Jump to a key chapter
What Is Quantile Regression?
Quantile regression is a statistical technique used to understand the relationship between independent variables and specific quantiles of a dependent variable. Unlike traditional regression methods that focus primarily on mean outcomes, quantile regression offers a more comprehensive view by examining various points of the distribution of the dependent variable. This method is particularly useful for datasets with non-normal distributions or when you're interested in more than just the average effect of independent variables.
Understanding Quantile Regression Definition
Quantile Regression: A statistical method that seeks to estimate the conditional median or other quantiles of the response variable's distribution, as a function of the independent variables. It expands upon traditional regression models by not merely focusing on the mean of the dependent variable.
The Basics of Quantile Regression Analysis
At its core, quantile regression analyses different parts of the distribution of the dependent variable, giving insights into how the independent variables affect the outcome at various points, not just on average. This flexibility makes it an invaluable tool across fields such as economics, medicine, and environmental science, where understanding the impact across different standings of the distribution is critical.
Example: In economic research, quantile regression could be used to study the impact of education on income. Instead of just estimating the average effect on income, it allows researchers to understand how the effect varies across the income distribution, helping to illustrate disparities in income based on educational attainment at different levels.
Quantile regression is especially useful for understanding outliers and the tails of the distribution, areas where traditional regression methods might provide less insight.
Quantile Regression Theory Explained
Underlying the quantile regression methodology is the principle that the impact of independent variables can differ across the distribution of the dependent variable. This requires a shift from focusing solely on the mean to exploring multiple quantiles (e.g., 25th, 50th, 75th percentiles) which reflect different points of the conditional distribution. Quantile regression applies linear programming to estimate these conditional quantiles, offering a robust alternative to understand complex relationships in the data.
The quantile regression model can be mathematically expressed as:
\[Q_{y}(\tau|X) = X\beta(\tau)\]
where \(Q_{y}(\tau|X)\) denotes the \(\tau\)-th quantile of the dependent variable \(y\) given the independent variables \(X\), and \(\beta(\tau)\) represents the quantile-specific coefficients. By varying \(\tau\), analyses can be conducted for different segments of the dependent variable's conditional distribution, providing a comprehensive understanding of the data.
Interestingly, the development of quantile regression has provided unique insights into fields such as econometrics and epidemiology, where assumptions about normality and homoscedasticity often do not hold. By allowing for an examination of the entire distribution rather than just the mean, quantile regression accommodates skewed distributions and heteroscedasticity better than traditional models. This adaptability underscores quantile regression's role in offering nuanced views of data and advancing research analysis methods.
Applications of Quantile Regression
Quantile regression, a versatile statistical method, plays a pivotal role in analysing the effects of variables across different quantiles. This technique has found applications in various fields, providing insights into the complexities of data beyond what is possible with standard regression analysis.
In this segment, we'll explore compelling real-world examples of quantile regression, highlighting its significant contributions to healthcare and economics. These illustrations will shed light on how this method can unravel intricate relationships within data.
Real-World Quantile Regression Examples
Quantile regression analysis is not limited by field or question, which makes it exceptionally adaptive to a multitude of research areas. Its application spans across finance, socio-economic research, healthcare, and more, each of which benefits from its nuanced perspective on data.
For instance, its use in environmental science to predict factors affecting air quality across different pollution levels provides crucial information for policy development. Similarly, in the realm of education, it aids in understanding the influence of various factors on students' performance across different achievement levels.
Example in Real Estate: In the real estate market, quantile regression can be employed to analyse the impact of location, size, and other features on property prices at various points in the price distribution. This enables stakeholders to comprehend how these factors influence not just average prices but the entire spectrum of property values.
Quantile Regression in Healthcare and Economics
In healthcare, quantile regression offers a powerful lens to study the effects of treatments across different severities of a condition. In economics, it helps in dissecting the impact of policy changes across income levels.
These applications illuminate how variables influence outcomes differently across the distribution, unlocking a deeper understanding of causality and impact in complex systems.
