Jump to a key chapter
Key Concepts of Mean and Variance of Poisson Distributions
In the world of statistics, Poisson distributions play an essential role in understanding the behaviour of a particular type of random event. It's crucial to have a grip on the key concepts that define these distributions: mean and variance. These two properties help us understand the distribution's central tendency and its dispersion, which in turn assists in making predictions and drawing conclusions from the data.A Poisson distribution is a discrete probability distribution that represents the number of events in a fixed interval of time or space, given a fixed average rate of occurrence, and with the events occurring independently of each other.
In a Poisson distribution, both the mean and variance are equal to the average rate of occurrences, which is denoted as \(\lambda\). The Poisson probability mass function (PMF) is given by: \(P(X=k)=\frac{e^{-\lambda}\lambda^k}{k!}\), where \(k\) is the number of events, and \(e\) is the base of the natural logarithm, approximately equal to 2.71828.
Importance of Poisson distribution in statistics
Poisson distributions are frequently employed in various fields, such as:- Physics: For counting the number of particles detected in a particular area.
- Telecommunications: To model the number of incoming calls or messages to a customer service centre.
- Finance: To analyse the number of customers who visit a store during a specific timeframe.
Connection between Mean and Variance
In Poisson distributions, there is a unique connection between the mean and variance, as they are equal to each other. This property simplifies the analysis and allows for easy prediction of the distribution's characteristics. Let \(X\) be a random variable representing the number of events occurring in a fixed interval following a Poisson distribution. We start by defining the mean \(\lambda\) and variance \(Var(X)\): \[E(X)=\lambda \quad \text{and} \quad Var(X)=\lambda\] When comparing discrete distributions like the Poisson distribution to other probability models (e.g., the binomial distribution), this relationship between mean and variance means that understanding one central property informs our understanding of the other. Additionally, the mean and variance's connection to the rate parameter \(\lambda\) helps simplify analysis in real-life situations where the rate of occurrence is known or measured. By knowing the relationship between the mean, variance, and rate parameter, we can efficiently analyse the properties of a distribution and make informed decisions based on the data.Derivation of Mean and Variance of Poisson Distribution
In this section, we will delve into the steps to derive the Mean and Variance of Poisson Distribution and their relationship. Understanding these derivations will help you grasp the intuition behind the connection between the mean, variance, and rate parameter in Poisson Distribution.
Steps to derive the Mean of Poisson Distribution
Deriving the mean of a Poisson Distribution involves a series of mathematical steps. The mean, represented as \(E(X)\), equals the rate parameter \(\lambda\). We will now outline the detailed steps to derive the mean: 1. Start with the Poisson probability mass function (PMF): \(P(X=k)=\frac{e^{-\lambda}\lambda^k}{k!}\) 2. To obtain the mean, we need to find the expected value of \(X\), which is denoted as \(E(X)\). \(E(X)=\sum_{k=0}^{\infty}kP(X=k)\) 3. Plug the Poisson PMF into the equation for the expected value: \(E(X)=\sum_{k=0}^{\infty}k\frac{e^{-\lambda}\lambda^k}{k!}\) 4. Notice that the sum begins at \(k = 0\), but since \(k = 0\) results in a zero term, we can start the sum at \(k = 1\). \(E(X)=\sum_{k=1}^{\infty}k\frac{e^{-\lambda}\lambda^k}{k!}\) 5. Simplify the equation by reducing the power and factorial terms: \(E(X)=e^{-\lambda}\sum_{k=1}^{\infty}\frac{\lambda^k}{(k-1)!