Jump to a key chapter
Suppose there is a legal trial, it is commonplace to assume someone is innocent unless there is enough evidence to suggest that they are guilty. After the trial, the judge finds the defendant guilty but it turns out that the defendant was not guilty. This is an example of a Type I error.
Definition of a Type I Error
Suppose you have carried out a hypothesis test that leads to the rejection of the null hypothesis \(H_0\). If it turns out that in fact the null hypothesis is true then you have committed a Type I error. Now suppose you have carried out a hypothesis test and accepted the null hypothesis but in fact the \(H_0\) is false, then you have committed a Type II error. A good way to remember this is by the following table:
\(H_0\) true | \(H_0\) false | |
Reject \(H_0\) | Type I error | No error |
Do not reject \(H_0\) | No error | Type II error |
A Type I error is when you have rejected \(H_0\) when \(H_0\) is true.
However there is another way to think about Type I errors.
A Type I Error is a False Positive
Type I errors are also known as false positives. This is because rejecting \(H_0\) when \(H_0\) is true implies that the statistician has falsely concluded that there is statistical significance in the test when there was not. A real world example of a false positive is when a fire alarm goes off when there is no fire or when you have been falsely diagnosed with a disease or illness. As you can imagine, false positives can lead to significant misinformation especially in the case of medical research. For example, when testing for COVID-19, the chance of testing positive when you don't have COVID-19 was estimated at being around \(2.3\%\). These false positives can lead to overestimation of the impact of the virus leading to a waste of resources.
Knowing that Type I errors are false positives is a good way to remembering the difference between Type I errors and Type II errors, which are referred to as false negatives.
Type I Errors and Alpha
A Type I error occurs when the null hypothesis is rejected when it is in fact true. The probability of a Type I error is commonly denoted by \(\alpha\) and this is known as the size of the test.
The size of a test, \(\alpha\), is the probability of rejecting the null hypothesis, \(H_0\), when the \(H_0\) is true and this is equal to the probability of a Type I error.
The size of a test is the significance level of the test and this is chosen before the test is carried out. The Type 1 errors have a probability of \(\alpha\) which correlates to the confidence level the statistician will set when performing the hypothesis test.
For example, if a statistician sets a confidence level of \(99\%\) then there is a \(1\%\) chance or a probability of \(\alpha=0.01\) that you will get a Type 1 error. Other common choices for \(\alpha\) are \(0.05\) and \(0.1\). Therefore, you can decrease the probability of a Type I error by decreasing the significance level of the test.
The Probability of a Type I Error
You can calculate the probability of a Type I error occurring by looking at the critical region or the significance level. The critical region of a test is determined such that it keeps the probability of a Type I error less than of equal to the significance level \(\alpha\).
There is an important distinction between continuous and discrete random variables to be made when looking at the probability of a Type I occurring. When looking at discrete random variables, the probability of a Type I error is the actual significance level, whereas when the random variable in question is continuous, the probability of a Type I error is equal to the significance level of the test.
To find the probability of a Type 1 error:
\[\begin{align} \mathbb{P}(\text{Type I error})&=\mathbb{P}(\text{rejecting } H_0 \text{ when }H_0 \text{ is true}) \\ &=\mathbb{P}(\text{being in the critical region}) \end{align}\]
For discrete random variables:
\[\mathbb{P}(\text{Type I error})\leq \alpha.\]
For continuous random variables:
\[\mathbb{P}(\text{Type I error})= \alpha.\]
Discrete Examples of Type I Errors
So how do you find the probability of a Type I error if you have a discrete random variable?
The random variable \(X\) is binomially distributed. Suppose a sample of 10 is taken and a statistician wants to test the null hypothesis \(H_0: \; p=0.45\) against the alternative hypothesis \(H_1:\; p\neq0.45\).
a) Find the critical region for this test.
b) State the probability of a Type I error for this test.