Example in Healthcare: Quantile regression analysis can reveal how a new drug affects patients differently based on the severity of their condition. This nuanced understanding aids in tailoring more effective treatment plans.
Example in Economics: Examining the effect of an educational program on income distribution, quantile regression can show how the program benefits people at different income levels, potentially highlighting gaps that need to be addressed to ensure equitable benefits from such programs.
The adoption of quantile regression in healthcare and economics underscores its capacity to provide actionable insights that are often obscured by traditional methods. In healthcare, it enables a refined approach to patient care by accounting for the heterogeneity in patient responses. In economics, it contributes to a more egalitarian perception of policy impact, promoting fairness and inclusivity.
Quantile regression thus stands out as a robust analytical tool that complements and enhances our understanding of data, driving advancements in both theory and practice across disciplines.
Quantile regression's ability to dissect the distribution provides a unique advantage in identifying outliers and understanding the factors influencing these extreme values.
Types of Quantile Regression
Quantile regression, a sophisticated statistical technique, unfolds in various types, each aimed at elucidating different aspects of data analysis. This section delves into Bayesian Quantile Regression and Conditional Quantile Regression, showcasing how these approaches tailor to specific needs within research and data science.
An Insight into Bayesian Quantile Regression
Bayesian Quantile Regression: A form of quantile regression that incorporates Bayesian statistics principles. It not only estimates the conditional quantile of the response variable but also provides a probabilistic interpretation, allowing for a more comprehensive uncertainty assessment around predictions.
Bayesian Quantile Regression extends beyond the capabilities of traditional quantile regression by integrating prior knowledge into the analysis. This incorporation allows for a nuanced understanding of the data, especially in situations with limited observations or when the underlying distribution is complex.
The Bayesian approach is characterised by its use of probability distributions to express uncertainty about the estimated quantiles. Instead of providing a single point estimate, Bayesian quantile regression offers a distribution indicating the range within which the true quantile is likely to lie.
Example: Consider a study on the effect of a new drug on blood pressure. Using Bayesian Quantile Regression, researchers can estimate the impact of the drug across the entire distribution of blood pressure levels. Moreover, they can incorporate prior studies into their current analysis, rendering a richer, more informed interpretation of the effect of the drug.
Bayesian Quantile Regression's approach to integrating prior knowledge is particularly beneficial in fields where historical data or expert knowledge is crucial. For instance, in finance, prior market conditions can provide invaluable insight into current market analyses. This method also stands out in terms of the richness of the data interpretation it offers, especially under conditions of extreme outcomes or outliers.
This depth of analysis, combined with its probabilistic nature, makes Bayesian Quantile Regression a powerful tool in predictive modelling and risk assessment tasks.
Conditional Quantile Regression Overview
Conditional Quantile Regression: Focuses on estimating quantiles of the dependent variable conditional on certain values of the independent variables. It's distinguished by its capacity to model the effects of the explanatory variables across different points in the distribution of the dependent variable.
Conditional Quantile Regression shows how the relationship between independent and dependent variables changes across different quantiles of the dependent variable. This type of regression is particularly useful for understanding heterogeneous effects—how a variable affects different subgroups within the population.
The model can be expressed mathematically as:
\[Q_{y}(\tau|X=x) = x\beta(\tau)\]
where \(Q_{y}(\tau|X=x)\) represents the conditional quantile of interest, \(x\) is the vector of independent variables, and \(\beta(\tau)\) are the coefficients that vary across different quantiles \(\tau\).
Example: In education research, Conditional Quantile Regression could explore how additional hours of study affect students' exam scores across different performance levels. This analysis might reveal that lower-performing students benefit more significantly from extra study hours than their higher-performing counterparts.
Conditional Quantile Regression is especially suited to data with non-uniform effects across the distribution, offering a granular perspective on relationships within the data.