}\) 6. Take \(\lambda\) as a common factor: \(E(X) = e^{-\lambda}\lambda\sum_{k=1}^{\infty}\frac{\lambda^{k-1}}{(k-1)!}\) 7. Make a substitution \(m = k - 1\) and adjust the index of the sum: \(E(X) = e^{-\lambda}\lambda\sum_{m=0}^{\infty}\frac{\lambda^m}{m!}\) 8. Recognise that the sum is now the Taylor series expansion of \(e^\lambda\): \(E(X) = e^{-\lambda}\lambda e^{\lambda}\) 9. Simplify the equation: \(E(X) = \lambda\) Therefore, the mean of a Poisson Distribution is equal to the rate parameter \(\lambda\).Deriving the Variance of Poisson Distribution formula
Similarly, we can derive the variance formula of a Poisson Distribution, which is denoted as \(Var(X)\). The variance of a Poisson Distribution equals the rate parameter \(\lambda\). Below are the steps to derive the variance: 1. Recall that the variance is given by: \(Var(X) = E(X^2) - [E(X)]^2\) 2. We already know that \(E(X) = \lambda\), so we need to find the expected value of \(X^2\), denoted by \(E(X^2)\). \(E(X^2) = \sum_{k=0}^{\infty}k^2P(X=k)\) 3. Insert the Poisson PMF into the equation for the expected value: \(E(X^2) = \sum_{k=0}^{\infty}k^2\frac{e^{-\lambda}\lambda^k}{k!}\) 4. Modify the sum to start at \(k = 1\), since the term for \(k = 0\) is zero: \(E(X^2) = \sum_{k=1}^{\infty}k^2\frac{e^{-\lambda}\lambda^k}{k!}\) 5. Simplify the equation: \(E(X^2) = e^{-\lambda}\sum_{k=1}^{\infty}\frac{k\lambda^k}{(k-1)!}\) 6. Make a substitution \(m = k - 1\) and adjust the index of the sum: \(E(X^2) = e^{-\lambda}\lambda^2\sum_{m=0}^{\infty}\frac{(m+1)\lambda^m}{m!}\) 7. Divide the sum into two parts: \(E(X^2) = e^{-\lambda}\lambda^2\left(\sum_{m=0}^{\infty}\frac{\lambda^m}{m!} + \sum_{m=0}^{\infty}\frac{m\lambda^m}{m!}\right)\) 8. Recognise again that these sums represent Taylor series expansions of \(e^\lambda\), and use the previously derived mean equation: \(E(X^2) = e^{-\lambda}\lambda^2\left(e^{\lambda}(\lambda + 1)\right)\) 9. Simplify the equation: \(E(X^2) = \lambda(\lambda + 1)\) 10. Plug back the expected values into the variance equation: \(Var(X) = \lambda(\lambda + 1) - \lambda^2\) 11. Simplify to get the final result: \(Var(X) = \lambda\) Thus, the variance of a Poisson Distribution is equal to the rate parameter \(\lambda\).Relationship between Mean and Variance in Poisson Distribution
As we have derived both the mean and variance of a Poisson Distribution, it is evident that the mean and variance share a unique relationship. In Poisson Distributions, the mean and variance are equal to each other and to the rate parameter \(\lambda\): \[E(X) = Var(X) = \lambda\] This relationship simplifies the analysis of Poisson Distributions, allowing us to study the central tendency and dispersion using a single measure \(\lambda\).Calculating Standard Deviation of Poisson Distribution
In a Poisson distribution, an important property to consider is the standard deviation, which gives useful information about the dispersion or spread of the data. By examining the standard deviation, we can gain insights into how far an event's occurrences deviate from the average rate, \(\lambda\), in the given distribution.Significance of Standard Deviation in Poisson Distribution
The standard deviation of a Poisson distribution represents the average dispersion of the number of events around the mean. It helps us assess how the data is distributed and what to expect from the event occurrences. The standard deviation can be used to:- Measure the consistency of event occurrences in the data.
- Evaluate the level of uncertainty and variability in the data.
- Identify outliers or unusual events that deviate significantly from the mean.
- Estimate the range within which a specific percentage of event occurrences are expected to fall, using various rules such as the Empirical Rule or Chebyshev's Inequality.
