Jump to a key chapter
Fig. 1: Even your coffee wants you to be happy!
Confidence Interval for the Difference of Two Means with Known Standard Deviations
If you were only interested in the average coffee price in one city you could do a confidence interval for a population mean. In that case, in order to do a proper confidence interval you would need that:
Either the sample size is large enough (\(n \ge 30\)) or the population distribution is approximately normal.
The sample is random or it is reasonable to assume it is representative of the larger population.
If you know the population standard deviation, \(\sigma\), the confidence interval is given by
\[ \bar{x} \pm (z \text{ critical value})\left(\frac{\sigma}{\sqrt{n}}\right)\]
where \(\bar{x}\) is the sample mean.
But here you have two different cities and you want to compare the average coffee price, so how do you construct the confidence interval? Let's start by listing some of the notation used going forward.
First the population notation:
Population \(1\) | Population \(2\) | |
Population Mean | \( \mu_1\) | \( \mu_2\) |
Population Standard Deviation | \(\sigma_1\) | \(\sigma_2\) |
And now for the samples:
Sample from Population \(1\) | Sample from Population \(2\) | |
Sample Size | \(n_1\) | \(n_2\) |
\(\bar{x}_1\) | \(\bar{x}_2\) | |
Sample Standard Deviation | \(s_1\) | \(s_2\) |
Then the conditions for constructing a confidence interval for the difference of two means are:
The samples are independent.
Either the sample size is large enough (\(n_1 \ge 30\) and \(n_2 \ge 30\)) or the population distribution is approximately normal.
The samples are random or it is reasonable to assume that the samples are representative of the larger population.
These conditions don't change even if you don't know the population standard deviations.
Because the samples are independent and random, you know that
\[ \mu_{\bar{x}_1 - \bar{x}_2} = \mu_1 - \mu_2\]
and that
\[ \sigma_{x_1 - x_2} = \sqrt{\frac{\sigma_1^2}{n_1} + \frac{\sigma_2^2}{n_2} }.\]
Then the confidence interval for the difference in the two population means is
\[\bar{x}_1 - \bar{x}_2 \pm (z \text{ critical value})\sqrt{\frac{\sigma_1^2}{n_1} +\frac{\sigma_2^2}{n_2} } .\]
In general you aren't going to know what the population standard deviations are, but let's look at an example illustrating the use of the formulas.
You do a survey of \(40\) small town coffee shops and \(49\) big city coffee shops, and find that the mean price of a large cup of coffee is \(\$3.75\) and in the big cities it is \(\$ 4.50\). You also know that the population standard deviation in small towns is \(1.20\), and in big cities the population standard deviation of \(0.98\).
Construct a \(99\%\) confidence interval for the difference of their two means, and draw conclusions from it.
Solution:
It helps to lay out the information you have. Call the small city Population \(1\) and the large city Population \(2\). Then you know that
\[ \begin{array}{lll} & n_1 = 40 & \bar{x}_1 = 3.75 & \sigma_1 = 1.20 \\ & n_2 = 49 & \bar{x}_2 = 4.50 & \sigma_2 = 0.98 . \end{array}\]
You know that the \(z\) critical value for a \(99\%\) confidence interval is \(2.58\). Then calculating the confidence interval for the difference in the means,
\[\begin{align} & \bar{x}_1 - \bar{x}_2 \pm (z \text{ critical value})\sqrt{\frac{\sigma_1^2}{n_1} +\frac{\sigma_2^2}{n_2} } \\ & \qquad = 3.75-4.50 \pm 2.58 \sqrt{\frac{(1.20)^2}{40} +\frac{(0.98)^2}{49} } \\ & \qquad = -0.75 \pm 2.58\sqrt{0.036 + 0.0196} \\ & \qquad \approx -0.75 \pm 0.61 \\ & \qquad = (-1.36, -0.14) .\end{align}\]
Now what can you conclude from this? First, you can conclude that the method used to construct this interval estimate is successful in capturing the actual difference in the population means about \(99\%\) of the time.
More importantly, you can conclude with \(99\%\) confidence that the actual difference in the mean price of a large cup of coffee is between \(-\$1.36\) and \(-\$0.14\). Because both endpoints of the confidence interval are negative, you can estimate that the mean price of a large cup of coffee is between \(\$0.14\) and \(\$1.36\) lower in a small town than it is in a big city.