Diving Deeper into Quantile Regression
Quantile regression expands our analytical toolkit, allowing an in-depth look at the relationships between variables across the entire distribution of the dependent variable. It's not just about the averages; this approach shines a light on the nuances missed by traditional regression methods.
This deeper dive focuses on interpreting the results derived from quantile regression analysis. Additionally, it addresses the inherent challenges and effective strategies to overcome them, enhancing one's proficiency in employing this robust statistical technique.
Understanding the Results of Quantile Regression Analysis
Interpreting results from quantile regression analysis involves distinguishing how the independent variables influence different quantiles of the dependent variable. Compared to ordinary least squares (OLS) regression that provides an average effect size, quantile regression elucidates the impacts across the distribution. This is paramount in identifying how these effects vary among the lower, median, and upper quantiles.
For example, a quantile regression analysis might reveal that an educational intervention has a pronounced effect on students performing at the lower quantiles of academic achievement but a minimal impact on those at higher quantiles. Such insights are critical for tailoring targeted interventions.
Example: Consider a dataset analysing the relationship between household income and spending on luxury goods. Quantile regression can show that for households at the 90th percentile of income, spending increases significantly with additional income, whereas the 50th percentile may show a more moderate increase. This analysis is critical for understanding consumer behaviour across different income levels.
Quantile regression coefficients can vary across quantiles, highlighting the necessity of examining results across the entire distribution for a comprehensive analysis.
Challenges and Solutions in Quantile Regression
While quantile regression offers nuanced insights into data, it presents unique challenges. High variance in estimates at extreme quantiles, computational complexity, and the interpretation of coefficient heterogeneity across different quantiles are common hurdles. However, solutions have been developed to address these challenges, ensuring the effective application of quantile regression.
For overcoming high variance at the tails, bootstrapping methods can provide more reliable estimates. Computational challenges are mitigated by advances in software tools that streamline the implementation of quantile regression, even in large datasets. Additionally, a careful, context-aware interpretation of the results, supported by domain knowledge, aids in navigating the seemingly complex interpretations of varying coefficients.
One intricate aspect of implementing quantile regression is handling non-linear relationships and interactions within the model. It requires an expert blend of statistical techniques and domain-specific knowledge to accurately specify models that capture these dynamics. For instance, interaction terms may have different effects across the distribution of the outcome, necessitating advanced modelling strategies and interpretation skills to uncover the true nature of these relationships.
This level of complexity underscores the importance of proficiency in statistical methods and an in-depth understanding of the subject matter, demonstrating that while quantile regression is a powerful tool, its effective utilisation demands a sophisticated approach to data analysis.
Quantile Regression - Key takeaways
- Quantile Regression Definition: A statistical technique estimating the relationships between independent variables and specific quantiles of a dependent variable, expanding beyond mean-focused traditional regressions.
- Quantile Regression Analysis: Analysis of the distribution of the dependent variable at different points, capturing the impact of independent variables across various segments of the distribution.
- Bayesian Quantile Regression: Integrates Bayesian principles to provide probabilistic interpretations and assess uncertainty in conditional quantile estimates.
- Conditional Quantile Regression: Models the relationship between variables across different quantiles of the dependent variable, revealing heterogeneous effects within subgroups.
- Quantile Regression Theory and Applications: Uses linear programming to estimate conditional quantiles and is applied across economics, healthcare, environmental science, and more, offering a detailed view of complex relationships in data.
