Jump to a key chapter
What Is Sequential Analysis?
Sequential analysis is a statistical method that involves the evaluation of data as it is gathered, rather than waiting for a complete set of data before making an analysis. This approach allows for decisions to be made at potentially any point during the data collection process, providing a flexible and often more efficient alternative to traditional methods.
Understanding Sequential Analysis Definition
Sequential analysis: A branch of statistics focusing on the analysis of data as it is collected, permitting the possibility of reaching conclusions and making decisions before the entire set of data is gathered.
Sequential analysis is designed for situations where data arrives in a sequence over time, and immediate decision-making is crucial. This technique stands apart by its ability to evaluate the necessity of additional data continuously and decide on the conclusion of an experiment based on the cumulative data collected to that point.
Example: In medical trials, sequential analysis might be used to assess the effectiveness of a new treatment. If early data indicates the treatment is significantly beneficial or harmful, the trial can be stopped early to either bring a beneficial treatment to market sooner or prevent further exposure to a harmful one.
The Origins and Applications of Sequential Analysis
The concept of sequential analysis was developed during World War II by Abraham Wald, a statistician working on quality control methods for industrial production. Initially aimed at improving the efficiency of ammunition inspection processes, the methodology has since been adopted across various fields for its effectiveness in reducing costs and time required for data analysis.
Sequential analysis plays a pivotal role in clinical trials, where rapid decision making can have significant impacts on patient health outcomes.
Applications of sequential analysis encompass a wide array of areas including, but not limited to, clinical trials, quality control in manufacturing, and environmental monitoring. The method's adaptability allows it to be tailored to specific needs of each field, providing valuable insights that can lead to timely and informed decisions.
One of the groundbreaking applications of sequential analysis is in the field of cybersecurity, where it is utilised to detect intrusions in real time. By continuously analysing network traffic, sequential analysis methods can identify anomalies that may indicate a cybersecurity threat, enabling prompt action to mitigate potential damages. This is a prime example of the method's capability to adapt to new challenges and provide solutions in fast-moving environments.
Sequential Analysis Examples
Sequential analysis provides a flexible approach to data analysis, allowing decisions to be made as the data becomes available. This real-time insight is invaluable across numerous fields, from healthcare to manufacturing. Here, we explore how this method is applied in everyday situations and specialised fields, enhancing understanding and decision-making processes.
Basic Sequential Analysis Example in Real Life
Consider a scenario where a new café wants to determine the ideal price point for its coffee to maximise profits without deterring customers. Instead of setting a fixed price and evaluating the outcome after several months, the café uses sequential analysis to make this decision more efficiently.By adjusting the price point slightly every week and monitoring the change in sales volume and customer feedback, the café can use sequential analysis to identify the optimal pricing strategy in real time. This approach allows the café to find the right balance between affordability for customers and profitability for the business.
Example: If the café starts with a price of £2.50 per cup and increases by £0.10 weekly, they analyse customer reactions and sales data every Sunday night. Using a formula for sequential analysis, perhaps something like \[z = \frac{(p_1 - p_2)}{\sqrt{p(1-p)(\frac{1}{n_1} + \frac{1}{n_2})}}\], where \(p_1\) and \(p_2\) are the proportions of sales before and after the price change, \(p\) is the combined proportion, and \(n_1\) and \(n_2\) are the sample sizes (weeks), they can statistically determine the price effect.
Sequential Analysis in Medical Studies
In the domain of medical research, sequential analysis is particularly crucial. It offers a means to rapidly identify the effectiveness or potential risks associated with new treatments or drugs. By evaluating data as it is collected from clinical trials, researchers can make early determinations about a treatment's benefit, potentially saving or enhancing lives.For example, in a study aiming to determine the efficacy of a new cardiovascular drug, patients are monitored continuously, and data is analysed at predefined intervals. This approach ensures that if the drug demonstrates significant benefit or causes adverse effects, the trial can be adjusted or halted promptly, ensuring patient safety and resource efficiency.