Relation between Variance and Standard Deviation
In any probability distribution, including the Poisson distribution, the variance and standard deviation have a direct relationship: \[Standard\ Deviation (SD) = \sqrt{Variance}\] As we have already derived the mean and variance for a Poisson distribution to be equal to \(\lambda\), it follows that the variance and standard deviation share a connection as well. For the standard deviation, denoted \(\sigma\), in a Poisson distribution, we get: \[\sigma = \sqrt{Var(X)}\] Replacing \(\lambda\) for \(Var(X)\), as they are equal, we get: \[\sigma = \sqrt{\lambda}\] The standard deviation in a Poisson distribution is, therefore, the square root of the rate parameter \(\lambda\). By knowing the standard deviation, we can easily determine the spread of the data and gain insights into its variability. In summary, the standard deviation in a Poisson distribution is a significant property that measures the dispersion of events around the mean. It assists in data analysis and decision-making applications in various fields. Understanding the relationship between variance and standard deviation further simplifies the calculations and supports a deeper understanding of the distribution's characteristics. By knowing the variance, or rate parameter \(\lambda\), we can easily derive the standard deviation as \(\sqrt{\lambda}\) and gain valuable information on the spread of the data within the Poisson distribution.Mean and Variance of Poisson Distributions in Practical Applications
Understanding the mean and variance of Poisson distributions adds significant value to a variety of practical applications in various fields. By accurately analysing the central tendency and dispersion of events, we can make informed decisions and design efficient processes or systems accordingly.Real-life examples of Poisson Distribution
Poisson distributions appear in a wide range of real-life situations, where events occur independently, and the mean rate of occurrences is constant over time. Some practical examples of Poisson distributions include:- Call centres: The number of incoming calls per hour in a customer service centre follows a Poisson distribution. Analysing the mean and variance can help in workforce planning and overall efficiency.
- Transportation: The number of vehicles passing through a toll booth in a given time. The analysis of mean and variance supports decisions on infrastructure and resource allocation.
- Website traffic: The number of visits to a website per day. Understanding the mean and variance can help improve the site's performance, allocate server resources, and develop marketing strategies.
- Biology: The number of mutations in a fixed length of DNA sequence. The analysis of mean and variance aids in understanding the mutation rates and improving gene editing technologies.
- Inventory management: The demand for a product in a store during a specific timeframe. Analysing the mean and variance can help optimise stock management and reduce wastage.
Importance of Mean and Variance in decision making
In the mentioned real-life examples and many others, the mean and variance of Poisson distributions play a crucial role in decision-making processes. Accurate knowledge about the central tendency and dispersion of events enables better outcomes and more efficient systems. Some key aspects of decision-making influenced by Poisson distributions include:- Resource allocation: By knowing the mean and variance, organisations can optimise resources to meet demand and avoid over- or underutilisation. In call centres, for instance, this helps in scheduling employees and ensuring efficient operation.
- Capacity planning: Analysing mean and variance aids in determining the required capacity for systems or infrastructure. For example, transportation systems can be optimised by considering the number of vehicles passing through a toll booth within a given time.
- Risk management: Understanding the dispersion of events enables better risk assessment and contingency planning. In finance, for example, assessing the credit default risk or investment returns using Poisson distributions can provide valuable insights for informed decision-making.
- Quality control: Poisson distributions can help identify deviations from expected behaviour and enhance quality assurance. For instance, in biology, detecting anomalous mutation rates in a DNA sequence can lead to improved gene editing technologies.
- Prediction and forecasting: Knowing the mean and variance in Poisson distributions allows for accurate predictions of future occurrences. This can be applied in various scenarios, such as stock price predictions, sales forecasting, or seasonal demand patterns.
Mean and Variance of Poisson Distributions - Key takeaways
Mean and Variance of Poisson Distributions are essential in understanding the behaviour of a particular type of random event in statistics.
In Poisson distributions, the mean and variance are equal to the average rate of occurrences, denoted as \(\lambda\).
The Poisson probability mass function (PMF) is given by: \(P(X=k)=\frac{e^{-\lambda}\lambda^k}{k!}\), where \(k\) is the number of events, and \(e\) is the base of the natural logarithm.
The standard deviation in a Poisson distribution is related to the variance and can be calculated as \(\sqrt{\lambda}\).
Understanding mean and variance of Poisson distributions is crucial in various real-life applications, including decision-making, resource allocation, capacity planning, risk management, and prediction.