Solution:
a) Since this is a two tailed test, at a \(5\%\) significance level, the critical values, \(c_1\) and \(c_2\) are such that
\[\begin{align} \mathbb{P}(X\leq c_1) &\leq0.025 \\ \text{ and } \mathbb{P}(X\geq c_2) &\leq 0.025. \end{align}\]
\(\mathbb{P}(X\geq c_2) = 1-\mathbb{P}(X\leq c_2-1)\leq0.025\) or \( \mathbb{P}(X\leq c_2-1) \geq0.975\)
Assume \(H_0\) is true. Then under the null-hypothesis \(X\sim B(10,0.45)\), from the statistical tables:
\[ \begin{align} &\mathbb{P}(X \leq 1)=0.0233<0.025 \\ & \mathbb{P}(X \leq 2)=0.0996>0.025.\end{align}\]
Therefore the critical value is \(c_1=1\). For the second critical value,
\[ \begin{align} &\mathbb{P}(X \leq 7)=0.9726<0.975 \\ & \mathbb{P}(X \leq 8)=0.996>0.975. \end{align}\]
Therefore \(c_2-1=8\) so the critical value is \(c_2=9\).
So the critical region for this test under a \(5\%\) significance level is
\[\left\{ X\leq 1\right\}\cup \left\{ X\geq 9\right\}.\]
b) A Type I error occurs when you reject \(H_0\) but \(H_0\) is true, i.e. it is the probability you are in the critical region given that the null hypothesis is true.
Under the null hypothesis, \(p=0.45\), therefore,
\[\begin{align} \mathbb{P}(\text{Type I error})&=\mathbb{P}(X\leq1 \mid p=0.45)+\mathbb{P}(X\geq9 \mid p=0.45) \\ &=0.0233+1-0.996 \\ &=0.0273. \end{align}\]
Let's take a look at another example.
A coin is tossed until a tail is obtained.
a) Using a suitable distribution, find the critical region for a hypothesis test that tests whether the coin is biased towards heads at the \(5\%\) significance level.
b) State the probability of a Type I error for this test.
Solution:
a) Let \(X\) be the number of coin tosses before a tail is obtained.
Then this can be answered using the geometric distribution as follows since the number of failures (heads) \(k - 1\) before the first success/tail with a probability of a tail given by \(p\).
Therefore, \(X\sim \rm{Geo}(p)\) where \(p\) is the probability of a tail being obtained. Therefore the null and alternative hypothesis are
\[ \begin{align} &H_0: \; p=\frac{1}{2} \\ \text{and } &H_1: \; p<\frac{1}{2}. \end{align}\]
Here the alternative hypothesis is the one that you want to establish, i.e. that the coin is biased towards heads, and the null hypothesis is the negation of that, i.e. the coin is not biased.
Under the null hypothesis \(X\sim \rm{Geo} \left(\frac{1}{2}\right)\).
Since you are dealing with a one-tailed test at the \(5\%\) significance level, you want to find the critical value \(c\) such that \(\mathbb{P}(X\geq c) \leq 0.05 \). This means you want
\[ \left(\frac{1}{2}\right)^{c-1} \leq 0.05. \]
Therefore
\[ (c-1)\ln\left(\frac{1}{2}\right) \leq \ln(0.05), \]
which means \(c >5.3219\).
Therefore, the critical region for this test is \(X \geq 5.3219=6\).
Here you have used the fact that, for a geometric distribution \(X\sim \rm{Geo}(p)\),
\[\mathbb{P}(X \geq x)=(1-p)^{x-1}.\]
b) Since \(X\) is a discrete random variable, \(\mathbb{P}(\text{Type I error})\leq \alpha\), and the probability of a Type I error is the actual significance level. So
\[\begin{align} \mathbb{P}(\text{Type I error})&= \mathbb{P}( \text{rejecting } H_0 \text{ when } H_0 \text{ is true}) \\ &=\mathbb{P}(X\geq 6 \mid p=0.5) \\ &= \left(\frac{1}{2}\right)^{6-1} \\ &=0.03125. \end{align}\]
Continuous Examples of a Type I Error
In the continuous case, when finding the probability of a Type I error, you will simply need to give the significance level of the test given in the question.
