Jump to a key chapter
What Are Zero-Inflated Models?
Zero-inflated models are powerful statistical tools used to analyse data sets that have an excess of zero values. They are especially useful in fields where occurrences of non-events are significant and need to be accurately represented in the data analysis.
Zero-Inflated Models Definition: Understanding the Basics
Zero-inflated models are a type of statistical model designed to handle data sets with a disproportionally high number of zero outcomes. These models are particularly suitable for count data, where the presence of 'zero-inflation' indicates that traditional modelling techniques might be inadequate.
Think of zero-inflated models as tailored suits, designed to perfectly fit datasets where zeros are more prevalent than any other count.
How Zero-Inflated Models Work: A Simple Explanation
At their core, zero-inflated models consist of two components: a binary model and a count model. The binary model, often a logistic regression, predicts the likelihood of an observation being a certain type of zero. The count model, frequently a Poisson or negative binomial regression, then analyses the count data for non-zero instances.The essence of zero-inflated models lies in their dual process. The initial stage predicts the occurrence of excess zeros, while the subsequent stage models the count data, taking into account the predictions from the first stage. This dual approach allows for a more nuanced understanding of the data, providing insights that could be missed with other models.
Example: Imagine a park where bird watchers record the number of a rare bird species seen each day. Many days might report zero sightings, not because the birds are absent but due to their rarity or the weather conditions. A zero-inflated model would first speculate whether the zero sightings were a result of genuine absence (true zeros) or missed sightings (excess zeros) and then analyse the count of actual sightings.
Zero-Inflated Models Example: Bringing Concepts to Life
To illustrate how zero-inflated models work, consider a data set from a local library's summer reading program. Here, the number of books read by participants might have a high incidence of zeros, as some registered participants might not read any books. Applying a zero-inflated model can help distinguish between those who did not participate (excess zeros) and those who participated but did not manage to read any books (true zeros).A zero-inflated model could discern between non-participation and lack of reading within the participant group, offering valuable insights for future program planning.
Types of Zero-Inflated Models
Zero-inflated models take on various forms, each suited to different kinds of data exhibiting an excess of zeros. These models are tailored to give the most accurate analysis and insights for count data that traditional models may poorly fit.
Zero Inflated Poisson Model Explained
The Zero Inflated Poisson (ZIP) model is a blend of a Poisson distribution model and a logistic model. It's designed for count data where the occurrence of zero is higher than what a standard Poisson distribution would predict. The model essentially operates in two stages: one part predicts whether an observation falls into the 'zero' category and the other predicts the count for non-zero observations using Poisson regression.One key assumption of the ZIP model is that the data can be split into two categories: 'structural zeros', which are true zeros, and 'sampling zeros', which occur due to the Poisson process.
Example: In traffic studies, the ZIP model helps analyse road sections with zero accidents. The zero observations might indicate either sections where accidents are impossible ('structural zeros') or where they are possible but didn't occur during the study period ('sampling zeros').
Understanding the Zero Inflated Binomial Model
The Zero Inflated Binomial (ZIB) model adapts the principles of the zero-inflated model to data that follows a binomial distribution. This model is useful when the data consists of the number of successes in a series of binary (yes/no) trials, and there's an excessive number of trials with zero successes. Similarly to the ZIP model, ZIB uses a logistic regression to model the binary outcome of having zero or more successes and a binomial regression for the count of successes.A ZIB model can account for the inflated number of zeros in the data, distinguishing between 'structural' zeros and zeros occurring by chance through the binomial process.
Remember, the difference between the Poisson and the Binomial models lies in the nature of the count data they address; while Poisson handles unrestricted counts, Binomial deals with counts out of a fixed number of trials.
Insights into the Zero Inflated Negative Binomial Model
The Zero Inflated Negative Binomial (ZINB) model is an extension of the binomial model tailored for count data that is over-dispersed; that is, the variance is greater than the mean. The Negative Binomial part of the model deals with the count data while the Zero Inflated part of the model handles the excess zeros. The ZINB model is particularly useful in cases where the data shows variance exceeding the mean, which cannot be adequately modelled by the Poisson or the binomial distributions alone.Like its counterparts, the ZINB model estimates the proportion of structural zeros and models the counts, adjusting for overdispersion, thus allowing for a more accurate representation of the data.