Example: Suppose a trial for a new antihypertensive medication involves two groups - one receiving the medication and the other receiving a placebo. Researchers might analyze the data at the end of each month, calculating the average reduction in blood pressure using a simple statistical model, like the difference in means. If the analysis shows a significant difference indicating the treatment's effectiveness, the study could be concluded early to expedite the medication's availability to patients.
The application of sequential analysis in medical studies highlights its potential to revolutionize healthcare by making treatments available sooner and preventing harm from ineffective or dangerous interventions. The method's reliance on continuous data evaluation promotes a more dynamic research environment. This adaptability is of paramount importance in fields like oncology or infectious diseases, where rapid developments and timely interventions can significantly impact outcomes. As statistical software and computational power continue to evolve, the scope for sequential analysis in medical research will likely expand, offering even more robust tools for scientists and clinicians.
Advanced Topics in Sequential Analysis
Exploring advanced topics within sequential analysis opens up a deeper understanding of its applications and methodologies. Here we delve into Bayesian sequential analysis, hypothesis testing, changepoint detection, and compare trial sequential analysis with group sequential analysis.
Bayesian Sequential Analysis Explained
Bayesian Sequential Analysis is a statistical method that combines Bayesian inference with sequential analysis for real-time data examination. It updates the probability estimates for a hypothesis as more evidence becomes available.
Unlike traditional methods that require a fixed sample size determined in advance, Bayesian sequential analysis allows for continuous learning and decision-making. This approach is invaluable in areas like online learning algorithms and adaptive testing, where immediate reactions to new information are crucial.One of the advantages of Bayesian sequential analysis is its flexibility in accumulating evidence and making informed decisions without the need for large sample sizes.
Example: Consider a digital marketing campaign where the effectiveness of two advertisement designs is being tested. Using Bayesian sequential analysis, the campaign manager can start with a prior belief about which design is more effective and update this belief as conversion data comes in. The analysis might utilise the formula: \[ P(H|E) = \frac{P(E|H) \cdot P(H)}{P(E)} \] where \(P(H|E)\) is the probability of the hypothesis given the evidence, \(P(E|H)\) is the likelihood of the evidence given the hypothesis, \(P(H)\) is the initial hypothesis probability, and \(P(E)\) is the probability of the evidence.
Sequential Analysis Hypothesis Testing and Changepoint Detection
Sequential analysis hypothesis testing and changepoint detection form the cornerstones of identifying shifts or trends within data streams in real-time. Hypothesis testing in this context involves determining if observed data contradicts a null hypothesis, whereas changepoint detection focuses on identifying points where the statistical properties of a sequence change.The ability to detect changepoints accurately is essential in fields like finance, where sudden market shifts can occur, and timely detection can lead to significant advantages.
Example: In the stock market, a changepoint might signify a sudden change in a stock's return volatility. Using sequential analysis, an algorithm can detect this change by comparing historical data points to current observations, potentially indicating a buy or sell decision using statistical models such as the CUSUM (Cumulative Sum) method.
One common challenge in changepoint detection is distinguishing true changes from random fluctuations, which requires careful consideration of the model and threshold settings.
Trial Sequential Analysis and Group Sequential Analysis: A Comparison
Trial Sequential Analysis (TSA) and Group Sequential Analysis (GSA) are both designed to address interim analyses in clinical trials but with distinct focuses. TSA emphasises the accumulation of evidence to a predetermined threshold to avoid type I and II errors, whereas GSA allows for multiple looks at the data with adjustments made for the increased risk of errors.Both methodologies offer frameworks for making early decisions in clinical trials, but their application can lead to different consequences on the trial's design and conclusion.
- Trial Sequential Analysis (TSA): A technique that applies a cumulative meta-analysis framework to interim data in clinical trials, aiming to conclude as soon as sufficient evidence is available.
- Group Sequential Analysis (GSA): A method allowing for several interim analyses during a clinical trial, with stringent stopping rules to control the overall error rate.
The choice between TSA and GSA can greatly influence the progression of a clinical trial. TSA is particularly useful for meta-analyses where researchers wish to aggregate evidence over time from multiple studies. In contrast, GSA is pivotal in large-scale clinical trials, where early stopping for efficacy or harm can save costs and protect patients.Understanding the theoretical underpinnings and practical applications of TSA and GSA requires familiarity with statistical power and error rates, showcasing the complexity of making informed decisions in the midst of uncertain and accumulating data.