Notice that in the previous example both ends of the confidence interval were negative. What happens if one end is negative and one end is positive? That implies that \(0\) is inside the confidence interval, so in other words it would be plausible that there was no difference in the two means.
Confidence Interval for the Difference of Two Independent Population Means
If you don't know the population standard deviations, but you do know that your samples are independent (meaning that choosing a member of the first population doesn't affect your choice for a member of the second population), then you can calculate the confidence interval using the formula:
\[\bar{x}_1 - \bar{x}_2 \pm (t \text{ critical value})\sqrt{\frac{s_1^2}{n_1} +\frac{s_2^2}{n_2} } ,\]
where the degree of freedom for the \(t\) critical value is calculated by
\[df = \frac{(V_1 + V_2)^2}{\dfrac{V_1^2}{n_1-1} + \dfrac{V_2^2}{n_2-1} },\]
and
\[ V_1 = \frac{s_1^2}{n_1}, \quad V_2 = \frac{s_2^2}{n_2} .\]
This is the same way you would calculate the degree of freedom for a two sample \(t\)-test.
Let's look at an example of applying these formulas and drawing conclusions.
You do a survey of \(40\) small town coffee shops and \(49\) big city coffee shops, and find that the mean price of a large cup of coffee is \(\$3.75\) and in the big cities it is \(\$ 4.50\). You also know that the sample standard deviation in small towns is \(1.00\), and in big cities the sample standard deviation of \(0.70\).
Construct a \(99\%\) confidence interval for the difference of their two means, and draw conclusions from it.
Solution:
First finding \(V_1\) and \(V_2\),
\[ \begin{align} V_1 &= \frac{s_1^2}{n_1} \\ &= \frac{1^2}{40} \\ &= 0.025 \end{align} \]
and
\[ \begin{align} V_2 &= \frac{s_2^2}{n_2} \\ &= \frac{0.70^2}{49} \\ &= 0.01, \end{align} \]
so
\[\begin{align} df &= \frac{(V_1 + V_2)^2}{\dfrac{V_1^2}{n_1-1} + \dfrac{V_2^2}{n_2-1} } \\ &= \frac{(0.025 + 0.01 )^2}{\dfrac{0.025^2}{40-1} + \dfrac{0.01^2}{49-1} } \\ &=\frac{0.001225}{\dfrac{0.000625}{39} + \dfrac{0.0001}{48} } \\ &\approx 67.6 . \end{align}\]
Most \(t\)-tables will not have \(df = 68\) in them, however a calculator will give you the appropriate \(t\)-critical value of \(2.65\).
Then calculating the confidence interval for the difference in the two population means,
\[\begin{align} \bar{x}_1 - \bar{x}_2 & \pm (t \text{ critical value})\sqrt{\frac{s_1^2}{n_1} +\frac{s_2^2}{n_2} } \\ &\quad = 3.75-4.50 \pm (2.65)\sqrt{\frac{1^2}{40} +\frac{0.75^2}{49} } \\ &\quad \approx -0.75 \pm 0.51 \\ &\quad = (-1.26, -0.24). \end{align}\]
So you can conclude with \(99\%\) confidence that the actual difference in the mean price of a large cup of coffee is between \(-\$1.26\) and \(-\$0.24\). Because both endpoints of the confidence interval are negative, you can estimate that the mean price of a large cup of coffee is between \(\$0.24\) and \(\$1.26\) lower in a small town than it is in a big city.
How is the margin of error different from the confidence interval?
Margin of Error of a Confidence Interval for the Difference between Two Population Means
The margin of error is actually defined as half of the width of the confidence interval. So in the case of the difference between two means where you don't know the population standard deviations, the margin of error is given by
\[ \text{margin of error } = (t \text{ critical value})\sqrt{\frac{s_1^2}{n_1} +\frac{s_2^2}{n_2} }. \]
On the other hand, if you do know the population standard deviations the margin of error is
\[ \text{margin of error } = (z \text{ critical value})\sqrt{\frac{\sigma_1^2}{n_1} +\frac{\sigma_2^2}{n_2} } . \]
In either case it is just half the width of the confidence interval.
