Jump to a key chapter
What is Structural Equation Modelling?
Structural Equation Modelling (SEM) is a comprehensive statistical approach used to test hypotheses about relationships among observed and latent variables. It integrates elements of multiple regression analysis, factor analysis, and path analysis to examine complex models of cause and effect or latent structures in data. In essence, SEM allows researchers to untangle and understand the intricate web of relationships that exist in real-world data, capturing both direct and indirect effects.This mathematical modelling technique is pivotal in various fields, including psychology, education, economics, and social sciences, enabling researchers to validate theoretical models with empirical data.
Structural Equation Modelling Definition
Structural Equation Modelling (SEM) is a statistical methodology that enables the construction and testing of complex models by considering multiple equations simultaneously. It typically involves two major components: the measurement model, which defines how latent variables are indicated by observed variables; and the structural model, which specifies the hypothesized relationships between the latent variables.
A key feature of SEM is its ability to handle latent variables, which are not directly observable but inferred from the observable (measured) variables. This is particularly useful in social sciences where many concepts of interest, like socioeconomic status or intelligence, cannot be directly measured.By incorporating latent variables, SEM provides a more nuanced and accurate depiction of the relationships between variables, going beyond what is possible with more traditional statistical techniques.
Principles and Practice of Structural Equation Modelling
The practice of Structural Equation Modelling follows a systematic approach, starting from the conceptualisation of the model based on theoretical or empirical grounds to the specification, estimation, evaluation, and finally, the modification of the model as required. SEM allows for both confirmatory and exploratory analysis, making it a versatile tool in research. The general steps in SEM include:
- Model Specification: Defining the structure of the model based on theory or previous research.
- Model Identification: Ensuring the model is mathematically solvable.
- Model Estimation: Calculating estimates of the free parameters in the model.
- Model Testing: Assessing the fit of the model to the data.
- Model Modification: Adjusting the model to improve fit based on statistical and substantive considerations.
Latent variables are unobservable variables that are estimated from observed data, often representing abstract concepts like attitudes or concepts in social science research.
While SEM is powerful in its ability to model complex relationships, it relies heavily on the quality and suitability of the data. Missing data, measurement errors, and sample size can greatly influence the results. Therefore, rigorous pre-analysis planning, including thorough variable selection and measurement, is crucial for the success of an SEM analysis.Another important aspect to consider is the model's identification status. An identified model has a unique solution that can be estimated from the data, whereas an unidentifiable model does not. This means that careful consideration must be given to the model's structure and the data used to ensure that meaningful and accurate conclusions can be drawn.
Example of using SEM in research: A study on employee motivation and productivity might conceptualise motivation as a latent variable, indicated by observed variables such as job satisfaction, organisational commitment, and work engagement. The structural model could then hypothesise the direct effects of motivation on productivity, as well as potential indirect effects through variables like job satisfaction.This approach allows the study to capture the complex, multifaceted nature of motivation and its impact on productivity, providing insights that would not be accessible through simpler analysis techniques.
SEM software packages, such as AMOS, LISREL, and Mplus, offer a range of tools for modelling and estimation, making SEM accessible even to those with limited statistical background.
Understanding Structural Equation Modelling Formula
Structural Equation Modelling (SEM) is deeply rooted in its unique formula, which sets it apart from other statistical methods. At its core, SEM operates through a series of mathematical equations designed to represent the relationships between variables in a model, both observed and latent.The SEM formula encompasses a combination of paths that describe the direct and indirect relationships among variables. This integration of multiple regression paths into a cohesive model allows for a comprehensive analysis of complex data structures, making SEM an invaluable tool in research across various disciplines.
Key Components of SEM Formula
The SEM formula is comprised of two primary components:
- Measurement Model: This part of the equation captures the relationship between latent variables and their indicators. It's represented by the equation \(\lambda\), which stands for the loadings of observed variables on latent variables.
- Structural Model: This component describes the relationships between the latent variables themselves. The path coefficients in the model, denoted by \(\beta\) and \(\gamma\), illustrate these relationships.
Latent variables are variables that are not directly observable but are inferred from other variables that are measurable. These often represent constructs like intelligence, satisfaction, or socio-economic status.