The random variable \(X\) is normally distributed such that \(X\sim N(\mu ,4)\). Suppose a random sample of \(16\) observations is taken and \(\bar{X}\) the test statistic. A statistician wants to test \(H_0:\mu=30\) against \(H_1:\mu<30\) using a \(5\%\) significance level.
a) Find the critical region.
b) State the probability of a Type I error.
Solution:
a) Under the null hypothesis you have \(\bar{X}\sim N(30,\frac{4}{16})\).
Define
\[Z=\frac{\bar{X}-\mu}{\frac{\mu}{\sqrt{n}}}\sim N(0,1).\]
At the \(5\%\) significance level for a one-sided test, from the statistical tables, the critical region for \(Z\) is \(Z<-1.6449\).
Therefore, you reject \(H_0\) if
\[\begin{align} \frac{\bar{X}-\mu}{\frac{\mu}{\sqrt{n}}}&=\frac{\bar{X}-30}{\frac{2}{\sqrt{16}}} \\ &\leq -1.6449.\end{align}\]
Therefore, with some rearranging, the critical region for \(\bar{X}\) is given by \(\bar{X} \leq 29.1776\).
b) Since \(X\) is a continuous random variable, there is no difference between the target significance level and the actual significance level. Therefore, \(\mathbb{P}(\text{Type I error})= \alpha\) i.e. the probability of a Type I error \(\alpha\) is the same as the significance level of the test, so
\[\mathbb{P}(\text{Type I error})=0.05.\]
Relationship between Type I and Type II Errors