Confidence Interval for the Difference between Two Means Formula
Being able to use the formula is certainly one part of making a confidence interval. Just as important is being able to use the information the formula gives you to draw conclusions. In fact most statistical software will take data you give it and do the calculations for you!
When looking at the confidence interval for the difference between two means, there are three things that can happen:
Both endpoints of the interval are negative.
Both endpoints of the interval are positive.
One endpoint is negative and one is positive.
You have already seen an example of a conclusion made when both endpoints are negative, so let's take a look at an example of the conclusion you can draw in each of the other two cases.
Suppose you have a new medical treatment, and you want to look at the mean days to recovery of people who get the treatment versus people who don't get the treatment. People were randomly assigned to either the treatment group or a placebo group. Define
- Population \(1\) - people who get the treatment; and
- Population \(2\) - people who get a placebo.
Suppose the \(90\%\) confidence interval for the difference in the two means, \(\bar{x}_1 - \bar{x}_2 \) is \( (14.7, 23.1)\). What conclusion can you draw about whether or not the treatment versus the placebo?
Solution:
Here both of the endpoints of the confidence interval are positive. This means you think \(\mu_1 - \mu_2 > 0\), or in other words that the mean time to recovery for people who got the medical treatment is larger than the mean time to recovery for people who got the placebo, and in fact the recovery time for people who get the medical treatment is longer by at least \(14\) days. Unfortunately this would imply that the new medical treatment does not help people recover faster.
Next, the case when one endpoint is negative and one is positive.
Let's use exactly the same setup as in the previous example. So
- Population \(1\) - people who get the treatment; and
- Population \(2\) - people who get a placebo.
Suppose the \(90\%\) confidence interval for the difference in the two means, \(\bar{x}_1 - \bar{x}_2 \) is \( (-3.4, 4.3)\). What conclusion can you draw about whether or not the treatment versus the placebo?
Solution:
Here zero is included in the confidence interval. That implies that it is plausible that \(\mu_1\) and \(\mu_2\) are the same. In other words, it is plausible that the new medical treatment was no more or less effective than the placebo. So you can say that while the new medical treatment probably didn't help, it also probably wasn't any worse than the placebo.
It is always helpful to see another example.
Example of Confidence Interval for the Difference between Two Population Means
Let's look at something that you might mistake for a difference between two means problem at first.
It is common for students in a class to be given a pre-test, then learn the material, then do an actual test. This is to (hopefully) measure how much students are learning in the class. Is this actually a case where you would construct a confidence interval for the difference between the two population means?
Solution:
Remember that one of the conditions to construct a confidence interval for the difference of two means is that your samples are independent. In this example, a student who takes the pre-test automatically is put in the group to take the actual test. These samples are definitely not independent!
So while it looks like a difference of two means question, in fact you will need to look at the people in the class and the difference in their test scores and do a confidence interval for a population mean.
Just because there is the word "difference" there doesn't imply you have to do a confidence interval for the difference between two means. These are considered matched paired samples, and a standard confidence interval for a population mean is the way to tackle this problem.
Next let's look at an example where the samples are independent.
Suppose that you want to know if the color of the coffee mug impacts how people think about the flavor. You get \(24\) people and assign them randomly to one of two treatment groups; either a white coffee mug or an orange coffee mug.
Is coffee in an orange mug somehow better?
Both groups were given exactly the same coffee and asked to rate the flavor on a scale of \(0\) to \(100\). The results are in the table below.
Sample | Sample size | Mean quality rating | Sample standard deviation |
Sample 1: white coffee mug | \(n_1 = 12\) | \(\bar{x}_1 = 50.35\) | \(s_1 = 20.17\) |
Sample 2: orange coffee mug | \(n_2 = 12\) | \(\bar{x}_2= 61.48\) | \(s_2 = 16.69\) |
Can you conclude that the color of the mug makes a difference in the mean quality rating of the coffee?
Solution:
First let's check to be sure that all of the conditions to construct a confidence interval for the difference of two means are satisfied. The samples are certainly independent and randomly selected, however the sample size is less than \(30\). That means you will need to assume that the distributions of the two quality ratings are approximately normal. It isn't unreasonable to assume that, but it will need to be mentioned when you make a conclusion.