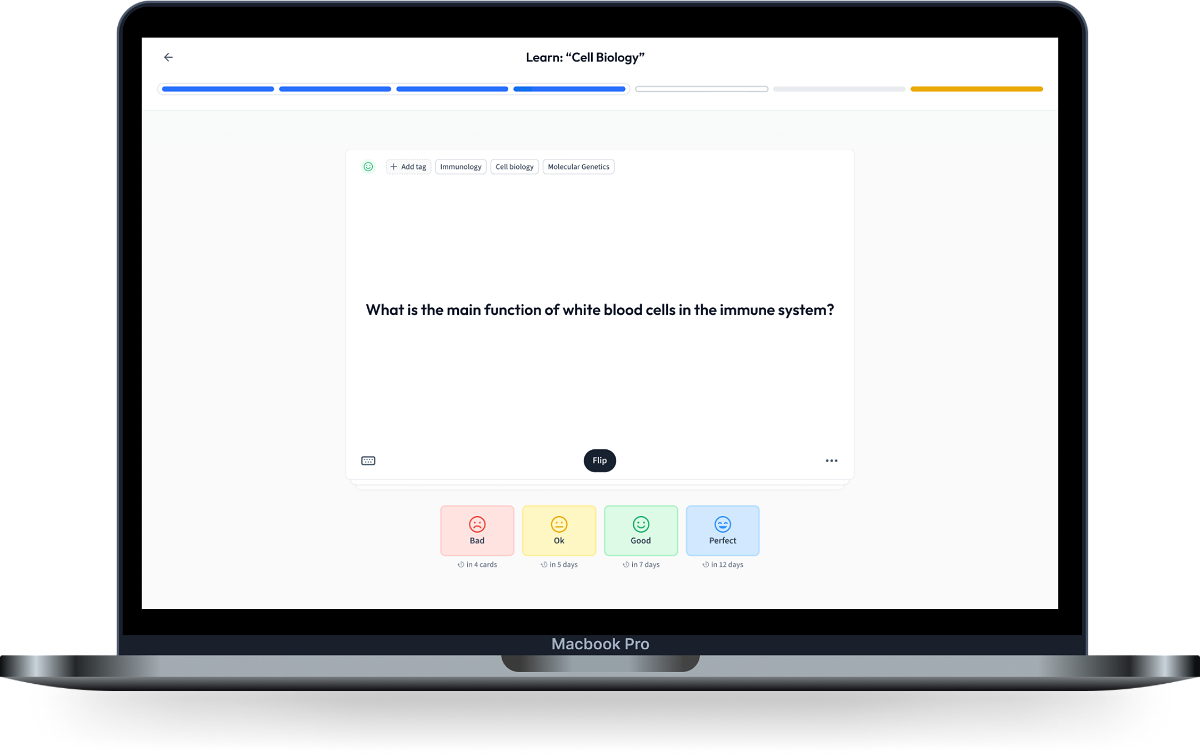
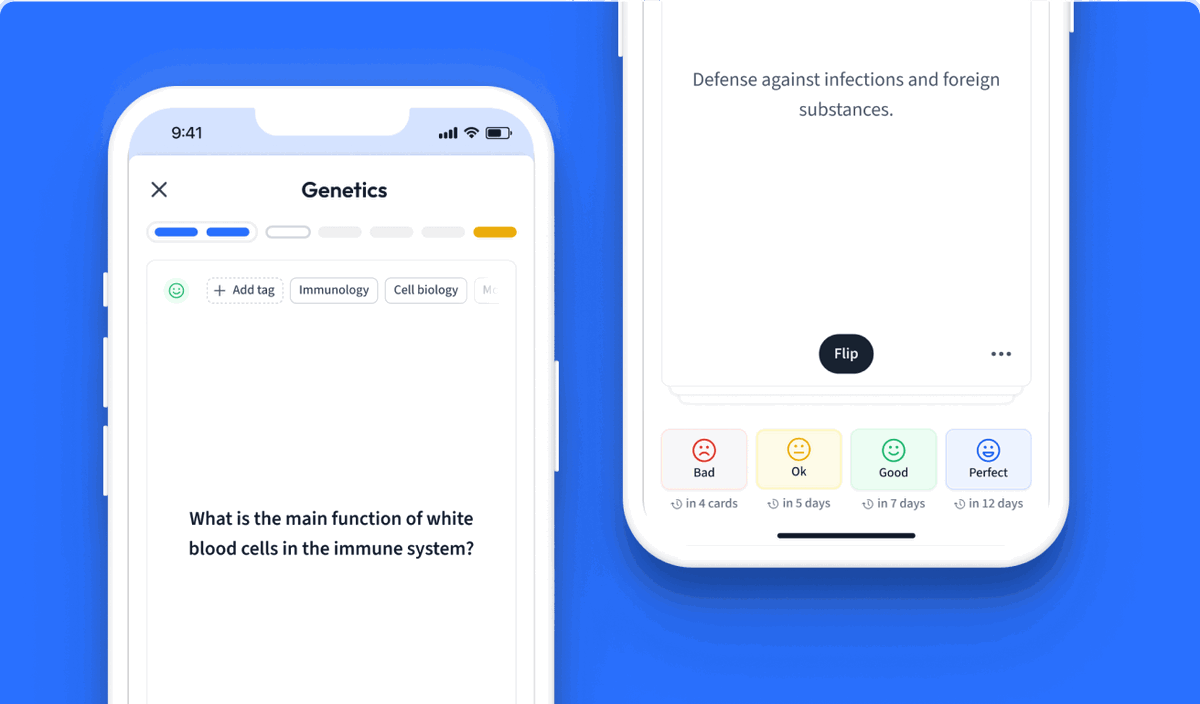
Learn with 0 Quantile Regression flashcards in the free StudySmarter app
We have 14,000 flashcards about Dynamic Landscapes.
Already have an account? Log in
Frequently Asked Questions about Quantile Regression
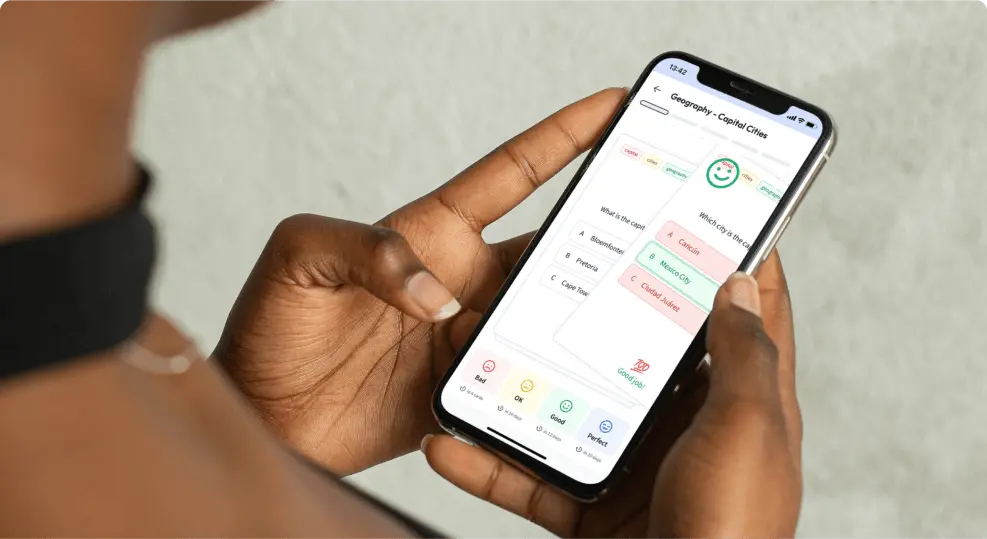
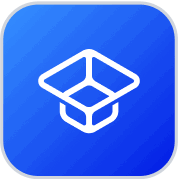
About StudySmarter
StudySmarter is a globally recognized educational technology company, offering a holistic learning platform designed for students of all ages and educational levels. Our platform provides learning support for a wide range of subjects, including STEM, Social Sciences, and Languages and also helps students to successfully master various tests and exams worldwide, such as GCSE, A Level, SAT, ACT, Abitur, and more. We offer an extensive library of learning materials, including interactive flashcards, comprehensive textbook solutions, and detailed explanations. The cutting-edge technology and tools we provide help students create their own learning materials. StudySmarter’s content is not only expert-verified but also regularly updated to ensure accuracy and relevance.
Learn more