Example: In a study exploring the impact of training on employee productivity, the measurement model could involve latent variables such as 'Employee Competence' and 'Job Satisfaction'. Here, 'Employee Competence' might be indicated by test scores and performance evaluations, whereas 'Job Satisfaction' could be indicated by survey responses. The structural model would then assess how 'Employee Competence' directly influences 'Job Satisfaction' and 'Employee Productivity', alongside examining direct effects from 'Training' on these latent variables.
Applying the Structural Equation Modelling Formula
Application of the SEM formula begins with the specification of the model based on theoretical or empirical foundations. Once specified, the model is then estimated using statistical software capable of handling SEM, such as AMOS, LISREL, or Mplus.The process involves:
- Identifying observed and latent variables relevant to the research question.
- Specifying the relationships between these variables within the SEM framework.
- Estimating the model to assess the strength and significance of the proposed relationships.
- Interpreting the results to infer conclusions about the underlying theory or hypothesis.
The power of SEM lies not only in its ability to model complex relationships but also in its flexibility regarding data types. SEM can handle a variety of data types, including continuous and categorical variables. This versatility makes it particularly appealing for investigating multifaceted phenomena.Furthermore, advancements in SEM techniques, such as the integration of Bayesian methods, have broadened its applicability, allowing researchers to incorporate prior information into their models and to handle data with more complex structures.
Remember, a good SEM analysis goes beyond just fitting the model to data. It includes a thoughtful consideration of model adequacy, potential limitations, and the implications of the findings in relation to theoretical and practical contexts.
Practical Applications: Structural Equation Modelling Examples
Structural Equation Modelling (SEM) is a versatile and powerful statistical methodology widely applied across various disciplines to understand complex relationships between observed and latent variables. Through its ability to model intricate pathways, SEM provides invaluable insights into the dynamics of different phenomena, bridging the gap between theory and empirical evidence.In the following sections, you'll explore a range of practical applications of SEM through case studies and real-world examples, illustrating the depth and breadth of its utility in research.
Case Studies: Structural Equation Modelling in Action
Case studies underscore the practical application of Structural Equation Modelling in various fields, shedding light on its capacity to unravel complex constructs and relationships. These encapsulate diverse scenarios where SEM's robust analytical capabilities are deployed, demonstrating both the methodology's versatility and its impact on advancing knowledge.From assessing psychological constructs to evaluating marketing strategies and beyond, these case studies exemplify how SEM facilitates a deeper understanding of the underlying dynamics in different research contexts.
Example: One notable case study involves assessing the impact of organisational culture on employee performance within the corporate sector. Researchers conceptualised organisational culture as a latent variable, indicated by factors such as leadership style, communication patterns, and teamwork.The study employed SEM to test a hypothetical model where organisational culture influences employee performance both directly and indirectly through employee satisfaction. The findings revealed significant pathways, providing empirical evidence to support the hypothesis and offering valuable insights for organisational development strategies.
Real-World Examples and Insights
Real-world examples further demonstrate Structural Equation Modelling's application across a wide array of sectors, from education and healthcare to finance and environmental studies. These examples not only highlight SEM's adaptability but also its role in addressing complex questions, offering insights that inform policy, practice, and further research.Below are insights from different sectors, illustrating how SEM contributes to understanding and solving pertinent issues.
Example: In the field of education, SEM has been used to explore factors affecting students' academic achievement. A study focused on the relationship between students' self-efficacy, motivation, learning strategies, and academic performance. Using SEM, it was possible to identify both the direct effects of these variables on academic achievement and the indirect effects mediated by motivation and learning strategies.This analysis provided a comprehensive understanding of the factors contributing to students' success, thereby informing targeted interventions to enhance educational outcomes.
An exploration into the application of SEM in environmental studies reveals its potential to tackle issues of sustainability and climate change. For instance, researchers have used SEM to model the complex interplay between human behaviours, environmental policies, and their impacts on climate change mitigation efforts.This approach allowed for assessing the direct effects of policies on sustainable behaviours, as well as the indirect effects mediated through public awareness and attitudes towards the environment. Such studies serve as a testament to SEM's utility in fostering a nuanced understanding of multifactorial issues, guiding more effective policy and community engagement strategies.