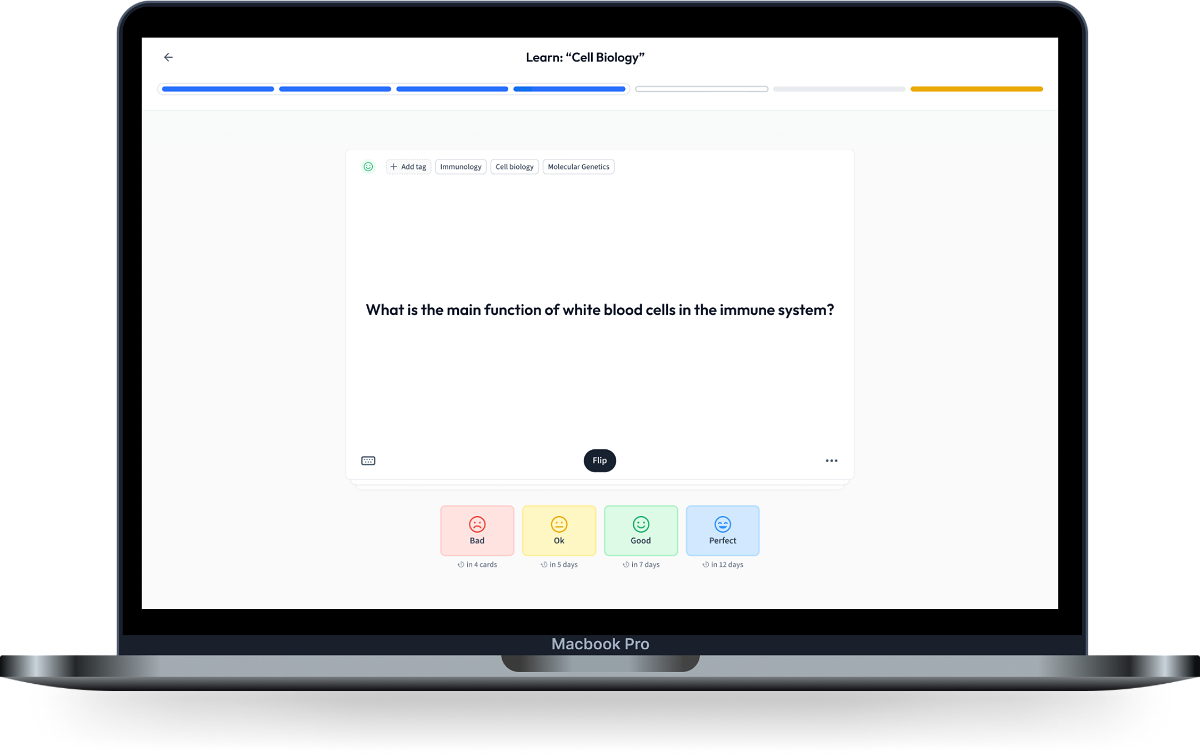
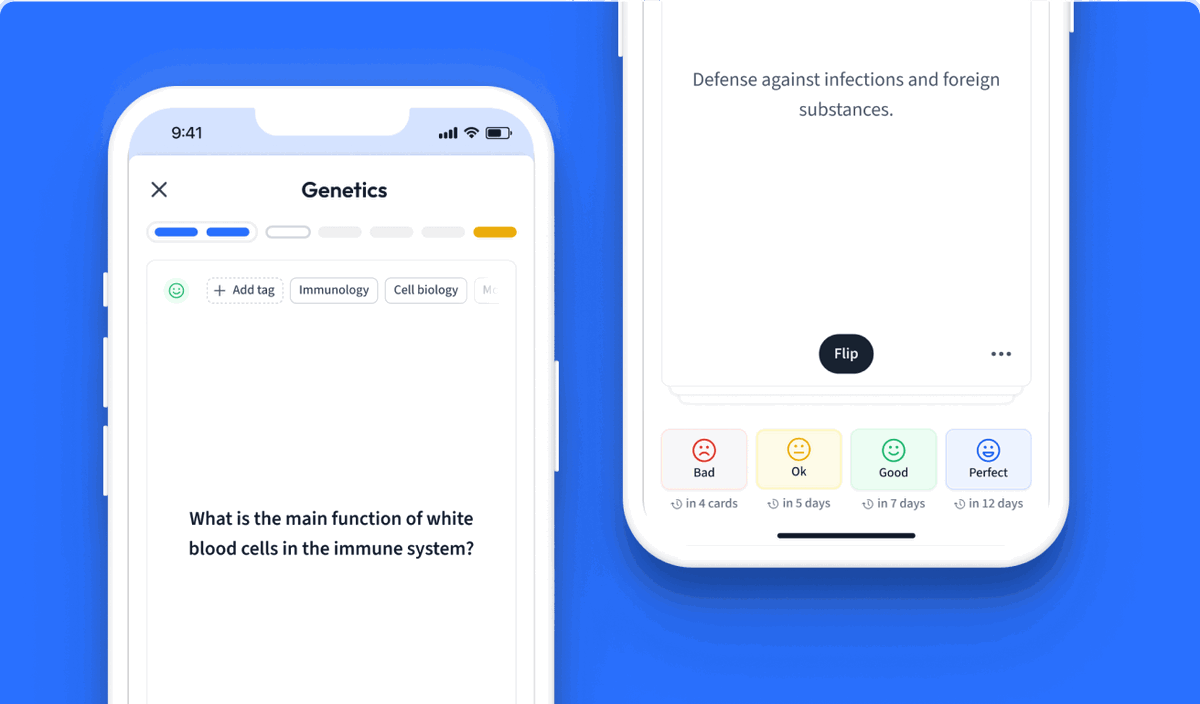
Learn with 15 Mean and Variance of Poisson Distributions flashcards in the free StudySmarter app
We have 14,000 flashcards about Dynamic Landscapes.
Already have an account? Log in
Frequently Asked Questions about Mean and Variance of Poisson Distributions
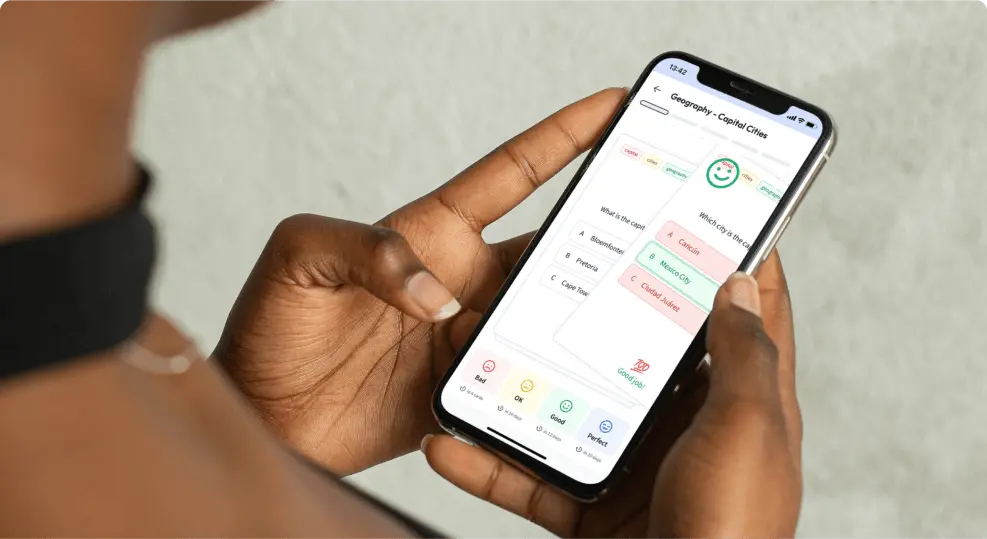
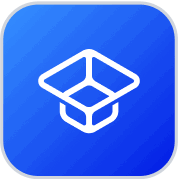
About StudySmarter
StudySmarter is a globally recognized educational technology company, offering a holistic learning platform designed for students of all ages and educational levels. Our platform provides learning support for a wide range of subjects, including STEM, Social Sciences, and Languages and also helps students to successfully master various tests and exams worldwide, such as GCSE, A Level, SAT, ACT, Abitur, and more. We offer an extensive library of learning materials, including interactive flashcards, comprehensive textbook solutions, and detailed explanations. The cutting-edge technology and tools we provide help students create their own learning materials. StudySmarter’s content is not only expert-verified but also regularly updated to ensure accuracy and relevance.
Learn more