leading to valid and reliable conclusions in biomedical research.
The Evolution of Biostatistics: From Past to Present
The journey of biostatistics from its inception to its current state is marked by significant milestones and contributions from many pioneering scientists. Initially rooted in simple data collection and analysis, the field has evolved, embracing complex mathematical models and computational techniques to address modern-day challenges in health and biology.
The 20th century saw a rapid expansion in biostatistical methods, partly driven by the need to analyse increasing volumes of data from public health and clinical research. Notable advancements during this period include the development of the randomized controlled trial (RCT), a cornerstone method in clinical research, and the introduction of sophisticated statistical models.
One landmark in the evolution of biostatistics was the work of Ronald A. Fisher in the early 20th century, who introduced key statistical concepts like variance analysis and maximum likelihood estimation. These tools not only enriched statistical methodology but also laid the groundwork for the complex data analysis used in today's biomedical research.
The introduction of computing technology in the latter half of the 20th century marked a turning point in biostatistics, allowing for the processing of large data sets and the implementation of intricate models that were previously unfeasible.
Today, biostatistics is an integral part of groundbreaking research in genetics, epidemiology, and medical imaging, among others, driving discoveries that impact public health policies and individual patient care.
Types of Sampling in Biostatistics
In the study of biostatistics, sampling is a fundamental technique used to collect data from a subset of a larger population. By analysing this sample, statisticians can draw conclusions about the population as a whole. Understanding the different types of sampling methods is crucial for executing studies accurately and efficiently.
An Overview of Sampling Techniques in Biostatistics
Sampling techniques in biostatistics can be broadly categorised into two main types: random sampling and non-random sampling. Each category comprises various methods, each suitable for specific research scenarios. This diversity allows researchers to choose the most appropriate sampling strategy based on their study objectives, population characteristics, and resource availability.
- Random Sampling: In this method, every member of the population has an equal chance of being selected. This approach is critical for reducing bias and ensuring the generalisability of the study findings.
- Non-Random Sampling: These methods don't give all members of the population an equal chance of being selected. These are used when random sampling is impractical, too costly, or when specific subsets of the population are of interest.
Random sampling includes techniques like simple random sampling, stratified sampling, and cluster sampling, among others. Non-random sampling methods encompass convenience sampling, quota sampling, and purposive sampling, to name a few. Each method has its advantages and limitations, impacting the study's design, data collection, and final analysis.
Comparing Random and Non-Random Sampling Methods
Choosing between random and non-random sampling methods depends on several factors, including the study's aim, the nature of the population, and the available resources. Each method influences the study's outcomes, validity, and potential bias.
Random Sampling MethodsAdvantages:
- Minimizes selection bias
- Increases the generalisability of the results to the broader population
- Fosters the application of statistical analysis due to randomisation
- May be difficult and costly to implement, especially for large or widely dispersed populations
- Requires access to a complete list of the population, which might not always be available
Non-Random Sampling MethodsAdvantages:
- More practical and cost-effective in many situations
- Ideal for preliminary or exploratory research where specific trends or phenomena need to be investigated
- Results may not be generalisable to the entire population
- Potential for higher bias, impacting the study's validity
Consider a study aiming to analyse dietary habits among teenagers. Using simple random sampling, a researcher could randomly select a subgroup of teenagers from schools across the country, ensuring a representative mix. Alternatively, if the focus is on teenagers with specific dietary restrictions, like vegetarians or vegans, purposive sampling, a non-random method, would allow the researcher to specifically include individuals that fit these criteria.
Cluster Sampling: A common random sampling method that divides the population into clusters (like schools or neighbourhoods) and then randomly selects some of these clusters for in-depth study. This approach can significantly reduce costs and logistical complexities involved in sampling, especially when dealing with large, dispersed populations. However, it may increase the sampling error compared to simple random sampling, as variability within clusters might not be fully captured.
Biostatistical Analysis Techniques
Understanding biostatistical analysis is essential for interpreting data in the life sciences. This field employs statistical techniques to analyse biological data, providing insights into health, disease, and medicine.
Mean, Median, and Mode: The Basics of Biostatistics
Mean, median, and mode are fundamental statistical measures that summarise data sets. Known as measures of central tendency, they help describe the distribution of data points.
Mean is the average of a data set, calculated by adding all the data points and dividing by the number of points.
Median is the middle value when a data set is ordered from least to greatest. If there is an even number of observations, the median is the average of the two middle values.
Mode represents the most frequently occurring value in a data set.
Consider a data set: 2, 3, 5, 5, 7. The mean would be \( (2+3+5+5+7)/5 = 4.4 \), the median is 5 (middle value), and the mode is also 5 (most frequent).
These measures provide different perspectives of a data set, with mean sensitive to outliers and median providing a better reflection in such cases.
In biostatistics, understanding these measures is crucial for data analysis. For example, in testing a new drug, the mean efficacy score from a sample gives an indication of the drug’s average effectiveness, whereas the mode can indicate the most common outcome experienced by participants.
