Jump to a key chapter
Understanding Multivariate Analysis
Multivariate analysis represents a pivotal part of modern statistics, facilitating the understanding and interpretation of the complexity inherent in real-world data. This type of analysis is key for recognising patterns, predicting future outcomes, and making decisions based on multiple variables.
Basics of multivariate statistical analysis
Multivariate statistical analysis is the process of analysing data that involves more than one variable to understand the relationship between them. Unlike simpler analysis forms that investigate one or two variables, multivariate analysis dives deep into datasets with multiple variables to uncover hidden patterns and correlations.Essential techniques in this field include multiple regression, factor analysis, and cluster analysis. These methods help in simplifying complex data sets, making them understandable and actionable.
Multivariate analysis: A branch of statistics that examines the influence of multiple variables on a certain outcome. It's used to understand complex phenomena where single or bivariate analysis falls short.
Consider a research study aiming to understand how exercise, diet, and sleep affect mental health. Here, multivariate analysis would consider all three variables simultaneously, unlike univariate or bivariate analyses, which would examine these factors in isolation or pairs.
Applications of multivariate analysis in real life
The applications of multivariate analysis span across various domains, demonstrating its versatility and significance. In healthcare, it aids in predicting patient outcomes based on multiple factors such as age, genetics, and lifestyle choices. In finance, it helps in portfolio management by analysing the performance of multiple assets simultaneously. Furthermore, in marketing, it enables companies to understand consumer behaviour by examining how different demographics respond to products.A noticeable trait of multivariate analysis is its capability of handling complex, real-world scenarios that involve multiple interacting variables, making it invaluable across numerous fields.
Did you know? Multivariate analysis can also help in sports analytics, understanding how various factors like training, diet, and psychology impact an athlete's performance.
Difference between univariate, bivariate, and multivariate analysis
Understanding the distinctions between univariate, bivariate, and multivariate analysis is crucial for selecting the correct statistical method for data analysis.
- Univariate analysis examines one variable at a time, such as the overall performance of a student in exams.
- Bivariate analysis explores the relationship between two variables, for example, how an increase in study hours might affect exam scores.
- Multivariate analysis, on the other hand, investigates more than two variables to understand the dynamics between them and how they jointly influence outcomes.
Multivariate Regression Analysis
Multivariate regression analysis enables researchers and statisticians to examine the relationship between multiple independent variables and more than one dependent variable. This approach is essential in fields where complex interactions and dependencies exist among variables.
Introduction to multivariate regression analysis
Multivariate regression analysis extends beyond simple linear regression by allowing for the simultaneous observation of multiple outcomes. This method is particularly useful in situations where variables are interrelated and where the effect of variables needs to be isolated.It provides a more nuanced understanding of data, enabling the identification of patterns and impacts that would be missed with univariate or bivariate analysis.
Multivariate regression analysis: A statistical technique used to understand the relationship between several independent variables and more than one dependent variable.
In healthcare research, a multivariate regression analysis could be used to study the impact of lifestyle choices (diet, physical activity, smoking status) on multiple health outcomes (blood pressure, cholesterol levels, body mass index).
How multivariate regression analysis works
The foundational concept of multivariate regression analysis involves the development of a mathematical model that can predict the values of multiple dependent variables based on the values of several independent variables. It creates an equation that best fits the observed data points.Let the dependent variables be represented by \(Y_1, Y_2, ..., Y_n\) and the independent variables by \(X_1, X_2, ..., X_m\). The multivariate regression model can be represented as follows: \[Y_1 = \beta_0 + \beta_1X_1 + \beta_2X_2 + ... + \beta_mX_m + \epsilon_1\]\[Y_2 = \beta_0' + \beta_1'X_1 + \beta_2'X_2 + ... + \beta_m'X_m + \epsilon_2\]...where \(\beta\) coefficients represent the impact of each independent variable on the dependent variables, and \(\epsilon\) represents the error terms.
The number of equations in a multivariate regression model corresponds to the number of dependent variables being studied.
Examples of multivariate regression analysis in studies
Multivariate regression analysis finds application across various disciplines, reflecting its flexibility and depth. Here are some instances where it is particularly useful:
- In economics, to study how different economic indicators like inflation rate, interest rate, and unemployment rate simultaneously affect stock market indices.
- In environmental science, to examine how various pollutants affect several aspects of air, water, and soil quality.
- In marketing, to determine how different advertising channels contribute to multiple aspects of customer engagement and sales outcomes.
Multivariate Analysis of Variance (MANOVA)
Multivariate Analysis of Variance, commonly known as MANOVA, is a powerful statistical test that extends the capabilities of the Analysis of Variance (ANOVA) to assess multiple dependent variables simultaneously. This statistical approach is instrumental in understanding the effects that independent variables have on multiple outcomes within a single study.By accommodating more complex research designs, MANOVA provides deeper insights into the interactions and differences among group means when several outcomes are of interest.