The relationship between the probabilities of Type I and Type II errors is important in hypothesis testing as statisticians want to minimise both. Yet to minimise the probability of one, you increase the probability of the other.
For example, if you reduce the probability of Type II error (the probability of not rejecting the null hypothesis when it is false) by decreasing the significance level of a test, doing this increases the probability of a Type I error. This trade-off phenomenon is often dealt with by prioritising the minimisation of the probability of Type I errors.
For more information on Type II errors check out our article on Type II Errors.
Type I Errors - Key takeaways
- A Type I error occurs when you have rejected \(H_0\) when \(H_0\) is true.
- Type I errors are also known as false positives.
- The size of a test, \(\alpha\), is the probability of rejecting the null hypothesis, \(H_0\), when the \(H_0\) is true and this is equal to the probability of a Type I error.
- You can decrease the probability of a Type I error by decreasing the significance level of the test.
- There is a trade-off between Type I and Type II errors since You cannot decrease the probability of a Type I error without increasing the probability of a Type II error, and vice versa.
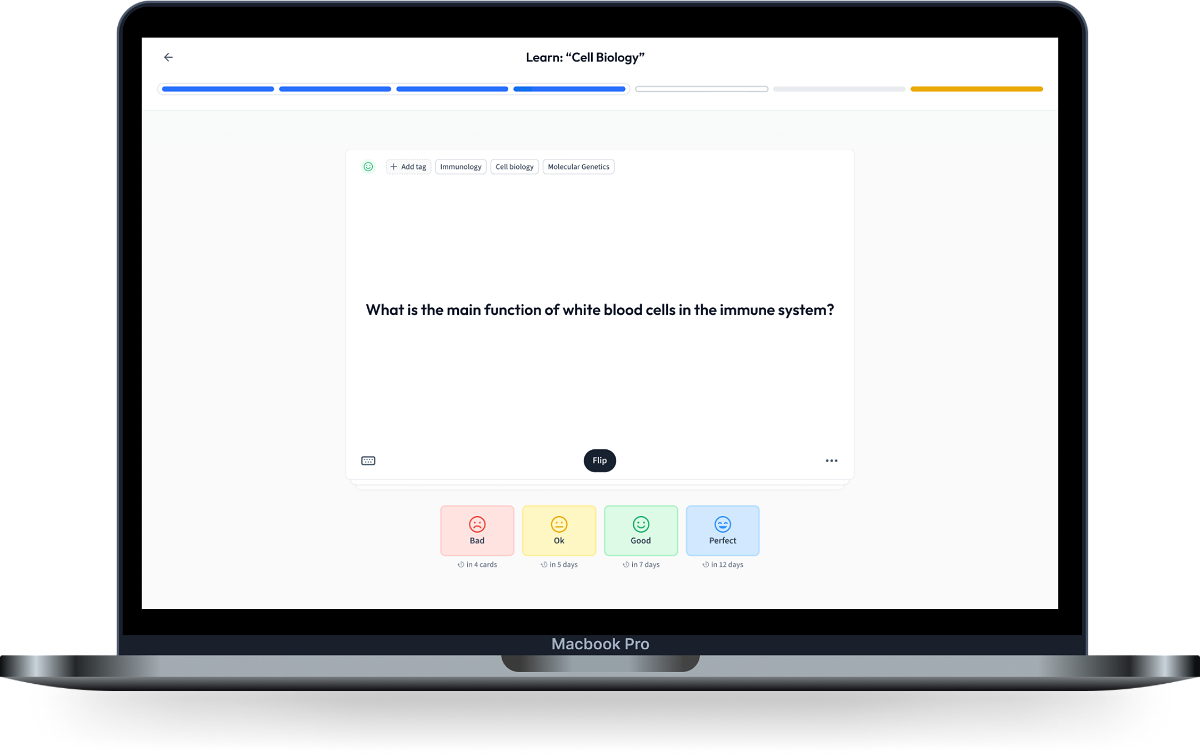
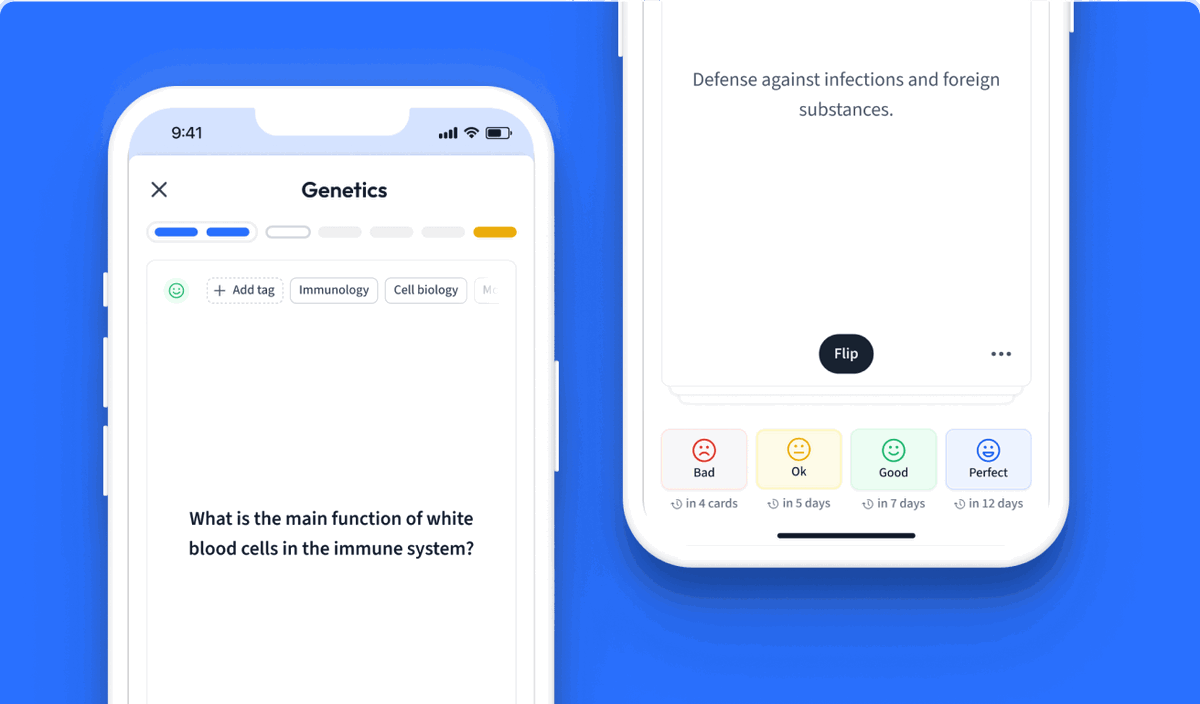
Learn with 3 Type I Error flashcards in the free StudySmarter app
We have 14,000 flashcards about Dynamic Landscapes.