While the ZIP model assumes variance equal to the mean, indicative of the Poisson distribution, the ZINB model relaxes this requirement, accommodating data with higher variability. This makes the ZINB an invaluable tool in fields like ecology and healthcare, where over-dispersion is common, and the presence of 'extra' zeros needs to be accounted for accurately.
Implementing Zero-Inflated Models in Statistics
Zero-inflated models have emerged as a strategic tool for tackling the analytical challenges posed by datasets characterised by an excess of zeros. The process of implementing these models into statistical analysis involves precise steps, from identifying the appropriate model based on the data's nature to confirming the presence of zero-inflation itself.These models are not just about managing data with an abundance of zeros but also about extracting meaningful insights that could otherwise be obscured due to the peculiar distribution of the data.
Steps to Construct a Zero-Inflated Regression Model
Constructing a zero-inflated regression model involves several systematic steps to ensure accurate results and insightful data interpretation:
- Identifying the Data Type: Determine if the data is count or binomial to choose between a Zero-Inflated Poisson (ZIP) model and a Zero-Inflated Binomial (ZIB) model.
- Data Segmentation: Segregate the zero and non-zero data points to analyse their distribution separately.
- Model Selection: Decide between Poisson, Negative Binomial, or Binomial models based on data dispersion.
- Parameter Estimation: Use statistical software to estimate the parameters for both the zero-inflation model and the count data model.
- Model Validation: Assess the model's fit through diagnostics like residual analysis and goodness-of-fit tests.
Example: Consider a health survey exploring factors affecting days absent from work due to sickness among employees. A high number of responses might be zero (no days absent), indicating potential zero-inflation. Through the steps described above, researchers can apply a zero-inflated model to distinguish between those never absent (structural zeros) and those who could have been absent but were not (sampling zeros).
Choosing Between Zero-Inflated Models: A Guide
Selecting the appropriate zero-inflated model is critical for achieving meaningful analytical results. The choice hinges on two main factors: the nature of the data (count or binomial) and its dispersion. A Zero-Inflated Poisson (ZIP) model is preferred for count data following a Poisson distribution with equal mean and variance. Conversely, for over-dispersed count data, where the variance exceeds the mean, a Zero-Inflated Negative Binomial (ZINB) model is more appropriate.For binomial data, a Zero-Inflated Binomial (ZIB) model should be considered. It’s pivotal to conduct an initial data analysis to determine the dispersion and distribution characteristics, guiding the selection of the correct zero-inflated model.
Consider using software packages known for handling count data, such as R or Python, which offer libraries specifically designed for zero-inflated models and can greatly simplify model selection and evaluation.
Detecting Zero-Inflation in Your Data
Detecting zero-inflation is an essential prerequisite before applying a zero-inflated model. This detection often relies on exploratory data analysis (EDA) and statistical tests. Looking at the distribution of the data can give an initial indication of zero-inflation. If the number of zeros exceeds what is expected under a conventional Poisson or binomial distribution, zero-inflation might be present.Statistical tests, such as Vuong's test, can offer more concrete evidence by comparing the fit of a zero-inflated model against a non-zero-inflated model. These methods collectively help in making informed decisions regarding the application of zero-inflated models.
For a more nuanced detection of zero-inflation, advanced diagnostic plots, like zero-inflation vs. non-zero inflation plot, can be utilised. These plots compare the distribution of observed zeros to the zeros expected by a given model, illuminating the presence and extent of zero-inflation. This combination of exploratory analysis and statistical testing forms a comprehensive approach to identifying zero-inflation in datasets.