Implementing Sequential Analysis in Studies
Implementing sequential analysis in research offers a responsive approach to data collection and analysis, enabling researchers to make timely decisions based on the data as it accumulates. This method differs significantly from traditional fixed-sample analysis by introducing flexibility and potential efficiency in the decision-making process.Here, we provide a comprehensive guide to conducting sequential analysis, understanding the role of specialised software, and overcoming common challenges.
Step-by-Step Guide to Conducting Sequential Analysis
Conducting sequential analysis involves several key steps, from planning to execution. Here’s a streamlined guide to get started:
- Define your research question and objectives clearly.
- Determine the sequential design appropriate for your study, including decision points.
- Collect preliminary data to establish baselines and initial hypotheses.
- Continuously collect and analyse data at predetermined intervals.
- Use statistical criteria to make interim decisions about continuing the study, adjusting it, or stopping it early.
- Finalise your analysis once the study concludes or the decision criteria are met.
The choice of statistical criteria in step 5 often depends on the specific objectives of the study and the nature of the data being collected.
The Role of Software in Sequential Analysis
The complexity and real-time nature of sequential analysis necessitate the use of specialised software. These tools manage continuous data collection, apply statistical models, and facilitate timely decision-making.Software solutions designed for sequential analysis often feature capabilities for:
- Audio and visual alerts for when data reaches critical thresholds.
- Automated data visualisation for ongoing monitoring of trends.
- Integration with data collection platforms for seamless analysis.
- Customisable statistical models based on the specific needs of a study.
Some popular software packages for sequential analysis include R, with its ‘Sequential’ package, and SAS, offering procedures tailored for this analytical approach.
Overcoming Challenges in Sequential Analysis
While sequential analysis brings flexibility and potential efficiency to research, it also presents unique challenges:
- Data Management: Handling continuously growing datasets requires robust data management strategies to ensure integrity and relevancy.
- Statistical Complexity: Designing and interpreting sequential models demand a deep understanding of statistical methodologies.
- Decision Fatigue: Frequent interim analysis may lead to decision fatigue, impacting the objectivity of researchers.
- Resource Constraints: Continuous monitoring and analysis necessitate significant resources in terms of time and computational power.
One of the critical aspects of overcoming challenges in sequential analysis is enhancing the statistical competency of the research team. This involves not only understanding complex statistical models but also being adept at interpreting and reacting to real-time data. Workshops, training sessions, and collaboration with statisticians can provide valuable insights into managing the intricacies of sequential analysis. Furthermore, embracing a dynamic approach to data management, where datasets are continuously evaluated for relevance and integrity, can significantly mitigate the risks associated with large and complex data streams.
Sequential Analysis - Key takeaways
- Sequential Analysis Definition: A statistical method that evaluates data as it is collected, allowing for conclusions and decisions before the full dataset is gathered, providing a flexible and efficient alternative to traditional fixed-sample size analysis.
- Sequential Analysis Example: In medical trials, it can be used to make early decisions about a treatment's effectiveness, possibly ending a trial early to expedite patient access to beneficial treatments or to prevent further harm.
- Bayesian Sequential Analysis: This method combines Bayesian inference with sequential analysis, updating probability estimates for hypotheses as new evidence becomes available, and is useful for continuous learning and decision-making.
- Sequential Analysis in Hypothesis Testing and Changepoint Detection: Essential for identifying real-time shifts or trends in data streams, allowing for timely decisions in dynamic fields such as finance or cybersecurity.
- Trial Sequential Analysis (TSA) vs. Group Sequential Analysis (GSA): TSA accumulates evidence to a predetermined threshold, while GSA involves multiple interim analyses with adjustments for error rates, both useful in clinical trial designs for early decision making.