Next you will need to calculate the degrees of freedom. Here
\[ \begin{align} V_1 &= \frac{s_1^2}{n_1} \\ &= \frac{(20.17)^2}{12} \\ & \approx 33.9, \end{align} \]
and
\[ \begin{align} V_2 &= \frac{s_2^2}{n_2} \\ &= \frac{(16.69)^2}{12} \\ &\approx 23.2, \end{align}\]
so
\[\begin{align} df &= \frac{(33.9 + 23.2)^2}{\dfrac{(33.9)^2}{12-1} + \dfrac{(23.2)^2}{12-1} } \\ &= \frac{3260.41}{\dfrac{1149.21}{11} + \dfrac{538.23}{11} } \\ &\approx 21.25 . \end{align}\]
A confidence level wasn't given, but a \(95\%\) level is common to use. So the \(t\)-critical value would be \(2.08\).
Then constructing the confidence interval,
\[ \begin{align} \bar{x}_1 - \bar{x}_2 &\pm (t \text{ critical value})\sqrt{\frac{s_1^2}{n_1} +\frac{s_2^2}{n_2} } \\ &\quad = 50.35 - 61.49 \pm 2.08\sqrt{\frac{(20.17)^2}{12} +\frac{(16.69)^2}{12} } \\ & \quad \approx -11.14 \pm 14.72 \\ &\quad = (-26.85, 4.67).\end{align}\]
So assuming that the distributions of the two quality ratings are approximately normal, you can conclude with \(95\%\) confidence that the actual difference in the mean rating is between \(-26.85\) and \(4.67\). Because zero is in the confidence interval it is plausible to conclude that there is no difference in the mean flavor scale rating between the white mug and the orange mug.
Confidence Interval for the Difference of Two Means - Key takeaways
- The conditions for constructing a confidence interval for the difference of two means are:
The samples are independent.
Either the sample size is large enough (\(n_1 \ge 30\) and \(n_2 \ge 30\)) or the population distribution is approximately normal.
The samples are random or it is reasonable to assume that the samples are representative of the larger population.
If you know the population standard deviations, the formula for the confidence interval for the difference in the two means is
\[\bar{x}_1 - \bar{x}_2 \pm (z \text{ critical value})\sqrt{\frac{\sigma_1^2}{n_1} +\frac{\sigma_2^2}{n_2} } ,\]where \(\bar{x}_1\) is the mean for sample \(1\), \(\bar{x}_2\) is the mean for sample \(2\), \(\sigma_1 \) is the standard deviation for population \(1\), and \(\sigma_2\) is the standard deviation for population \(2\).
The degree of freedom for a confidence interval for the difference of two means is calculated by
\[df = \frac{(V_1 + V_2)^2}{\dfrac{V_1^2}{n_1-1} + \dfrac{V_2^2}{n_2-1} },\]
where \(n_1\) and \(n_2\) are the sample sizes, \(s_1\) and \(s_2\) are the sample standard deviations, and
\[ V_1 = \frac{s_1^2}{n_1}, \quad V_2 = \frac{s_2^2}{n_2} .\]
If you don't know the population standard deviation, the formula for the confidence interval for the difference in two means is
\[\bar{x}_1 - \bar{x}_2 \pm (t \text{ critical value})\sqrt{\frac{s_1^2}{n_1} +\frac{s_2^2}{n_2} } ,\]where \(n_1\) and \(n_2\) are the sample sizes, \(s_1\) and \(s_2\) are the sample standard deviations, and \(\bar{x_1}\) and \(\bar{x}_2\) are the sample means.