Real-Life Applications of Zero-Inflated Models
Zero-inflated models have revolutionised the way researchers handle datasets with an abundance of zeroes, providing insights that would otherwise remain hidden. These models have found their niche across various fields, from healthcare and education to environmental science, proving their versatility and effectiveness.By appropriately modelling the excess zeros and distinguishing between different types of zero observations, zero-inflated models enable more accurate analyses and predictions, thus impacting decision-making and policy formulation in significant ways.
Zero-Inflated Models in Healthcare Studies
In healthcare research, zero-inflated models address the nuances of data where occurrences of a particular event, such as disease outbreaks or hospital readmissions, might be sparse. These models help in understanding patterns, identifying risk factors, and evaluating interventions by accurately accounting for the excess zeros in datasets.For instance, the number of hospital visits by patients with a rare disease might predominantly be zeros due to the low prevalence of the condition. Zero-inflated models can separate these observations into groups: those who never visited because they didn't need to (true zeroes) and those who didn't visit for other reasons (excess zeros), thus ensuring a more nuanced analysis of healthcare data.
Example: Monitoring asthma-related emergency department visits. Suppose an area has a high number of non-visits (zeros), which could be interpreted as either a sign of effective asthma control measures (true zeros) or lack of access to emergency services (excess zeros). A zero-inflated model would allow analysts to accurately distinguish between these possibilities, guiding healthcare providers in improving asthma management strategies.
Utilising Zero-Inflated Models in Educational Research
Education research often grapples with data on student engagement or achievement where not all students may participate in certain activities, leading to datasets with many zeros. Zero-inflated models are instrumental in deciphering these data patterns by differentiating between lack of engagement and opportunities to engage.Whether analysing the number of books read, math problems solved, or hours spent on homework, these models help educators understand the underlying reasons for zero participation, facilitating targeted interventions to improve student outcomes.
The use of zero-inflated models can reveal hidden subpopulations within educational data, such as distinguishing between students who do not participate due to lack of interest versus those who face barriers to participation.
The Role of Zero-Inflated Models in Environmental Science
Environmental science benefits from zero-inflated models, particularly in studies of species distribution, pollution levels, or climate change impacts where data may include a significant number of zeroes. These models contribute to a deeper understanding of environmental phenomena by accurately modelling occurrences of rare events and non-events.For example, in studying the distribution of a specific animal species, the zero-inflated model can differentiate between areas where the species is genuinely not present and areas where detection was not possible due to certain conditions, offering insights into habitat preferences and conservation needs.
An interesting application of zero-inflated models in environmental science is the analysis of air quality data. Cities with varying levels of pollution monitoring can have disparate data records, many showing zero or near-zero pollution levels. Zero-inflated models can help differentiate between times and places with genuinely good air quality (true zeros) and those where monitoring may not have been as effective or frequent (excess zeros). This distinction is crucial for accurately assessing air quality and implementing appropriate environmental policies.
Zero-Inflated Models - Key takeaways
- Zero-Inflated Models Definition: Statistical models that manage data sets with a high number of zero outcomes, ideal for count data with 'zero-inflation'.
- Zero Inflated Poisson Model (ZIP): Combines a logistic model with Poisson distribution for count data with excess zeros, distinguishing between 'structural zeros' (true zeros) and 'sampling zeros' (occurred by chance).
- Zero Inflated Binomial Model (ZIB): Adapts zero-inflated model principles to binomial distribution data, where there's an excessive number of trials with zero successes, using logistic and binomial regressions.
- Zero Inflated Negative Binomial Model (ZINB): Suits count data with high variance and deals with over-dispersion and excess zeros using a negative binomial distribution combined with modelling for zero-inflation.
- Implementation: Constructing a zero-inflated regression model involves identifying data type, segregating zeros, selecting the right model (Poisson, Negative Binomial, or Binomial), estimating parameters, and model validation through diagnostics.
Learn faster with the 0 flashcards about Zero-Inflated Models
Sign up for free to gain access to all our flashcards.