Breaking down multivariate analysis of variance
MANOVA assesses whether mean differences among groups on a combination of dependent variables are likely to have occurred by chance. Through this analysis, it's possible to determine not just the impact of independent variables on each dependent variable, but also their collective impact on a set of dependent variables.The essence of MANOVA can be captured by the following equation: \[ U = \sum {\lambda_i(X_i - M_i)^2} \]Here, \(U\) represents the test statistic, \({\lambda_i}\) are the eigenvalues, \(X_i\) the observed values, and \(M_i\) the group means for each dependent variable.
Multivariate Analysis of Variance (MANOVA): A statistical approach that assesses the impact of one or more independent variables on two or more dependent variables simultaneously.
Imagine a study examining the effect of diet (low fat, medium fat, high fat) on body composition outcomes such as weight, BMI, and body fat percentage. MANOVA would allow researchers to assess the combined effect of the diet on all three outcomes simultaneously, revealing whether diet groups differ significantly across the set of body composition variables.
Utilising MANOVA in research
In research, MANOVA serves multiple functions. It is particularly useful when:
- There are multiple interrelated outcomes being studied.
- Reducing the risk of Type I errors is crucial, especially when dealing with multiple ANOVAs separately.
- Exploring the interactions among dependent variables provides additional insights into their relational dynamics.
While ANOVA examines one dependent variable, MANOVA permits a holistic view by considering multiple dependent variables. This not only enhances understanding of the data but also aids in discovery of patterns and correlations that may not be apparent in univariate analyses. It's the multidimensionality of MANOVA that equips researchers with a broader analytical perspective.
Advantages of multivariate analysis of variance over ANOVA
MANOVA offers several advantages over its univariate counterpart, ANOVA, such as:
- Precision: By examining multiple dependent variables together, MANOVA provides a more precise analysis of the data.
- Efficiency: It is more efficient in terms of statistical power and controlling Type I error rate when multiple outcomes are evaluated.
- Insight: MANOVA can uncover interactions and patterns among dependent variables that would be missed with multiple ANOVAs.
MANOVA is particularly effective when the dependent variables are correlated. The analysis takes advantage of this correlation to provide more insightful results.
Advanced Topics in Multivariate Analysis
As the field of statistics evolves, multivariate analysis continues to expand its horizons, offering deeper insights into complex datasets. Advanced topics within this area, such as multivariate time series analysis, applied techniques, and pattern analysis, provide powerful tools for interpreting the interactions between multiple variables over time and across various contexts.
Exploring multivariate time series analysis
Multivariate time series analysis deals with monitoring and interpreting data where multiple variables are observed over specific intervals of time. This technique is especially useful in financial markets, weather forecasting, and engineering, where variables interact dynamically over time.At its core, this analysis aims to predict future values based on historical data. Time series models such as Vector Autoregression (VAR) and Multivariate Vector AutoRegressive Moving Average (VARMA) are commonly employed to capture the temporal dynamics and relationships among the variables.
Multivariate time series analysis: A statistical approach used to predict and interpret the behaviour of variables that change over time and are interdependent.
Consider the analysis of stock market data, where the closing prices, trading volumes, and number of transactions are all interrelated variables observed daily. Multivariate time series analysis can help predict future stock movements based on their past performances.
Applied multivariate statistical analysis techniques
Applied multivariate statistical analysis encompasses a range of techniques aimed at solving real-world problems by analysing datasets with multiple variables. Techniques such as Principal Component Analysis (PCA), Canonical Correlation Analysis (CCA), and Discriminant Analysis (DA) are integral for reducing dimensionality, understanding relationships among variables, and classifying data into distinct groups respectively.The application of these methods spans across various disciplines including biology, marketing, and social sciences, illustrating their versatility in dissecting complex datasets.
Principal Component Analysis (PCA) is particularly effective for data visualisation and identifying patterns in high-dimensional data.
Understanding multivariate pattern analysis
Multivariate pattern analysis (MPA) delves into recognising patterns across multiple variables within datasets, contributing valuable predictions and insights. This analytical method is prevalent in neuroscience for identifying brain activity patterns related to specific cognitive tasks or emotional states.MPA employs sophisticated machine learning algorithms, including support vector machines (SVM) and neural networks, to distinguish between different conditions or categories based on the observed data patterns.
Multivariate pattern analysis: A technique in statistics and machine learning used for identifying and utilising patterns in data that involve multiple variables.
An illustrative example of multivariate pattern analysis is in brain-imaging studies, where it's applied to functional magnetic resonance imaging (fMRI) data to uncover specific patterns associated with various mental tasks. This approach not just analyses the data in rich detail but also contributes to our understanding of brain functionality in different psychological or physiological states.
Multivariate pattern analysis can be significantly enhanced with the incorporation of machine learning techniques, enriching the analytical process with predictive capabilities and pattern recognition.
Multivariate Analysis - Key takeaways
- Multivariate Analysis: A branch of statistics that analyses multiple variables to understand their relationships and collective influence on outcomes.