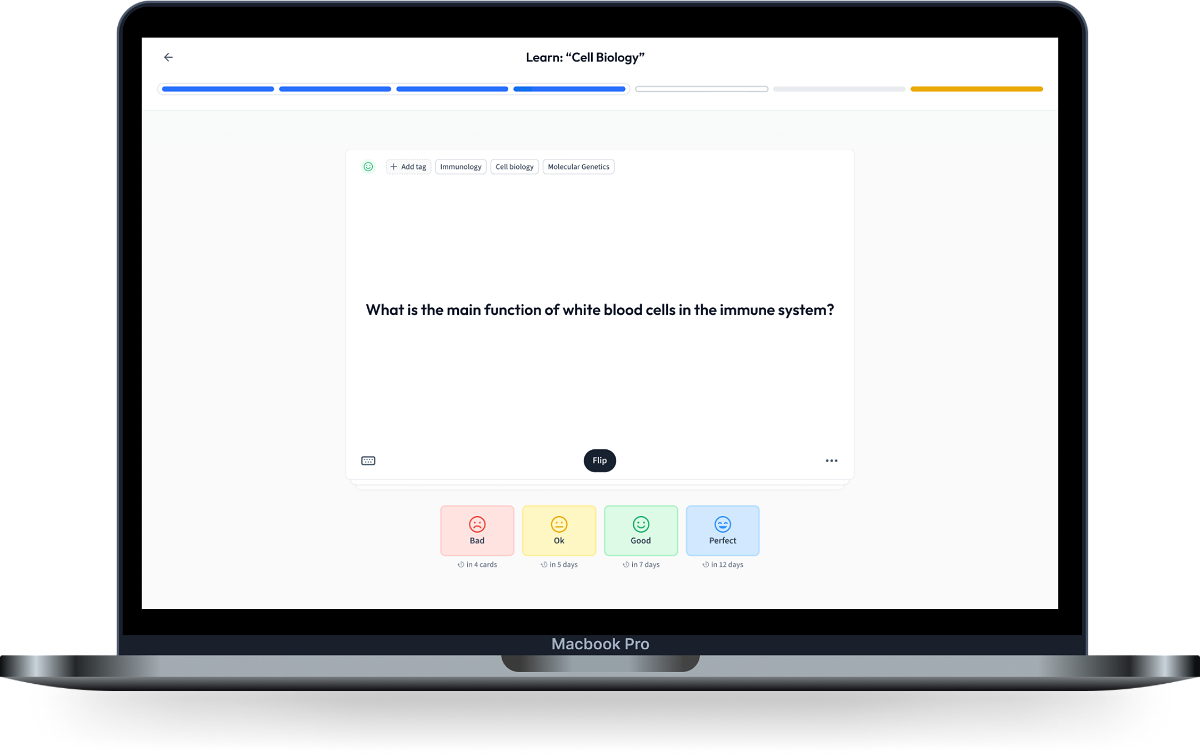
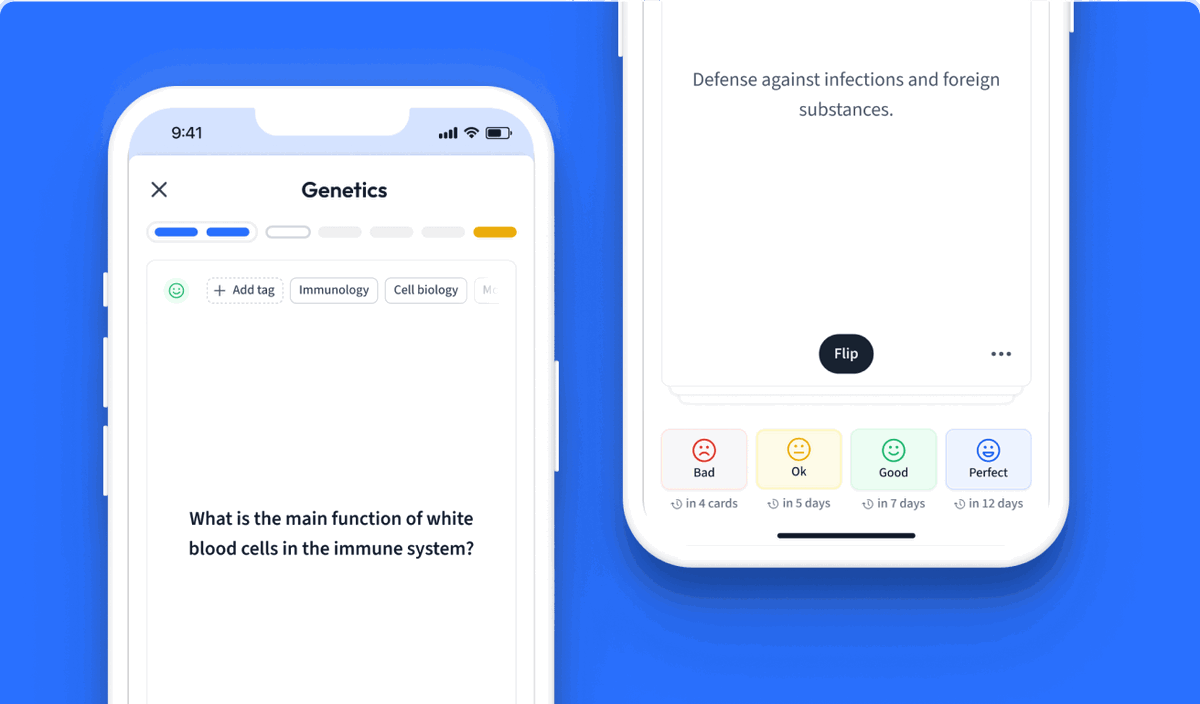
Learn with 0 Sequential Analysis flashcards in the free StudySmarter app
We have 14,000 flashcards about Dynamic Landscapes.
Already have an account? Log in
Frequently Asked Questions about Sequential Analysis
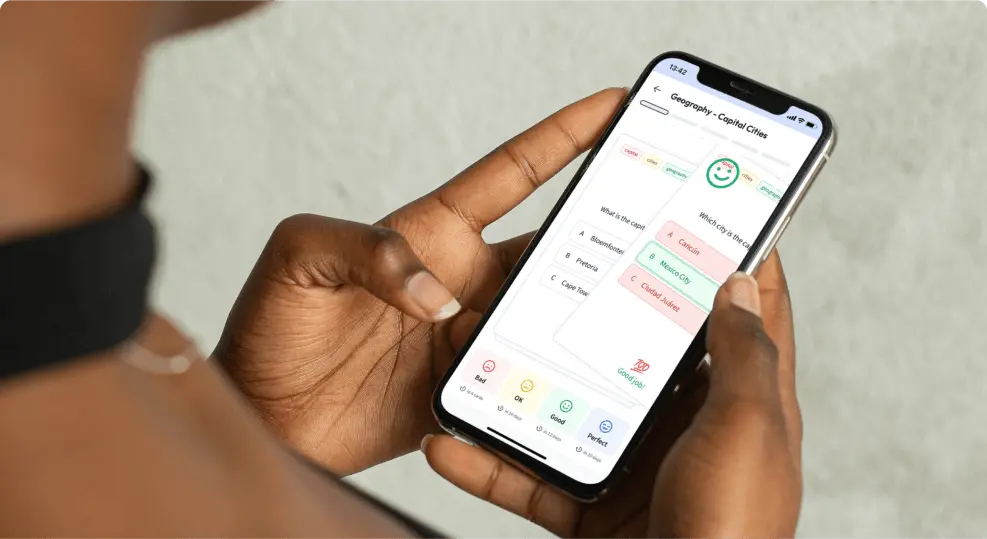
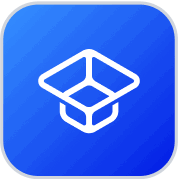
About StudySmarter
StudySmarter is a globally recognized educational technology company, offering a holistic learning platform designed for students of all ages and educational levels. Our platform provides learning support for a wide range of subjects, including STEM, Social Sciences, and Languages and also helps students to successfully master various tests and exams worldwide, such as GCSE, A Level, SAT, ACT, Abitur, and more. We offer an extensive library of learning materials, including interactive flashcards, comprehensive textbook solutions, and detailed explanations. The cutting-edge technology and tools we provide help students create their own learning materials. StudySmarter’s content is not only expert-verified but also regularly updated to ensure accuracy and relevance.
Learn more