Advanced Biostatistical Analysis: Deep Dive into Modern Techniques
Beyond basic descriptive statistics, biostatistics utilises sophisticated methods to handle complex data. These advanced techniques include regression analysis, ANOVA (Analysis of Variance), and survival analysis, among others.
Regression analysis explores relationships between variables, often used to predict outcomes based on predictor variables.
ANOVA is used to compare means among three or more groups, assessing if at least one group differs significantly.
Survival analysis investigates the expected duration until one or more events happen, such as death in biological organisms.
In a study examining the effect of different diets on weight loss, ANOVA could be applied to compare the average weight loss across diets, determining if any diet is significantly better.
Survival analysis not only estimates the median survival time but also allows for the comparison of survival times across groups or treatment conditions, incorporating censoring (when the exact time of an event is unknown). This technique is extensively used in clinical trials to evaluate drug efficacy over time.
The choice of analysis technique depends on the research question, data type, and distribution. Advanced biostatistical methods enable researchers to uncover deeper insights from their data.
Biostatistics - Key takeaways
- Biostatistics Definition: A field combining biology and statistics to analyse and interpret biological data, facilitating medical and healthcare advancements.
- Sampling in Biostatistics: A technique used to collect data from a part of a population to make inferences about the whole, with random sampling being key for bias reduction.