Already have an account? Log in
Frequently Asked Questions about Type I Error
How to calculate type I error?
For continuous random variables, the probability of a type I error is the significance level of the test.
For discrete random variables, the probability of a type I error is the actual significance level, which is found by calculating the critical region then finding the probability that you are in the critical region.
What is a type I error?
A type I error is when you have rejected the null hypothesis when it is true.
What is an example of a Type I error?
An example of a type I error is when someone has tested positive for Covid-19 but they don't actually have Covid-19.
Which is worse type 1 or 2 error?
In most cases, Type 1 errors are seen as worse than Type 2 errors. This is because incorrectly rejecting the null hypothesis usually leads to more significant consequences.
Why are type I and type II errors important?
Type I and Type II errors are important because it means that an incorrect conclusion has been made in a hypothesis/statistical test. This can lead to issues such as false information or costly errors.
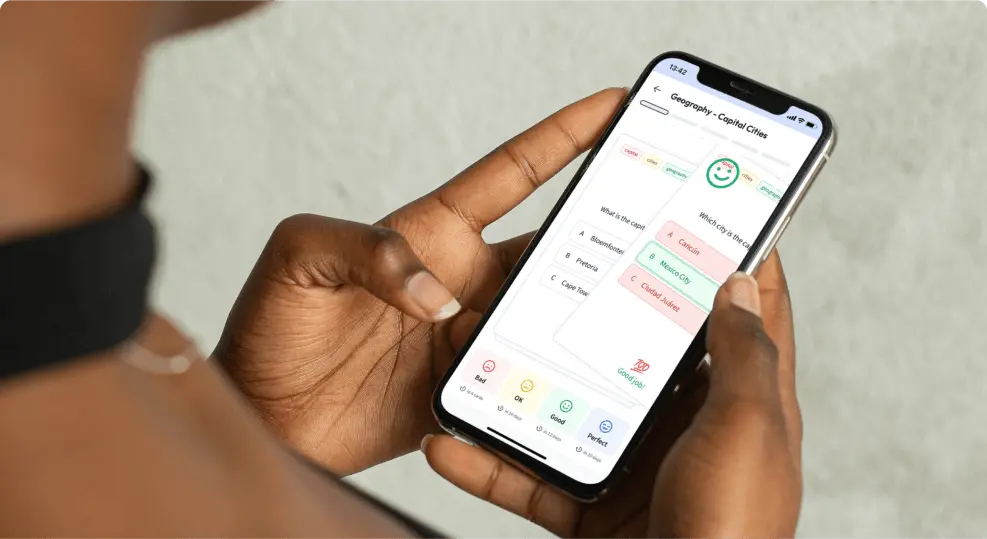
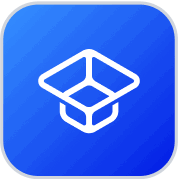
About StudySmarter
StudySmarter is a globally recognized educational technology company, offering a holistic learning platform designed for students of all ages and educational levels. Our platform provides learning support for a wide range of subjects, including STEM, Social Sciences, and Languages and also helps students to successfully master various tests and exams worldwide, such as GCSE, A Level, SAT, ACT, Abitur, and more. We offer an extensive library of learning materials, including interactive flashcards, comprehensive textbook solutions, and detailed explanations. The cutting-edge technology and tools we provide help students create their own learning materials. StudySmarter’s content is not only expert-verified but also regularly updated to ensure accuracy and relevance.
Learn more