It is noteworthy that SEM's efficacy hinges on the quality of the model specification and the data. An accurately specified model that truly represents the theoretical constructs and their relationships is critical for deriving valid and reliable insights from SEM analysis.
Advanced Techniques in Structural Equation Modelling
Structural Equation Modelling (SEM) encompasses a broad spectrum of statistical techniques, each offering unique insights into data analysis. Among the various methodologies, some advanced techniques have emerged that provide sophisticated approaches to modelling complex relationships between observed and latent variables.These advanced techniques facilitate a deeper understanding of data structures, enabling researchers to uncover nuanced patterns and infer causal relationships with greater accuracy. In this section, you'll learn about two of these methods: Partial Least Squares Structural Equation Modelling and implementing SEM in R.
Partial Least Square Structural Equation Modelling
Partial Least Squares Structural Equation Modelling (PLS-SEM) is a variance-based SEM technique that is particularly useful when the goal is to predict constructs or identify key driver constructs within a model. Unlike covariance-based SEM, PLS-SEM focuses on maximizing the explained variance of the dependent latent variables.PLS-SEM is advantageous in situations with complex models, small sample sizes, or when the data does not meet the normality assumption. This method is highly appreciated in exploratory research where the primary interest lies in theory building rather than theory testing.
Example: Consider a research model exploring the impact of e-service quality on customer satisfaction and loyalty in online retailing. PLS-SEM can be used to analyse the direct effects of e-service quality on customer loyalty and the mediating role of customer satisfaction. In this context, e-service quality, customer satisfaction, and loyalty are modelled as latent variables.Through PLS-SEM, researchers can estimate the model parameters efficiently, even with a relatively small sample size, and predict the significant drivers affecting customer loyalty in the online retail sector.
One of the key benefits of PLS-SEM is its ability to handle highly complex models with many indicators and constructs, making it a go-to method for exploratory studies.
Implementing Structural Equation Modelling in R
R, a versatile programming language for statistical computing, provides a powerful environment for implementing Structural Equation Modelling. With an assortment of packages such as lavaan, R makes it convenient for researchers to perform SEM analyses, from simple path models to more sophisticated latent variable models.Implementing SEM in R involves several steps: specifying the model, estimating the parameters, assessing the model fit, and interpreting the results. Thanks to R's comprehensive functionalities, even users with limited programming skills can successfully execute SEM analyses.
Here is a step-by-step guide to implementing SEM in R using the lavaan package:
- Install and load the lavaan package.
- Specify the SEM model in lavaan's model syntax.
- Estimate the model using the sem() function.
- Assess model fit with various fit indices available in lavaan.
- Interpret the parameter estimates and model fit indices to derive conclusions.
Example: Suppose you're analysing the relationship between academic motivation, study habits, and academic performance among university students. Using R and the lavaan package, you can specify a model that captures these relationships, estimate the model's parameters, and evaluate how well the model fits your data.This flexibility and power make R an ideal tool for conducting SEM studies, providing researchers with a deeper insight into their data's underlying patterns.
R and its SEM packages are constantly evolving, with an active community contributing to its development. Staying updated on the latest packages and functionalities can greatly enrich your SEM analyses.
Structural Equation Modeling - Key takeaways
- Structural Equation Modelling (SEM) definition: A statistical approach integrating multiple regression, factor analysis, and path analysis for testing hypotheses about relationships among observed and latent variables.
- SEM formula components: Measurement Model (relationship between latent variables and indicators) represented by \\(\lambda\\) and Structural Model (relationships among latent variables) with path coefficients \\(\beta\\) and \\(\gamma\\).
- SEM principles and practice include model specification, identification, estimation, testing, and potential modification, encompassing confirmatory and exploratory analysis.
- Partial Least Square Structural Equation Modelling (PLS-SEM): A variance-based method suitable for prediction, small sample sizes, or non-normal data distribution, emphasising exploratory research.
- SEM in R: Using packages like lavaan, researchers specify, estimate, and interpret SEM models even with limited programming background.