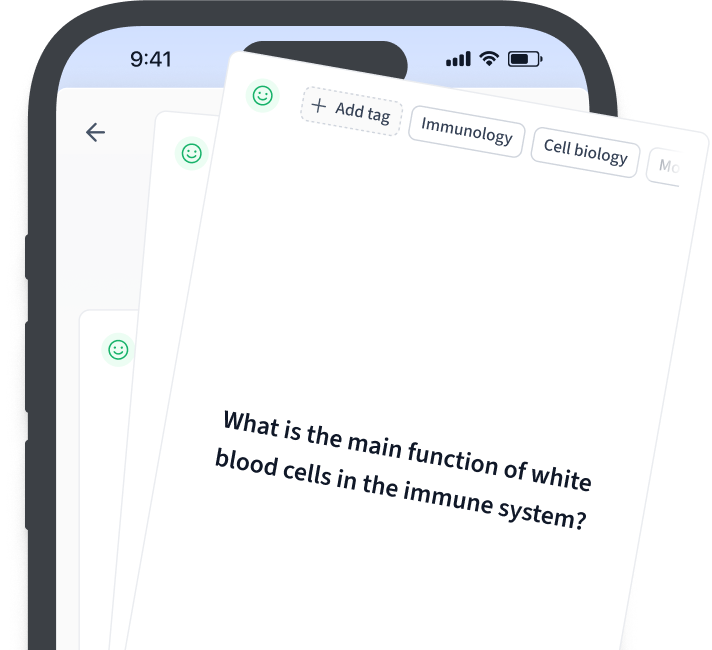
Frequently Asked Questions about Zero-Inflated Models
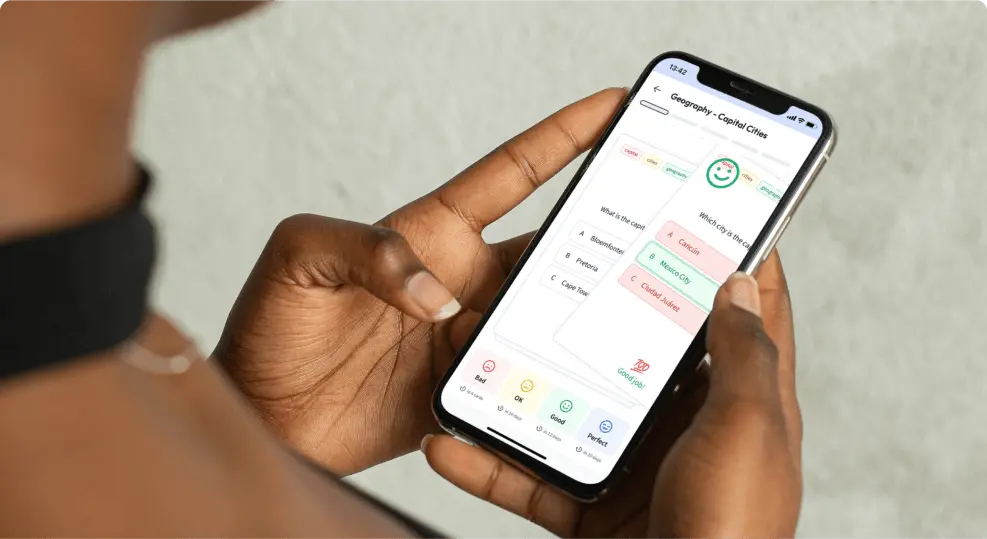
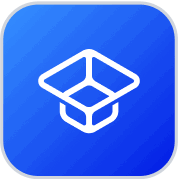
About StudySmarter
StudySmarter is a globally recognized educational technology company, offering a holistic learning platform designed for students of all ages and educational levels. Our platform provides learning support for a wide range of subjects, including STEM, Social Sciences, and Languages and also helps students to successfully master various tests and exams worldwide, such as GCSE, A Level, SAT, ACT, Abitur, and more. We offer an extensive library of learning materials, including interactive flashcards, comprehensive textbook solutions, and detailed explanations. The cutting-edge technology and tools we provide help students create their own learning materials. StudySmarter’s content is not only expert-verified but also regularly updated to ensure accuracy and relevance.
Learn more