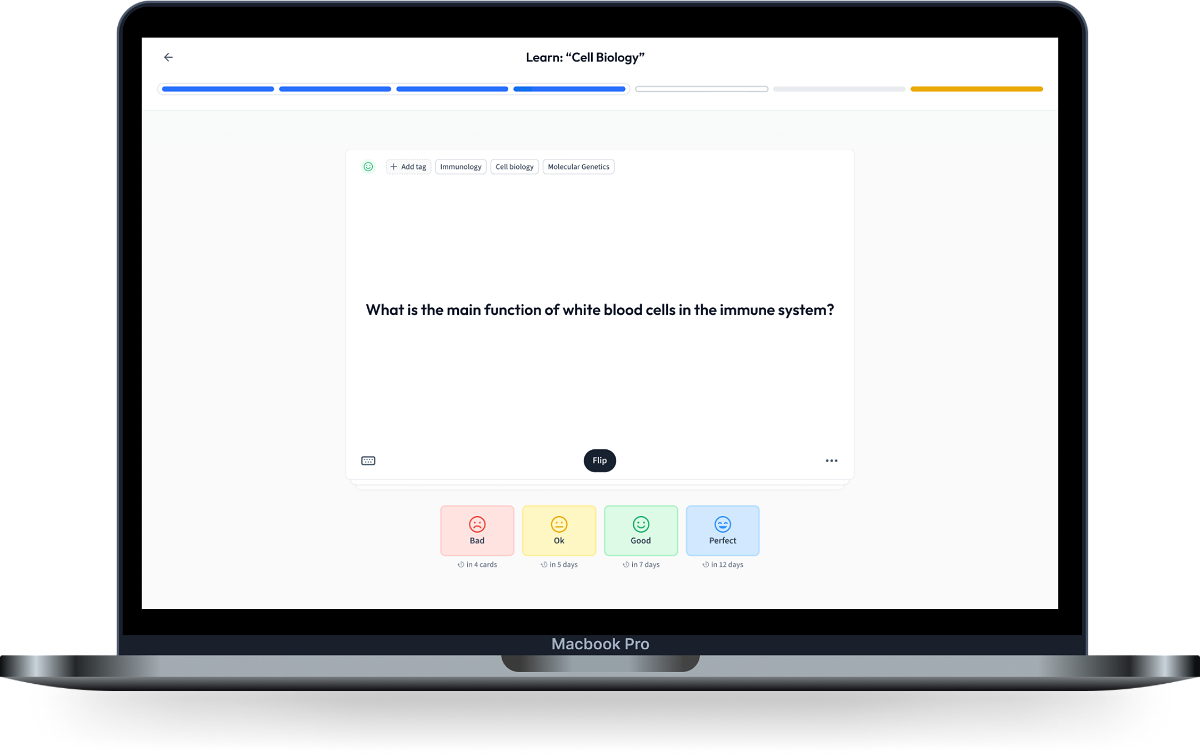
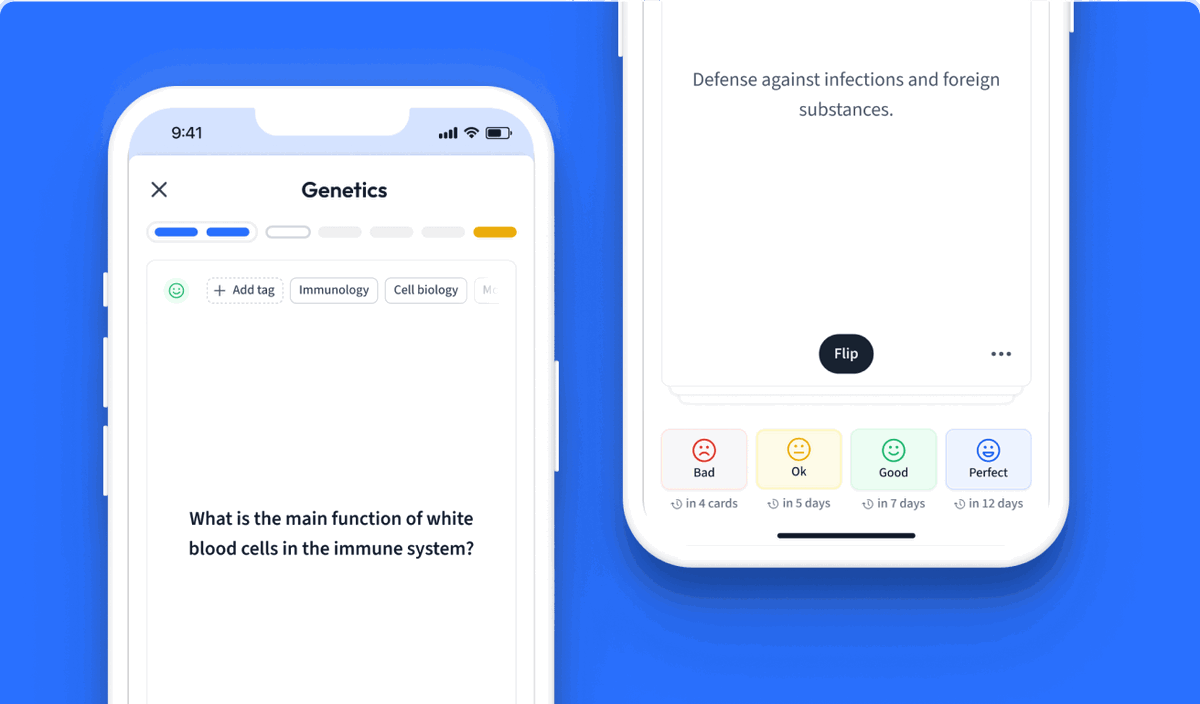
Learn with 11 Confidence Interval for the Difference of Two Means flashcards in the free StudySmarter app
We have 14,000 flashcards about Dynamic Landscapes.