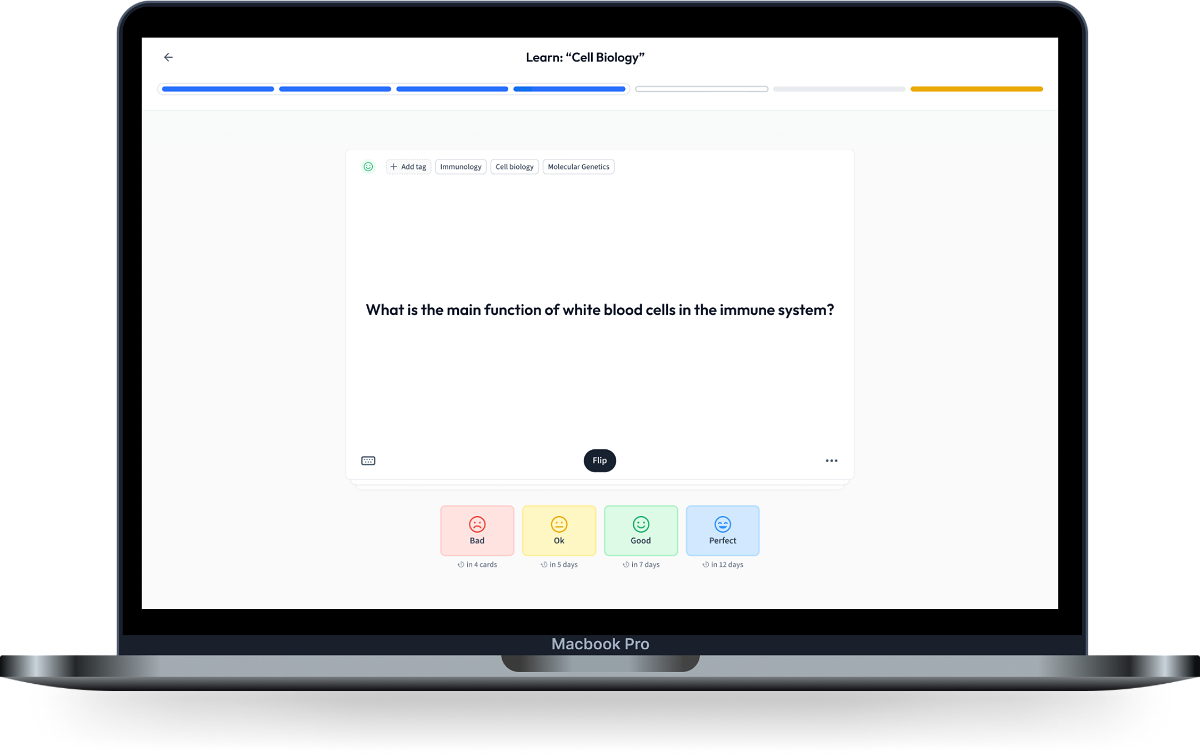
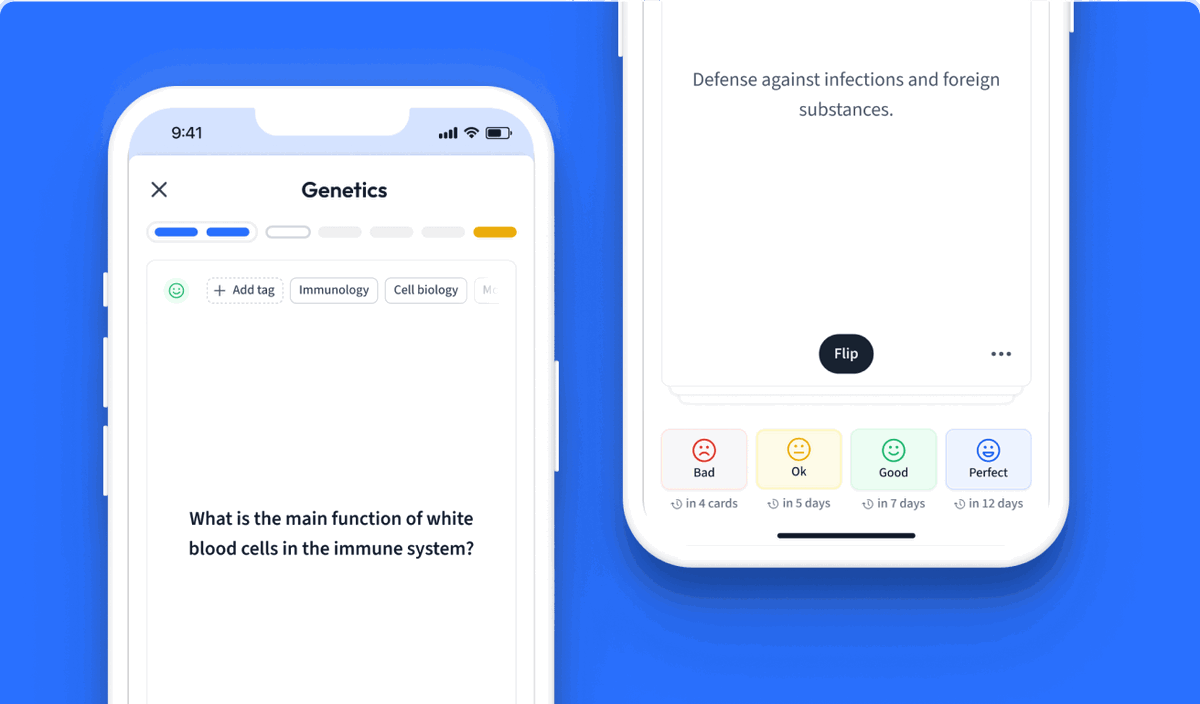
Learn with 0 Structural Equation Modeling flashcards in the free StudySmarter app
We have 14,000 flashcards about Dynamic Landscapes.
Already have an account? Log in
Frequently Asked Questions about Structural Equation Modeling
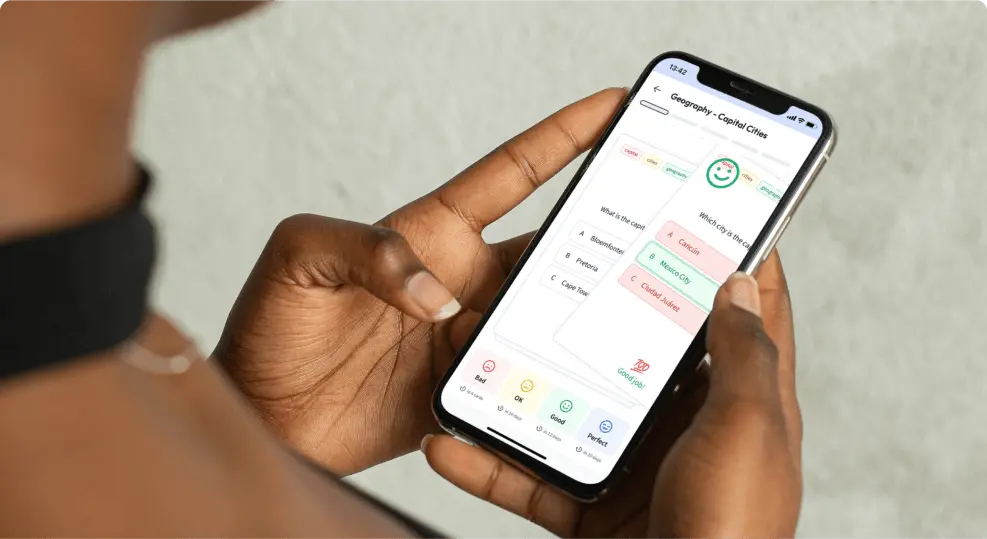
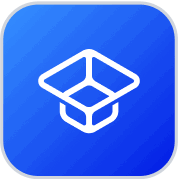
About StudySmarter
StudySmarter is a globally recognized educational technology company, offering a holistic learning platform designed for students of all ages and educational levels. Our platform provides learning support for a wide range of subjects, including STEM, Social Sciences, and Languages and also helps students to successfully master various tests and exams worldwide, such as GCSE, A Level, SAT, ACT, Abitur, and more. We offer an extensive library of learning materials, including interactive flashcards, comprehensive textbook solutions, and detailed explanations. The cutting-edge technology and tools we provide help students create their own learning materials. StudySmarter’s content is not only expert-verified but also regularly updated to ensure accuracy and relevance.
Learn more