- Multivariate Statistical Analysis: Involves techniques like multiple regression, factor analysis, and cluster analysis to simplify complex datasets and uncover hidden patterns.
- Multivariate Regression Analysis: A statistical technique that explores the relationship between several independent variables and more than one dependent variable, offering insights into complex interactions and dependencies.
- Multivariate Analysis of Variance (MANOVA): An extension of ANOVA that assesses the effect of independent variables on multiple dependent variables simultaneously, providing a more nuanced view of data.
- Advanced Multivariate Techniques: Includes multivariate time series analysis, applied statistical methods like PCA and MPA, and the use of machine learning for pattern recognition in complex datasets.
Learn faster with the 0 flashcards about Multivariate Analysis
Sign up for free to gain access to all our flashcards.
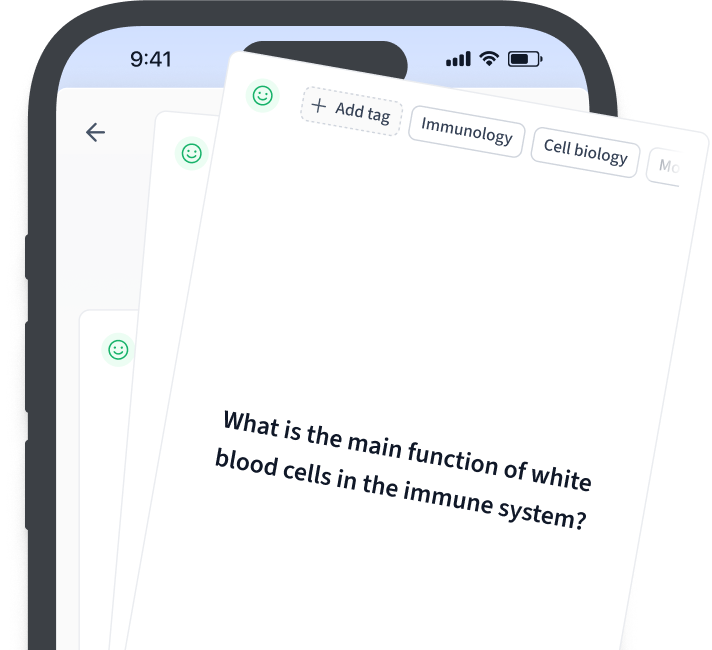
Frequently Asked Questions about Multivariate Analysis
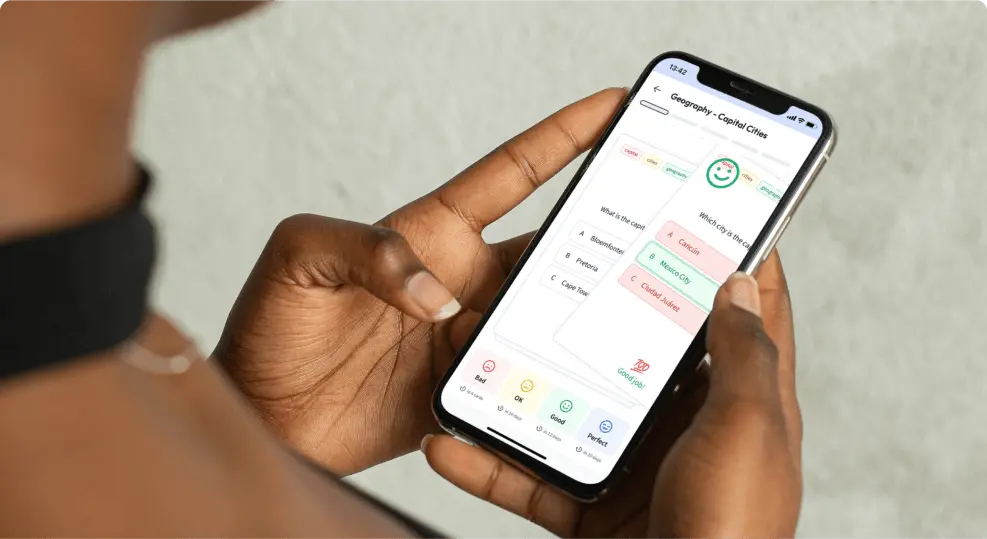
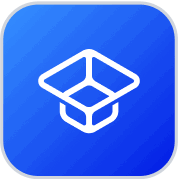
About StudySmarter
StudySmarter is a globally recognized educational technology company, offering a holistic learning platform designed for students of all ages and educational levels. Our platform provides learning support for a wide range of subjects, including STEM, Social Sciences, and Languages and also helps students to successfully master various tests and exams worldwide, such as GCSE, A Level, SAT, ACT, Abitur, and more. We offer an extensive library of learning materials, including interactive flashcards, comprehensive textbook solutions, and detailed explanations. The cutting-edge technology and tools we provide help students create their own learning materials. StudySmarter’s content is not only expert-verified but also regularly updated to ensure accuracy and relevance.
Learn more