Already have an account? Log in
Frequently Asked Questions about Confidence Interval for the Difference of Two Means
What is the 95% confidence interval for the difference in means?
It is an interval that you can say the true difference in population means will be in 95% of the time.
How do you compare two population means?
It depends on if they are independent or not. If the are independent you construct a confidence interval for the difference of two means. If they are dependent, also called match-paired, then you use a confidence interval for a population mean instead.
How do you find the significant difference between two means?
Construct a confidence interval for the difference of two means. If zero is in the confidence interval then it is plausible there is no difference in the actual mean of the two populations.
Why do we use p 0.05 level of significance?
It is mostly convention. Sometimes you will use a significance level of 0.1 or 0.01, depending on the application.
What is the difference between p-value and confidence interval?
A confidence interval gives you a range of values that the true value of the parameter is in with a certain confidence level. The p-value is a level of significance number, not an interval.
What is p value at 95 confidence interval?
Generally a p value is used to reject or accept a null hypothesis, not when constructing a confidence interval.
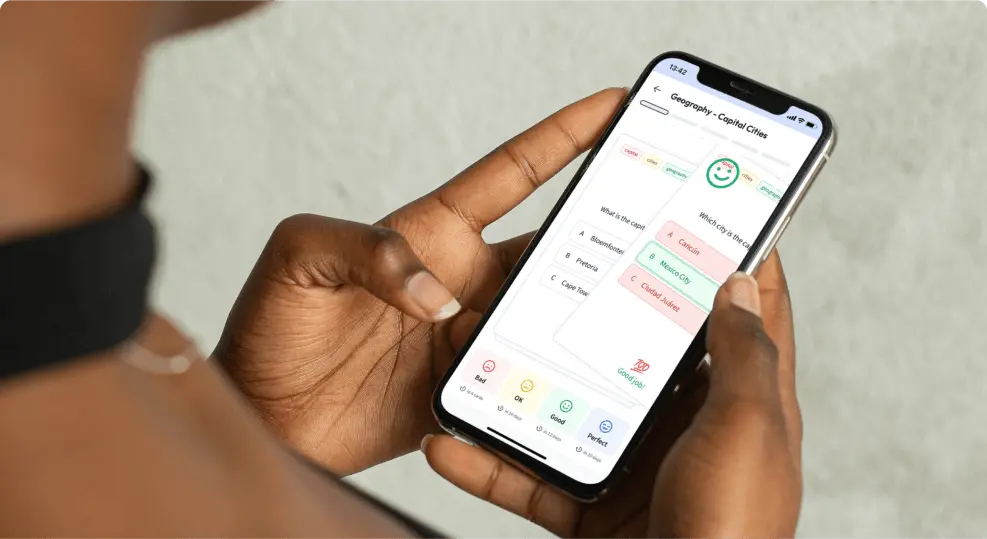
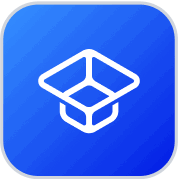
About StudySmarter
StudySmarter is a globally recognized educational technology company, offering a holistic learning platform designed for students of all ages and educational levels. Our platform provides learning support for a wide range of subjects, including STEM, Social Sciences, and Languages and also helps students to successfully master various tests and exams worldwide, such as GCSE, A Level, SAT, ACT, Abitur, and more. We offer an extensive library of learning materials, including interactive flashcards, comprehensive textbook solutions, and detailed explanations. The cutting-edge technology and tools we provide help students create their own learning materials. StudySmarter’s content is not only expert-verified but also regularly updated to ensure accuracy and relevance.
Learn more