- Mean, Median, Mode Biostatistics: Fundamental statistical measures that summarise data sets, with mean being the average, median the middle value, and mode the most frequent value.
- Types of Sampling in Biostatistics: Include random sampling (every member of the population has an equal chance to be selected) and non-random sampling (not all members have an equal chance).
- Biostatistical Analysis Techniques: Complex methods like regression analysis, ANOVA, and survival analysis are used to analyse data and predict outcomes or compare group differences.
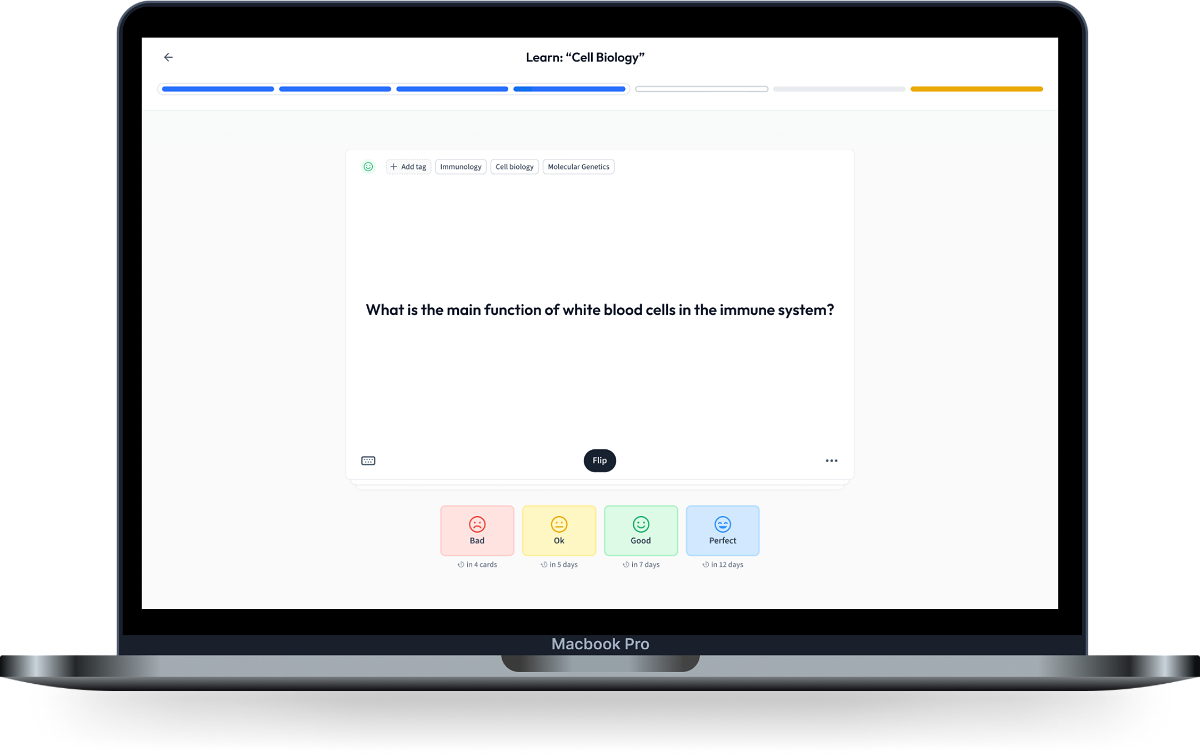
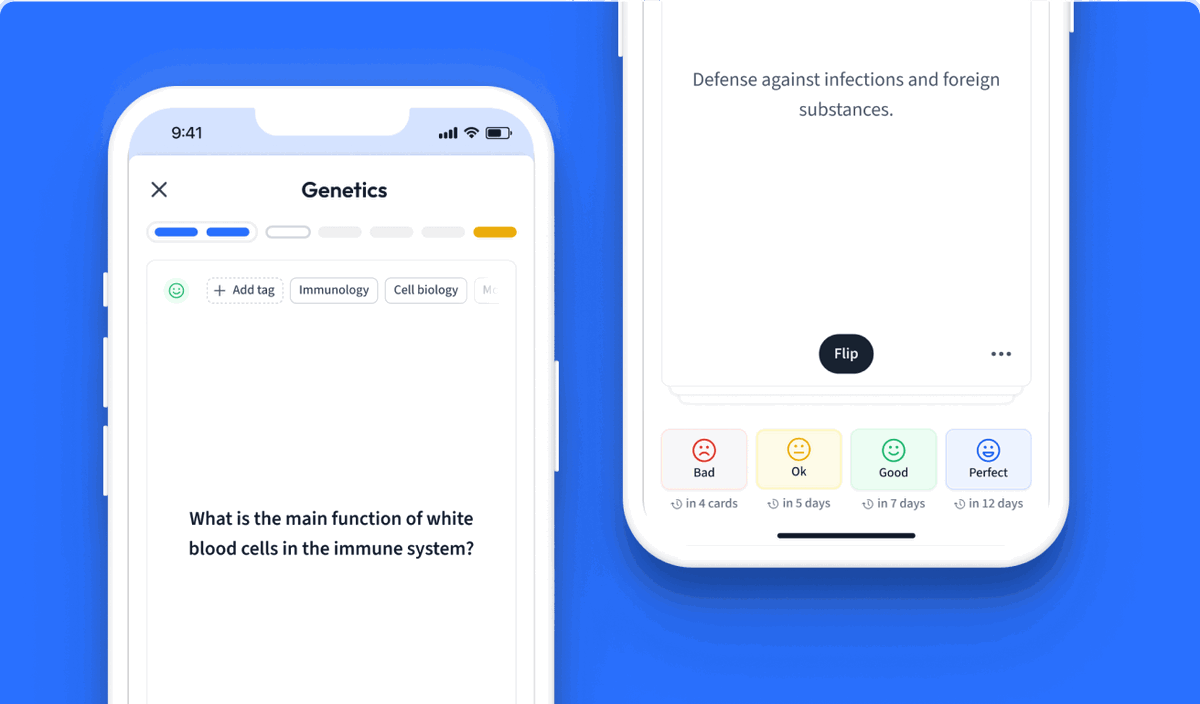
Learn with 0 Biostatistics flashcards in the free StudySmarter app
We have 14,000 flashcards about Dynamic Landscapes.
Already have an account? Log in
Frequently Asked Questions about Biostatistics
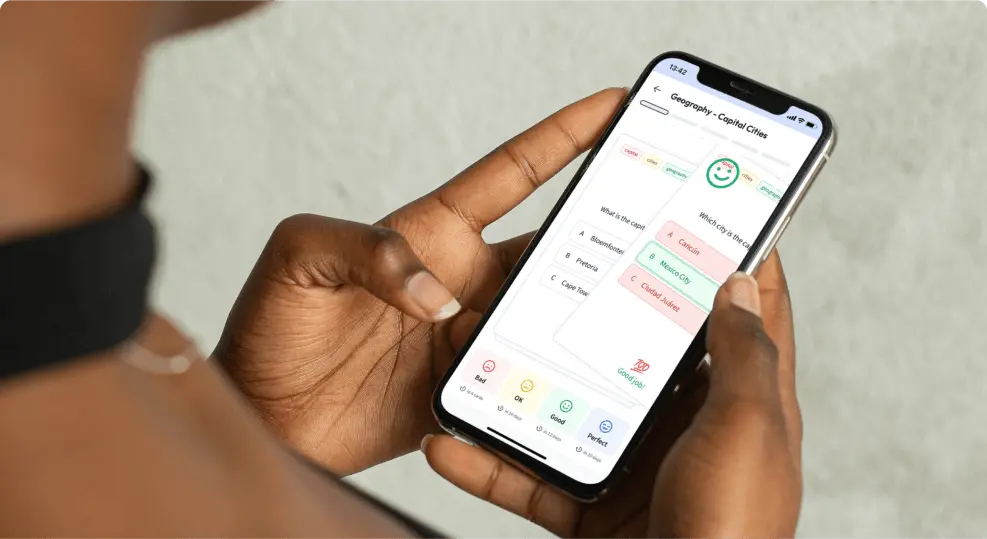
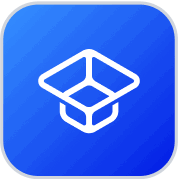
About StudySmarter
StudySmarter is a globally recognized educational technology company, offering a holistic learning platform designed for students of all ages and educational levels. Our platform provides learning support for a wide range of subjects, including STEM, Social Sciences, and Languages and also helps students to successfully master various tests and exams worldwide, such as GCSE, A Level, SAT, ACT, Abitur, and more. We offer an extensive library of learning materials, including interactive flashcards, comprehensive textbook solutions, and detailed explanations. The cutting-edge technology and tools we provide help students create their own learning materials. StudySmarter’s content is not only expert-verified but also regularly updated to ensure accuracy and relevance.
Learn more